+
+A lightweight benchmarking tool based inspired by [oha](https://github.com/hatoo/oha)
+and powered by [tui](https://github.com/tui-rs-revival/ratatui).
+
+## Install
+
+```shell
+make install-benchmark
+```
+
+## Run
+
+First, start `text-generation-inference`:
+
+```shell
+text-generation-launcher --model-id bigscience/bloom-560m
+```
+
+Then run the benchmarking tool:
+
+```shell
+text-generation-benchmark --tokenizer-name bigscience/bloom-560m
+```
diff --git a/benchmark/src/app.rs b/benchmark/src/app.rs
new file mode 100644
index 00000000..a0a9313a
--- /dev/null
+++ b/benchmark/src/app.rs
@@ -0,0 +1,692 @@
+/// Inspired by https://github.com/hatoo/oha/blob/bb989ea3cd77727e7743e7daa60a19894bb5e901/src/monitor.rs
+use crate::generation::{Decode, Message, Prefill};
+use crossterm::event::{KeyCode, KeyEvent, KeyModifiers};
+use text_generation_client::ClientError;
+use tokio::sync::mpsc;
+use tui::backend::Backend;
+use tui::layout::{Alignment, Constraint, Direction, Layout};
+use tui::style::{Color, Modifier, Style};
+use tui::text::{Line, Span};
+use tui::widgets::{
+ Axis, BarChart, Block, Borders, Chart, Dataset, Gauge, GraphType, Paragraph, Tabs,
+};
+use tui::{symbols, Frame};
+
+/// TUI powered App
+pub(crate) struct App {
+ pub(crate) running: bool,
+ pub(crate) data: Data,
+ completed_runs: Vec,
+ completed_batch: usize,
+ current_batch: usize,
+ current_tab: usize,
+ touched_tab: bool,
+ zoom: bool,
+ is_error: bool,
+ tokenizer_name: String,
+ sequence_length: u32,
+ decode_length: u32,
+ n_run: usize,
+ receiver: mpsc::Receiver>,
+}
+
+impl App {
+ pub(crate) fn new(
+ receiver: mpsc::Receiver>,
+ tokenizer_name: String,
+ sequence_length: u32,
+ decode_length: u32,
+ n_run: usize,
+ batch_size: Vec,
+ ) -> Self {
+ let current_tab = 0;
+
+ let completed_runs: Vec = (0..batch_size.len()).map(|_| 0).collect();
+ let completed_batch = 0;
+ let current_batch = 0;
+ let is_error = false;
+
+ let data = Data::new(n_run, batch_size);
+
+ Self {
+ running: true,
+ data,
+ completed_runs,
+ completed_batch,
+ current_batch,
+ current_tab,
+ touched_tab: false,
+ zoom: false,
+ is_error,
+ tokenizer_name,
+ sequence_length,
+ decode_length,
+ n_run,
+ receiver,
+ }
+ }
+
+ /// Handle crossterm key events
+ pub(crate) fn handle_key_event(&mut self, key_event: KeyEvent) {
+ match key_event {
+ // Increase and wrap tab
+ KeyEvent {
+ code: KeyCode::Right,
+ ..
+ }
+ | KeyEvent {
+ code: KeyCode::Tab, ..
+ } => {
+ self.touched_tab = true;
+ self.current_tab = (self.current_tab + 1) % self.data.batch_size.len();
+ }
+ // Decrease and wrap tab
+ KeyEvent {
+ code: KeyCode::Left,
+ ..
+ } => {
+ self.touched_tab = true;
+ if self.current_tab > 0 {
+ self.current_tab -= 1;
+ } else {
+ self.current_tab = self.data.batch_size.len() - 1;
+ }
+ }
+ // Zoom on throughput/latency fig
+ KeyEvent {
+ code: KeyCode::Char('+'),
+ ..
+ } => {
+ self.zoom = true;
+ }
+ // Unzoom on throughput/latency fig
+ KeyEvent {
+ code: KeyCode::Char('-'),
+ ..
+ } => {
+ self.zoom = false;
+ }
+ // Quit
+ KeyEvent {
+ code: KeyCode::Char('q'),
+ ..
+ }
+ | KeyEvent {
+ code: KeyCode::Char('c'),
+ modifiers: KeyModifiers::CONTROL,
+ ..
+ } => {
+ self.running = false;
+ }
+ _ => (),
+ }
+ }
+
+ /// Get all pending messages from generation task
+ pub(crate) fn tick(&mut self) {
+ while let Ok(message) = self.receiver.try_recv() {
+ match message {
+ Ok(message) => match message {
+ Message::Prefill(step) => self.data.push_prefill(step, self.current_batch),
+ Message::Decode(step) => self.data.push_decode(step, self.current_batch),
+ Message::EndRun => {
+ self.completed_runs[self.current_batch] += 1;
+ }
+ Message::EndBatch => {
+ self.data.end_batch(self.current_batch);
+ self.completed_batch += 1;
+
+ if self.current_batch < self.data.batch_size.len() - 1 {
+ // Only go to next tab if the user never touched the tab keys
+ if !self.touched_tab {
+ self.current_tab += 1;
+ }
+
+ self.current_batch += 1;
+ }
+ }
+ Message::Warmup => {}
+ },
+ Err(_) => self.is_error = true,
+ }
+ }
+ }
+
+ /// Render frame
+ pub fn render(&mut self, f: &mut Frame<'_, B>) {
+ let batch_progress =
+ (self.completed_batch as f64 / self.data.batch_size.len() as f64).clamp(0.0, 1.0);
+ let run_progress =
+ (self.completed_runs[self.current_batch] as f64 / self.n_run as f64).clamp(0.0, 1.0);
+
+ // Vertical layout
+ let row5 = Layout::default()
+ .direction(Direction::Vertical)
+ .constraints(
+ [
+ Constraint::Length(1),
+ Constraint::Length(3),
+ Constraint::Length(3),
+ Constraint::Length(13),
+ Constraint::Min(10),
+ ]
+ .as_ref(),
+ )
+ .split(f.size());
+
+ // Top row horizontal layout
+ let top = Layout::default()
+ .direction(Direction::Horizontal)
+ .constraints([Constraint::Percentage(50), Constraint::Percentage(50)].as_ref())
+ .split(row5[2]);
+
+ // Mid row horizontal layout
+ let mid = Layout::default()
+ .direction(Direction::Horizontal)
+ .constraints(
+ [
+ Constraint::Percentage(25),
+ Constraint::Percentage(25),
+ Constraint::Percentage(25),
+ Constraint::Percentage(25),
+ ]
+ .as_ref(),
+ )
+ .split(row5[3]);
+
+ // Left mid row vertical layout
+ let prefill_text = Layout::default()
+ .direction(Direction::Vertical)
+ .constraints([Constraint::Length(8), Constraint::Length(5)].as_ref())
+ .split(mid[0]);
+
+ // Right mid row vertical layout
+ let decode_text = Layout::default()
+ .direction(Direction::Vertical)
+ .constraints([Constraint::Length(8), Constraint::Length(5)].as_ref())
+ .split(mid[2]);
+ let decode_text_latency = Layout::default()
+ .direction(Direction::Horizontal)
+ .constraints([Constraint::Percentage(50), Constraint::Percentage(50)].as_ref())
+ .split(decode_text[0]);
+
+ // Bottom row horizontal layout
+ let bottom = Layout::default()
+ .direction(Direction::Horizontal)
+ .constraints([Constraint::Percentage(50), Constraint::Percentage(50)].as_ref())
+ .split(row5[4]);
+
+ // Title
+ let title = Block::default()
+ .borders(Borders::NONE)
+ .title(format!(
+ "Model: {} | Sequence Length: {} | Decode Length: {}",
+ self.tokenizer_name, self.sequence_length, self.decode_length
+ ))
+ .style(
+ Style::default()
+ .add_modifier(Modifier::BOLD)
+ .fg(Color::White),
+ );
+ f.render_widget(title, row5[0]);
+
+ // Helper
+ let helper = Block::default()
+ .borders(Borders::NONE)
+ .title("<- | tab | ->: change batch tab | q / CTRL + c: quit | +/-: zoom")
+ .title_alignment(Alignment::Right)
+ .style(Style::default().fg(Color::White));
+ f.render_widget(helper, row5[0]);
+
+ // Batch tabs
+ let titles = self
+ .data
+ .batch_size
+ .iter()
+ .map(|b| {
+ Line::from(vec![Span::styled(
+ format!("Batch: {b}"),
+ Style::default().fg(Color::White),
+ )])
+ })
+ .collect();
+ let tabs = Tabs::new(titles)
+ .block(Block::default().borders(Borders::ALL).title("Tabs"))
+ .select(self.current_tab)
+ .style(Style::default().fg(Color::LightCyan))
+ .highlight_style(
+ Style::default()
+ .add_modifier(Modifier::BOLD)
+ .bg(Color::Black),
+ );
+ f.render_widget(tabs, row5[1]);
+
+ // Total progress bar
+ let color = if self.is_error {
+ Color::Red
+ } else {
+ Color::LightGreen
+ };
+ let batch_gauge = progress_gauge(
+ "Total Progress",
+ format!("{} / {}", self.completed_batch, self.data.batch_size.len()),
+ batch_progress,
+ color,
+ );
+ f.render_widget(batch_gauge, top[0]);
+
+ // Batch progress Bar
+ let color = if self.is_error {
+ Color::Red
+ } else {
+ Color::LightBlue
+ };
+ let run_gauge = progress_gauge(
+ "Batch Progress",
+ format!(
+ "{} / {}",
+ self.completed_runs[self.current_batch], self.n_run
+ ),
+ run_progress,
+ color,
+ );
+ f.render_widget(run_gauge, top[1]);
+
+ // Prefill text infos
+ let prefill_latency_block = latency_paragraph(
+ &mut self.data.prefill_latencies[self.current_tab],
+ "Prefill",
+ );
+ let prefill_throughput_block =
+ throughput_paragraph(&self.data.prefill_throughputs[self.current_tab], "Prefill");
+
+ f.render_widget(prefill_latency_block, prefill_text[0]);
+ f.render_widget(prefill_throughput_block, prefill_text[1]);
+
+ // Prefill latency histogram
+ let histo_width = 7;
+ let bins = if mid[1].width < 2 {
+ 0
+ } else {
+ (mid[1].width as usize - 2) / (histo_width + 1)
+ }
+ .max(2);
+
+ let histo_data =
+ latency_histogram_data(&self.data.prefill_latencies[self.current_tab], bins);
+ let histo_data_str: Vec<(&str, u64)> =
+ histo_data.iter().map(|(l, v)| (l.as_str(), *v)).collect();
+ let prefill_histogram =
+ latency_histogram(&histo_data_str, "Prefill").bar_width(histo_width as u16);
+ f.render_widget(prefill_histogram, mid[1]);
+
+ // Decode text info
+ let decode_latency_block = latency_paragraph(
+ &mut self.data.decode_latencies[self.current_tab],
+ "Decode Total",
+ );
+ let decode_token_latency_block = latency_paragraph(
+ &mut self.data.decode_token_latencies[self.current_tab],
+ "Decode Token",
+ );
+ let decode_throughput_block =
+ throughput_paragraph(&self.data.decode_throughputs[self.current_tab], "Decode");
+ f.render_widget(decode_latency_block, decode_text_latency[0]);
+ f.render_widget(decode_token_latency_block, decode_text_latency[1]);
+ f.render_widget(decode_throughput_block, decode_text[1]);
+
+ // Decode latency histogram
+ let histo_data =
+ latency_histogram_data(&self.data.decode_latencies[self.current_tab], bins);
+ let histo_data_str: Vec<(&str, u64)> =
+ histo_data.iter().map(|(l, v)| (l.as_str(), *v)).collect();
+ let decode_histogram =
+ latency_histogram(&histo_data_str, "Decode").bar_width(histo_width as u16);
+ f.render_widget(decode_histogram, mid[3]);
+
+ // Prefill latency/throughput chart
+ let prefill_latency_throughput_chart = latency_throughput_chart(
+ &self.data.prefill_batch_latency_throughput,
+ &self.data.batch_size,
+ self.zoom,
+ "Prefill",
+ );
+ f.render_widget(prefill_latency_throughput_chart, bottom[0]);
+
+ // Decode latency/throughput chart
+ let decode_latency_throughput_chart = latency_throughput_chart(
+ &self.data.decode_batch_latency_throughput,
+ &self.data.batch_size,
+ self.zoom,
+ "Decode",
+ );
+ f.render_widget(decode_latency_throughput_chart, bottom[1]);
+ }
+}
+
+/// App internal data struct
+pub(crate) struct Data {
+ pub(crate) batch_size: Vec,
+ pub(crate) prefill_latencies: Vec>,
+ pub(crate) prefill_throughputs: Vec>,
+ pub(crate) decode_latencies: Vec>,
+ pub(crate) decode_token_latencies: Vec>,
+ pub(crate) decode_throughputs: Vec>,
+ pub(crate) prefill_batch_latency_throughput: Vec<(f64, f64)>,
+ pub(crate) decode_batch_latency_throughput: Vec<(f64, f64)>,
+}
+
+impl Data {
+ fn new(n_run: usize, batch_size: Vec) -> Self {
+ let prefill_latencies: Vec> = (0..batch_size.len())
+ .map(|_| Vec::with_capacity(n_run))
+ .collect();
+ let prefill_throughputs: Vec> = prefill_latencies.clone();
+
+ let decode_latencies: Vec> = prefill_latencies.clone();
+ let decode_token_latencies: Vec> = decode_latencies.clone();
+ let decode_throughputs: Vec> = prefill_throughputs.clone();
+
+ let prefill_batch_latency_throughput: Vec<(f64, f64)> =
+ Vec::with_capacity(batch_size.len());
+ let decode_batch_latency_throughput: Vec<(f64, f64)> =
+ prefill_batch_latency_throughput.clone();
+
+ Self {
+ batch_size,
+ prefill_latencies,
+ prefill_throughputs,
+ decode_latencies,
+ decode_token_latencies,
+ decode_throughputs,
+ prefill_batch_latency_throughput,
+ decode_batch_latency_throughput,
+ }
+ }
+
+ fn push_prefill(&mut self, prefill: Prefill, batch_idx: usize) {
+ let latency = prefill.latency.as_micros() as f64 / 1000.0;
+ self.prefill_latencies[batch_idx].push(latency);
+ self.prefill_throughputs[batch_idx].push(prefill.throughput);
+ }
+
+ fn push_decode(&mut self, decode: Decode, batch_idx: usize) {
+ let latency = decode.latency.as_micros() as f64 / 1000.0;
+ let token_latency = decode.token_latency.as_micros() as f64 / 1000.0;
+ self.decode_latencies[batch_idx].push(latency);
+ self.decode_token_latencies[batch_idx].push(token_latency);
+ self.decode_throughputs[batch_idx].push(decode.throughput);
+ }
+
+ fn end_batch(&mut self, batch_idx: usize) {
+ self.prefill_batch_latency_throughput.push((
+ self.prefill_latencies[batch_idx].iter().sum::()
+ / self.prefill_latencies[batch_idx].len() as f64,
+ self.prefill_throughputs[batch_idx].iter().sum::()
+ / self.prefill_throughputs[batch_idx].len() as f64,
+ ));
+ self.decode_batch_latency_throughput.push((
+ self.decode_latencies[batch_idx].iter().sum::()
+ / self.decode_latencies[batch_idx].len() as f64,
+ self.decode_throughputs[batch_idx].iter().sum::()
+ / self.decode_throughputs[batch_idx].len() as f64,
+ ));
+ }
+}
+
+/// Progress bar
+fn progress_gauge(title: &str, label: String, progress: f64, color: Color) -> Gauge {
+ Gauge::default()
+ .block(Block::default().title(title).borders(Borders::ALL))
+ .gauge_style(Style::default().fg(color))
+ .label(Span::raw(label))
+ .ratio(progress)
+}
+
+/// Throughput paragraph
+fn throughput_paragraph<'a>(throughput: &[f64], name: &'static str) -> Paragraph<'a> {
+ // Throughput average/high/low texts
+ let throughput_texts = statis_spans(throughput, "tokens/secs");
+
+ // Throughput block
+ Paragraph::new(throughput_texts).block(
+ Block::default()
+ .title(Span::raw(format!("{name} Throughput")))
+ .borders(Borders::ALL),
+ )
+}
+
+/// Latency paragraph
+fn latency_paragraph<'a>(latency: &mut [f64], name: &'static str) -> Paragraph<'a> {
+ // Latency average/high/low texts
+ let mut latency_texts = statis_spans(latency, "ms");
+
+ // Sort latency for percentiles
+ float_ord::sort(latency);
+ let latency_percentiles = crate::utils::percentiles(latency, &[50, 90, 99]);
+
+ // Latency p50/p90/p99 texts
+ let colors = [Color::LightGreen, Color::LightYellow, Color::LightRed];
+ for (i, (name, value)) in latency_percentiles.iter().enumerate() {
+ let span = Line::from(vec![Span::styled(
+ format!("{name}: {value:.2} ms"),
+ Style::default().fg(colors[i]),
+ )]);
+ latency_texts.push(span);
+ }
+
+ Paragraph::new(latency_texts).block(
+ Block::default()
+ .title(Span::raw(format!("{name} Latency")))
+ .borders(Borders::ALL),
+ )
+}
+
+/// Average/High/Low spans
+fn statis_spans<'a>(data: &[f64], unit: &'static str) -> Vec> {
+ vec![
+ Line::from(vec![Span::styled(
+ format!(
+ "Average: {:.2} {unit}",
+ data.iter().sum::() / data.len() as f64
+ ),
+ Style::default().fg(Color::LightBlue),
+ )]),
+ Line::from(vec![Span::styled(
+ format!(
+ "Lowest: {:.2} {unit}",
+ data.iter()
+ .min_by(|a, b| a.total_cmp(b))
+ .unwrap_or(&f64::NAN)
+ ),
+ Style::default().fg(Color::Reset),
+ )]),
+ Line::from(vec![Span::styled(
+ format!(
+ "Highest: {:.2} {unit}",
+ data.iter()
+ .max_by(|a, b| a.total_cmp(b))
+ .unwrap_or(&f64::NAN)
+ ),
+ Style::default().fg(Color::Reset),
+ )]),
+ ]
+}
+
+/// Latency histogram data
+fn latency_histogram_data(latency: &[f64], bins: usize) -> Vec<(String, u64)> {
+ let histo_data: Vec<(String, u64)> = {
+ let histo = crate::utils::histogram(latency, bins);
+ histo
+ .into_iter()
+ .map(|(label, v)| (format!("{label:.2}"), v as u64))
+ .collect()
+ };
+
+ histo_data
+}
+
+/// Latency Histogram
+fn latency_histogram<'a>(
+ histo_data_str: &'a Vec<(&'a str, u64)>,
+ name: &'static str,
+) -> BarChart<'a> {
+ BarChart::default()
+ .block(
+ Block::default()
+ .title(format!("{name} latency histogram"))
+ .style(Style::default().fg(Color::LightYellow).bg(Color::Reset))
+ .borders(Borders::ALL),
+ )
+ .data(histo_data_str.as_slice())
+}
+
+/// Latency/Throughput chart
+fn latency_throughput_chart<'a>(
+ latency_throughput: &'a [(f64, f64)],
+ batch_sizes: &'a [u32],
+ zoom: bool,
+ name: &'static str,
+) -> Chart<'a> {
+ let latency_iter = latency_throughput.iter().map(|(l, _)| l);
+ let throughput_iter = latency_throughput.iter().map(|(_, t)| t);
+
+ // Get extreme values
+ let min_latency: f64 = *latency_iter
+ .clone()
+ .min_by(|a, b| a.total_cmp(b))
+ .unwrap_or(&f64::NAN);
+ let max_latency: f64 = *latency_iter
+ .max_by(|a, b| a.total_cmp(b))
+ .unwrap_or(&f64::NAN);
+ let min_throughput: f64 = *throughput_iter
+ .clone()
+ .min_by(|a, b| a.total_cmp(b))
+ .unwrap_or(&f64::NAN);
+ let max_throughput: f64 = *throughput_iter
+ .max_by(|a, b| a.total_cmp(b))
+ .unwrap_or(&f64::NAN);
+
+ // Char min max values
+ let min_x = if zoom {
+ ((min_latency - 0.05 * min_latency) / 100.0).floor() * 100.0
+ } else {
+ 0.0
+ };
+ let max_x = ((max_latency + 0.05 * max_latency) / 100.0).ceil() * 100.0;
+ let step_x = (max_x - min_x) / 4.0;
+
+ // Chart min max values
+ let min_y = if zoom {
+ ((min_throughput - 0.05 * min_throughput) / 100.0).floor() * 100.0
+ } else {
+ 0.0
+ };
+ let max_y = ((max_throughput + 0.05 * max_throughput) / 100.0).ceil() * 100.0;
+ let step_y = (max_y - min_y) / 4.0;
+
+ // Labels
+ let mut x_labels = vec![Span::styled(
+ format!("{min_x:.2}"),
+ Style::default()
+ .add_modifier(Modifier::BOLD)
+ .fg(Color::Gray)
+ .bg(Color::Reset),
+ )];
+ for i in 0..3 {
+ x_labels.push(Span::styled(
+ format!("{:.2}", min_x + ((i + 1) as f64 * step_x)),
+ Style::default().fg(Color::Gray).bg(Color::Reset),
+ ));
+ }
+ x_labels.push(Span::styled(
+ format!("{max_x:.2}"),
+ Style::default()
+ .add_modifier(Modifier::BOLD)
+ .fg(Color::Gray)
+ .bg(Color::Reset),
+ ));
+
+ // Labels
+ let mut y_labels = vec![Span::styled(
+ format!("{min_y:.2}"),
+ Style::default()
+ .add_modifier(Modifier::BOLD)
+ .fg(Color::Gray)
+ .bg(Color::Reset),
+ )];
+ for i in 0..3 {
+ y_labels.push(Span::styled(
+ format!("{:.2}", min_y + ((i + 1) as f64 * step_y)),
+ Style::default().fg(Color::Gray).bg(Color::Reset),
+ ));
+ }
+ y_labels.push(Span::styled(
+ format!("{max_y:.2}"),
+ Style::default()
+ .add_modifier(Modifier::BOLD)
+ .fg(Color::Gray)
+ .bg(Color::Reset),
+ ));
+
+ // Chart dataset
+ let colors = color_vec();
+ let datasets: Vec = (0..latency_throughput.len())
+ .map(|i| {
+ let color_idx = i % colors.len();
+
+ Dataset::default()
+ .name(batch_sizes[i].to_string())
+ .marker(symbols::Marker::Block)
+ .style(Style::default().fg(colors[color_idx]))
+ .graph_type(GraphType::Scatter)
+ .data(&latency_throughput[i..(i + 1)])
+ })
+ .collect();
+
+ // Chart
+ Chart::new(datasets)
+ .style(Style::default().fg(Color::Cyan).bg(Color::Reset))
+ .block(
+ Block::default()
+ .title(Span::styled(
+ format!("{name} throughput over latency"),
+ Style::default().fg(Color::Gray).bg(Color::Reset),
+ ))
+ .borders(Borders::ALL),
+ )
+ .x_axis(
+ Axis::default()
+ .title("ms")
+ .style(Style::default().fg(Color::Gray).bg(Color::Reset))
+ .labels(x_labels)
+ .bounds([min_x, max_x]),
+ )
+ .y_axis(
+ Axis::default()
+ .title("tokens/secs")
+ .style(Style::default().fg(Color::Gray).bg(Color::Reset))
+ .labels(y_labels)
+ .bounds([min_y, max_y]),
+ )
+}
+
+// Colors for latency/throughput chart
+fn color_vec() -> Vec {
+ vec![
+ Color::Red,
+ Color::Green,
+ Color::Yellow,
+ Color::Blue,
+ Color::Magenta,
+ Color::Cyan,
+ Color::Gray,
+ Color::DarkGray,
+ Color::LightRed,
+ Color::LightGreen,
+ Color::LightYellow,
+ Color::LightBlue,
+ Color::LightMagenta,
+ Color::LightCyan,
+ ]
+}
diff --git a/benchmark/src/event.rs b/benchmark/src/event.rs
new file mode 100644
index 00000000..07482aed
--- /dev/null
+++ b/benchmark/src/event.rs
@@ -0,0 +1,65 @@
+/// Inspired by https://github.com/orhun/rust-tui-template/blob/472aa515119d4c94903eac12d9784417281dc7f5/src/event.rs
+use crossterm::event;
+use std::time::{Duration, Instant};
+use tokio::sync::{broadcast, mpsc};
+
+/// Events
+#[derive(Debug)]
+pub(crate) enum Event {
+ /// Terminal tick.
+ Tick,
+ /// Key press.
+ Key(event::KeyEvent),
+ /// Terminal resize.
+ Resize,
+}
+
+pub(crate) async fn terminal_event_task(
+ fps: u32,
+ event_sender: mpsc::Sender,
+ mut shutdown_receiver: broadcast::Receiver<()>,
+ _shutdown_guard_sender: mpsc::Sender<()>,
+) {
+ // End task if a message is received on shutdown_receiver
+ // _shutdown_guard_sender will be dropped once the task is finished
+ tokio::select! {
+ _ = event_loop(fps, event_sender) => {
+ },
+ _ = shutdown_receiver.recv() => {}
+ }
+}
+
+/// Main event loop
+async fn event_loop(fps: u32, event_sender: mpsc::Sender) {
+ // Frame budget
+ let per_frame = Duration::from_secs(1) / fps;
+
+ // When was last frame executed
+ let mut last_frame = Instant::now();
+
+ loop {
+ // Sleep to avoid blocking the thread for too long
+ if let Some(sleep) = per_frame.checked_sub(last_frame.elapsed()) {
+ tokio::time::sleep(sleep).await;
+ }
+
+ // Get crossterm event and send a new one over the channel
+ if event::poll(Duration::from_secs(0)).expect("no events available") {
+ match event::read().expect("unable to read event") {
+ event::Event::Key(e) => event_sender.send(Event::Key(e)).await.unwrap_or(()),
+ event::Event::Resize(_w, _h) => {
+ event_sender.send(Event::Resize).await.unwrap_or(())
+ }
+ _ => (),
+ }
+ }
+
+ // Frame budget exceeded
+ if last_frame.elapsed() >= per_frame {
+ // Send tick
+ event_sender.send(Event::Tick).await.unwrap_or(());
+ // Rest last_frame time
+ last_frame = Instant::now();
+ }
+ }
+}
diff --git a/benchmark/src/generation.rs b/benchmark/src/generation.rs
new file mode 100644
index 00000000..5135f02f
--- /dev/null
+++ b/benchmark/src/generation.rs
@@ -0,0 +1,228 @@
+use std::time::{Duration, Instant};
+use text_generation_client::{
+ Batch, CachedBatch, ClientError, NextTokenChooserParameters, Request, ShardedClient,
+ StoppingCriteriaParameters,
+};
+use tokenizers::{Tokenizer, TruncationDirection};
+use tokio::sync::{broadcast, mpsc};
+
+const LOREM_IPSUM: &str = "Lorem ipsum dolor sit amet, consectetur adipiscing elit, sed do eiusmod tempor incididunt ut labore et dolore magna aliqua. Ut enim ad minim veniam, quis nostrud exercitation ullamco laboris nisi ut aliquip ex ea commodo consequat. Duis aute irure dolor in reprehenderit in voluptate velit esse cillum dolore eu fugiat nulla pariatur. Excepteur sint occaecat cupidatat non proident, sunt in culpa qui officia deserunt mollit anim id est laborum.";
+
+#[derive(Debug, Clone)]
+pub(crate) struct Prefill {
+ pub(crate) latency: Duration,
+ pub(crate) throughput: f64,
+}
+
+#[derive(Debug, Clone)]
+pub(crate) struct Decode {
+ pub(crate) latency: Duration,
+ pub(crate) token_latency: Duration,
+ pub(crate) throughput: f64,
+}
+
+#[derive(Debug)]
+pub(crate) enum Message {
+ Warmup,
+ Prefill(Prefill),
+ Decode(Decode),
+ EndRun,
+ EndBatch,
+}
+
+/// Benchmarking task
+#[allow(clippy::too_many_arguments)]
+pub(crate) async fn generation_task(
+ tokenizer: Tokenizer,
+ batch_size: Vec,
+ sequence_length: u32,
+ decode_length: u32,
+ top_n_tokens: Option,
+ n_runs: usize,
+ warmups: usize,
+ parameters: NextTokenChooserParameters,
+ client: ShardedClient,
+ run_sender: mpsc::Sender>,
+ mut shutdown_receiver: broadcast::Receiver<()>,
+ _shutdown_guard_sender: mpsc::Sender<()>,
+) {
+ // End task if a message is received on shutdown_receiver
+ // _shutdown_guard_sender will be dropped once the task is finished
+ tokio::select! {
+ res = generate_runs(tokenizer, batch_size, sequence_length, decode_length, top_n_tokens, n_runs, warmups, parameters, client, run_sender.clone()) => {
+ if let Err(err) = res {
+ run_sender.send(Err(err)).await.unwrap_or(());
+ }
+ },
+ _ = shutdown_receiver.recv() => {}
+ }
+}
+
+/// Benchmark prefill/decode
+#[allow(clippy::too_many_arguments)]
+async fn generate_runs(
+ tokenizer: Tokenizer,
+ batch_size: Vec,
+ sequence_length: u32,
+ decode_length: u32,
+ top_n_tokens: Option,
+ n_runs: usize,
+ warmups: usize,
+ parameters: NextTokenChooserParameters,
+ mut client: ShardedClient,
+ run_sender: mpsc::Sender>,
+) -> Result<(), ClientError> {
+ // Create a dummy sequence
+ let sequence = create_sequence(sequence_length, tokenizer);
+
+ for b in batch_size {
+ // Warmups on batch size
+ for _ in 0..warmups {
+ let (_, decode_batch) = prefill(
+ sequence.clone(),
+ sequence_length,
+ b,
+ decode_length,
+ parameters.clone(),
+ top_n_tokens,
+ &mut client,
+ )
+ .await?;
+ let _ = decode(decode_batch, &mut client).await?;
+ // Send warmup message
+ run_sender.send(Ok(Message::Warmup)).await.unwrap_or(());
+ }
+
+ for _ in 0..n_runs {
+ let (prefill, decode_batch) = prefill(
+ sequence.clone(),
+ sequence_length,
+ b,
+ decode_length,
+ parameters.clone(),
+ top_n_tokens,
+ &mut client,
+ )
+ .await?;
+ // Send prefill message
+ run_sender
+ .send(Ok(Message::Prefill(prefill)))
+ .await
+ .unwrap_or(());
+
+ let decode = decode(decode_batch, &mut client).await?;
+
+ // Send decode message
+ run_sender
+ .send(Ok(Message::Decode(decode)))
+ .await
+ .unwrap_or(());
+
+ // Send run ended message
+ run_sender.send(Ok(Message::EndRun)).await.unwrap_or(());
+ }
+ // Batch ended
+ run_sender.send(Ok(Message::EndBatch)).await.unwrap_or(());
+ }
+ Ok(())
+}
+
+// Run a prefill step
+async fn prefill(
+ sequence: String,
+ sequence_length: u32,
+ batch_size: u32,
+ decode_length: u32,
+ parameters: NextTokenChooserParameters,
+ top_n_tokens: Option,
+ client: &mut ShardedClient,
+) -> Result<(Prefill, CachedBatch), ClientError> {
+ // Create requests
+ let requests = (0..batch_size)
+ .map(|id| Request {
+ id: id.into(),
+ prefill_logprobs: false,
+ inputs: sequence.clone(),
+ truncate: sequence_length,
+ parameters: Some(parameters.clone()),
+ stopping_parameters: Some(StoppingCriteriaParameters {
+ max_new_tokens: decode_length,
+ stop_sequences: vec![],
+ ignore_eos_token: true, // Will not stop even if a eos token is generated
+ }),
+ top_n_tokens: top_n_tokens.unwrap_or(0),
+ lora_id: None,
+ })
+ .collect();
+
+ let batch = Batch {
+ id: 0,
+ requests,
+ size: batch_size,
+ max_tokens: batch_size * (sequence_length + decode_length),
+ };
+
+ // Run prefill
+ let start_time = Instant::now();
+ let (_, decode_batch, _) = client.prefill(batch.clone()).await?;
+
+ // Get latency
+ let latency = start_time.elapsed();
+
+ // Compute throughput from latency and batch size
+ let throughput = batch_size as f64 / latency.as_secs_f64();
+
+ // Decode batch cannot be empty
+ let decode_batch = decode_batch.expect("decode_batch is None. This is a bug.");
+
+ let step = Prefill {
+ latency,
+ throughput,
+ };
+
+ Ok((step, decode_batch))
+}
+
+/// Run a full decode
+async fn decode(batch: CachedBatch, client: &mut ShardedClient) -> Result {
+ let mut decode_length = 0;
+ let batch_size = batch.size;
+
+ let start_time = Instant::now();
+
+ // Full decode over decode length
+ let mut next_batch = Some(batch);
+ while let Some(batch) = next_batch {
+ let result = client.decode(vec![batch]).await?;
+ next_batch = result.1;
+ decode_length += 1;
+ }
+
+ // Get latency
+ let latency = start_time.elapsed();
+ let token_latency = latency / decode_length;
+
+ // Compute throughput from latency, batch size and decode length
+ let throughput = (batch_size * decode_length) as f64 / latency.as_secs_f64();
+
+ let step = Decode {
+ latency,
+ token_latency,
+ throughput,
+ };
+ Ok(step)
+}
+
+/// Create a dummy sequence of the correct length
+fn create_sequence(sequence_length: u32, tokenizer: Tokenizer) -> String {
+ let lorem_ipsum_length = tokenizer.encode(LOREM_IPSUM, true).unwrap().len();
+ // Repeat lorem ipsum to cover sequence length
+ let string_sequence =
+ LOREM_IPSUM.repeat((0..sequence_length).step_by(lorem_ipsum_length).len());
+ // Encode sequence
+ let mut encoding = tokenizer.encode(string_sequence, true).unwrap();
+ // Truncate to sequence_length
+ encoding.truncate(sequence_length as usize, 0, TruncationDirection::Left);
+ // Decode
+ tokenizer.decode(encoding.get_ids(), false).unwrap()
+}
diff --git a/benchmark/src/lib.rs b/benchmark/src/lib.rs
new file mode 100644
index 00000000..638c6514
--- /dev/null
+++ b/benchmark/src/lib.rs
@@ -0,0 +1,160 @@
+mod app;
+mod event;
+mod generation;
+mod table;
+mod utils;
+
+use crate::app::App;
+use crate::event::Event;
+use crossterm::ExecutableCommand;
+use std::io;
+use text_generation_client::{GrammarType, NextTokenChooserParameters, ShardedClient};
+use tokenizers::Tokenizer;
+use tokio::sync::{broadcast, mpsc};
+use tui::backend::CrosstermBackend;
+use tui::Terminal;
+
+/// Run benchmarking app
+#[allow(clippy::too_many_arguments)]
+pub async fn run(
+ tokenizer_name: String,
+ tokenizer: Tokenizer,
+ batch_size: Vec,
+ sequence_length: u32,
+ decode_length: u32,
+ top_n_tokens: Option,
+ n_runs: usize,
+ warmups: usize,
+ temperature: Option,
+ top_k: Option,
+ top_p: Option,
+ typical_p: Option,
+ repetition_penalty: Option,
+ frequency_penalty: Option,
+ watermark: bool,
+ do_sample: bool,
+ client: ShardedClient,
+) -> Result<(), std::io::Error> {
+ let parameters = NextTokenChooserParameters {
+ temperature: temperature.unwrap_or(1.0),
+ top_k: top_k.unwrap_or(0),
+ top_p: top_p.unwrap_or(1.0),
+ typical_p: typical_p.unwrap_or(1.0),
+ do_sample,
+ seed: 0,
+ repetition_penalty: repetition_penalty.unwrap_or(1.0),
+ frequency_penalty: frequency_penalty.unwrap_or(0.0),
+ watermark,
+ grammar: String::new(),
+ grammar_type: GrammarType::None as i32,
+ };
+
+ // Initialize terminal properties
+ crossterm::terminal::enable_raw_mode()?;
+ io::stdout().execute(crossterm::terminal::EnterAlternateScreen)?;
+ io::stdout().execute(crossterm::cursor::Hide)?;
+
+ // Initialize terminal
+ let mut terminal = {
+ let backend = CrosstermBackend::new(io::stdout());
+ Terminal::new(backend)?
+ };
+
+ // Create message channel between generation_task and app
+ let (run_sender, run_receiver) = mpsc::channel(8);
+ // Crossterm event channel
+ let (event_sender, mut event_receiver) = mpsc::channel(8);
+ // Shutdown channel to terminate tasks
+ let (shutdown_sender, _) = broadcast::channel(1);
+ // Channel to check if tasks terminated
+ let (shutdown_guard_sender, mut shutdown_guard_receiver) = mpsc::channel(1);
+
+ // Create generation task
+ tokio::spawn(generation::generation_task(
+ tokenizer,
+ batch_size.clone(),
+ sequence_length,
+ decode_length,
+ top_n_tokens,
+ n_runs,
+ warmups,
+ parameters,
+ client,
+ run_sender,
+ shutdown_sender.subscribe(),
+ shutdown_guard_sender.clone(),
+ ));
+
+ // Create event task
+ tokio::spawn(event::terminal_event_task(
+ 250,
+ event_sender,
+ shutdown_sender.subscribe(),
+ shutdown_guard_sender.clone(),
+ ));
+
+ // Drop our end of shutdown sender
+ drop(shutdown_guard_sender);
+
+ // Create App
+ let mut app = App::new(
+ run_receiver,
+ tokenizer_name.clone(),
+ sequence_length,
+ decode_length,
+ n_runs,
+ batch_size,
+ );
+
+ while app.running {
+ // Draw frame
+ terminal.draw(|frame| app.render(frame))?;
+
+ // Await a new event from event handling task
+ match event_receiver.recv().await {
+ None => break,
+ // Update app state
+ Some(event) => match event {
+ Event::Tick => app.tick(),
+ Event::Key(key_event) => app.handle_key_event(key_event),
+ _ => {}
+ },
+ }
+ }
+
+ // Ask tasks to shutdown
+ let _ = shutdown_sender.send(());
+ // Wait for tasks to shutdown
+ let _ = shutdown_guard_receiver.recv().await;
+
+ // Revert terminal to original view
+ io::stdout().execute(crossterm::terminal::LeaveAlternateScreen)?;
+ crossterm::terminal::disable_raw_mode()?;
+ io::stdout().execute(crossterm::cursor::Show)?;
+
+ let parameters_table = table::parameters_table(
+ tokenizer_name,
+ sequence_length,
+ decode_length,
+ top_n_tokens,
+ n_runs,
+ warmups,
+ temperature,
+ top_k,
+ top_p,
+ typical_p,
+ repetition_penalty,
+ frequency_penalty,
+ watermark,
+ do_sample,
+ );
+ println!("\n{parameters_table}\n");
+
+ let latency_table = table::latency_table(&app.data);
+ println!("\n{latency_table}\n");
+
+ let throughput_table = table::throughput_table(&app.data);
+ println!("\n{throughput_table}\n");
+
+ Ok(())
+}
diff --git a/benchmark/src/main.rs b/benchmark/src/main.rs
new file mode 100644
index 00000000..2d89e045
--- /dev/null
+++ b/benchmark/src/main.rs
@@ -0,0 +1,222 @@
+/// Text Generation Inference benchmarking tool
+///
+/// Inspired by the great Oha app: https://github.com/hatoo/oha
+/// and: https://github.com/orhun/rust-tui-template
+use clap::Parser;
+use std::path::Path;
+use text_generation_client::ShardedClient;
+use tokenizers::{FromPretrainedParameters, Tokenizer};
+use tracing_subscriber::layer::SubscriberExt;
+use tracing_subscriber::util::SubscriberInitExt;
+use tracing_subscriber::EnvFilter;
+
+/// App Configuration
+#[derive(Parser, Debug)]
+#[clap(author, version, about, long_about = None)]
+struct Args {
+ /// The name of the tokenizer (as in model_id on the huggingface hub, or local path).
+ #[clap(short, long, env)]
+ tokenizer_name: String,
+
+ /// The revision to use for the tokenizer if on the hub.
+ #[clap(default_value = "main", long, env)]
+ revision: String,
+
+ /// The various batch sizes to benchmark for, the idea is to get enough
+ /// batching to start seeing increased latency, this usually means you're
+ /// moving from memory bound (usual as BS=1) to compute bound, and this is
+ /// a sweet spot for the maximum batch size for the model under test
+ #[clap(short, long)]
+ batch_size: Option>,
+
+ /// This is the initial prompt sent to the text-generation-server length
+ /// in token. Longer prompt will slow down the benchmark. Usually the
+ /// latency grows somewhat linearly with this for the prefill step.
+ ///
+ /// Most importantly, the prefill step is usually not the one dominating
+ /// your runtime, so it's ok to keep it short.
+ #[clap(default_value = "10", short, long, env)]
+ sequence_length: u32,
+
+ /// This is how many tokens will be generated by the server and averaged out
+ /// to give the `decode` latency. This is the *critical* number you want to optimize for
+ /// LLM spend most of their time doing decoding.
+ ///
+ /// Decode latency is usually quite stable.
+ #[clap(default_value = "8", short, long, env)]
+ decode_length: u32,
+
+ ///How many runs should we average from
+ #[clap(default_value = "10", short, long, env)]
+ runs: usize,
+
+ /// Number of warmup cycles
+ #[clap(default_value = "1", short, long, env)]
+ warmups: usize,
+
+ /// The location of the grpc socket. This benchmark tool bypasses the router
+ /// completely and directly talks to the gRPC processes
+ #[clap(default_value = "/tmp/text-generation-server-0", short, long, env)]
+ master_shard_uds_path: String,
+
+ /// Generation parameter in case you want to specifically test/debug particular
+ /// decoding strategies, for full doc refer to the `text-generation-server`
+ #[clap(long, env)]
+ temperature: Option,
+
+ /// Generation parameter in case you want to specifically test/debug particular
+ /// decoding strategies, for full doc refer to the `text-generation-server`
+ #[clap(long, env)]
+ top_k: Option,
+
+ /// Generation parameter in case you want to specifically test/debug particular
+ /// decoding strategies, for full doc refer to the `text-generation-server`
+ #[clap(long, env)]
+ top_p: Option,
+
+ /// Generation parameter in case you want to specifically test/debug particular
+ /// decoding strategies, for full doc refer to the `text-generation-server`
+ #[clap(long, env)]
+ typical_p: Option,
+
+ /// Generation parameter in case you want to specifically test/debug particular
+ /// decoding strategies, for full doc refer to the `text-generation-server`
+ #[clap(long, env)]
+ repetition_penalty: Option,
+
+ /// Generation parameter in case you want to specifically test/debug particular
+ /// decoding strategies, for full doc refer to the `text-generation-server`
+ #[clap(long, env)]
+ frequency_penalty: Option,
+
+ /// Generation parameter in case you want to specifically test/debug particular
+ /// decoding strategies, for full doc refer to the `text-generation-server`
+ #[clap(long, env)]
+ watermark: bool,
+
+ /// Generation parameter in case you want to specifically test/debug particular
+ /// decoding strategies, for full doc refer to the `text-generation-server`
+ #[clap(long, env)]
+ do_sample: bool,
+
+ /// Generation parameter in case you want to specifically test/debug particular
+ /// decoding strategies, for full doc refer to the `text-generation-server`
+ #[clap(long, env)]
+ top_n_tokens: Option,
+}
+
+fn main() -> Result<(), Box> {
+ init_logging();
+
+ // Get args
+ let args = Args::parse();
+ // Pattern match configuration
+ let Args {
+ tokenizer_name,
+ revision,
+ batch_size,
+ sequence_length,
+ decode_length,
+ runs,
+ warmups,
+ temperature,
+ top_k,
+ top_p,
+ typical_p,
+ repetition_penalty,
+ frequency_penalty,
+ watermark,
+ do_sample,
+ master_shard_uds_path,
+ top_n_tokens,
+ } = args;
+
+ let batch_size = batch_size.unwrap_or(vec![1, 2, 4, 8, 16, 32]);
+
+ // Tokenizer instance
+ // This will only be used to validate payloads
+ tracing::info!("Loading tokenizer");
+ let local_path = Path::new(&tokenizer_name);
+ let tokenizer =
+ if local_path.exists() && local_path.is_dir() && local_path.join("tokenizer.json").exists()
+ {
+ // Load local tokenizer
+ tracing::info!("Found local tokenizer");
+ Tokenizer::from_file(local_path.join("tokenizer.json")).unwrap()
+ } else {
+ tracing::info!("Downloading tokenizer");
+
+ // Parse Huggingface hub token
+ let auth_token = std::env::var("HUGGING_FACE_HUB_TOKEN").ok();
+
+ // Download and instantiate tokenizer
+ // We need to download it outside of the Tokio runtime
+ let params = FromPretrainedParameters {
+ revision,
+ auth_token,
+ ..Default::default()
+ };
+ Tokenizer::from_pretrained(tokenizer_name.clone(), Some(params)).unwrap()
+ };
+ tracing::info!("Tokenizer loaded");
+
+ // Launch Tokio runtime
+ tokio::runtime::Builder::new_multi_thread()
+ .enable_all()
+ .build()
+ .unwrap()
+ .block_on(async {
+ // Instantiate sharded client from the master unix socket
+ tracing::info!("Connect to model server");
+ let mut sharded_client = ShardedClient::connect_uds(master_shard_uds_path)
+ .await
+ .expect("Could not connect to server");
+ // Clear the cache; useful if the webserver rebooted
+ sharded_client
+ .clear_cache(None)
+ .await
+ .expect("Unable to clear cache");
+ tracing::info!("Connected");
+
+ // Run app
+ text_generation_benchmark::run(
+ tokenizer_name,
+ tokenizer,
+ batch_size,
+ sequence_length,
+ decode_length,
+ top_n_tokens,
+ runs,
+ warmups,
+ temperature,
+ top_k,
+ top_p,
+ typical_p,
+ repetition_penalty,
+ frequency_penalty,
+ watermark,
+ do_sample,
+ sharded_client,
+ )
+ .await
+ .unwrap();
+ });
+ Ok(())
+}
+
+/// Init logging using LOG_LEVEL
+fn init_logging() {
+ // STDOUT/STDERR layer
+ let fmt_layer = tracing_subscriber::fmt::layer()
+ .with_file(true)
+ .with_line_number(true);
+
+ // Filter events with LOG_LEVEL
+ let env_filter =
+ EnvFilter::try_from_env("LOG_LEVEL").unwrap_or_else(|_| EnvFilter::new("info"));
+
+ tracing_subscriber::registry()
+ .with(env_filter)
+ .with(fmt_layer)
+ .init();
+}
diff --git a/benchmark/src/table.rs b/benchmark/src/table.rs
new file mode 100644
index 00000000..1585a25f
--- /dev/null
+++ b/benchmark/src/table.rs
@@ -0,0 +1,174 @@
+use crate::app::Data;
+use tabled::settings::Merge;
+use tabled::{builder::Builder, settings::Style, Table};
+
+#[allow(clippy::too_many_arguments)]
+pub(crate) fn parameters_table(
+ tokenizer_name: String,
+ sequence_length: u32,
+ decode_length: u32,
+ top_n_tokens: Option,
+ n_runs: usize,
+ warmups: usize,
+ temperature: Option,
+ top_k: Option,
+ top_p: Option,
+ typical_p: Option,
+ repetition_penalty: Option,
+ frequency_penalty: Option,
+ watermark: bool,
+ do_sample: bool,
+) -> Table {
+ let mut builder = Builder::default();
+
+ builder.set_header(["Parameter", "Value"]);
+
+ builder.push_record(["Model", &tokenizer_name]);
+ builder.push_record(["Sequence Length", &sequence_length.to_string()]);
+ builder.push_record(["Decode Length", &decode_length.to_string()]);
+ builder.push_record(["Top N Tokens", &format!("{top_n_tokens:?}")]);
+ builder.push_record(["N Runs", &n_runs.to_string()]);
+ builder.push_record(["Warmups", &warmups.to_string()]);
+ builder.push_record(["Temperature", &format!("{temperature:?}")]);
+ builder.push_record(["Top K", &format!("{top_k:?}")]);
+ builder.push_record(["Top P", &format!("{top_p:?}")]);
+ builder.push_record(["Typical P", &format!("{typical_p:?}")]);
+ builder.push_record(["Repetition Penalty", &format!("{repetition_penalty:?}")]);
+ builder.push_record(["Frequency Penalty", &format!("{frequency_penalty:?}")]);
+ builder.push_record(["Watermark", &watermark.to_string()]);
+ builder.push_record(["Do Sample", &do_sample.to_string()]);
+
+ let mut table = builder.build();
+ table.with(Style::markdown());
+ table
+}
+
+pub(crate) fn latency_table(data: &Data) -> Table {
+ let mut builder = Builder::default();
+
+ builder.set_header([
+ "Step",
+ "Batch Size",
+ "Average",
+ "Lowest",
+ "Highest",
+ "p50",
+ "p90",
+ "p99",
+ ]);
+
+ add_latencies(
+ &mut builder,
+ "Prefill",
+ &data.batch_size,
+ &data.prefill_latencies,
+ );
+ add_latencies(
+ &mut builder,
+ "Decode (token)",
+ &data.batch_size,
+ &data.decode_token_latencies,
+ );
+ add_latencies(
+ &mut builder,
+ "Decode (total)",
+ &data.batch_size,
+ &data.decode_latencies,
+ );
+
+ let mut table = builder.build();
+ table.with(Style::markdown()).with(Merge::vertical());
+ table
+}
+
+pub(crate) fn throughput_table(data: &Data) -> Table {
+ let mut builder = Builder::default();
+
+ builder.set_header(["Step", "Batch Size", "Average", "Lowest", "Highest"]);
+
+ add_throuhgputs(
+ &mut builder,
+ "Prefill",
+ &data.batch_size,
+ &data.prefill_throughputs,
+ );
+ add_throuhgputs(
+ &mut builder,
+ "Decode",
+ &data.batch_size,
+ &data.decode_throughputs,
+ );
+
+ let mut table = builder.build();
+ table.with(Style::markdown()).with(Merge::vertical());
+ table
+}
+
+fn add_latencies(
+ builder: &mut Builder,
+ step: &'static str,
+ batch_size: &[u32],
+ batch_latencies: &[Vec],
+) {
+ for (i, b) in batch_size.iter().enumerate() {
+ let latencies = &batch_latencies[i];
+ let (avg, min, max) = avg_min_max(latencies);
+
+ let row = [
+ step,
+ &b.to_string(),
+ &format_value(avg, "ms"),
+ &format_value(min, "ms"),
+ &format_value(max, "ms"),
+ &format_value(px(latencies, 50), "ms"),
+ &format_value(px(latencies, 90), "ms"),
+ &format_value(px(latencies, 99), "ms"),
+ ];
+
+ builder.push_record(row);
+ }
+}
+
+fn add_throuhgputs(
+ builder: &mut Builder,
+ step: &'static str,
+ batch_size: &[u32],
+ batch_throughputs: &[Vec],
+) {
+ for (i, b) in batch_size.iter().enumerate() {
+ let throughputs = &batch_throughputs[i];
+ let (avg, min, max) = avg_min_max(throughputs);
+
+ let row = [
+ step,
+ &b.to_string(),
+ &format_value(avg, "tokens/secs"),
+ &format_value(min, "tokens/secs"),
+ &format_value(max, "tokens/secs"),
+ ];
+
+ builder.push_record(row);
+ }
+}
+
+fn avg_min_max(data: &[f64]) -> (f64, f64, f64) {
+ let average = data.iter().sum::() / data.len() as f64;
+ let min = data
+ .iter()
+ .min_by(|a, b| a.total_cmp(b))
+ .unwrap_or(&f64::NAN);
+ let max = data
+ .iter()
+ .max_by(|a, b| a.total_cmp(b))
+ .unwrap_or(&f64::NAN);
+ (average, *min, *max)
+}
+
+fn px(data: &[f64], p: u32) -> f64 {
+ let i = (f64::from(p) / 100.0 * data.len() as f64) as usize;
+ *data.get(i).unwrap_or(&f64::NAN)
+}
+
+fn format_value(value: f64, unit: &'static str) -> String {
+ format!("{:.2} {unit}", value)
+}
diff --git a/benchmark/src/utils.rs b/benchmark/src/utils.rs
new file mode 100644
index 00000000..20469991
--- /dev/null
+++ b/benchmark/src/utils.rs
@@ -0,0 +1,43 @@
+/// MIT License
+//
+// Copyright (c) 2020 hatoo
+//
+// Permission is hereby granted, free of charge, to any person obtaining a copy
+// of this software and associated documentation files (the "Software"), to deal
+// in the Software without restriction, including without limitation the rights
+// to use, copy, modify, merge, publish, distribute, sublicense, and/or sell
+// copies of the Software, and to permit persons to whom the Software is
+// furnished to do so, subject to the following conditions:
+//
+// The above copyright notice and this permission notice shall be included in all
+// copies or substantial portions of the Software.
+use std::collections::BTreeMap;
+
+pub(crate) fn histogram(values: &[f64], bins: usize) -> Vec<(f64, usize)> {
+ assert!(bins >= 2);
+ let mut bucket: Vec = vec![0; bins];
+ let min = values.iter().collect::().min();
+ let max = values.iter().collect::().max();
+ let step = (max - min) / (bins - 1) as f64;
+
+ for &v in values {
+ let i = std::cmp::min(((v - min) / step).ceil() as usize, bins - 1);
+ bucket[i] += 1;
+ }
+
+ bucket
+ .into_iter()
+ .enumerate()
+ .map(|(i, v)| (min + step * i as f64, v))
+ .collect()
+}
+
+pub(crate) fn percentiles(values: &[f64], pecents: &[i32]) -> BTreeMap {
+ pecents
+ .iter()
+ .map(|&p| {
+ let i = (f64::from(p) / 100.0 * values.len() as f64) as usize;
+ (format!("p{p}"), *values.get(i).unwrap_or(&f64::NAN))
+ })
+ .collect()
+}
diff --git a/clients/python/.gitignore b/clients/python/.gitignore
new file mode 100644
index 00000000..5a8ecaa7
--- /dev/null
+++ b/clients/python/.gitignore
@@ -0,0 +1,158 @@
+# Byte-compiled / optimized / DLL files
+__pycache__/
+text_generation/__pycache__/
+text_generation/pb/__pycache__/
+*.py[cod]
+*$py.class
+
+# C extensions
+*.so
+
+# Distribution / packaging
+.Python
+build/
+develop-eggs/
+dist/
+downloads/
+eggs/
+.eggs/
+lib/
+lib64/
+parts/
+sdist/
+var/
+wheels/
+share/python-wheels/
+*.egg-info/
+.installed.cfg
+*.egg
+MANIFEST
+
+# PyInstaller
+# Usually these files are written by a python script from a template
+# before PyInstaller builds the exe, so as to inject date/other infos into it.
+*.manifest
+*.spec
+
+# Installer logs
+pip-log.txt
+pip-delete-this-directory.txt
+
+# Unit test / coverage reports
+htmlcov/
+.tox/
+.nox/
+.coverage
+.coverage.*
+.cache
+nosetests.xml
+coverage.xml
+*.cover
+*.py,cover
+.hypothesis/
+.pytest_cache/
+cover/
+
+# Translations
+*.mo
+*.pot
+
+# Django stuff:
+*.log
+local_settings.py
+db.sqlite3
+db.sqlite3-journal
+
+# Flask stuff:
+instance/
+.webassets-cache
+
+# Scrapy stuff:
+.scrapy
+
+# Sphinx documentation
+docs/_build/
+
+# PyBuilder
+.pybuilder/
+target/
+
+# Jupyter Notebook
+.ipynb_checkpoints
+
+# IPython
+profile_default/
+ipython_config.py
+
+# pyenv
+# For a library or package, you might want to ignore these files since the code is
+# intended to run in multiple environments; otherwise, check them in:
+# .python-version
+
+# pipenv
+# According to pypa/pipenv#598, it is recommended to include Pipfile.lock in version control.
+# However, in case of collaboration, if having platform-specific dependencies or dependencies
+# having no cross-platform support, pipenv may install dependencies that don't work, or not
+# install all needed dependencies.
+#Pipfile.lock
+
+# poetry
+# Similar to Pipfile.lock, it is generally recommended to include poetry.lock in version control.
+# This is especially recommended for binary packages to ensure reproducibility, and is more
+# commonly ignored for libraries.
+# https://python-poetry.org/docs/basic-usage/#commit-your-poetrylock-file-to-version-control
+#poetry.lock
+
+# pdm
+# Similar to Pipfile.lock, it is generally recommended to include pdm.lock in version control.
+#pdm.lock
+# pdm stores project-wide configurations in .pdm.toml, but it is recommended to not include it
+# in version control.
+# https://pdm.fming.dev/#use-with-ide
+.pdm.toml
+
+# PEP 582; used by e.g. github.com/David-OConnor/pyflow and github.com/pdm-project/pdm
+__pypackages__/
+
+# Celery stuff
+celerybeat-schedule
+celerybeat.pid
+
+# SageMath parsed files
+*.sage.py
+
+# Environments
+.env
+.venv
+env/
+venv/
+ENV/
+env.bak/
+venv.bak/
+
+# Spyder project settings
+.spyderproject
+.spyproject
+
+# Rope project settings
+.ropeproject
+
+# mkdocs documentation
+/site
+
+# mypy
+.mypy_cache/
+.dmypy.json
+dmypy.json
+
+# Pyre type checker
+.pyre/
+
+# pytype static type analyzer
+.pytype/
+
+# Cython debug symbols
+cython_debug/
+
+transformers
+safetensors
diff --git a/clients/python/Makefile b/clients/python/Makefile
new file mode 100644
index 00000000..42720875
--- /dev/null
+++ b/clients/python/Makefile
@@ -0,0 +1,6 @@
+unit-tests:
+ python -m pytest --cov=text_generation tests
+
+install:
+ pip install pip --upgrade
+ pip install -e .
diff --git a/clients/python/README.md b/clients/python/README.md
new file mode 100644
index 00000000..bf37508e
--- /dev/null
+++ b/clients/python/README.md
@@ -0,0 +1,279 @@
+# Text Generation
+
+The Hugging Face Text Generation Python library provides a convenient way of interfacing with a
+`text-generation-inference` instance running on
+[Hugging Face Inference Endpoints](https://huggingface.co/inference-endpoints) or on the Hugging Face Hub.
+
+## Get Started
+
+### Install
+
+```shell
+pip install text-generation
+```
+
+### Inference API Usage
+
+```python
+from text_generation import InferenceAPIClient
+
+client = InferenceAPIClient("bigscience/bloomz")
+text = client.generate("Why is the sky blue?").generated_text
+print(text)
+# ' Rayleigh scattering'
+
+# Token Streaming
+text = ""
+for response in client.generate_stream("Why is the sky blue?"):
+ if not response.token.special:
+ text += response.token.text
+
+print(text)
+# ' Rayleigh scattering'
+```
+
+or with the asynchronous client:
+
+```python
+from text_generation import InferenceAPIAsyncClient
+
+client = InferenceAPIAsyncClient("bigscience/bloomz")
+response = await client.generate("Why is the sky blue?")
+print(response.generated_text)
+# ' Rayleigh scattering'
+
+# Token Streaming
+text = ""
+async for response in client.generate_stream("Why is the sky blue?"):
+ if not response.token.special:
+ text += response.token.text
+
+print(text)
+# ' Rayleigh scattering'
+```
+
+Check all currently deployed models on the Huggingface Inference API with `Text Generation` support:
+
+```python
+from text_generation.inference_api import deployed_models
+
+print(deployed_models())
+```
+
+### Hugging Face Inference Endpoint usage
+
+```python
+from text_generation import Client
+
+endpoint_url = "https://YOUR_ENDPOINT.endpoints.huggingface.cloud"
+
+client = Client(endpoint_url)
+text = client.generate("Why is the sky blue?").generated_text
+print(text)
+# ' Rayleigh scattering'
+
+# Token Streaming
+text = ""
+for response in client.generate_stream("Why is the sky blue?"):
+ if not response.token.special:
+ text += response.token.text
+
+print(text)
+# ' Rayleigh scattering'
+```
+
+or with the asynchronous client:
+
+```python
+from text_generation import AsyncClient
+
+endpoint_url = "https://YOUR_ENDPOINT.endpoints.huggingface.cloud"
+
+client = AsyncClient(endpoint_url)
+response = await client.generate("Why is the sky blue?")
+print(response.generated_text)
+# ' Rayleigh scattering'
+
+# Token Streaming
+text = ""
+async for response in client.generate_stream("Why is the sky blue?"):
+ if not response.token.special:
+ text += response.token.text
+
+print(text)
+# ' Rayleigh scattering'
+```
+
+### Types
+
+```python
+# enum for grammar type
+class GrammarType(Enum):
+ Json = "json"
+ Regex = "regex"
+
+
+# Grammar type and value
+class Grammar:
+ # Grammar type
+ type: GrammarType
+ # Grammar value
+ value: Union[str, dict]
+
+class Parameters:
+ # Activate logits sampling
+ do_sample: bool
+ # Maximum number of generated tokens
+ max_new_tokens: int
+ # The parameter for repetition penalty. 1.0 means no penalty.
+ # See [this paper](https://arxiv.org/pdf/1909.05858.pdf) for more details.
+ repetition_penalty: Optional[float]
+ # The parameter for frequency penalty. 1.0 means no penalty
+ # Penalize new tokens based on their existing frequency in the text so far,
+ # decreasing the model's likelihood to repeat the same line verbatim.
+ frequency_penalty: Optional[float]
+ # Whether to prepend the prompt to the generated text
+ return_full_text: bool
+ # Stop generating tokens if a member of `stop_sequences` is generated
+ stop: List[str]
+ # Random sampling seed
+ seed: Optional[int]
+ # The value used to module the logits distribution.
+ temperature: Optional[float]
+ # The number of highest probability vocabulary tokens to keep for top-k-filtering.
+ top_k: Optional[int]
+ # If set to < 1, only the smallest set of most probable tokens with probabilities that add up to `top_p` or
+ # higher are kept for generation.
+ top_p: Optional[float]
+ # truncate inputs tokens to the given size
+ truncate: Optional[int]
+ # Typical Decoding mass
+ # See [Typical Decoding for Natural Language Generation](https://arxiv.org/abs/2202.00666) for more information
+ typical_p: Optional[float]
+ # Generate best_of sequences and return the one if the highest token logprobs
+ best_of: Optional[int]
+ # Watermarking with [A Watermark for Large Language Models](https://arxiv.org/abs/2301.10226)
+ watermark: bool
+ # Get generation details
+ details: bool
+ # Get decoder input token logprobs and ids
+ decoder_input_details: bool
+ # Return the N most likely tokens at each step
+ top_n_tokens: Optional[int]
+ # grammar to use for generation
+ grammar: Optional[Grammar]
+
+class Request:
+ # Prompt
+ inputs: str
+ # Generation parameters
+ parameters: Optional[Parameters]
+ # Whether to stream output tokens
+ stream: bool
+
+# Decoder input tokens
+class InputToken:
+ # Token ID from the model tokenizer
+ id: int
+ # Token text
+ text: str
+ # Logprob
+ # Optional since the logprob of the first token cannot be computed
+ logprob: Optional[float]
+
+
+# Generated tokens
+class Token:
+ # Token ID from the model tokenizer
+ id: int
+ # Token text
+ text: str
+ # Logprob
+ logprob: Optional[float]
+ # Is the token a special token
+ # Can be used to ignore tokens when concatenating
+ special: bool
+
+
+# Generation finish reason
+class FinishReason(Enum):
+ # number of generated tokens == `max_new_tokens`
+ Length = "length"
+ # the model generated its end of sequence token
+ EndOfSequenceToken = "eos_token"
+ # the model generated a text included in `stop_sequences`
+ StopSequence = "stop_sequence"
+
+
+# Additional sequences when using the `best_of` parameter
+class BestOfSequence:
+ # Generated text
+ generated_text: str
+ # Generation finish reason
+ finish_reason: FinishReason
+ # Number of generated tokens
+ generated_tokens: int
+ # Sampling seed if sampling was activated
+ seed: Optional[int]
+ # Decoder input tokens, empty if decoder_input_details is False
+ prefill: List[InputToken]
+ # Generated tokens
+ tokens: List[Token]
+ # Most likely tokens
+ top_tokens: Optional[List[List[Token]]]
+
+
+# `generate` details
+class Details:
+ # Generation finish reason
+ finish_reason: FinishReason
+ # Number of generated tokens
+ generated_tokens: int
+ # Sampling seed if sampling was activated
+ seed: Optional[int]
+ # Decoder input tokens, empty if decoder_input_details is False
+ prefill: List[InputToken]
+ # Generated tokens
+ tokens: List[Token]
+ # Most likely tokens
+ top_tokens: Optional[List[List[Token]]]
+ # Additional sequences when using the `best_of` parameter
+ best_of_sequences: Optional[List[BestOfSequence]]
+
+
+# `generate` return value
+class Response:
+ # Generated text
+ generated_text: str
+ # Generation details
+ details: Details
+
+
+# `generate_stream` details
+class StreamDetails:
+ # Generation finish reason
+ finish_reason: FinishReason
+ # Number of generated tokens
+ generated_tokens: int
+ # Sampling seed if sampling was activated
+ seed: Optional[int]
+
+
+# `generate_stream` return value
+class StreamResponse:
+ # Generated token
+ token: Token
+ # Most likely tokens
+ top_tokens: Optional[List[Token]]
+ # Complete generated text
+ # Only available when the generation is finished
+ generated_text: Optional[str]
+ # Generation details
+ # Only available when the generation is finished
+ details: Optional[StreamDetails]
+
+# Inference API currently deployed model
+class DeployedModel:
+ model_id: str
+ sha: str
+```
diff --git a/clients/python/poetry.lock b/clients/python/poetry.lock
new file mode 100644
index 00000000..148d9906
--- /dev/null
+++ b/clients/python/poetry.lock
@@ -0,0 +1,1163 @@
+# This file is automatically @generated by Poetry 1.7.1 and should not be changed by hand.
+
+[[package]]
+name = "aiohttp"
+version = "3.8.5"
+description = "Async http client/server framework (asyncio)"
+optional = false
+python-versions = ">=3.6"
+files = [
+ {file = "aiohttp-3.8.5-cp310-cp310-macosx_10_9_universal2.whl", hash = "sha256:a94159871304770da4dd371f4291b20cac04e8c94f11bdea1c3478e557fbe0d8"},
+ {file = "aiohttp-3.8.5-cp310-cp310-macosx_10_9_x86_64.whl", hash = "sha256:13bf85afc99ce6f9ee3567b04501f18f9f8dbbb2ea11ed1a2e079670403a7c84"},
+ {file = "aiohttp-3.8.5-cp310-cp310-macosx_11_0_arm64.whl", hash = "sha256:2ce2ac5708501afc4847221a521f7e4b245abf5178cf5ddae9d5b3856ddb2f3a"},
+ {file = "aiohttp-3.8.5-cp310-cp310-manylinux_2_17_aarch64.manylinux2014_aarch64.whl", hash = "sha256:96943e5dcc37a6529d18766597c491798b7eb7a61d48878611298afc1fca946c"},
+ {file = "aiohttp-3.8.5-cp310-cp310-manylinux_2_17_ppc64le.manylinux2014_ppc64le.whl", hash = "sha256:2ad5c3c4590bb3cc28b4382f031f3783f25ec223557124c68754a2231d989e2b"},
+ {file = "aiohttp-3.8.5-cp310-cp310-manylinux_2_17_s390x.manylinux2014_s390x.whl", hash = "sha256:0c413c633d0512df4dc7fd2373ec06cc6a815b7b6d6c2f208ada7e9e93a5061d"},
+ {file = "aiohttp-3.8.5-cp310-cp310-manylinux_2_17_x86_64.manylinux2014_x86_64.whl", hash = "sha256:df72ac063b97837a80d80dec8d54c241af059cc9bb42c4de68bd5b61ceb37caa"},
+ {file = "aiohttp-3.8.5-cp310-cp310-manylinux_2_5_i686.manylinux1_i686.manylinux_2_17_i686.manylinux2014_i686.whl", hash = "sha256:c48c5c0271149cfe467c0ff8eb941279fd6e3f65c9a388c984e0e6cf57538e14"},
+ {file = "aiohttp-3.8.5-cp310-cp310-musllinux_1_1_aarch64.whl", hash = "sha256:368a42363c4d70ab52c2c6420a57f190ed3dfaca6a1b19afda8165ee16416a82"},
+ {file = "aiohttp-3.8.5-cp310-cp310-musllinux_1_1_i686.whl", hash = "sha256:7607ec3ce4993464368505888af5beb446845a014bc676d349efec0e05085905"},
+ {file = "aiohttp-3.8.5-cp310-cp310-musllinux_1_1_ppc64le.whl", hash = "sha256:0d21c684808288a98914e5aaf2a7c6a3179d4df11d249799c32d1808e79503b5"},
+ {file = "aiohttp-3.8.5-cp310-cp310-musllinux_1_1_s390x.whl", hash = "sha256:312fcfbacc7880a8da0ae8b6abc6cc7d752e9caa0051a53d217a650b25e9a691"},
+ {file = "aiohttp-3.8.5-cp310-cp310-musllinux_1_1_x86_64.whl", hash = "sha256:ad093e823df03bb3fd37e7dec9d4670c34f9e24aeace76808fc20a507cace825"},
+ {file = "aiohttp-3.8.5-cp310-cp310-win32.whl", hash = "sha256:33279701c04351a2914e1100b62b2a7fdb9a25995c4a104259f9a5ead7ed4802"},
+ {file = "aiohttp-3.8.5-cp310-cp310-win_amd64.whl", hash = "sha256:6e4a280e4b975a2e7745573e3fc9c9ba0d1194a3738ce1cbaa80626cc9b4f4df"},
+ {file = "aiohttp-3.8.5-cp311-cp311-macosx_10_9_universal2.whl", hash = "sha256:ae871a964e1987a943d83d6709d20ec6103ca1eaf52f7e0d36ee1b5bebb8b9b9"},
+ {file = "aiohttp-3.8.5-cp311-cp311-macosx_10_9_x86_64.whl", hash = "sha256:461908b2578955045efde733719d62f2b649c404189a09a632d245b445c9c975"},
+ {file = "aiohttp-3.8.5-cp311-cp311-macosx_11_0_arm64.whl", hash = "sha256:72a860c215e26192379f57cae5ab12b168b75db8271f111019509a1196dfc780"},
+ {file = "aiohttp-3.8.5-cp311-cp311-manylinux_2_17_aarch64.manylinux2014_aarch64.whl", hash = "sha256:cc14be025665dba6202b6a71cfcdb53210cc498e50068bc088076624471f8bb9"},
+ {file = "aiohttp-3.8.5-cp311-cp311-manylinux_2_17_ppc64le.manylinux2014_ppc64le.whl", hash = "sha256:8af740fc2711ad85f1a5c034a435782fbd5b5f8314c9a3ef071424a8158d7f6b"},
+ {file = "aiohttp-3.8.5-cp311-cp311-manylinux_2_17_s390x.manylinux2014_s390x.whl", hash = "sha256:841cd8233cbd2111a0ef0a522ce016357c5e3aff8a8ce92bcfa14cef890d698f"},
+ {file = "aiohttp-3.8.5-cp311-cp311-manylinux_2_17_x86_64.manylinux2014_x86_64.whl", hash = "sha256:5ed1c46fb119f1b59304b5ec89f834f07124cd23ae5b74288e364477641060ff"},
+ {file = "aiohttp-3.8.5-cp311-cp311-manylinux_2_5_i686.manylinux1_i686.manylinux_2_17_i686.manylinux2014_i686.whl", hash = "sha256:84f8ae3e09a34f35c18fa57f015cc394bd1389bce02503fb30c394d04ee6b938"},
+ {file = "aiohttp-3.8.5-cp311-cp311-musllinux_1_1_aarch64.whl", hash = "sha256:62360cb771707cb70a6fd114b9871d20d7dd2163a0feafe43fd115cfe4fe845e"},
+ {file = "aiohttp-3.8.5-cp311-cp311-musllinux_1_1_i686.whl", hash = "sha256:23fb25a9f0a1ca1f24c0a371523546366bb642397c94ab45ad3aedf2941cec6a"},
+ {file = "aiohttp-3.8.5-cp311-cp311-musllinux_1_1_ppc64le.whl", hash = "sha256:b0ba0d15164eae3d878260d4c4df859bbdc6466e9e6689c344a13334f988bb53"},
+ {file = "aiohttp-3.8.5-cp311-cp311-musllinux_1_1_s390x.whl", hash = "sha256:5d20003b635fc6ae3f96d7260281dfaf1894fc3aa24d1888a9b2628e97c241e5"},
+ {file = "aiohttp-3.8.5-cp311-cp311-musllinux_1_1_x86_64.whl", hash = "sha256:0175d745d9e85c40dcc51c8f88c74bfbaef9e7afeeeb9d03c37977270303064c"},
+ {file = "aiohttp-3.8.5-cp311-cp311-win32.whl", hash = "sha256:2e1b1e51b0774408f091d268648e3d57f7260c1682e7d3a63cb00d22d71bb945"},
+ {file = "aiohttp-3.8.5-cp311-cp311-win_amd64.whl", hash = "sha256:043d2299f6dfdc92f0ac5e995dfc56668e1587cea7f9aa9d8a78a1b6554e5755"},
+ {file = "aiohttp-3.8.5-cp36-cp36m-macosx_10_9_x86_64.whl", hash = "sha256:cae533195e8122584ec87531d6df000ad07737eaa3c81209e85c928854d2195c"},
+ {file = "aiohttp-3.8.5-cp36-cp36m-manylinux_2_17_aarch64.manylinux2014_aarch64.whl", hash = "sha256:4f21e83f355643c345177a5d1d8079f9f28b5133bcd154193b799d380331d5d3"},
+ {file = "aiohttp-3.8.5-cp36-cp36m-manylinux_2_17_ppc64le.manylinux2014_ppc64le.whl", hash = "sha256:a7a75ef35f2df54ad55dbf4b73fe1da96f370e51b10c91f08b19603c64004acc"},
+ {file = "aiohttp-3.8.5-cp36-cp36m-manylinux_2_17_s390x.manylinux2014_s390x.whl", hash = "sha256:2e2e9839e14dd5308ee773c97115f1e0a1cb1d75cbeeee9f33824fa5144c7634"},
+ {file = "aiohttp-3.8.5-cp36-cp36m-manylinux_2_17_x86_64.manylinux2014_x86_64.whl", hash = "sha256:c44e65da1de4403d0576473e2344828ef9c4c6244d65cf4b75549bb46d40b8dd"},
+ {file = "aiohttp-3.8.5-cp36-cp36m-manylinux_2_5_i686.manylinux1_i686.manylinux_2_17_i686.manylinux2014_i686.whl", hash = "sha256:78d847e4cde6ecc19125ccbc9bfac4a7ab37c234dd88fbb3c5c524e8e14da543"},
+ {file = "aiohttp-3.8.5-cp36-cp36m-musllinux_1_1_aarch64.whl", hash = "sha256:c7a815258e5895d8900aec4454f38dca9aed71085f227537208057853f9d13f2"},
+ {file = "aiohttp-3.8.5-cp36-cp36m-musllinux_1_1_i686.whl", hash = "sha256:8b929b9bd7cd7c3939f8bcfffa92fae7480bd1aa425279d51a89327d600c704d"},
+ {file = "aiohttp-3.8.5-cp36-cp36m-musllinux_1_1_ppc64le.whl", hash = "sha256:5db3a5b833764280ed7618393832e0853e40f3d3e9aa128ac0ba0f8278d08649"},
+ {file = "aiohttp-3.8.5-cp36-cp36m-musllinux_1_1_s390x.whl", hash = "sha256:a0215ce6041d501f3155dc219712bc41252d0ab76474615b9700d63d4d9292af"},
+ {file = "aiohttp-3.8.5-cp36-cp36m-musllinux_1_1_x86_64.whl", hash = "sha256:fd1ed388ea7fbed22c4968dd64bab0198de60750a25fe8c0c9d4bef5abe13824"},
+ {file = "aiohttp-3.8.5-cp36-cp36m-win32.whl", hash = "sha256:6e6783bcc45f397fdebc118d772103d751b54cddf5b60fbcc958382d7dd64f3e"},
+ {file = "aiohttp-3.8.5-cp36-cp36m-win_amd64.whl", hash = "sha256:b5411d82cddd212644cf9360879eb5080f0d5f7d809d03262c50dad02f01421a"},
+ {file = "aiohttp-3.8.5-cp37-cp37m-macosx_10_9_x86_64.whl", hash = "sha256:01d4c0c874aa4ddfb8098e85d10b5e875a70adc63db91f1ae65a4b04d3344cda"},
+ {file = "aiohttp-3.8.5-cp37-cp37m-manylinux_2_17_aarch64.manylinux2014_aarch64.whl", hash = "sha256:e5980a746d547a6ba173fd5ee85ce9077e72d118758db05d229044b469d9029a"},
+ {file = "aiohttp-3.8.5-cp37-cp37m-manylinux_2_17_ppc64le.manylinux2014_ppc64le.whl", hash = "sha256:2a482e6da906d5e6e653be079b29bc173a48e381600161c9932d89dfae5942ef"},
+ {file = "aiohttp-3.8.5-cp37-cp37m-manylinux_2_17_s390x.manylinux2014_s390x.whl", hash = "sha256:80bd372b8d0715c66c974cf57fe363621a02f359f1ec81cba97366948c7fc873"},
+ {file = "aiohttp-3.8.5-cp37-cp37m-manylinux_2_17_x86_64.manylinux2014_x86_64.whl", hash = "sha256:c1161b345c0a444ebcf46bf0a740ba5dcf50612fd3d0528883fdc0eff578006a"},
+ {file = "aiohttp-3.8.5-cp37-cp37m-manylinux_2_5_i686.manylinux1_i686.manylinux_2_17_i686.manylinux2014_i686.whl", hash = "sha256:cd56db019015b6acfaaf92e1ac40eb8434847d9bf88b4be4efe5bfd260aee692"},
+ {file = "aiohttp-3.8.5-cp37-cp37m-musllinux_1_1_aarch64.whl", hash = "sha256:153c2549f6c004d2754cc60603d4668899c9895b8a89397444a9c4efa282aaf4"},
+ {file = "aiohttp-3.8.5-cp37-cp37m-musllinux_1_1_i686.whl", hash = "sha256:4a01951fabc4ce26ab791da5f3f24dca6d9a6f24121746eb19756416ff2d881b"},
+ {file = "aiohttp-3.8.5-cp37-cp37m-musllinux_1_1_ppc64le.whl", hash = "sha256:bfb9162dcf01f615462b995a516ba03e769de0789de1cadc0f916265c257e5d8"},
+ {file = "aiohttp-3.8.5-cp37-cp37m-musllinux_1_1_s390x.whl", hash = "sha256:7dde0009408969a43b04c16cbbe252c4f5ef4574ac226bc8815cd7342d2028b6"},
+ {file = "aiohttp-3.8.5-cp37-cp37m-musllinux_1_1_x86_64.whl", hash = "sha256:4149d34c32f9638f38f544b3977a4c24052042affa895352d3636fa8bffd030a"},
+ {file = "aiohttp-3.8.5-cp37-cp37m-win32.whl", hash = "sha256:68c5a82c8779bdfc6367c967a4a1b2aa52cd3595388bf5961a62158ee8a59e22"},
+ {file = "aiohttp-3.8.5-cp37-cp37m-win_amd64.whl", hash = "sha256:2cf57fb50be5f52bda004b8893e63b48530ed9f0d6c96c84620dc92fe3cd9b9d"},
+ {file = "aiohttp-3.8.5-cp38-cp38-macosx_10_9_universal2.whl", hash = "sha256:eca4bf3734c541dc4f374ad6010a68ff6c6748f00451707f39857f429ca36ced"},
+ {file = "aiohttp-3.8.5-cp38-cp38-macosx_10_9_x86_64.whl", hash = "sha256:1274477e4c71ce8cfe6c1ec2f806d57c015ebf84d83373676036e256bc55d690"},
+ {file = "aiohttp-3.8.5-cp38-cp38-macosx_11_0_arm64.whl", hash = "sha256:28c543e54710d6158fc6f439296c7865b29e0b616629767e685a7185fab4a6b9"},
+ {file = "aiohttp-3.8.5-cp38-cp38-manylinux_2_17_aarch64.manylinux2014_aarch64.whl", hash = "sha256:910bec0c49637d213f5d9877105d26e0c4a4de2f8b1b29405ff37e9fc0ad52b8"},
+ {file = "aiohttp-3.8.5-cp38-cp38-manylinux_2_17_ppc64le.manylinux2014_ppc64le.whl", hash = "sha256:5443910d662db951b2e58eb70b0fbe6b6e2ae613477129a5805d0b66c54b6cb7"},
+ {file = "aiohttp-3.8.5-cp38-cp38-manylinux_2_17_s390x.manylinux2014_s390x.whl", hash = "sha256:2e460be6978fc24e3df83193dc0cc4de46c9909ed92dd47d349a452ef49325b7"},
+ {file = "aiohttp-3.8.5-cp38-cp38-manylinux_2_17_x86_64.manylinux2014_x86_64.whl", hash = "sha256:fb1558def481d84f03b45888473fc5a1f35747b5f334ef4e7a571bc0dfcb11f8"},
+ {file = "aiohttp-3.8.5-cp38-cp38-manylinux_2_5_i686.manylinux1_i686.manylinux_2_17_i686.manylinux2014_i686.whl", hash = "sha256:34dd0c107799dcbbf7d48b53be761a013c0adf5571bf50c4ecad5643fe9cfcd0"},
+ {file = "aiohttp-3.8.5-cp38-cp38-musllinux_1_1_aarch64.whl", hash = "sha256:aa1990247f02a54185dc0dff92a6904521172a22664c863a03ff64c42f9b5410"},
+ {file = "aiohttp-3.8.5-cp38-cp38-musllinux_1_1_i686.whl", hash = "sha256:0e584a10f204a617d71d359fe383406305a4b595b333721fa50b867b4a0a1548"},
+ {file = "aiohttp-3.8.5-cp38-cp38-musllinux_1_1_ppc64le.whl", hash = "sha256:a3cf433f127efa43fee6b90ea4c6edf6c4a17109d1d037d1a52abec84d8f2e42"},
+ {file = "aiohttp-3.8.5-cp38-cp38-musllinux_1_1_s390x.whl", hash = "sha256:c11f5b099adafb18e65c2c997d57108b5bbeaa9eeee64a84302c0978b1ec948b"},
+ {file = "aiohttp-3.8.5-cp38-cp38-musllinux_1_1_x86_64.whl", hash = "sha256:84de26ddf621d7ac4c975dbea4c945860e08cccde492269db4e1538a6a6f3c35"},
+ {file = "aiohttp-3.8.5-cp38-cp38-win32.whl", hash = "sha256:ab88bafedc57dd0aab55fa728ea10c1911f7e4d8b43e1d838a1739f33712921c"},
+ {file = "aiohttp-3.8.5-cp38-cp38-win_amd64.whl", hash = "sha256:5798a9aad1879f626589f3df0f8b79b3608a92e9beab10e5fda02c8a2c60db2e"},
+ {file = "aiohttp-3.8.5-cp39-cp39-macosx_10_9_universal2.whl", hash = "sha256:a6ce61195c6a19c785df04e71a4537e29eaa2c50fe745b732aa937c0c77169f3"},
+ {file = "aiohttp-3.8.5-cp39-cp39-macosx_10_9_x86_64.whl", hash = "sha256:773dd01706d4db536335fcfae6ea2440a70ceb03dd3e7378f3e815b03c97ab51"},
+ {file = "aiohttp-3.8.5-cp39-cp39-macosx_11_0_arm64.whl", hash = "sha256:f83a552443a526ea38d064588613aca983d0ee0038801bc93c0c916428310c28"},
+ {file = "aiohttp-3.8.5-cp39-cp39-manylinux_2_17_aarch64.manylinux2014_aarch64.whl", hash = "sha256:1f7372f7341fcc16f57b2caded43e81ddd18df53320b6f9f042acad41f8e049a"},
+ {file = "aiohttp-3.8.5-cp39-cp39-manylinux_2_17_ppc64le.manylinux2014_ppc64le.whl", hash = "sha256:ea353162f249c8097ea63c2169dd1aa55de1e8fecbe63412a9bc50816e87b761"},
+ {file = "aiohttp-3.8.5-cp39-cp39-manylinux_2_17_s390x.manylinux2014_s390x.whl", hash = "sha256:e5d47ae48db0b2dcf70bc8a3bc72b3de86e2a590fc299fdbbb15af320d2659de"},
+ {file = "aiohttp-3.8.5-cp39-cp39-manylinux_2_17_x86_64.manylinux2014_x86_64.whl", hash = "sha256:d827176898a2b0b09694fbd1088c7a31836d1a505c243811c87ae53a3f6273c1"},
+ {file = "aiohttp-3.8.5-cp39-cp39-manylinux_2_5_i686.manylinux1_i686.manylinux_2_17_i686.manylinux2014_i686.whl", hash = "sha256:3562b06567c06439d8b447037bb655ef69786c590b1de86c7ab81efe1c9c15d8"},
+ {file = "aiohttp-3.8.5-cp39-cp39-musllinux_1_1_aarch64.whl", hash = "sha256:4e874cbf8caf8959d2adf572a78bba17cb0e9d7e51bb83d86a3697b686a0ab4d"},
+ {file = "aiohttp-3.8.5-cp39-cp39-musllinux_1_1_i686.whl", hash = "sha256:6809a00deaf3810e38c628e9a33271892f815b853605a936e2e9e5129762356c"},
+ {file = "aiohttp-3.8.5-cp39-cp39-musllinux_1_1_ppc64le.whl", hash = "sha256:33776e945d89b29251b33a7e7d006ce86447b2cfd66db5e5ded4e5cd0340585c"},
+ {file = "aiohttp-3.8.5-cp39-cp39-musllinux_1_1_s390x.whl", hash = "sha256:eaeed7abfb5d64c539e2db173f63631455f1196c37d9d8d873fc316470dfbacd"},
+ {file = "aiohttp-3.8.5-cp39-cp39-musllinux_1_1_x86_64.whl", hash = "sha256:e91d635961bec2d8f19dfeb41a539eb94bd073f075ca6dae6c8dc0ee89ad6f91"},
+ {file = "aiohttp-3.8.5-cp39-cp39-win32.whl", hash = "sha256:00ad4b6f185ec67f3e6562e8a1d2b69660be43070bd0ef6fcec5211154c7df67"},
+ {file = "aiohttp-3.8.5-cp39-cp39-win_amd64.whl", hash = "sha256:c0a9034379a37ae42dea7ac1e048352d96286626251862e448933c0f59cbd79c"},
+ {file = "aiohttp-3.8.5.tar.gz", hash = "sha256:b9552ec52cc147dbf1944ac7ac98af7602e51ea2dcd076ed194ca3c0d1c7d0bc"},
+]
+
+[package.dependencies]
+aiosignal = ">=1.1.2"
+async-timeout = ">=4.0.0a3,<5.0"
+asynctest = {version = "0.13.0", markers = "python_version < \"3.8\""}
+attrs = ">=17.3.0"
+charset-normalizer = ">=2.0,<4.0"
+frozenlist = ">=1.1.1"
+multidict = ">=4.5,<7.0"
+typing-extensions = {version = ">=3.7.4", markers = "python_version < \"3.8\""}
+yarl = ">=1.0,<2.0"
+
+[package.extras]
+speedups = ["Brotli", "aiodns", "cchardet"]
+
+[[package]]
+name = "aiosignal"
+version = "1.3.1"
+description = "aiosignal: a list of registered asynchronous callbacks"
+optional = false
+python-versions = ">=3.7"
+files = [
+ {file = "aiosignal-1.3.1-py3-none-any.whl", hash = "sha256:f8376fb07dd1e86a584e4fcdec80b36b7f81aac666ebc724e2c090300dd83b17"},
+ {file = "aiosignal-1.3.1.tar.gz", hash = "sha256:54cd96e15e1649b75d6c87526a6ff0b6c1b0dd3459f43d9ca11d48c339b68cfc"},
+]
+
+[package.dependencies]
+frozenlist = ">=1.1.0"
+
+[[package]]
+name = "annotated-types"
+version = "0.5.0"
+description = "Reusable constraint types to use with typing.Annotated"
+optional = false
+python-versions = ">=3.7"
+files = [
+ {file = "annotated_types-0.5.0-py3-none-any.whl", hash = "sha256:58da39888f92c276ad970249761ebea80ba544b77acddaa1a4d6cf78287d45fd"},
+ {file = "annotated_types-0.5.0.tar.gz", hash = "sha256:47cdc3490d9ac1506ce92c7aaa76c579dc3509ff11e098fc867e5130ab7be802"},
+]
+
+[package.dependencies]
+typing-extensions = {version = ">=4.0.0", markers = "python_version < \"3.9\""}
+
+[[package]]
+name = "async-timeout"
+version = "4.0.3"
+description = "Timeout context manager for asyncio programs"
+optional = false
+python-versions = ">=3.7"
+files = [
+ {file = "async-timeout-4.0.3.tar.gz", hash = "sha256:4640d96be84d82d02ed59ea2b7105a0f7b33abe8703703cd0ab0bf87c427522f"},
+ {file = "async_timeout-4.0.3-py3-none-any.whl", hash = "sha256:7405140ff1230c310e51dc27b3145b9092d659ce68ff733fb0cefe3ee42be028"},
+]
+
+[package.dependencies]
+typing-extensions = {version = ">=3.6.5", markers = "python_version < \"3.8\""}
+
+[[package]]
+name = "asynctest"
+version = "0.13.0"
+description = "Enhance the standard unittest package with features for testing asyncio libraries"
+optional = false
+python-versions = ">=3.5"
+files = [
+ {file = "asynctest-0.13.0-py3-none-any.whl", hash = "sha256:5da6118a7e6d6b54d83a8f7197769d046922a44d2a99c21382f0a6e4fadae676"},
+ {file = "asynctest-0.13.0.tar.gz", hash = "sha256:c27862842d15d83e6a34eb0b2866c323880eb3a75e4485b079ea11748fd77fac"},
+]
+
+[[package]]
+name = "atomicwrites"
+version = "1.4.1"
+description = "Atomic file writes."
+optional = false
+python-versions = ">=2.7, !=3.0.*, !=3.1.*, !=3.2.*, !=3.3.*"
+files = [
+ {file = "atomicwrites-1.4.1.tar.gz", hash = "sha256:81b2c9071a49367a7f770170e5eec8cb66567cfbbc8c73d20ce5ca4a8d71cf11"},
+]
+
+[[package]]
+name = "attrs"
+version = "23.1.0"
+description = "Classes Without Boilerplate"
+optional = false
+python-versions = ">=3.7"
+files = [
+ {file = "attrs-23.1.0-py3-none-any.whl", hash = "sha256:1f28b4522cdc2fb4256ac1a020c78acf9cba2c6b461ccd2c126f3aa8e8335d04"},
+ {file = "attrs-23.1.0.tar.gz", hash = "sha256:6279836d581513a26f1bf235f9acd333bc9115683f14f7e8fae46c98fc50e015"},
+]
+
+[package.dependencies]
+importlib-metadata = {version = "*", markers = "python_version < \"3.8\""}
+
+[package.extras]
+cov = ["attrs[tests]", "coverage[toml] (>=5.3)"]
+dev = ["attrs[docs,tests]", "pre-commit"]
+docs = ["furo", "myst-parser", "sphinx", "sphinx-notfound-page", "sphinxcontrib-towncrier", "towncrier", "zope-interface"]
+tests = ["attrs[tests-no-zope]", "zope-interface"]
+tests-no-zope = ["cloudpickle", "hypothesis", "mypy (>=1.1.1)", "pympler", "pytest (>=4.3.0)", "pytest-mypy-plugins", "pytest-xdist[psutil]"]
+
+[[package]]
+name = "certifi"
+version = "2023.7.22"
+description = "Python package for providing Mozilla's CA Bundle."
+optional = false
+python-versions = ">=3.6"
+files = [
+ {file = "certifi-2023.7.22-py3-none-any.whl", hash = "sha256:92d6037539857d8206b8f6ae472e8b77db8058fec5937a1ef3f54304089edbb9"},
+ {file = "certifi-2023.7.22.tar.gz", hash = "sha256:539cc1d13202e33ca466e88b2807e29f4c13049d6d87031a3c110744495cb082"},
+]
+
+[[package]]
+name = "charset-normalizer"
+version = "3.2.0"
+description = "The Real First Universal Charset Detector. Open, modern and actively maintained alternative to Chardet."
+optional = false
+python-versions = ">=3.7.0"
+files = [
+ {file = "charset-normalizer-3.2.0.tar.gz", hash = "sha256:3bb3d25a8e6c0aedd251753a79ae98a093c7e7b471faa3aa9a93a81431987ace"},
+ {file = "charset_normalizer-3.2.0-cp310-cp310-macosx_10_9_universal2.whl", hash = "sha256:0b87549028f680ca955556e3bd57013ab47474c3124dc069faa0b6545b6c9710"},
+ {file = "charset_normalizer-3.2.0-cp310-cp310-macosx_10_9_x86_64.whl", hash = "sha256:7c70087bfee18a42b4040bb9ec1ca15a08242cf5867c58726530bdf3945672ed"},
+ {file = "charset_normalizer-3.2.0-cp310-cp310-macosx_11_0_arm64.whl", hash = "sha256:a103b3a7069b62f5d4890ae1b8f0597618f628b286b03d4bc9195230b154bfa9"},
+ {file = "charset_normalizer-3.2.0-cp310-cp310-manylinux_2_17_aarch64.manylinux2014_aarch64.whl", hash = "sha256:94aea8eff76ee6d1cdacb07dd2123a68283cb5569e0250feab1240058f53b623"},
+ {file = "charset_normalizer-3.2.0-cp310-cp310-manylinux_2_17_ppc64le.manylinux2014_ppc64le.whl", hash = "sha256:db901e2ac34c931d73054d9797383d0f8009991e723dab15109740a63e7f902a"},
+ {file = "charset_normalizer-3.2.0-cp310-cp310-manylinux_2_17_s390x.manylinux2014_s390x.whl", hash = "sha256:b0dac0ff919ba34d4df1b6131f59ce95b08b9065233446be7e459f95554c0dc8"},
+ {file = "charset_normalizer-3.2.0-cp310-cp310-manylinux_2_17_x86_64.manylinux2014_x86_64.whl", hash = "sha256:193cbc708ea3aca45e7221ae58f0fd63f933753a9bfb498a3b474878f12caaad"},
+ {file = "charset_normalizer-3.2.0-cp310-cp310-manylinux_2_5_i686.manylinux1_i686.manylinux_2_17_i686.manylinux2014_i686.whl", hash = "sha256:09393e1b2a9461950b1c9a45d5fd251dc7c6f228acab64da1c9c0165d9c7765c"},
+ {file = "charset_normalizer-3.2.0-cp310-cp310-musllinux_1_1_aarch64.whl", hash = "sha256:baacc6aee0b2ef6f3d308e197b5d7a81c0e70b06beae1f1fcacffdbd124fe0e3"},
+ {file = "charset_normalizer-3.2.0-cp310-cp310-musllinux_1_1_i686.whl", hash = "sha256:bf420121d4c8dce6b889f0e8e4ec0ca34b7f40186203f06a946fa0276ba54029"},
+ {file = "charset_normalizer-3.2.0-cp310-cp310-musllinux_1_1_ppc64le.whl", hash = "sha256:c04a46716adde8d927adb9457bbe39cf473e1e2c2f5d0a16ceb837e5d841ad4f"},
+ {file = "charset_normalizer-3.2.0-cp310-cp310-musllinux_1_1_s390x.whl", hash = "sha256:aaf63899c94de41fe3cf934601b0f7ccb6b428c6e4eeb80da72c58eab077b19a"},
+ {file = "charset_normalizer-3.2.0-cp310-cp310-musllinux_1_1_x86_64.whl", hash = "sha256:d62e51710986674142526ab9f78663ca2b0726066ae26b78b22e0f5e571238dd"},
+ {file = "charset_normalizer-3.2.0-cp310-cp310-win32.whl", hash = "sha256:04e57ab9fbf9607b77f7d057974694b4f6b142da9ed4a199859d9d4d5c63fe96"},
+ {file = "charset_normalizer-3.2.0-cp310-cp310-win_amd64.whl", hash = "sha256:48021783bdf96e3d6de03a6e39a1171ed5bd7e8bb93fc84cc649d11490f87cea"},
+ {file = "charset_normalizer-3.2.0-cp311-cp311-macosx_10_9_universal2.whl", hash = "sha256:4957669ef390f0e6719db3613ab3a7631e68424604a7b448f079bee145da6e09"},
+ {file = "charset_normalizer-3.2.0-cp311-cp311-macosx_10_9_x86_64.whl", hash = "sha256:46fb8c61d794b78ec7134a715a3e564aafc8f6b5e338417cb19fe9f57a5a9bf2"},
+ {file = "charset_normalizer-3.2.0-cp311-cp311-macosx_11_0_arm64.whl", hash = "sha256:f779d3ad205f108d14e99bb3859aa7dd8e9c68874617c72354d7ecaec2a054ac"},
+ {file = "charset_normalizer-3.2.0-cp311-cp311-manylinux_2_17_aarch64.manylinux2014_aarch64.whl", hash = "sha256:f25c229a6ba38a35ae6e25ca1264621cc25d4d38dca2942a7fce0b67a4efe918"},
+ {file = "charset_normalizer-3.2.0-cp311-cp311-manylinux_2_17_ppc64le.manylinux2014_ppc64le.whl", hash = "sha256:2efb1bd13885392adfda4614c33d3b68dee4921fd0ac1d3988f8cbb7d589e72a"},
+ {file = "charset_normalizer-3.2.0-cp311-cp311-manylinux_2_17_s390x.manylinux2014_s390x.whl", hash = "sha256:1f30b48dd7fa1474554b0b0f3fdfdd4c13b5c737a3c6284d3cdc424ec0ffff3a"},
+ {file = "charset_normalizer-3.2.0-cp311-cp311-manylinux_2_17_x86_64.manylinux2014_x86_64.whl", hash = "sha256:246de67b99b6851627d945db38147d1b209a899311b1305dd84916f2b88526c6"},
+ {file = "charset_normalizer-3.2.0-cp311-cp311-manylinux_2_5_i686.manylinux1_i686.manylinux_2_17_i686.manylinux2014_i686.whl", hash = "sha256:9bd9b3b31adcb054116447ea22caa61a285d92e94d710aa5ec97992ff5eb7cf3"},
+ {file = "charset_normalizer-3.2.0-cp311-cp311-musllinux_1_1_aarch64.whl", hash = "sha256:8c2f5e83493748286002f9369f3e6607c565a6a90425a3a1fef5ae32a36d749d"},
+ {file = "charset_normalizer-3.2.0-cp311-cp311-musllinux_1_1_i686.whl", hash = "sha256:3170c9399da12c9dc66366e9d14da8bf7147e1e9d9ea566067bbce7bb74bd9c2"},
+ {file = "charset_normalizer-3.2.0-cp311-cp311-musllinux_1_1_ppc64le.whl", hash = "sha256:7a4826ad2bd6b07ca615c74ab91f32f6c96d08f6fcc3902ceeedaec8cdc3bcd6"},
+ {file = "charset_normalizer-3.2.0-cp311-cp311-musllinux_1_1_s390x.whl", hash = "sha256:3b1613dd5aee995ec6d4c69f00378bbd07614702a315a2cf6c1d21461fe17c23"},
+ {file = "charset_normalizer-3.2.0-cp311-cp311-musllinux_1_1_x86_64.whl", hash = "sha256:9e608aafdb55eb9f255034709e20d5a83b6d60c054df0802fa9c9883d0a937aa"},
+ {file = "charset_normalizer-3.2.0-cp311-cp311-win32.whl", hash = "sha256:f2a1d0fd4242bd8643ce6f98927cf9c04540af6efa92323e9d3124f57727bfc1"},
+ {file = "charset_normalizer-3.2.0-cp311-cp311-win_amd64.whl", hash = "sha256:681eb3d7e02e3c3655d1b16059fbfb605ac464c834a0c629048a30fad2b27489"},
+ {file = "charset_normalizer-3.2.0-cp37-cp37m-macosx_10_9_x86_64.whl", hash = "sha256:c57921cda3a80d0f2b8aec7e25c8aa14479ea92b5b51b6876d975d925a2ea346"},
+ {file = "charset_normalizer-3.2.0-cp37-cp37m-manylinux_2_17_aarch64.manylinux2014_aarch64.whl", hash = "sha256:41b25eaa7d15909cf3ac4c96088c1f266a9a93ec44f87f1d13d4a0e86c81b982"},
+ {file = "charset_normalizer-3.2.0-cp37-cp37m-manylinux_2_17_ppc64le.manylinux2014_ppc64le.whl", hash = "sha256:f058f6963fd82eb143c692cecdc89e075fa0828db2e5b291070485390b2f1c9c"},
+ {file = "charset_normalizer-3.2.0-cp37-cp37m-manylinux_2_17_s390x.manylinux2014_s390x.whl", hash = "sha256:a7647ebdfb9682b7bb97e2a5e7cb6ae735b1c25008a70b906aecca294ee96cf4"},
+ {file = "charset_normalizer-3.2.0-cp37-cp37m-manylinux_2_17_x86_64.manylinux2014_x86_64.whl", hash = "sha256:eef9df1eefada2c09a5e7a40991b9fc6ac6ef20b1372abd48d2794a316dc0449"},
+ {file = "charset_normalizer-3.2.0-cp37-cp37m-manylinux_2_5_i686.manylinux1_i686.manylinux_2_17_i686.manylinux2014_i686.whl", hash = "sha256:e03b8895a6990c9ab2cdcd0f2fe44088ca1c65ae592b8f795c3294af00a461c3"},
+ {file = "charset_normalizer-3.2.0-cp37-cp37m-musllinux_1_1_aarch64.whl", hash = "sha256:ee4006268ed33370957f55bf2e6f4d263eaf4dc3cfc473d1d90baff6ed36ce4a"},
+ {file = "charset_normalizer-3.2.0-cp37-cp37m-musllinux_1_1_i686.whl", hash = "sha256:c4983bf937209c57240cff65906b18bb35e64ae872da6a0db937d7b4af845dd7"},
+ {file = "charset_normalizer-3.2.0-cp37-cp37m-musllinux_1_1_ppc64le.whl", hash = "sha256:3bb7fda7260735efe66d5107fb7e6af6a7c04c7fce9b2514e04b7a74b06bf5dd"},
+ {file = "charset_normalizer-3.2.0-cp37-cp37m-musllinux_1_1_s390x.whl", hash = "sha256:72814c01533f51d68702802d74f77ea026b5ec52793c791e2da806a3844a46c3"},
+ {file = "charset_normalizer-3.2.0-cp37-cp37m-musllinux_1_1_x86_64.whl", hash = "sha256:70c610f6cbe4b9fce272c407dd9d07e33e6bf7b4aa1b7ffb6f6ded8e634e3592"},
+ {file = "charset_normalizer-3.2.0-cp37-cp37m-win32.whl", hash = "sha256:a401b4598e5d3f4a9a811f3daf42ee2291790c7f9d74b18d75d6e21dda98a1a1"},
+ {file = "charset_normalizer-3.2.0-cp37-cp37m-win_amd64.whl", hash = "sha256:c0b21078a4b56965e2b12f247467b234734491897e99c1d51cee628da9786959"},
+ {file = "charset_normalizer-3.2.0-cp38-cp38-macosx_10_9_universal2.whl", hash = "sha256:95eb302ff792e12aba9a8b8f8474ab229a83c103d74a750ec0bd1c1eea32e669"},
+ {file = "charset_normalizer-3.2.0-cp38-cp38-macosx_10_9_x86_64.whl", hash = "sha256:1a100c6d595a7f316f1b6f01d20815d916e75ff98c27a01ae817439ea7726329"},
+ {file = "charset_normalizer-3.2.0-cp38-cp38-macosx_11_0_arm64.whl", hash = "sha256:6339d047dab2780cc6220f46306628e04d9750f02f983ddb37439ca47ced7149"},
+ {file = "charset_normalizer-3.2.0-cp38-cp38-manylinux_2_17_aarch64.manylinux2014_aarch64.whl", hash = "sha256:e4b749b9cc6ee664a3300bb3a273c1ca8068c46be705b6c31cf5d276f8628a94"},
+ {file = "charset_normalizer-3.2.0-cp38-cp38-manylinux_2_17_ppc64le.manylinux2014_ppc64le.whl", hash = "sha256:a38856a971c602f98472050165cea2cdc97709240373041b69030be15047691f"},
+ {file = "charset_normalizer-3.2.0-cp38-cp38-manylinux_2_17_s390x.manylinux2014_s390x.whl", hash = "sha256:f87f746ee241d30d6ed93969de31e5ffd09a2961a051e60ae6bddde9ec3583aa"},
+ {file = "charset_normalizer-3.2.0-cp38-cp38-manylinux_2_17_x86_64.manylinux2014_x86_64.whl", hash = "sha256:89f1b185a01fe560bc8ae5f619e924407efca2191b56ce749ec84982fc59a32a"},
+ {file = "charset_normalizer-3.2.0-cp38-cp38-manylinux_2_5_i686.manylinux1_i686.manylinux_2_17_i686.manylinux2014_i686.whl", hash = "sha256:e1c8a2f4c69e08e89632defbfabec2feb8a8d99edc9f89ce33c4b9e36ab63037"},
+ {file = "charset_normalizer-3.2.0-cp38-cp38-musllinux_1_1_aarch64.whl", hash = "sha256:2f4ac36d8e2b4cc1aa71df3dd84ff8efbe3bfb97ac41242fbcfc053c67434f46"},
+ {file = "charset_normalizer-3.2.0-cp38-cp38-musllinux_1_1_i686.whl", hash = "sha256:a386ebe437176aab38c041de1260cd3ea459c6ce5263594399880bbc398225b2"},
+ {file = "charset_normalizer-3.2.0-cp38-cp38-musllinux_1_1_ppc64le.whl", hash = "sha256:ccd16eb18a849fd8dcb23e23380e2f0a354e8daa0c984b8a732d9cfaba3a776d"},
+ {file = "charset_normalizer-3.2.0-cp38-cp38-musllinux_1_1_s390x.whl", hash = "sha256:e6a5bf2cba5ae1bb80b154ed68a3cfa2fa00fde979a7f50d6598d3e17d9ac20c"},
+ {file = "charset_normalizer-3.2.0-cp38-cp38-musllinux_1_1_x86_64.whl", hash = "sha256:45de3f87179c1823e6d9e32156fb14c1927fcc9aba21433f088fdfb555b77c10"},
+ {file = "charset_normalizer-3.2.0-cp38-cp38-win32.whl", hash = "sha256:1000fba1057b92a65daec275aec30586c3de2401ccdcd41f8a5c1e2c87078706"},
+ {file = "charset_normalizer-3.2.0-cp38-cp38-win_amd64.whl", hash = "sha256:8b2c760cfc7042b27ebdb4a43a4453bd829a5742503599144d54a032c5dc7e9e"},
+ {file = "charset_normalizer-3.2.0-cp39-cp39-macosx_10_9_universal2.whl", hash = "sha256:855eafa5d5a2034b4621c74925d89c5efef61418570e5ef9b37717d9c796419c"},
+ {file = "charset_normalizer-3.2.0-cp39-cp39-macosx_10_9_x86_64.whl", hash = "sha256:203f0c8871d5a7987be20c72442488a0b8cfd0f43b7973771640fc593f56321f"},
+ {file = "charset_normalizer-3.2.0-cp39-cp39-macosx_11_0_arm64.whl", hash = "sha256:e857a2232ba53ae940d3456f7533ce6ca98b81917d47adc3c7fd55dad8fab858"},
+ {file = "charset_normalizer-3.2.0-cp39-cp39-manylinux_2_17_aarch64.manylinux2014_aarch64.whl", hash = "sha256:5e86d77b090dbddbe78867a0275cb4df08ea195e660f1f7f13435a4649e954e5"},
+ {file = "charset_normalizer-3.2.0-cp39-cp39-manylinux_2_17_ppc64le.manylinux2014_ppc64le.whl", hash = "sha256:c4fb39a81950ec280984b3a44f5bd12819953dc5fa3a7e6fa7a80db5ee853952"},
+ {file = "charset_normalizer-3.2.0-cp39-cp39-manylinux_2_17_s390x.manylinux2014_s390x.whl", hash = "sha256:2dee8e57f052ef5353cf608e0b4c871aee320dd1b87d351c28764fc0ca55f9f4"},
+ {file = "charset_normalizer-3.2.0-cp39-cp39-manylinux_2_17_x86_64.manylinux2014_x86_64.whl", hash = "sha256:8700f06d0ce6f128de3ccdbc1acaea1ee264d2caa9ca05daaf492fde7c2a7200"},
+ {file = "charset_normalizer-3.2.0-cp39-cp39-manylinux_2_5_i686.manylinux1_i686.manylinux_2_17_i686.manylinux2014_i686.whl", hash = "sha256:1920d4ff15ce893210c1f0c0e9d19bfbecb7983c76b33f046c13a8ffbd570252"},
+ {file = "charset_normalizer-3.2.0-cp39-cp39-musllinux_1_1_aarch64.whl", hash = "sha256:c1c76a1743432b4b60ab3358c937a3fe1341c828ae6194108a94c69028247f22"},
+ {file = "charset_normalizer-3.2.0-cp39-cp39-musllinux_1_1_i686.whl", hash = "sha256:f7560358a6811e52e9c4d142d497f1a6e10103d3a6881f18d04dbce3729c0e2c"},
+ {file = "charset_normalizer-3.2.0-cp39-cp39-musllinux_1_1_ppc64le.whl", hash = "sha256:c8063cf17b19661471ecbdb3df1c84f24ad2e389e326ccaf89e3fb2484d8dd7e"},
+ {file = "charset_normalizer-3.2.0-cp39-cp39-musllinux_1_1_s390x.whl", hash = "sha256:cd6dbe0238f7743d0efe563ab46294f54f9bc8f4b9bcf57c3c666cc5bc9d1299"},
+ {file = "charset_normalizer-3.2.0-cp39-cp39-musllinux_1_1_x86_64.whl", hash = "sha256:1249cbbf3d3b04902ff081ffbb33ce3377fa6e4c7356f759f3cd076cc138d020"},
+ {file = "charset_normalizer-3.2.0-cp39-cp39-win32.whl", hash = "sha256:6c409c0deba34f147f77efaa67b8e4bb83d2f11c8806405f76397ae5b8c0d1c9"},
+ {file = "charset_normalizer-3.2.0-cp39-cp39-win_amd64.whl", hash = "sha256:7095f6fbfaa55defb6b733cfeb14efaae7a29f0b59d8cf213be4e7ca0b857b80"},
+ {file = "charset_normalizer-3.2.0-py3-none-any.whl", hash = "sha256:8e098148dd37b4ce3baca71fb394c81dc5d9c7728c95df695d2dca218edf40e6"},
+]
+
+[[package]]
+name = "colorama"
+version = "0.4.6"
+description = "Cross-platform colored terminal text."
+optional = false
+python-versions = "!=3.0.*,!=3.1.*,!=3.2.*,!=3.3.*,!=3.4.*,!=3.5.*,!=3.6.*,>=2.7"
+files = [
+ {file = "colorama-0.4.6-py2.py3-none-any.whl", hash = "sha256:4f1d9991f5acc0ca119f9d443620b77f9d6b33703e51011c16baf57afb285fc6"},
+ {file = "colorama-0.4.6.tar.gz", hash = "sha256:08695f5cb7ed6e0531a20572697297273c47b8cae5a63ffc6d6ed5c201be6e44"},
+]
+
+[[package]]
+name = "coverage"
+version = "7.2.7"
+description = "Code coverage measurement for Python"
+optional = false
+python-versions = ">=3.7"
+files = [
+ {file = "coverage-7.2.7-cp310-cp310-macosx_10_9_x86_64.whl", hash = "sha256:d39b5b4f2a66ccae8b7263ac3c8170994b65266797fb96cbbfd3fb5b23921db8"},
+ {file = "coverage-7.2.7-cp310-cp310-macosx_11_0_arm64.whl", hash = "sha256:6d040ef7c9859bb11dfeb056ff5b3872436e3b5e401817d87a31e1750b9ae2fb"},
+ {file = "coverage-7.2.7-cp310-cp310-manylinux_2_17_aarch64.manylinux2014_aarch64.whl", hash = "sha256:ba90a9563ba44a72fda2e85302c3abc71c5589cea608ca16c22b9804262aaeb6"},
+ {file = "coverage-7.2.7-cp310-cp310-manylinux_2_5_i686.manylinux1_i686.manylinux_2_17_i686.manylinux2014_i686.whl", hash = "sha256:e7d9405291c6928619403db1d10bd07888888ec1abcbd9748fdaa971d7d661b2"},
+ {file = "coverage-7.2.7-cp310-cp310-manylinux_2_5_x86_64.manylinux1_x86_64.manylinux_2_17_x86_64.manylinux2014_x86_64.whl", hash = "sha256:31563e97dae5598556600466ad9beea39fb04e0229e61c12eaa206e0aa202063"},
+ {file = "coverage-7.2.7-cp310-cp310-musllinux_1_1_aarch64.whl", hash = "sha256:ebba1cd308ef115925421d3e6a586e655ca5a77b5bf41e02eb0e4562a111f2d1"},
+ {file = "coverage-7.2.7-cp310-cp310-musllinux_1_1_i686.whl", hash = "sha256:cb017fd1b2603ef59e374ba2063f593abe0fc45f2ad9abdde5b4d83bd922a353"},
+ {file = "coverage-7.2.7-cp310-cp310-musllinux_1_1_x86_64.whl", hash = "sha256:d62a5c7dad11015c66fbb9d881bc4caa5b12f16292f857842d9d1871595f4495"},
+ {file = "coverage-7.2.7-cp310-cp310-win32.whl", hash = "sha256:ee57190f24fba796e36bb6d3aa8a8783c643d8fa9760c89f7a98ab5455fbf818"},
+ {file = "coverage-7.2.7-cp310-cp310-win_amd64.whl", hash = "sha256:f75f7168ab25dd93110c8a8117a22450c19976afbc44234cbf71481094c1b850"},
+ {file = "coverage-7.2.7-cp311-cp311-macosx_10_9_x86_64.whl", hash = "sha256:06a9a2be0b5b576c3f18f1a241f0473575c4a26021b52b2a85263a00f034d51f"},
+ {file = "coverage-7.2.7-cp311-cp311-macosx_11_0_arm64.whl", hash = "sha256:5baa06420f837184130752b7c5ea0808762083bf3487b5038d68b012e5937dbe"},
+ {file = "coverage-7.2.7-cp311-cp311-manylinux_2_17_aarch64.manylinux2014_aarch64.whl", hash = "sha256:fdec9e8cbf13a5bf63290fc6013d216a4c7232efb51548594ca3631a7f13c3a3"},
+ {file = "coverage-7.2.7-cp311-cp311-manylinux_2_5_i686.manylinux1_i686.manylinux_2_17_i686.manylinux2014_i686.whl", hash = "sha256:52edc1a60c0d34afa421c9c37078817b2e67a392cab17d97283b64c5833f427f"},
+ {file = "coverage-7.2.7-cp311-cp311-manylinux_2_5_x86_64.manylinux1_x86_64.manylinux_2_17_x86_64.manylinux2014_x86_64.whl", hash = "sha256:63426706118b7f5cf6bb6c895dc215d8a418d5952544042c8a2d9fe87fcf09cb"},
+ {file = "coverage-7.2.7-cp311-cp311-musllinux_1_1_aarch64.whl", hash = "sha256:afb17f84d56068a7c29f5fa37bfd38d5aba69e3304af08ee94da8ed5b0865833"},
+ {file = "coverage-7.2.7-cp311-cp311-musllinux_1_1_i686.whl", hash = "sha256:48c19d2159d433ccc99e729ceae7d5293fbffa0bdb94952d3579983d1c8c9d97"},
+ {file = "coverage-7.2.7-cp311-cp311-musllinux_1_1_x86_64.whl", hash = "sha256:0e1f928eaf5469c11e886fe0885ad2bf1ec606434e79842a879277895a50942a"},
+ {file = "coverage-7.2.7-cp311-cp311-win32.whl", hash = "sha256:33d6d3ea29d5b3a1a632b3c4e4f4ecae24ef170b0b9ee493883f2df10039959a"},
+ {file = "coverage-7.2.7-cp311-cp311-win_amd64.whl", hash = "sha256:5b7540161790b2f28143191f5f8ec02fb132660ff175b7747b95dcb77ac26562"},
+ {file = "coverage-7.2.7-cp312-cp312-macosx_10_9_x86_64.whl", hash = "sha256:f2f67fe12b22cd130d34d0ef79206061bfb5eda52feb6ce0dba0644e20a03cf4"},
+ {file = "coverage-7.2.7-cp312-cp312-manylinux_2_17_aarch64.manylinux2014_aarch64.whl", hash = "sha256:a342242fe22407f3c17f4b499276a02b01e80f861f1682ad1d95b04018e0c0d4"},
+ {file = "coverage-7.2.7-cp312-cp312-manylinux_2_5_i686.manylinux1_i686.manylinux_2_17_i686.manylinux2014_i686.whl", hash = "sha256:171717c7cb6b453aebac9a2ef603699da237f341b38eebfee9be75d27dc38e01"},
+ {file = "coverage-7.2.7-cp312-cp312-manylinux_2_5_x86_64.manylinux1_x86_64.manylinux_2_17_x86_64.manylinux2014_x86_64.whl", hash = "sha256:49969a9f7ffa086d973d91cec8d2e31080436ef0fb4a359cae927e742abfaaa6"},
+ {file = "coverage-7.2.7-cp312-cp312-musllinux_1_1_aarch64.whl", hash = "sha256:b46517c02ccd08092f4fa99f24c3b83d8f92f739b4657b0f146246a0ca6a831d"},
+ {file = "coverage-7.2.7-cp312-cp312-musllinux_1_1_i686.whl", hash = "sha256:a3d33a6b3eae87ceaefa91ffdc130b5e8536182cd6dfdbfc1aa56b46ff8c86de"},
+ {file = "coverage-7.2.7-cp312-cp312-musllinux_1_1_x86_64.whl", hash = "sha256:976b9c42fb2a43ebf304fa7d4a310e5f16cc99992f33eced91ef6f908bd8f33d"},
+ {file = "coverage-7.2.7-cp312-cp312-win32.whl", hash = "sha256:8de8bb0e5ad103888d65abef8bca41ab93721647590a3f740100cd65c3b00511"},
+ {file = "coverage-7.2.7-cp312-cp312-win_amd64.whl", hash = "sha256:9e31cb64d7de6b6f09702bb27c02d1904b3aebfca610c12772452c4e6c21a0d3"},
+ {file = "coverage-7.2.7-cp37-cp37m-macosx_10_9_x86_64.whl", hash = "sha256:58c2ccc2f00ecb51253cbe5d8d7122a34590fac9646a960d1430d5b15321d95f"},
+ {file = "coverage-7.2.7-cp37-cp37m-manylinux_2_17_aarch64.manylinux2014_aarch64.whl", hash = "sha256:d22656368f0e6189e24722214ed8d66b8022db19d182927b9a248a2a8a2f67eb"},
+ {file = "coverage-7.2.7-cp37-cp37m-manylinux_2_5_i686.manylinux1_i686.manylinux_2_17_i686.manylinux2014_i686.whl", hash = "sha256:a895fcc7b15c3fc72beb43cdcbdf0ddb7d2ebc959edac9cef390b0d14f39f8a9"},
+ {file = "coverage-7.2.7-cp37-cp37m-manylinux_2_5_x86_64.manylinux1_x86_64.manylinux_2_17_x86_64.manylinux2014_x86_64.whl", hash = "sha256:e84606b74eb7de6ff581a7915e2dab7a28a0517fbe1c9239eb227e1354064dcd"},
+ {file = "coverage-7.2.7-cp37-cp37m-musllinux_1_1_aarch64.whl", hash = "sha256:0a5f9e1dbd7fbe30196578ca36f3fba75376fb99888c395c5880b355e2875f8a"},
+ {file = "coverage-7.2.7-cp37-cp37m-musllinux_1_1_i686.whl", hash = "sha256:419bfd2caae268623dd469eff96d510a920c90928b60f2073d79f8fe2bbc5959"},
+ {file = "coverage-7.2.7-cp37-cp37m-musllinux_1_1_x86_64.whl", hash = "sha256:2aee274c46590717f38ae5e4650988d1af340fe06167546cc32fe2f58ed05b02"},
+ {file = "coverage-7.2.7-cp37-cp37m-win32.whl", hash = "sha256:61b9a528fb348373c433e8966535074b802c7a5d7f23c4f421e6c6e2f1697a6f"},
+ {file = "coverage-7.2.7-cp37-cp37m-win_amd64.whl", hash = "sha256:b1c546aca0ca4d028901d825015dc8e4d56aac4b541877690eb76490f1dc8ed0"},
+ {file = "coverage-7.2.7-cp38-cp38-macosx_10_9_x86_64.whl", hash = "sha256:54b896376ab563bd38453cecb813c295cf347cf5906e8b41d340b0321a5433e5"},
+ {file = "coverage-7.2.7-cp38-cp38-macosx_11_0_arm64.whl", hash = "sha256:3d376df58cc111dc8e21e3b6e24606b5bb5dee6024f46a5abca99124b2229ef5"},
+ {file = "coverage-7.2.7-cp38-cp38-manylinux_2_17_aarch64.manylinux2014_aarch64.whl", hash = "sha256:5e330fc79bd7207e46c7d7fd2bb4af2963f5f635703925543a70b99574b0fea9"},
+ {file = "coverage-7.2.7-cp38-cp38-manylinux_2_5_i686.manylinux1_i686.manylinux_2_17_i686.manylinux2014_i686.whl", hash = "sha256:1e9d683426464e4a252bf70c3498756055016f99ddaec3774bf368e76bbe02b6"},
+ {file = "coverage-7.2.7-cp38-cp38-manylinux_2_5_x86_64.manylinux1_x86_64.manylinux_2_17_x86_64.manylinux2014_x86_64.whl", hash = "sha256:8d13c64ee2d33eccf7437961b6ea7ad8673e2be040b4f7fd4fd4d4d28d9ccb1e"},
+ {file = "coverage-7.2.7-cp38-cp38-musllinux_1_1_aarch64.whl", hash = "sha256:b7aa5f8a41217360e600da646004f878250a0d6738bcdc11a0a39928d7dc2050"},
+ {file = "coverage-7.2.7-cp38-cp38-musllinux_1_1_i686.whl", hash = "sha256:8fa03bce9bfbeeef9f3b160a8bed39a221d82308b4152b27d82d8daa7041fee5"},
+ {file = "coverage-7.2.7-cp38-cp38-musllinux_1_1_x86_64.whl", hash = "sha256:245167dd26180ab4c91d5e1496a30be4cd721a5cf2abf52974f965f10f11419f"},
+ {file = "coverage-7.2.7-cp38-cp38-win32.whl", hash = "sha256:d2c2db7fd82e9b72937969bceac4d6ca89660db0a0967614ce2481e81a0b771e"},
+ {file = "coverage-7.2.7-cp38-cp38-win_amd64.whl", hash = "sha256:2e07b54284e381531c87f785f613b833569c14ecacdcb85d56b25c4622c16c3c"},
+ {file = "coverage-7.2.7-cp39-cp39-macosx_10_9_x86_64.whl", hash = "sha256:537891ae8ce59ef63d0123f7ac9e2ae0fc8b72c7ccbe5296fec45fd68967b6c9"},
+ {file = "coverage-7.2.7-cp39-cp39-macosx_11_0_arm64.whl", hash = "sha256:06fb182e69f33f6cd1d39a6c597294cff3143554b64b9825d1dc69d18cc2fff2"},
+ {file = "coverage-7.2.7-cp39-cp39-manylinux_2_17_aarch64.manylinux2014_aarch64.whl", hash = "sha256:201e7389591af40950a6480bd9edfa8ed04346ff80002cec1a66cac4549c1ad7"},
+ {file = "coverage-7.2.7-cp39-cp39-manylinux_2_5_i686.manylinux1_i686.manylinux_2_17_i686.manylinux2014_i686.whl", hash = "sha256:f6951407391b639504e3b3be51b7ba5f3528adbf1a8ac3302b687ecababf929e"},
+ {file = "coverage-7.2.7-cp39-cp39-manylinux_2_5_x86_64.manylinux1_x86_64.manylinux_2_17_x86_64.manylinux2014_x86_64.whl", hash = "sha256:6f48351d66575f535669306aa7d6d6f71bc43372473b54a832222803eb956fd1"},
+ {file = "coverage-7.2.7-cp39-cp39-musllinux_1_1_aarch64.whl", hash = "sha256:b29019c76039dc3c0fd815c41392a044ce555d9bcdd38b0fb60fb4cd8e475ba9"},
+ {file = "coverage-7.2.7-cp39-cp39-musllinux_1_1_i686.whl", hash = "sha256:81c13a1fc7468c40f13420732805a4c38a105d89848b7c10af65a90beff25250"},
+ {file = "coverage-7.2.7-cp39-cp39-musllinux_1_1_x86_64.whl", hash = "sha256:975d70ab7e3c80a3fe86001d8751f6778905ec723f5b110aed1e450da9d4b7f2"},
+ {file = "coverage-7.2.7-cp39-cp39-win32.whl", hash = "sha256:7ee7d9d4822c8acc74a5e26c50604dff824710bc8de424904c0982e25c39c6cb"},
+ {file = "coverage-7.2.7-cp39-cp39-win_amd64.whl", hash = "sha256:eb393e5ebc85245347950143969b241d08b52b88a3dc39479822e073a1a8eb27"},
+ {file = "coverage-7.2.7-pp37.pp38.pp39-none-any.whl", hash = "sha256:b7b4c971f05e6ae490fef852c218b0e79d4e52f79ef0c8475566584a8fb3e01d"},
+ {file = "coverage-7.2.7.tar.gz", hash = "sha256:924d94291ca674905fe9481f12294eb11f2d3d3fd1adb20314ba89e94f44ed59"},
+]
+
+[package.dependencies]
+tomli = {version = "*", optional = true, markers = "python_full_version <= \"3.11.0a6\" and extra == \"toml\""}
+
+[package.extras]
+toml = ["tomli"]
+
+[[package]]
+name = "filelock"
+version = "3.12.2"
+description = "A platform independent file lock."
+optional = false
+python-versions = ">=3.7"
+files = [
+ {file = "filelock-3.12.2-py3-none-any.whl", hash = "sha256:cbb791cdea2a72f23da6ac5b5269ab0a0d161e9ef0100e653b69049a7706d1ec"},
+ {file = "filelock-3.12.2.tar.gz", hash = "sha256:002740518d8aa59a26b0c76e10fb8c6e15eae825d34b6fdf670333fd7b938d81"},
+]
+
+[package.extras]
+docs = ["furo (>=2023.5.20)", "sphinx (>=7.0.1)", "sphinx-autodoc-typehints (>=1.23,!=1.23.4)"]
+testing = ["covdefaults (>=2.3)", "coverage (>=7.2.7)", "diff-cover (>=7.5)", "pytest (>=7.3.1)", "pytest-cov (>=4.1)", "pytest-mock (>=3.10)", "pytest-timeout (>=2.1)"]
+
+[[package]]
+name = "frozenlist"
+version = "1.3.3"
+description = "A list-like structure which implements collections.abc.MutableSequence"
+optional = false
+python-versions = ">=3.7"
+files = [
+ {file = "frozenlist-1.3.3-cp310-cp310-macosx_10_9_universal2.whl", hash = "sha256:ff8bf625fe85e119553b5383ba0fb6aa3d0ec2ae980295aaefa552374926b3f4"},
+ {file = "frozenlist-1.3.3-cp310-cp310-macosx_10_9_x86_64.whl", hash = "sha256:dfbac4c2dfcc082fcf8d942d1e49b6aa0766c19d3358bd86e2000bf0fa4a9cf0"},
+ {file = "frozenlist-1.3.3-cp310-cp310-macosx_11_0_arm64.whl", hash = "sha256:b1c63e8d377d039ac769cd0926558bb7068a1f7abb0f003e3717ee003ad85530"},
+ {file = "frozenlist-1.3.3-cp310-cp310-manylinux_2_17_aarch64.manylinux2014_aarch64.whl", hash = "sha256:7fdfc24dcfce5b48109867c13b4cb15e4660e7bd7661741a391f821f23dfdca7"},
+ {file = "frozenlist-1.3.3-cp310-cp310-manylinux_2_17_ppc64le.manylinux2014_ppc64le.whl", hash = "sha256:2c926450857408e42f0bbc295e84395722ce74bae69a3b2aa2a65fe22cb14b99"},
+ {file = "frozenlist-1.3.3-cp310-cp310-manylinux_2_17_s390x.manylinux2014_s390x.whl", hash = "sha256:1841e200fdafc3d51f974d9d377c079a0694a8f06de2e67b48150328d66d5483"},
+ {file = "frozenlist-1.3.3-cp310-cp310-manylinux_2_5_i686.manylinux1_i686.manylinux_2_17_i686.manylinux2014_i686.whl", hash = "sha256:f470c92737afa7d4c3aacc001e335062d582053d4dbe73cda126f2d7031068dd"},
+ {file = "frozenlist-1.3.3-cp310-cp310-manylinux_2_5_x86_64.manylinux1_x86_64.manylinux_2_17_x86_64.manylinux2014_x86_64.whl", hash = "sha256:783263a4eaad7c49983fe4b2e7b53fa9770c136c270d2d4bbb6d2192bf4d9caf"},
+ {file = "frozenlist-1.3.3-cp310-cp310-musllinux_1_1_aarch64.whl", hash = "sha256:924620eef691990dfb56dc4709f280f40baee568c794b5c1885800c3ecc69816"},
+ {file = "frozenlist-1.3.3-cp310-cp310-musllinux_1_1_i686.whl", hash = "sha256:ae4dc05c465a08a866b7a1baf360747078b362e6a6dbeb0c57f234db0ef88ae0"},
+ {file = "frozenlist-1.3.3-cp310-cp310-musllinux_1_1_ppc64le.whl", hash = "sha256:bed331fe18f58d844d39ceb398b77d6ac0b010d571cba8267c2e7165806b00ce"},
+ {file = "frozenlist-1.3.3-cp310-cp310-musllinux_1_1_s390x.whl", hash = "sha256:02c9ac843e3390826a265e331105efeab489ffaf4dd86384595ee8ce6d35ae7f"},
+ {file = "frozenlist-1.3.3-cp310-cp310-musllinux_1_1_x86_64.whl", hash = "sha256:9545a33965d0d377b0bc823dcabf26980e77f1b6a7caa368a365a9497fb09420"},
+ {file = "frozenlist-1.3.3-cp310-cp310-win32.whl", hash = "sha256:d5cd3ab21acbdb414bb6c31958d7b06b85eeb40f66463c264a9b343a4e238642"},
+ {file = "frozenlist-1.3.3-cp310-cp310-win_amd64.whl", hash = "sha256:b756072364347cb6aa5b60f9bc18e94b2f79632de3b0190253ad770c5df17db1"},
+ {file = "frozenlist-1.3.3-cp311-cp311-macosx_10_9_universal2.whl", hash = "sha256:b4395e2f8d83fbe0c627b2b696acce67868793d7d9750e90e39592b3626691b7"},
+ {file = "frozenlist-1.3.3-cp311-cp311-macosx_10_9_x86_64.whl", hash = "sha256:14143ae966a6229350021384870458e4777d1eae4c28d1a7aa47f24d030e6678"},
+ {file = "frozenlist-1.3.3-cp311-cp311-macosx_11_0_arm64.whl", hash = "sha256:5d8860749e813a6f65bad8285a0520607c9500caa23fea6ee407e63debcdbef6"},
+ {file = "frozenlist-1.3.3-cp311-cp311-manylinux_2_17_aarch64.manylinux2014_aarch64.whl", hash = "sha256:23d16d9f477bb55b6154654e0e74557040575d9d19fe78a161bd33d7d76808e8"},
+ {file = "frozenlist-1.3.3-cp311-cp311-manylinux_2_17_ppc64le.manylinux2014_ppc64le.whl", hash = "sha256:eb82dbba47a8318e75f679690190c10a5e1f447fbf9df41cbc4c3afd726d88cb"},
+ {file = "frozenlist-1.3.3-cp311-cp311-manylinux_2_17_s390x.manylinux2014_s390x.whl", hash = "sha256:9309869032abb23d196cb4e4db574232abe8b8be1339026f489eeb34a4acfd91"},
+ {file = "frozenlist-1.3.3-cp311-cp311-manylinux_2_5_i686.manylinux1_i686.manylinux_2_17_i686.manylinux2014_i686.whl", hash = "sha256:a97b4fe50b5890d36300820abd305694cb865ddb7885049587a5678215782a6b"},
+ {file = "frozenlist-1.3.3-cp311-cp311-manylinux_2_5_x86_64.manylinux1_x86_64.manylinux_2_17_x86_64.manylinux2014_x86_64.whl", hash = "sha256:c188512b43542b1e91cadc3c6c915a82a5eb95929134faf7fd109f14f9892ce4"},
+ {file = "frozenlist-1.3.3-cp311-cp311-musllinux_1_1_aarch64.whl", hash = "sha256:303e04d422e9b911a09ad499b0368dc551e8c3cd15293c99160c7f1f07b59a48"},
+ {file = "frozenlist-1.3.3-cp311-cp311-musllinux_1_1_i686.whl", hash = "sha256:0771aed7f596c7d73444c847a1c16288937ef988dc04fb9f7be4b2aa91db609d"},
+ {file = "frozenlist-1.3.3-cp311-cp311-musllinux_1_1_ppc64le.whl", hash = "sha256:66080ec69883597e4d026f2f71a231a1ee9887835902dbe6b6467d5a89216cf6"},
+ {file = "frozenlist-1.3.3-cp311-cp311-musllinux_1_1_s390x.whl", hash = "sha256:41fe21dc74ad3a779c3d73a2786bdf622ea81234bdd4faf90b8b03cad0c2c0b4"},
+ {file = "frozenlist-1.3.3-cp311-cp311-musllinux_1_1_x86_64.whl", hash = "sha256:f20380df709d91525e4bee04746ba612a4df0972c1b8f8e1e8af997e678c7b81"},
+ {file = "frozenlist-1.3.3-cp311-cp311-win32.whl", hash = "sha256:f30f1928162e189091cf4d9da2eac617bfe78ef907a761614ff577ef4edfb3c8"},
+ {file = "frozenlist-1.3.3-cp311-cp311-win_amd64.whl", hash = "sha256:a6394d7dadd3cfe3f4b3b186e54d5d8504d44f2d58dcc89d693698e8b7132b32"},
+ {file = "frozenlist-1.3.3-cp37-cp37m-macosx_10_9_x86_64.whl", hash = "sha256:8df3de3a9ab8325f94f646609a66cbeeede263910c5c0de0101079ad541af332"},
+ {file = "frozenlist-1.3.3-cp37-cp37m-manylinux_2_17_aarch64.manylinux2014_aarch64.whl", hash = "sha256:0693c609e9742c66ba4870bcee1ad5ff35462d5ffec18710b4ac89337ff16e27"},
+ {file = "frozenlist-1.3.3-cp37-cp37m-manylinux_2_17_ppc64le.manylinux2014_ppc64le.whl", hash = "sha256:cd4210baef299717db0a600d7a3cac81d46ef0e007f88c9335db79f8979c0d3d"},
+ {file = "frozenlist-1.3.3-cp37-cp37m-manylinux_2_17_s390x.manylinux2014_s390x.whl", hash = "sha256:394c9c242113bfb4b9aa36e2b80a05ffa163a30691c7b5a29eba82e937895d5e"},
+ {file = "frozenlist-1.3.3-cp37-cp37m-manylinux_2_5_i686.manylinux1_i686.manylinux_2_17_i686.manylinux2014_i686.whl", hash = "sha256:6327eb8e419f7d9c38f333cde41b9ae348bec26d840927332f17e887a8dcb70d"},
+ {file = "frozenlist-1.3.3-cp37-cp37m-manylinux_2_5_x86_64.manylinux1_x86_64.manylinux_2_17_x86_64.manylinux2014_x86_64.whl", hash = "sha256:2e24900aa13212e75e5b366cb9065e78bbf3893d4baab6052d1aca10d46d944c"},
+ {file = "frozenlist-1.3.3-cp37-cp37m-musllinux_1_1_aarch64.whl", hash = "sha256:3843f84a6c465a36559161e6c59dce2f2ac10943040c2fd021cfb70d58c4ad56"},
+ {file = "frozenlist-1.3.3-cp37-cp37m-musllinux_1_1_i686.whl", hash = "sha256:84610c1502b2461255b4c9b7d5e9c48052601a8957cd0aea6ec7a7a1e1fb9420"},
+ {file = "frozenlist-1.3.3-cp37-cp37m-musllinux_1_1_ppc64le.whl", hash = "sha256:c21b9aa40e08e4f63a2f92ff3748e6b6c84d717d033c7b3438dd3123ee18f70e"},
+ {file = "frozenlist-1.3.3-cp37-cp37m-musllinux_1_1_s390x.whl", hash = "sha256:efce6ae830831ab6a22b9b4091d411698145cb9b8fc869e1397ccf4b4b6455cb"},
+ {file = "frozenlist-1.3.3-cp37-cp37m-musllinux_1_1_x86_64.whl", hash = "sha256:40de71985e9042ca00b7953c4f41eabc3dc514a2d1ff534027f091bc74416401"},
+ {file = "frozenlist-1.3.3-cp37-cp37m-win32.whl", hash = "sha256:180c00c66bde6146a860cbb81b54ee0df350d2daf13ca85b275123bbf85de18a"},
+ {file = "frozenlist-1.3.3-cp37-cp37m-win_amd64.whl", hash = "sha256:9bbbcedd75acdfecf2159663b87f1bb5cfc80e7cd99f7ddd9d66eb98b14a8411"},
+ {file = "frozenlist-1.3.3-cp38-cp38-macosx_10_9_universal2.whl", hash = "sha256:034a5c08d36649591be1cbb10e09da9f531034acfe29275fc5454a3b101ce41a"},
+ {file = "frozenlist-1.3.3-cp38-cp38-macosx_10_9_x86_64.whl", hash = "sha256:ba64dc2b3b7b158c6660d49cdb1d872d1d0bf4e42043ad8d5006099479a194e5"},
+ {file = "frozenlist-1.3.3-cp38-cp38-macosx_11_0_arm64.whl", hash = "sha256:47df36a9fe24054b950bbc2db630d508cca3aa27ed0566c0baf661225e52c18e"},
+ {file = "frozenlist-1.3.3-cp38-cp38-manylinux_2_17_aarch64.manylinux2014_aarch64.whl", hash = "sha256:008a054b75d77c995ea26629ab3a0c0d7281341f2fa7e1e85fa6153ae29ae99c"},
+ {file = "frozenlist-1.3.3-cp38-cp38-manylinux_2_17_ppc64le.manylinux2014_ppc64le.whl", hash = "sha256:841ea19b43d438a80b4de62ac6ab21cfe6827bb8a9dc62b896acc88eaf9cecba"},
+ {file = "frozenlist-1.3.3-cp38-cp38-manylinux_2_17_s390x.manylinux2014_s390x.whl", hash = "sha256:e235688f42b36be2b6b06fc37ac2126a73b75fb8d6bc66dd632aa35286238703"},
+ {file = "frozenlist-1.3.3-cp38-cp38-manylinux_2_5_i686.manylinux1_i686.manylinux_2_17_i686.manylinux2014_i686.whl", hash = "sha256:ca713d4af15bae6e5d79b15c10c8522859a9a89d3b361a50b817c98c2fb402a2"},
+ {file = "frozenlist-1.3.3-cp38-cp38-manylinux_2_5_x86_64.manylinux1_x86_64.manylinux_2_17_x86_64.manylinux2014_x86_64.whl", hash = "sha256:9ac5995f2b408017b0be26d4a1d7c61bce106ff3d9e3324374d66b5964325448"},
+ {file = "frozenlist-1.3.3-cp38-cp38-musllinux_1_1_aarch64.whl", hash = "sha256:a4ae8135b11652b08a8baf07631d3ebfe65a4c87909dbef5fa0cdde440444ee4"},
+ {file = "frozenlist-1.3.3-cp38-cp38-musllinux_1_1_i686.whl", hash = "sha256:4ea42116ceb6bb16dbb7d526e242cb6747b08b7710d9782aa3d6732bd8d27649"},
+ {file = "frozenlist-1.3.3-cp38-cp38-musllinux_1_1_ppc64le.whl", hash = "sha256:810860bb4bdce7557bc0febb84bbd88198b9dbc2022d8eebe5b3590b2ad6c842"},
+ {file = "frozenlist-1.3.3-cp38-cp38-musllinux_1_1_s390x.whl", hash = "sha256:ee78feb9d293c323b59a6f2dd441b63339a30edf35abcb51187d2fc26e696d13"},
+ {file = "frozenlist-1.3.3-cp38-cp38-musllinux_1_1_x86_64.whl", hash = "sha256:0af2e7c87d35b38732e810befb9d797a99279cbb85374d42ea61c1e9d23094b3"},
+ {file = "frozenlist-1.3.3-cp38-cp38-win32.whl", hash = "sha256:899c5e1928eec13fd6f6d8dc51be23f0d09c5281e40d9cf4273d188d9feeaf9b"},
+ {file = "frozenlist-1.3.3-cp38-cp38-win_amd64.whl", hash = "sha256:7f44e24fa70f6fbc74aeec3e971f60a14dde85da364aa87f15d1be94ae75aeef"},
+ {file = "frozenlist-1.3.3-cp39-cp39-macosx_10_9_universal2.whl", hash = "sha256:2b07ae0c1edaa0a36339ec6cce700f51b14a3fc6545fdd32930d2c83917332cf"},
+ {file = "frozenlist-1.3.3-cp39-cp39-macosx_10_9_x86_64.whl", hash = "sha256:ebb86518203e12e96af765ee89034a1dbb0c3c65052d1b0c19bbbd6af8a145e1"},
+ {file = "frozenlist-1.3.3-cp39-cp39-macosx_11_0_arm64.whl", hash = "sha256:5cf820485f1b4c91e0417ea0afd41ce5cf5965011b3c22c400f6d144296ccbc0"},
+ {file = "frozenlist-1.3.3-cp39-cp39-manylinux_2_17_aarch64.manylinux2014_aarch64.whl", hash = "sha256:5c11e43016b9024240212d2a65043b70ed8dfd3b52678a1271972702d990ac6d"},
+ {file = "frozenlist-1.3.3-cp39-cp39-manylinux_2_17_ppc64le.manylinux2014_ppc64le.whl", hash = "sha256:8fa3c6e3305aa1146b59a09b32b2e04074945ffcfb2f0931836d103a2c38f936"},
+ {file = "frozenlist-1.3.3-cp39-cp39-manylinux_2_17_s390x.manylinux2014_s390x.whl", hash = "sha256:352bd4c8c72d508778cf05ab491f6ef36149f4d0cb3c56b1b4302852255d05d5"},
+ {file = "frozenlist-1.3.3-cp39-cp39-manylinux_2_5_i686.manylinux1_i686.manylinux_2_17_i686.manylinux2014_i686.whl", hash = "sha256:65a5e4d3aa679610ac6e3569e865425b23b372277f89b5ef06cf2cdaf1ebf22b"},
+ {file = "frozenlist-1.3.3-cp39-cp39-manylinux_2_5_x86_64.manylinux1_x86_64.manylinux_2_17_x86_64.manylinux2014_x86_64.whl", hash = "sha256:b1e2c1185858d7e10ff045c496bbf90ae752c28b365fef2c09cf0fa309291669"},
+ {file = "frozenlist-1.3.3-cp39-cp39-musllinux_1_1_aarch64.whl", hash = "sha256:f163d2fd041c630fed01bc48d28c3ed4a3b003c00acd396900e11ee5316b56bb"},
+ {file = "frozenlist-1.3.3-cp39-cp39-musllinux_1_1_i686.whl", hash = "sha256:05cdb16d09a0832eedf770cb7bd1fe57d8cf4eaf5aced29c4e41e3f20b30a784"},
+ {file = "frozenlist-1.3.3-cp39-cp39-musllinux_1_1_ppc64le.whl", hash = "sha256:8bae29d60768bfa8fb92244b74502b18fae55a80eac13c88eb0b496d4268fd2d"},
+ {file = "frozenlist-1.3.3-cp39-cp39-musllinux_1_1_s390x.whl", hash = "sha256:eedab4c310c0299961ac285591acd53dc6723a1ebd90a57207c71f6e0c2153ab"},
+ {file = "frozenlist-1.3.3-cp39-cp39-musllinux_1_1_x86_64.whl", hash = "sha256:3bbdf44855ed8f0fbcd102ef05ec3012d6a4fd7c7562403f76ce6a52aeffb2b1"},
+ {file = "frozenlist-1.3.3-cp39-cp39-win32.whl", hash = "sha256:efa568b885bca461f7c7b9e032655c0c143d305bf01c30caf6db2854a4532b38"},
+ {file = "frozenlist-1.3.3-cp39-cp39-win_amd64.whl", hash = "sha256:cfe33efc9cb900a4c46f91a5ceba26d6df370ffddd9ca386eb1d4f0ad97b9ea9"},
+ {file = "frozenlist-1.3.3.tar.gz", hash = "sha256:58bcc55721e8a90b88332d6cd441261ebb22342e238296bb330968952fbb3a6a"},
+]
+
+[[package]]
+name = "fsspec"
+version = "2023.1.0"
+description = "File-system specification"
+optional = false
+python-versions = ">=3.7"
+files = [
+ {file = "fsspec-2023.1.0-py3-none-any.whl", hash = "sha256:b833e2e541e9e8cde0ab549414187871243177feb3d344f9d27b25a93f5d8139"},
+ {file = "fsspec-2023.1.0.tar.gz", hash = "sha256:fbae7f20ff801eb5f7d0bedf81f25c787c0dfac5e982d98fa3884a9cde2b5411"},
+]
+
+[package.extras]
+abfs = ["adlfs"]
+adl = ["adlfs"]
+arrow = ["pyarrow (>=1)"]
+dask = ["dask", "distributed"]
+dropbox = ["dropbox", "dropboxdrivefs", "requests"]
+entrypoints = ["importlib-metadata"]
+fuse = ["fusepy"]
+gcs = ["gcsfs"]
+git = ["pygit2"]
+github = ["requests"]
+gs = ["gcsfs"]
+gui = ["panel"]
+hdfs = ["pyarrow (>=1)"]
+http = ["aiohttp (!=4.0.0a0,!=4.0.0a1)", "requests"]
+libarchive = ["libarchive-c"]
+oci = ["ocifs"]
+s3 = ["s3fs"]
+sftp = ["paramiko"]
+smb = ["smbprotocol"]
+ssh = ["paramiko"]
+tqdm = ["tqdm"]
+
+[[package]]
+name = "huggingface-hub"
+version = "0.16.4"
+description = "Client library to download and publish models, datasets and other repos on the huggingface.co hub"
+optional = false
+python-versions = ">=3.7.0"
+files = [
+ {file = "huggingface_hub-0.16.4-py3-none-any.whl", hash = "sha256:0d3df29932f334fead024afc7cb4cc5149d955238b8b5e42dcf9740d6995a349"},
+ {file = "huggingface_hub-0.16.4.tar.gz", hash = "sha256:608c7d4f3d368b326d1747f91523dbd1f692871e8e2e7a4750314a2dd8b63e14"},
+]
+
+[package.dependencies]
+filelock = "*"
+fsspec = "*"
+importlib-metadata = {version = "*", markers = "python_version < \"3.8\""}
+packaging = ">=20.9"
+pyyaml = ">=5.1"
+requests = "*"
+tqdm = ">=4.42.1"
+typing-extensions = ">=3.7.4.3"
+
+[package.extras]
+all = ["InquirerPy (==0.3.4)", "Jinja2", "Pillow", "aiohttp", "black (>=23.1,<24.0)", "gradio", "jedi", "mypy (==0.982)", "numpy", "pydantic", "pytest", "pytest-asyncio", "pytest-cov", "pytest-env", "pytest-vcr", "pytest-xdist", "ruff (>=0.0.241)", "soundfile", "types-PyYAML", "types-requests", "types-simplejson", "types-toml", "types-tqdm", "types-urllib3", "urllib3 (<2.0)"]
+cli = ["InquirerPy (==0.3.4)"]
+dev = ["InquirerPy (==0.3.4)", "Jinja2", "Pillow", "aiohttp", "black (>=23.1,<24.0)", "gradio", "jedi", "mypy (==0.982)", "numpy", "pydantic", "pytest", "pytest-asyncio", "pytest-cov", "pytest-env", "pytest-vcr", "pytest-xdist", "ruff (>=0.0.241)", "soundfile", "types-PyYAML", "types-requests", "types-simplejson", "types-toml", "types-tqdm", "types-urllib3", "urllib3 (<2.0)"]
+fastai = ["fastai (>=2.4)", "fastcore (>=1.3.27)", "toml"]
+inference = ["aiohttp", "pydantic"]
+quality = ["black (>=23.1,<24.0)", "mypy (==0.982)", "ruff (>=0.0.241)"]
+tensorflow = ["graphviz", "pydot", "tensorflow"]
+testing = ["InquirerPy (==0.3.4)", "Jinja2", "Pillow", "aiohttp", "gradio", "jedi", "numpy", "pydantic", "pytest", "pytest-asyncio", "pytest-cov", "pytest-env", "pytest-vcr", "pytest-xdist", "soundfile", "urllib3 (<2.0)"]
+torch = ["torch"]
+typing = ["pydantic", "types-PyYAML", "types-requests", "types-simplejson", "types-toml", "types-tqdm", "types-urllib3"]
+
+[[package]]
+name = "idna"
+version = "3.4"
+description = "Internationalized Domain Names in Applications (IDNA)"
+optional = false
+python-versions = ">=3.5"
+files = [
+ {file = "idna-3.4-py3-none-any.whl", hash = "sha256:90b77e79eaa3eba6de819a0c442c0b4ceefc341a7a2ab77d7562bf49f425c5c2"},
+ {file = "idna-3.4.tar.gz", hash = "sha256:814f528e8dead7d329833b91c5faa87d60bf71824cd12a7530b5526063d02cb4"},
+]
+
+[[package]]
+name = "importlib-metadata"
+version = "6.7.0"
+description = "Read metadata from Python packages"
+optional = false
+python-versions = ">=3.7"
+files = [
+ {file = "importlib_metadata-6.7.0-py3-none-any.whl", hash = "sha256:cb52082e659e97afc5dac71e79de97d8681de3aa07ff18578330904a9d18e5b5"},
+ {file = "importlib_metadata-6.7.0.tar.gz", hash = "sha256:1aaf550d4f73e5d6783e7acb77aec43d49da8017410afae93822cc9cca98c4d4"},
+]
+
+[package.dependencies]
+typing-extensions = {version = ">=3.6.4", markers = "python_version < \"3.8\""}
+zipp = ">=0.5"
+
+[package.extras]
+docs = ["furo", "jaraco.packaging (>=9)", "jaraco.tidelift (>=1.4)", "rst.linker (>=1.9)", "sphinx (>=3.5)", "sphinx-lint"]
+perf = ["ipython"]
+testing = ["flufl.flake8", "importlib-resources (>=1.3)", "packaging", "pyfakefs", "pytest (>=6)", "pytest-black (>=0.3.7)", "pytest-checkdocs (>=2.4)", "pytest-cov", "pytest-enabler (>=1.3)", "pytest-mypy (>=0.9.1)", "pytest-perf (>=0.9.2)", "pytest-ruff"]
+
+[[package]]
+name = "iniconfig"
+version = "2.0.0"
+description = "brain-dead simple config-ini parsing"
+optional = false
+python-versions = ">=3.7"
+files = [
+ {file = "iniconfig-2.0.0-py3-none-any.whl", hash = "sha256:b6a85871a79d2e3b22d2d1b94ac2824226a63c6b741c88f7ae975f18b6778374"},
+ {file = "iniconfig-2.0.0.tar.gz", hash = "sha256:2d91e135bf72d31a410b17c16da610a82cb55f6b0477d1a902134b24a455b8b3"},
+]
+
+[[package]]
+name = "multidict"
+version = "6.0.4"
+description = "multidict implementation"
+optional = false
+python-versions = ">=3.7"
+files = [
+ {file = "multidict-6.0.4-cp310-cp310-macosx_10_9_universal2.whl", hash = "sha256:0b1a97283e0c85772d613878028fec909f003993e1007eafa715b24b377cb9b8"},
+ {file = "multidict-6.0.4-cp310-cp310-macosx_10_9_x86_64.whl", hash = "sha256:eeb6dcc05e911516ae3d1f207d4b0520d07f54484c49dfc294d6e7d63b734171"},
+ {file = "multidict-6.0.4-cp310-cp310-macosx_11_0_arm64.whl", hash = "sha256:d6d635d5209b82a3492508cf5b365f3446afb65ae7ebd755e70e18f287b0adf7"},
+ {file = "multidict-6.0.4-cp310-cp310-manylinux_2_17_aarch64.manylinux2014_aarch64.whl", hash = "sha256:c048099e4c9e9d615545e2001d3d8a4380bd403e1a0578734e0d31703d1b0c0b"},
+ {file = "multidict-6.0.4-cp310-cp310-manylinux_2_17_ppc64le.manylinux2014_ppc64le.whl", hash = "sha256:ea20853c6dbbb53ed34cb4d080382169b6f4554d394015f1bef35e881bf83547"},
+ {file = "multidict-6.0.4-cp310-cp310-manylinux_2_17_s390x.manylinux2014_s390x.whl", hash = "sha256:16d232d4e5396c2efbbf4f6d4df89bfa905eb0d4dc5b3549d872ab898451f569"},
+ {file = "multidict-6.0.4-cp310-cp310-manylinux_2_17_x86_64.manylinux2014_x86_64.whl", hash = "sha256:36c63aaa167f6c6b04ef2c85704e93af16c11d20de1d133e39de6a0e84582a93"},
+ {file = "multidict-6.0.4-cp310-cp310-manylinux_2_5_i686.manylinux1_i686.manylinux_2_17_i686.manylinux2014_i686.whl", hash = "sha256:64bdf1086b6043bf519869678f5f2757f473dee970d7abf6da91ec00acb9cb98"},
+ {file = "multidict-6.0.4-cp310-cp310-musllinux_1_1_aarch64.whl", hash = "sha256:43644e38f42e3af682690876cff722d301ac585c5b9e1eacc013b7a3f7b696a0"},
+ {file = "multidict-6.0.4-cp310-cp310-musllinux_1_1_i686.whl", hash = "sha256:7582a1d1030e15422262de9f58711774e02fa80df0d1578995c76214f6954988"},
+ {file = "multidict-6.0.4-cp310-cp310-musllinux_1_1_ppc64le.whl", hash = "sha256:ddff9c4e225a63a5afab9dd15590432c22e8057e1a9a13d28ed128ecf047bbdc"},
+ {file = "multidict-6.0.4-cp310-cp310-musllinux_1_1_s390x.whl", hash = "sha256:ee2a1ece51b9b9e7752e742cfb661d2a29e7bcdba2d27e66e28a99f1890e4fa0"},
+ {file = "multidict-6.0.4-cp310-cp310-musllinux_1_1_x86_64.whl", hash = "sha256:a2e4369eb3d47d2034032a26c7a80fcb21a2cb22e1173d761a162f11e562caa5"},
+ {file = "multidict-6.0.4-cp310-cp310-win32.whl", hash = "sha256:574b7eae1ab267e5f8285f0fe881f17efe4b98c39a40858247720935b893bba8"},
+ {file = "multidict-6.0.4-cp310-cp310-win_amd64.whl", hash = "sha256:4dcbb0906e38440fa3e325df2359ac6cb043df8e58c965bb45f4e406ecb162cc"},
+ {file = "multidict-6.0.4-cp311-cp311-macosx_10_9_universal2.whl", hash = "sha256:0dfad7a5a1e39c53ed00d2dd0c2e36aed4650936dc18fd9a1826a5ae1cad6f03"},
+ {file = "multidict-6.0.4-cp311-cp311-macosx_10_9_x86_64.whl", hash = "sha256:64da238a09d6039e3bd39bb3aee9c21a5e34f28bfa5aa22518581f910ff94af3"},
+ {file = "multidict-6.0.4-cp311-cp311-macosx_11_0_arm64.whl", hash = "sha256:ff959bee35038c4624250473988b24f846cbeb2c6639de3602c073f10410ceba"},
+ {file = "multidict-6.0.4-cp311-cp311-manylinux_2_17_aarch64.manylinux2014_aarch64.whl", hash = "sha256:01a3a55bd90018c9c080fbb0b9f4891db37d148a0a18722b42f94694f8b6d4c9"},
+ {file = "multidict-6.0.4-cp311-cp311-manylinux_2_17_ppc64le.manylinux2014_ppc64le.whl", hash = "sha256:c5cb09abb18c1ea940fb99360ea0396f34d46566f157122c92dfa069d3e0e982"},
+ {file = "multidict-6.0.4-cp311-cp311-manylinux_2_17_s390x.manylinux2014_s390x.whl", hash = "sha256:666daae833559deb2d609afa4490b85830ab0dfca811a98b70a205621a6109fe"},
+ {file = "multidict-6.0.4-cp311-cp311-manylinux_2_17_x86_64.manylinux2014_x86_64.whl", hash = "sha256:11bdf3f5e1518b24530b8241529d2050014c884cf18b6fc69c0c2b30ca248710"},
+ {file = "multidict-6.0.4-cp311-cp311-manylinux_2_5_i686.manylinux1_i686.manylinux_2_17_i686.manylinux2014_i686.whl", hash = "sha256:7d18748f2d30f94f498e852c67d61261c643b349b9d2a581131725595c45ec6c"},
+ {file = "multidict-6.0.4-cp311-cp311-musllinux_1_1_aarch64.whl", hash = "sha256:458f37be2d9e4c95e2d8866a851663cbc76e865b78395090786f6cd9b3bbf4f4"},
+ {file = "multidict-6.0.4-cp311-cp311-musllinux_1_1_i686.whl", hash = "sha256:b1a2eeedcead3a41694130495593a559a668f382eee0727352b9a41e1c45759a"},
+ {file = "multidict-6.0.4-cp311-cp311-musllinux_1_1_ppc64le.whl", hash = "sha256:7d6ae9d593ef8641544d6263c7fa6408cc90370c8cb2bbb65f8d43e5b0351d9c"},
+ {file = "multidict-6.0.4-cp311-cp311-musllinux_1_1_s390x.whl", hash = "sha256:5979b5632c3e3534e42ca6ff856bb24b2e3071b37861c2c727ce220d80eee9ed"},
+ {file = "multidict-6.0.4-cp311-cp311-musllinux_1_1_x86_64.whl", hash = "sha256:dcfe792765fab89c365123c81046ad4103fcabbc4f56d1c1997e6715e8015461"},
+ {file = "multidict-6.0.4-cp311-cp311-win32.whl", hash = "sha256:3601a3cece3819534b11d4efc1eb76047488fddd0c85a3948099d5da4d504636"},
+ {file = "multidict-6.0.4-cp311-cp311-win_amd64.whl", hash = "sha256:81a4f0b34bd92df3da93315c6a59034df95866014ac08535fc819f043bfd51f0"},
+ {file = "multidict-6.0.4-cp37-cp37m-macosx_10_9_x86_64.whl", hash = "sha256:67040058f37a2a51ed8ea8f6b0e6ee5bd78ca67f169ce6122f3e2ec80dfe9b78"},
+ {file = "multidict-6.0.4-cp37-cp37m-manylinux_2_17_aarch64.manylinux2014_aarch64.whl", hash = "sha256:853888594621e6604c978ce2a0444a1e6e70c8d253ab65ba11657659dcc9100f"},
+ {file = "multidict-6.0.4-cp37-cp37m-manylinux_2_17_ppc64le.manylinux2014_ppc64le.whl", hash = "sha256:39ff62e7d0f26c248b15e364517a72932a611a9b75f35b45be078d81bdb86603"},
+ {file = "multidict-6.0.4-cp37-cp37m-manylinux_2_17_s390x.manylinux2014_s390x.whl", hash = "sha256:af048912e045a2dc732847d33821a9d84ba553f5c5f028adbd364dd4765092ac"},
+ {file = "multidict-6.0.4-cp37-cp37m-manylinux_2_17_x86_64.manylinux2014_x86_64.whl", hash = "sha256:b1e8b901e607795ec06c9e42530788c45ac21ef3aaa11dbd0c69de543bfb79a9"},
+ {file = "multidict-6.0.4-cp37-cp37m-manylinux_2_5_i686.manylinux1_i686.manylinux_2_17_i686.manylinux2014_i686.whl", hash = "sha256:62501642008a8b9871ddfccbf83e4222cf8ac0d5aeedf73da36153ef2ec222d2"},
+ {file = "multidict-6.0.4-cp37-cp37m-musllinux_1_1_aarch64.whl", hash = "sha256:99b76c052e9f1bc0721f7541e5e8c05db3941eb9ebe7b8553c625ef88d6eefde"},
+ {file = "multidict-6.0.4-cp37-cp37m-musllinux_1_1_i686.whl", hash = "sha256:509eac6cf09c794aa27bcacfd4d62c885cce62bef7b2c3e8b2e49d365b5003fe"},
+ {file = "multidict-6.0.4-cp37-cp37m-musllinux_1_1_ppc64le.whl", hash = "sha256:21a12c4eb6ddc9952c415f24eef97e3e55ba3af61f67c7bc388dcdec1404a067"},
+ {file = "multidict-6.0.4-cp37-cp37m-musllinux_1_1_s390x.whl", hash = "sha256:5cad9430ab3e2e4fa4a2ef4450f548768400a2ac635841bc2a56a2052cdbeb87"},
+ {file = "multidict-6.0.4-cp37-cp37m-musllinux_1_1_x86_64.whl", hash = "sha256:ab55edc2e84460694295f401215f4a58597f8f7c9466faec545093045476327d"},
+ {file = "multidict-6.0.4-cp37-cp37m-win32.whl", hash = "sha256:5a4dcf02b908c3b8b17a45fb0f15b695bf117a67b76b7ad18b73cf8e92608775"},
+ {file = "multidict-6.0.4-cp37-cp37m-win_amd64.whl", hash = "sha256:6ed5f161328b7df384d71b07317f4d8656434e34591f20552c7bcef27b0ab88e"},
+ {file = "multidict-6.0.4-cp38-cp38-macosx_10_9_universal2.whl", hash = "sha256:5fc1b16f586f049820c5c5b17bb4ee7583092fa0d1c4e28b5239181ff9532e0c"},
+ {file = "multidict-6.0.4-cp38-cp38-macosx_10_9_x86_64.whl", hash = "sha256:1502e24330eb681bdaa3eb70d6358e818e8e8f908a22a1851dfd4e15bc2f8161"},
+ {file = "multidict-6.0.4-cp38-cp38-macosx_11_0_arm64.whl", hash = "sha256:b692f419760c0e65d060959df05f2a531945af31fda0c8a3b3195d4efd06de11"},
+ {file = "multidict-6.0.4-cp38-cp38-manylinux_2_17_aarch64.manylinux2014_aarch64.whl", hash = "sha256:45e1ecb0379bfaab5eef059f50115b54571acfbe422a14f668fc8c27ba410e7e"},
+ {file = "multidict-6.0.4-cp38-cp38-manylinux_2_17_ppc64le.manylinux2014_ppc64le.whl", hash = "sha256:ddd3915998d93fbcd2566ddf9cf62cdb35c9e093075f862935573d265cf8f65d"},
+ {file = "multidict-6.0.4-cp38-cp38-manylinux_2_17_s390x.manylinux2014_s390x.whl", hash = "sha256:59d43b61c59d82f2effb39a93c48b845efe23a3852d201ed2d24ba830d0b4cf2"},
+ {file = "multidict-6.0.4-cp38-cp38-manylinux_2_17_x86_64.manylinux2014_x86_64.whl", hash = "sha256:cc8e1d0c705233c5dd0c5e6460fbad7827d5d36f310a0fadfd45cc3029762258"},
+ {file = "multidict-6.0.4-cp38-cp38-manylinux_2_5_i686.manylinux1_i686.manylinux_2_17_i686.manylinux2014_i686.whl", hash = "sha256:d6aa0418fcc838522256761b3415822626f866758ee0bc6632c9486b179d0b52"},
+ {file = "multidict-6.0.4-cp38-cp38-musllinux_1_1_aarch64.whl", hash = "sha256:6748717bb10339c4760c1e63da040f5f29f5ed6e59d76daee30305894069a660"},
+ {file = "multidict-6.0.4-cp38-cp38-musllinux_1_1_i686.whl", hash = "sha256:4d1a3d7ef5e96b1c9e92f973e43aa5e5b96c659c9bc3124acbbd81b0b9c8a951"},
+ {file = "multidict-6.0.4-cp38-cp38-musllinux_1_1_ppc64le.whl", hash = "sha256:4372381634485bec7e46718edc71528024fcdc6f835baefe517b34a33c731d60"},
+ {file = "multidict-6.0.4-cp38-cp38-musllinux_1_1_s390x.whl", hash = "sha256:fc35cb4676846ef752816d5be2193a1e8367b4c1397b74a565a9d0389c433a1d"},
+ {file = "multidict-6.0.4-cp38-cp38-musllinux_1_1_x86_64.whl", hash = "sha256:4b9d9e4e2b37daddb5c23ea33a3417901fa7c7b3dee2d855f63ee67a0b21e5b1"},
+ {file = "multidict-6.0.4-cp38-cp38-win32.whl", hash = "sha256:e41b7e2b59679edfa309e8db64fdf22399eec4b0b24694e1b2104fb789207779"},
+ {file = "multidict-6.0.4-cp38-cp38-win_amd64.whl", hash = "sha256:d6c254ba6e45d8e72739281ebc46ea5eb5f101234f3ce171f0e9f5cc86991480"},
+ {file = "multidict-6.0.4-cp39-cp39-macosx_10_9_universal2.whl", hash = "sha256:16ab77bbeb596e14212e7bab8429f24c1579234a3a462105cda4a66904998664"},
+ {file = "multidict-6.0.4-cp39-cp39-macosx_10_9_x86_64.whl", hash = "sha256:bc779e9e6f7fda81b3f9aa58e3a6091d49ad528b11ed19f6621408806204ad35"},
+ {file = "multidict-6.0.4-cp39-cp39-macosx_11_0_arm64.whl", hash = "sha256:4ceef517eca3e03c1cceb22030a3e39cb399ac86bff4e426d4fc6ae49052cc60"},
+ {file = "multidict-6.0.4-cp39-cp39-manylinux_2_17_aarch64.manylinux2014_aarch64.whl", hash = "sha256:281af09f488903fde97923c7744bb001a9b23b039a909460d0f14edc7bf59706"},
+ {file = "multidict-6.0.4-cp39-cp39-manylinux_2_17_ppc64le.manylinux2014_ppc64le.whl", hash = "sha256:52f2dffc8acaba9a2f27174c41c9e57f60b907bb9f096b36b1a1f3be71c6284d"},
+ {file = "multidict-6.0.4-cp39-cp39-manylinux_2_17_s390x.manylinux2014_s390x.whl", hash = "sha256:b41156839806aecb3641f3208c0dafd3ac7775b9c4c422d82ee2a45c34ba81ca"},
+ {file = "multidict-6.0.4-cp39-cp39-manylinux_2_17_x86_64.manylinux2014_x86_64.whl", hash = "sha256:d5e3fc56f88cc98ef8139255cf8cd63eb2c586531e43310ff859d6bb3a6b51f1"},
+ {file = "multidict-6.0.4-cp39-cp39-manylinux_2_5_i686.manylinux1_i686.manylinux_2_17_i686.manylinux2014_i686.whl", hash = "sha256:8316a77808c501004802f9beebde51c9f857054a0c871bd6da8280e718444449"},
+ {file = "multidict-6.0.4-cp39-cp39-musllinux_1_1_aarch64.whl", hash = "sha256:f70b98cd94886b49d91170ef23ec5c0e8ebb6f242d734ed7ed677b24d50c82cf"},
+ {file = "multidict-6.0.4-cp39-cp39-musllinux_1_1_i686.whl", hash = "sha256:bf6774e60d67a9efe02b3616fee22441d86fab4c6d335f9d2051d19d90a40063"},
+ {file = "multidict-6.0.4-cp39-cp39-musllinux_1_1_ppc64le.whl", hash = "sha256:e69924bfcdda39b722ef4d9aa762b2dd38e4632b3641b1d9a57ca9cd18f2f83a"},
+ {file = "multidict-6.0.4-cp39-cp39-musllinux_1_1_s390x.whl", hash = "sha256:6b181d8c23da913d4ff585afd1155a0e1194c0b50c54fcfe286f70cdaf2b7176"},
+ {file = "multidict-6.0.4-cp39-cp39-musllinux_1_1_x86_64.whl", hash = "sha256:52509b5be062d9eafc8170e53026fbc54cf3b32759a23d07fd935fb04fc22d95"},
+ {file = "multidict-6.0.4-cp39-cp39-win32.whl", hash = "sha256:27c523fbfbdfd19c6867af7346332b62b586eed663887392cff78d614f9ec313"},
+ {file = "multidict-6.0.4-cp39-cp39-win_amd64.whl", hash = "sha256:33029f5734336aa0d4c0384525da0387ef89148dc7191aae00ca5fb23d7aafc2"},
+ {file = "multidict-6.0.4.tar.gz", hash = "sha256:3666906492efb76453c0e7b97f2cf459b0682e7402c0489a95484965dbc1da49"},
+]
+
+[[package]]
+name = "packaging"
+version = "23.1"
+description = "Core utilities for Python packages"
+optional = false
+python-versions = ">=3.7"
+files = [
+ {file = "packaging-23.1-py3-none-any.whl", hash = "sha256:994793af429502c4ea2ebf6bf664629d07c1a9fe974af92966e4b8d2df7edc61"},
+ {file = "packaging-23.1.tar.gz", hash = "sha256:a392980d2b6cffa644431898be54b0045151319d1e7ec34f0cfed48767dd334f"},
+]
+
+[[package]]
+name = "pluggy"
+version = "1.2.0"
+description = "plugin and hook calling mechanisms for python"
+optional = false
+python-versions = ">=3.7"
+files = [
+ {file = "pluggy-1.2.0-py3-none-any.whl", hash = "sha256:c2fd55a7d7a3863cba1a013e4e2414658b1d07b6bc57b3919e0c63c9abb99849"},
+ {file = "pluggy-1.2.0.tar.gz", hash = "sha256:d12f0c4b579b15f5e054301bb226ee85eeeba08ffec228092f8defbaa3a4c4b3"},
+]
+
+[package.dependencies]
+importlib-metadata = {version = ">=0.12", markers = "python_version < \"3.8\""}
+
+[package.extras]
+dev = ["pre-commit", "tox"]
+testing = ["pytest", "pytest-benchmark"]
+
+[[package]]
+name = "py"
+version = "1.11.0"
+description = "library with cross-python path, ini-parsing, io, code, log facilities"
+optional = false
+python-versions = ">=2.7, !=3.0.*, !=3.1.*, !=3.2.*, !=3.3.*, !=3.4.*"
+files = [
+ {file = "py-1.11.0-py2.py3-none-any.whl", hash = "sha256:607c53218732647dff4acdfcd50cb62615cedf612e72d1724fb1a0cc6405b378"},
+ {file = "py-1.11.0.tar.gz", hash = "sha256:51c75c4126074b472f746a24399ad32f6053d1b34b68d2fa41e558e6f4a98719"},
+]
+
+[[package]]
+name = "pydantic"
+version = "2.5.3"
+description = "Data validation using Python type hints"
+optional = false
+python-versions = ">=3.7"
+files = [
+ {file = "pydantic-2.5.3-py3-none-any.whl", hash = "sha256:d0caf5954bee831b6bfe7e338c32b9e30c85dfe080c843680783ac2b631673b4"},
+ {file = "pydantic-2.5.3.tar.gz", hash = "sha256:b3ef57c62535b0941697cce638c08900d87fcb67e29cfa99e8a68f747f393f7a"},
+]
+
+[package.dependencies]
+annotated-types = ">=0.4.0"
+importlib-metadata = {version = "*", markers = "python_version == \"3.7\""}
+pydantic-core = "2.14.6"
+typing-extensions = ">=4.6.1"
+
+[package.extras]
+email = ["email-validator (>=2.0.0)"]
+
+[[package]]
+name = "pydantic-core"
+version = "2.14.6"
+description = ""
+optional = false
+python-versions = ">=3.7"
+files = [
+ {file = "pydantic_core-2.14.6-cp310-cp310-macosx_10_7_x86_64.whl", hash = "sha256:72f9a942d739f09cd42fffe5dc759928217649f070056f03c70df14f5770acf9"},
+ {file = "pydantic_core-2.14.6-cp310-cp310-macosx_11_0_arm64.whl", hash = "sha256:6a31d98c0d69776c2576dda4b77b8e0c69ad08e8b539c25c7d0ca0dc19a50d6c"},
+ {file = "pydantic_core-2.14.6-cp310-cp310-manylinux_2_17_aarch64.manylinux2014_aarch64.whl", hash = "sha256:5aa90562bc079c6c290f0512b21768967f9968e4cfea84ea4ff5af5d917016e4"},
+ {file = "pydantic_core-2.14.6-cp310-cp310-manylinux_2_17_armv7l.manylinux2014_armv7l.whl", hash = "sha256:370ffecb5316ed23b667d99ce4debe53ea664b99cc37bfa2af47bc769056d534"},
+ {file = "pydantic_core-2.14.6-cp310-cp310-manylinux_2_17_ppc64le.manylinux2014_ppc64le.whl", hash = "sha256:f85f3843bdb1fe80e8c206fe6eed7a1caeae897e496542cee499c374a85c6e08"},
+ {file = "pydantic_core-2.14.6-cp310-cp310-manylinux_2_17_s390x.manylinux2014_s390x.whl", hash = "sha256:9862bf828112e19685b76ca499b379338fd4c5c269d897e218b2ae8fcb80139d"},
+ {file = "pydantic_core-2.14.6-cp310-cp310-manylinux_2_17_x86_64.manylinux2014_x86_64.whl", hash = "sha256:036137b5ad0cb0004c75b579445a1efccd072387a36c7f217bb8efd1afbe5245"},
+ {file = "pydantic_core-2.14.6-cp310-cp310-manylinux_2_5_i686.manylinux1_i686.whl", hash = "sha256:92879bce89f91f4b2416eba4429c7b5ca22c45ef4a499c39f0c5c69257522c7c"},
+ {file = "pydantic_core-2.14.6-cp310-cp310-musllinux_1_1_aarch64.whl", hash = "sha256:0c08de15d50fa190d577e8591f0329a643eeaed696d7771760295998aca6bc66"},
+ {file = "pydantic_core-2.14.6-cp310-cp310-musllinux_1_1_x86_64.whl", hash = "sha256:36099c69f6b14fc2c49d7996cbf4f87ec4f0e66d1c74aa05228583225a07b590"},
+ {file = "pydantic_core-2.14.6-cp310-none-win32.whl", hash = "sha256:7be719e4d2ae6c314f72844ba9d69e38dff342bc360379f7c8537c48e23034b7"},
+ {file = "pydantic_core-2.14.6-cp310-none-win_amd64.whl", hash = "sha256:36fa402dcdc8ea7f1b0ddcf0df4254cc6b2e08f8cd80e7010d4c4ae6e86b2a87"},
+ {file = "pydantic_core-2.14.6-cp311-cp311-macosx_10_7_x86_64.whl", hash = "sha256:dea7fcd62915fb150cdc373212141a30037e11b761fbced340e9db3379b892d4"},
+ {file = "pydantic_core-2.14.6-cp311-cp311-macosx_11_0_arm64.whl", hash = "sha256:ffff855100bc066ff2cd3aa4a60bc9534661816b110f0243e59503ec2df38421"},
+ {file = "pydantic_core-2.14.6-cp311-cp311-manylinux_2_17_aarch64.manylinux2014_aarch64.whl", hash = "sha256:1b027c86c66b8627eb90e57aee1f526df77dc6d8b354ec498be9a757d513b92b"},
+ {file = "pydantic_core-2.14.6-cp311-cp311-manylinux_2_17_armv7l.manylinux2014_armv7l.whl", hash = "sha256:00b1087dabcee0b0ffd104f9f53d7d3eaddfaa314cdd6726143af6bc713aa27e"},
+ {file = "pydantic_core-2.14.6-cp311-cp311-manylinux_2_17_ppc64le.manylinux2014_ppc64le.whl", hash = "sha256:75ec284328b60a4e91010c1acade0c30584f28a1f345bc8f72fe8b9e46ec6a96"},
+ {file = "pydantic_core-2.14.6-cp311-cp311-manylinux_2_17_s390x.manylinux2014_s390x.whl", hash = "sha256:7e1f4744eea1501404b20b0ac059ff7e3f96a97d3e3f48ce27a139e053bb370b"},
+ {file = "pydantic_core-2.14.6-cp311-cp311-manylinux_2_17_x86_64.manylinux2014_x86_64.whl", hash = "sha256:b2602177668f89b38b9f84b7b3435d0a72511ddef45dc14446811759b82235a1"},
+ {file = "pydantic_core-2.14.6-cp311-cp311-manylinux_2_5_i686.manylinux1_i686.whl", hash = "sha256:6c8edaea3089bf908dd27da8f5d9e395c5b4dc092dbcce9b65e7156099b4b937"},
+ {file = "pydantic_core-2.14.6-cp311-cp311-musllinux_1_1_aarch64.whl", hash = "sha256:478e9e7b360dfec451daafe286998d4a1eeaecf6d69c427b834ae771cad4b622"},
+ {file = "pydantic_core-2.14.6-cp311-cp311-musllinux_1_1_x86_64.whl", hash = "sha256:b6ca36c12a5120bad343eef193cc0122928c5c7466121da7c20f41160ba00ba2"},
+ {file = "pydantic_core-2.14.6-cp311-none-win32.whl", hash = "sha256:2b8719037e570639e6b665a4050add43134d80b687288ba3ade18b22bbb29dd2"},
+ {file = "pydantic_core-2.14.6-cp311-none-win_amd64.whl", hash = "sha256:78ee52ecc088c61cce32b2d30a826f929e1708f7b9247dc3b921aec367dc1b23"},
+ {file = "pydantic_core-2.14.6-cp311-none-win_arm64.whl", hash = "sha256:a19b794f8fe6569472ff77602437ec4430f9b2b9ec7a1105cfd2232f9ba355e6"},
+ {file = "pydantic_core-2.14.6-cp312-cp312-macosx_10_7_x86_64.whl", hash = "sha256:667aa2eac9cd0700af1ddb38b7b1ef246d8cf94c85637cbb03d7757ca4c3fdec"},
+ {file = "pydantic_core-2.14.6-cp312-cp312-macosx_11_0_arm64.whl", hash = "sha256:cdee837710ef6b56ebd20245b83799fce40b265b3b406e51e8ccc5b85b9099b7"},
+ {file = "pydantic_core-2.14.6-cp312-cp312-manylinux_2_17_aarch64.manylinux2014_aarch64.whl", hash = "sha256:2c5bcf3414367e29f83fd66f7de64509a8fd2368b1edf4351e862910727d3e51"},
+ {file = "pydantic_core-2.14.6-cp312-cp312-manylinux_2_17_armv7l.manylinux2014_armv7l.whl", hash = "sha256:26a92ae76f75d1915806b77cf459811e772d8f71fd1e4339c99750f0e7f6324f"},
+ {file = "pydantic_core-2.14.6-cp312-cp312-manylinux_2_17_ppc64le.manylinux2014_ppc64le.whl", hash = "sha256:a983cca5ed1dd9a35e9e42ebf9f278d344603bfcb174ff99a5815f953925140a"},
+ {file = "pydantic_core-2.14.6-cp312-cp312-manylinux_2_17_s390x.manylinux2014_s390x.whl", hash = "sha256:cb92f9061657287eded380d7dc455bbf115430b3aa4741bdc662d02977e7d0af"},
+ {file = "pydantic_core-2.14.6-cp312-cp312-manylinux_2_17_x86_64.manylinux2014_x86_64.whl", hash = "sha256:e4ace1e220b078c8e48e82c081e35002038657e4b37d403ce940fa679e57113b"},
+ {file = "pydantic_core-2.14.6-cp312-cp312-manylinux_2_5_i686.manylinux1_i686.whl", hash = "sha256:ef633add81832f4b56d3b4c9408b43d530dfca29e68fb1b797dcb861a2c734cd"},
+ {file = "pydantic_core-2.14.6-cp312-cp312-musllinux_1_1_aarch64.whl", hash = "sha256:7e90d6cc4aad2cc1f5e16ed56e46cebf4877c62403a311af20459c15da76fd91"},
+ {file = "pydantic_core-2.14.6-cp312-cp312-musllinux_1_1_x86_64.whl", hash = "sha256:e8a5ac97ea521d7bde7621d86c30e86b798cdecd985723c4ed737a2aa9e77d0c"},
+ {file = "pydantic_core-2.14.6-cp312-none-win32.whl", hash = "sha256:f27207e8ca3e5e021e2402ba942e5b4c629718e665c81b8b306f3c8b1ddbb786"},
+ {file = "pydantic_core-2.14.6-cp312-none-win_amd64.whl", hash = "sha256:b3e5fe4538001bb82e2295b8d2a39356a84694c97cb73a566dc36328b9f83b40"},
+ {file = "pydantic_core-2.14.6-cp312-none-win_arm64.whl", hash = "sha256:64634ccf9d671c6be242a664a33c4acf12882670b09b3f163cd00a24cffbd74e"},
+ {file = "pydantic_core-2.14.6-cp37-cp37m-macosx_10_7_x86_64.whl", hash = "sha256:24368e31be2c88bd69340fbfe741b405302993242ccb476c5c3ff48aeee1afe0"},
+ {file = "pydantic_core-2.14.6-cp37-cp37m-macosx_11_0_arm64.whl", hash = "sha256:e33b0834f1cf779aa839975f9d8755a7c2420510c0fa1e9fa0497de77cd35d2c"},
+ {file = "pydantic_core-2.14.6-cp37-cp37m-manylinux_2_17_aarch64.manylinux2014_aarch64.whl", hash = "sha256:6af4b3f52cc65f8a0bc8b1cd9676f8c21ef3e9132f21fed250f6958bd7223bed"},
+ {file = "pydantic_core-2.14.6-cp37-cp37m-manylinux_2_17_armv7l.manylinux2014_armv7l.whl", hash = "sha256:d15687d7d7f40333bd8266f3814c591c2e2cd263fa2116e314f60d82086e353a"},
+ {file = "pydantic_core-2.14.6-cp37-cp37m-manylinux_2_17_ppc64le.manylinux2014_ppc64le.whl", hash = "sha256:095b707bb287bfd534044166ab767bec70a9bba3175dcdc3371782175c14e43c"},
+ {file = "pydantic_core-2.14.6-cp37-cp37m-manylinux_2_17_s390x.manylinux2014_s390x.whl", hash = "sha256:94fc0e6621e07d1e91c44e016cc0b189b48db053061cc22d6298a611de8071bb"},
+ {file = "pydantic_core-2.14.6-cp37-cp37m-manylinux_2_17_x86_64.manylinux2014_x86_64.whl", hash = "sha256:1ce830e480f6774608dedfd4a90c42aac4a7af0a711f1b52f807130c2e434c06"},
+ {file = "pydantic_core-2.14.6-cp37-cp37m-manylinux_2_5_i686.manylinux1_i686.whl", hash = "sha256:a306cdd2ad3a7d795d8e617a58c3a2ed0f76c8496fb7621b6cd514eb1532cae8"},
+ {file = "pydantic_core-2.14.6-cp37-cp37m-musllinux_1_1_aarch64.whl", hash = "sha256:2f5fa187bde8524b1e37ba894db13aadd64faa884657473b03a019f625cee9a8"},
+ {file = "pydantic_core-2.14.6-cp37-cp37m-musllinux_1_1_x86_64.whl", hash = "sha256:438027a975cc213a47c5d70672e0d29776082155cfae540c4e225716586be75e"},
+ {file = "pydantic_core-2.14.6-cp37-none-win32.whl", hash = "sha256:f96ae96a060a8072ceff4cfde89d261837b4294a4f28b84a28765470d502ccc6"},
+ {file = "pydantic_core-2.14.6-cp37-none-win_amd64.whl", hash = "sha256:e646c0e282e960345314f42f2cea5e0b5f56938c093541ea6dbf11aec2862391"},
+ {file = "pydantic_core-2.14.6-cp38-cp38-macosx_10_7_x86_64.whl", hash = "sha256:db453f2da3f59a348f514cfbfeb042393b68720787bbef2b4c6068ea362c8149"},
+ {file = "pydantic_core-2.14.6-cp38-cp38-macosx_11_0_arm64.whl", hash = "sha256:3860c62057acd95cc84044e758e47b18dcd8871a328ebc8ccdefd18b0d26a21b"},
+ {file = "pydantic_core-2.14.6-cp38-cp38-manylinux_2_17_aarch64.manylinux2014_aarch64.whl", hash = "sha256:36026d8f99c58d7044413e1b819a67ca0e0b8ebe0f25e775e6c3d1fabb3c38fb"},
+ {file = "pydantic_core-2.14.6-cp38-cp38-manylinux_2_17_armv7l.manylinux2014_armv7l.whl", hash = "sha256:8ed1af8692bd8d2a29d702f1a2e6065416d76897d726e45a1775b1444f5928a7"},
+ {file = "pydantic_core-2.14.6-cp38-cp38-manylinux_2_17_ppc64le.manylinux2014_ppc64le.whl", hash = "sha256:314ccc4264ce7d854941231cf71b592e30d8d368a71e50197c905874feacc8a8"},
+ {file = "pydantic_core-2.14.6-cp38-cp38-manylinux_2_17_s390x.manylinux2014_s390x.whl", hash = "sha256:982487f8931067a32e72d40ab6b47b1628a9c5d344be7f1a4e668fb462d2da42"},
+ {file = "pydantic_core-2.14.6-cp38-cp38-manylinux_2_17_x86_64.manylinux2014_x86_64.whl", hash = "sha256:2dbe357bc4ddda078f79d2a36fc1dd0494a7f2fad83a0a684465b6f24b46fe80"},
+ {file = "pydantic_core-2.14.6-cp38-cp38-manylinux_2_5_i686.manylinux1_i686.whl", hash = "sha256:2f6ffc6701a0eb28648c845f4945a194dc7ab3c651f535b81793251e1185ac3d"},
+ {file = "pydantic_core-2.14.6-cp38-cp38-musllinux_1_1_aarch64.whl", hash = "sha256:7f5025db12fc6de7bc1104d826d5aee1d172f9ba6ca936bf6474c2148ac336c1"},
+ {file = "pydantic_core-2.14.6-cp38-cp38-musllinux_1_1_x86_64.whl", hash = "sha256:dab03ed811ed1c71d700ed08bde8431cf429bbe59e423394f0f4055f1ca0ea60"},
+ {file = "pydantic_core-2.14.6-cp38-none-win32.whl", hash = "sha256:dfcbebdb3c4b6f739a91769aea5ed615023f3c88cb70df812849aef634c25fbe"},
+ {file = "pydantic_core-2.14.6-cp38-none-win_amd64.whl", hash = "sha256:99b14dbea2fdb563d8b5a57c9badfcd72083f6006caf8e126b491519c7d64ca8"},
+ {file = "pydantic_core-2.14.6-cp39-cp39-macosx_10_7_x86_64.whl", hash = "sha256:4ce8299b481bcb68e5c82002b96e411796b844d72b3e92a3fbedfe8e19813eab"},
+ {file = "pydantic_core-2.14.6-cp39-cp39-macosx_11_0_arm64.whl", hash = "sha256:b9a9d92f10772d2a181b5ca339dee066ab7d1c9a34ae2421b2a52556e719756f"},
+ {file = "pydantic_core-2.14.6-cp39-cp39-manylinux_2_17_aarch64.manylinux2014_aarch64.whl", hash = "sha256:fd9e98b408384989ea4ab60206b8e100d8687da18b5c813c11e92fd8212a98e0"},
+ {file = "pydantic_core-2.14.6-cp39-cp39-manylinux_2_17_armv7l.manylinux2014_armv7l.whl", hash = "sha256:4f86f1f318e56f5cbb282fe61eb84767aee743ebe32c7c0834690ebea50c0a6b"},
+ {file = "pydantic_core-2.14.6-cp39-cp39-manylinux_2_17_ppc64le.manylinux2014_ppc64le.whl", hash = "sha256:86ce5fcfc3accf3a07a729779d0b86c5d0309a4764c897d86c11089be61da160"},
+ {file = "pydantic_core-2.14.6-cp39-cp39-manylinux_2_17_s390x.manylinux2014_s390x.whl", hash = "sha256:3dcf1978be02153c6a31692d4fbcc2a3f1db9da36039ead23173bc256ee3b91b"},
+ {file = "pydantic_core-2.14.6-cp39-cp39-manylinux_2_17_x86_64.manylinux2014_x86_64.whl", hash = "sha256:eedf97be7bc3dbc8addcef4142f4b4164066df0c6f36397ae4aaed3eb187d8ab"},
+ {file = "pydantic_core-2.14.6-cp39-cp39-manylinux_2_5_i686.manylinux1_i686.whl", hash = "sha256:d5f916acf8afbcab6bacbb376ba7dc61f845367901ecd5e328fc4d4aef2fcab0"},
+ {file = "pydantic_core-2.14.6-cp39-cp39-musllinux_1_1_aarch64.whl", hash = "sha256:8a14c192c1d724c3acbfb3f10a958c55a2638391319ce8078cb36c02283959b9"},
+ {file = "pydantic_core-2.14.6-cp39-cp39-musllinux_1_1_x86_64.whl", hash = "sha256:0348b1dc6b76041516e8a854ff95b21c55f5a411c3297d2ca52f5528e49d8411"},
+ {file = "pydantic_core-2.14.6-cp39-none-win32.whl", hash = "sha256:de2a0645a923ba57c5527497daf8ec5df69c6eadf869e9cd46e86349146e5975"},
+ {file = "pydantic_core-2.14.6-cp39-none-win_amd64.whl", hash = "sha256:aca48506a9c20f68ee61c87f2008f81f8ee99f8d7f0104bff3c47e2d148f89d9"},
+ {file = "pydantic_core-2.14.6-pp310-pypy310_pp73-macosx_10_7_x86_64.whl", hash = "sha256:d5c28525c19f5bb1e09511669bb57353d22b94cf8b65f3a8d141c389a55dec95"},
+ {file = "pydantic_core-2.14.6-pp310-pypy310_pp73-macosx_11_0_arm64.whl", hash = "sha256:78d0768ee59baa3de0f4adac9e3748b4b1fffc52143caebddfd5ea2961595277"},
+ {file = "pydantic_core-2.14.6-pp310-pypy310_pp73-manylinux_2_17_aarch64.manylinux2014_aarch64.whl", hash = "sha256:8b93785eadaef932e4fe9c6e12ba67beb1b3f1e5495631419c784ab87e975670"},
+ {file = "pydantic_core-2.14.6-pp310-pypy310_pp73-manylinux_2_17_x86_64.manylinux2014_x86_64.whl", hash = "sha256:a874f21f87c485310944b2b2734cd6d318765bcbb7515eead33af9641816506e"},
+ {file = "pydantic_core-2.14.6-pp310-pypy310_pp73-manylinux_2_5_i686.manylinux1_i686.whl", hash = "sha256:b89f4477d915ea43b4ceea6756f63f0288941b6443a2b28c69004fe07fde0d0d"},
+ {file = "pydantic_core-2.14.6-pp310-pypy310_pp73-musllinux_1_1_aarch64.whl", hash = "sha256:172de779e2a153d36ee690dbc49c6db568d7b33b18dc56b69a7514aecbcf380d"},
+ {file = "pydantic_core-2.14.6-pp310-pypy310_pp73-musllinux_1_1_x86_64.whl", hash = "sha256:dfcebb950aa7e667ec226a442722134539e77c575f6cfaa423f24371bb8d2e94"},
+ {file = "pydantic_core-2.14.6-pp310-pypy310_pp73-win_amd64.whl", hash = "sha256:55a23dcd98c858c0db44fc5c04fc7ed81c4b4d33c653a7c45ddaebf6563a2f66"},
+ {file = "pydantic_core-2.14.6-pp37-pypy37_pp73-macosx_10_7_x86_64.whl", hash = "sha256:4241204e4b36ab5ae466ecec5c4c16527a054c69f99bba20f6f75232a6a534e2"},
+ {file = "pydantic_core-2.14.6-pp37-pypy37_pp73-manylinux_2_17_aarch64.manylinux2014_aarch64.whl", hash = "sha256:e574de99d735b3fc8364cba9912c2bec2da78775eba95cbb225ef7dda6acea24"},
+ {file = "pydantic_core-2.14.6-pp37-pypy37_pp73-manylinux_2_17_x86_64.manylinux2014_x86_64.whl", hash = "sha256:1302a54f87b5cd8528e4d6d1bf2133b6aa7c6122ff8e9dc5220fbc1e07bffebd"},
+ {file = "pydantic_core-2.14.6-pp37-pypy37_pp73-manylinux_2_5_i686.manylinux1_i686.whl", hash = "sha256:f8e81e4b55930e5ffab4a68db1af431629cf2e4066dbdbfef65348b8ab804ea8"},
+ {file = "pydantic_core-2.14.6-pp37-pypy37_pp73-musllinux_1_1_aarch64.whl", hash = "sha256:c99462ffc538717b3e60151dfaf91125f637e801f5ab008f81c402f1dff0cd0f"},
+ {file = "pydantic_core-2.14.6-pp37-pypy37_pp73-musllinux_1_1_x86_64.whl", hash = "sha256:e4cf2d5829f6963a5483ec01578ee76d329eb5caf330ecd05b3edd697e7d768a"},
+ {file = "pydantic_core-2.14.6-pp38-pypy38_pp73-macosx_10_7_x86_64.whl", hash = "sha256:cf10b7d58ae4a1f07fccbf4a0a956d705356fea05fb4c70608bb6fa81d103cda"},
+ {file = "pydantic_core-2.14.6-pp38-pypy38_pp73-macosx_11_0_arm64.whl", hash = "sha256:399ac0891c284fa8eb998bcfa323f2234858f5d2efca3950ae58c8f88830f145"},
+ {file = "pydantic_core-2.14.6-pp38-pypy38_pp73-manylinux_2_17_aarch64.manylinux2014_aarch64.whl", hash = "sha256:9c6a5c79b28003543db3ba67d1df336f253a87d3112dac3a51b94f7d48e4c0e1"},
+ {file = "pydantic_core-2.14.6-pp38-pypy38_pp73-manylinux_2_17_x86_64.manylinux2014_x86_64.whl", hash = "sha256:599c87d79cab2a6a2a9df4aefe0455e61e7d2aeede2f8577c1b7c0aec643ee8e"},
+ {file = "pydantic_core-2.14.6-pp38-pypy38_pp73-manylinux_2_5_i686.manylinux1_i686.whl", hash = "sha256:43e166ad47ba900f2542a80d83f9fc65fe99eb63ceec4debec160ae729824052"},
+ {file = "pydantic_core-2.14.6-pp38-pypy38_pp73-musllinux_1_1_aarch64.whl", hash = "sha256:3a0b5db001b98e1c649dd55afa928e75aa4087e587b9524a4992316fa23c9fba"},
+ {file = "pydantic_core-2.14.6-pp38-pypy38_pp73-musllinux_1_1_x86_64.whl", hash = "sha256:747265448cb57a9f37572a488a57d873fd96bf51e5bb7edb52cfb37124516da4"},
+ {file = "pydantic_core-2.14.6-pp38-pypy38_pp73-win_amd64.whl", hash = "sha256:7ebe3416785f65c28f4f9441e916bfc8a54179c8dea73c23023f7086fa601c5d"},
+ {file = "pydantic_core-2.14.6-pp39-pypy39_pp73-macosx_10_7_x86_64.whl", hash = "sha256:86c963186ca5e50d5c8287b1d1c9d3f8f024cbe343d048c5bd282aec2d8641f2"},
+ {file = "pydantic_core-2.14.6-pp39-pypy39_pp73-macosx_11_0_arm64.whl", hash = "sha256:e0641b506486f0b4cd1500a2a65740243e8670a2549bb02bc4556a83af84ae03"},
+ {file = "pydantic_core-2.14.6-pp39-pypy39_pp73-manylinux_2_17_aarch64.manylinux2014_aarch64.whl", hash = "sha256:71d72ca5eaaa8d38c8df16b7deb1a2da4f650c41b58bb142f3fb75d5ad4a611f"},
+ {file = "pydantic_core-2.14.6-pp39-pypy39_pp73-manylinux_2_17_x86_64.manylinux2014_x86_64.whl", hash = "sha256:27e524624eace5c59af499cd97dc18bb201dc6a7a2da24bfc66ef151c69a5f2a"},
+ {file = "pydantic_core-2.14.6-pp39-pypy39_pp73-manylinux_2_5_i686.manylinux1_i686.whl", hash = "sha256:a3dde6cac75e0b0902778978d3b1646ca9f438654395a362cb21d9ad34b24acf"},
+ {file = "pydantic_core-2.14.6-pp39-pypy39_pp73-musllinux_1_1_aarch64.whl", hash = "sha256:00646784f6cd993b1e1c0e7b0fdcbccc375d539db95555477771c27555e3c556"},
+ {file = "pydantic_core-2.14.6-pp39-pypy39_pp73-musllinux_1_1_x86_64.whl", hash = "sha256:23598acb8ccaa3d1d875ef3b35cb6376535095e9405d91a3d57a8c7db5d29341"},
+ {file = "pydantic_core-2.14.6-pp39-pypy39_pp73-win_amd64.whl", hash = "sha256:7f41533d7e3cf9520065f610b41ac1c76bc2161415955fbcead4981b22c7611e"},
+ {file = "pydantic_core-2.14.6.tar.gz", hash = "sha256:1fd0c1d395372843fba13a51c28e3bb9d59bd7aebfeb17358ffaaa1e4dbbe948"},
+]
+
+[package.dependencies]
+typing-extensions = ">=4.6.0,<4.7.0 || >4.7.0"
+
+[[package]]
+name = "pytest"
+version = "6.2.5"
+description = "pytest: simple powerful testing with Python"
+optional = false
+python-versions = ">=3.6"
+files = [
+ {file = "pytest-6.2.5-py3-none-any.whl", hash = "sha256:7310f8d27bc79ced999e760ca304d69f6ba6c6649c0b60fb0e04a4a77cacc134"},
+ {file = "pytest-6.2.5.tar.gz", hash = "sha256:131b36680866a76e6781d13f101efb86cf674ebb9762eb70d3082b6f29889e89"},
+]
+
+[package.dependencies]
+atomicwrites = {version = ">=1.0", markers = "sys_platform == \"win32\""}
+attrs = ">=19.2.0"
+colorama = {version = "*", markers = "sys_platform == \"win32\""}
+importlib-metadata = {version = ">=0.12", markers = "python_version < \"3.8\""}
+iniconfig = "*"
+packaging = "*"
+pluggy = ">=0.12,<2.0"
+py = ">=1.8.2"
+toml = "*"
+
+[package.extras]
+testing = ["argcomplete", "hypothesis (>=3.56)", "mock", "nose", "requests", "xmlschema"]
+
+[[package]]
+name = "pytest-asyncio"
+version = "0.17.2"
+description = "Pytest support for asyncio"
+optional = false
+python-versions = ">=3.7"
+files = [
+ {file = "pytest-asyncio-0.17.2.tar.gz", hash = "sha256:6d895b02432c028e6957d25fc936494e78c6305736e785d9fee408b1efbc7ff4"},
+ {file = "pytest_asyncio-0.17.2-py3-none-any.whl", hash = "sha256:e0fe5dbea40516b661ef1bcfe0bd9461c2847c4ef4bb40012324f2454fb7d56d"},
+]
+
+[package.dependencies]
+pytest = ">=6.1.0"
+typing-extensions = {version = ">=4.0", markers = "python_version < \"3.8\""}
+
+[package.extras]
+testing = ["coverage (==6.2)", "flaky (>=3.5.0)", "hypothesis (>=5.7.1)", "mypy (==0.931)"]
+
+[[package]]
+name = "pytest-cov"
+version = "3.0.0"
+description = "Pytest plugin for measuring coverage."
+optional = false
+python-versions = ">=3.6"
+files = [
+ {file = "pytest-cov-3.0.0.tar.gz", hash = "sha256:e7f0f5b1617d2210a2cabc266dfe2f4c75a8d32fb89eafb7ad9d06f6d076d470"},
+ {file = "pytest_cov-3.0.0-py3-none-any.whl", hash = "sha256:578d5d15ac4a25e5f961c938b85a05b09fdaae9deef3bb6de9a6e766622ca7a6"},
+]
+
+[package.dependencies]
+coverage = {version = ">=5.2.1", extras = ["toml"]}
+pytest = ">=4.6"
+
+[package.extras]
+testing = ["fields", "hunter", "process-tests", "pytest-xdist", "six", "virtualenv"]
+
+[[package]]
+name = "pyyaml"
+version = "6.0.1"
+description = "YAML parser and emitter for Python"
+optional = false
+python-versions = ">=3.6"
+files = [
+ {file = "PyYAML-6.0.1-cp310-cp310-macosx_10_9_x86_64.whl", hash = "sha256:d858aa552c999bc8a8d57426ed01e40bef403cd8ccdd0fc5f6f04a00414cac2a"},
+ {file = "PyYAML-6.0.1-cp310-cp310-macosx_11_0_arm64.whl", hash = "sha256:fd66fc5d0da6d9815ba2cebeb4205f95818ff4b79c3ebe268e75d961704af52f"},
+ {file = "PyYAML-6.0.1-cp310-cp310-manylinux_2_17_aarch64.manylinux2014_aarch64.whl", hash = "sha256:69b023b2b4daa7548bcfbd4aa3da05b3a74b772db9e23b982788168117739938"},
+ {file = "PyYAML-6.0.1-cp310-cp310-manylinux_2_17_s390x.manylinux2014_s390x.whl", hash = "sha256:81e0b275a9ecc9c0c0c07b4b90ba548307583c125f54d5b6946cfee6360c733d"},
+ {file = "PyYAML-6.0.1-cp310-cp310-manylinux_2_17_x86_64.manylinux2014_x86_64.whl", hash = "sha256:ba336e390cd8e4d1739f42dfe9bb83a3cc2e80f567d8805e11b46f4a943f5515"},
+ {file = "PyYAML-6.0.1-cp310-cp310-musllinux_1_1_x86_64.whl", hash = "sha256:326c013efe8048858a6d312ddd31d56e468118ad4cdeda36c719bf5bb6192290"},
+ {file = "PyYAML-6.0.1-cp310-cp310-win32.whl", hash = "sha256:bd4af7373a854424dabd882decdc5579653d7868b8fb26dc7d0e99f823aa5924"},
+ {file = "PyYAML-6.0.1-cp310-cp310-win_amd64.whl", hash = "sha256:fd1592b3fdf65fff2ad0004b5e363300ef59ced41c2e6b3a99d4089fa8c5435d"},
+ {file = "PyYAML-6.0.1-cp311-cp311-macosx_10_9_x86_64.whl", hash = "sha256:6965a7bc3cf88e5a1c3bd2e0b5c22f8d677dc88a455344035f03399034eb3007"},
+ {file = "PyYAML-6.0.1-cp311-cp311-macosx_11_0_arm64.whl", hash = "sha256:f003ed9ad21d6a4713f0a9b5a7a0a79e08dd0f221aff4525a2be4c346ee60aab"},
+ {file = "PyYAML-6.0.1-cp311-cp311-manylinux_2_17_aarch64.manylinux2014_aarch64.whl", hash = "sha256:42f8152b8dbc4fe7d96729ec2b99c7097d656dc1213a3229ca5383f973a5ed6d"},
+ {file = "PyYAML-6.0.1-cp311-cp311-manylinux_2_17_s390x.manylinux2014_s390x.whl", hash = "sha256:062582fca9fabdd2c8b54a3ef1c978d786e0f6b3a1510e0ac93ef59e0ddae2bc"},
+ {file = "PyYAML-6.0.1-cp311-cp311-manylinux_2_17_x86_64.manylinux2014_x86_64.whl", hash = "sha256:d2b04aac4d386b172d5b9692e2d2da8de7bfb6c387fa4f801fbf6fb2e6ba4673"},
+ {file = "PyYAML-6.0.1-cp311-cp311-musllinux_1_1_x86_64.whl", hash = "sha256:e7d73685e87afe9f3b36c799222440d6cf362062f78be1013661b00c5c6f678b"},
+ {file = "PyYAML-6.0.1-cp311-cp311-win32.whl", hash = "sha256:1635fd110e8d85d55237ab316b5b011de701ea0f29d07611174a1b42f1444741"},
+ {file = "PyYAML-6.0.1-cp311-cp311-win_amd64.whl", hash = "sha256:bf07ee2fef7014951eeb99f56f39c9bb4af143d8aa3c21b1677805985307da34"},
+ {file = "PyYAML-6.0.1-cp312-cp312-macosx_10_9_x86_64.whl", hash = "sha256:855fb52b0dc35af121542a76b9a84f8d1cd886ea97c84703eaa6d88e37a2ad28"},
+ {file = "PyYAML-6.0.1-cp312-cp312-macosx_11_0_arm64.whl", hash = "sha256:40df9b996c2b73138957fe23a16a4f0ba614f4c0efce1e9406a184b6d07fa3a9"},
+ {file = "PyYAML-6.0.1-cp312-cp312-manylinux_2_17_aarch64.manylinux2014_aarch64.whl", hash = "sha256:a08c6f0fe150303c1c6b71ebcd7213c2858041a7e01975da3a99aed1e7a378ef"},
+ {file = "PyYAML-6.0.1-cp312-cp312-manylinux_2_17_x86_64.manylinux2014_x86_64.whl", hash = "sha256:6c22bec3fbe2524cde73d7ada88f6566758a8f7227bfbf93a408a9d86bcc12a0"},
+ {file = "PyYAML-6.0.1-cp312-cp312-musllinux_1_1_x86_64.whl", hash = "sha256:8d4e9c88387b0f5c7d5f281e55304de64cf7f9c0021a3525bd3b1c542da3b0e4"},
+ {file = "PyYAML-6.0.1-cp312-cp312-win32.whl", hash = "sha256:d483d2cdf104e7c9fa60c544d92981f12ad66a457afae824d146093b8c294c54"},
+ {file = "PyYAML-6.0.1-cp312-cp312-win_amd64.whl", hash = "sha256:0d3304d8c0adc42be59c5f8a4d9e3d7379e6955ad754aa9d6ab7a398b59dd1df"},
+ {file = "PyYAML-6.0.1-cp36-cp36m-macosx_10_9_x86_64.whl", hash = "sha256:50550eb667afee136e9a77d6dc71ae76a44df8b3e51e41b77f6de2932bfe0f47"},
+ {file = "PyYAML-6.0.1-cp36-cp36m-manylinux_2_17_aarch64.manylinux2014_aarch64.whl", hash = "sha256:1fe35611261b29bd1de0070f0b2f47cb6ff71fa6595c077e42bd0c419fa27b98"},
+ {file = "PyYAML-6.0.1-cp36-cp36m-manylinux_2_17_s390x.manylinux2014_s390x.whl", hash = "sha256:704219a11b772aea0d8ecd7058d0082713c3562b4e271b849ad7dc4a5c90c13c"},
+ {file = "PyYAML-6.0.1-cp36-cp36m-manylinux_2_17_x86_64.manylinux2014_x86_64.whl", hash = "sha256:afd7e57eddb1a54f0f1a974bc4391af8bcce0b444685d936840f125cf046d5bd"},
+ {file = "PyYAML-6.0.1-cp36-cp36m-win32.whl", hash = "sha256:fca0e3a251908a499833aa292323f32437106001d436eca0e6e7833256674585"},
+ {file = "PyYAML-6.0.1-cp36-cp36m-win_amd64.whl", hash = "sha256:f22ac1c3cac4dbc50079e965eba2c1058622631e526bd9afd45fedd49ba781fa"},
+ {file = "PyYAML-6.0.1-cp37-cp37m-macosx_10_9_x86_64.whl", hash = "sha256:b1275ad35a5d18c62a7220633c913e1b42d44b46ee12554e5fd39c70a243d6a3"},
+ {file = "PyYAML-6.0.1-cp37-cp37m-manylinux_2_17_aarch64.manylinux2014_aarch64.whl", hash = "sha256:18aeb1bf9a78867dc38b259769503436b7c72f7a1f1f4c93ff9a17de54319b27"},
+ {file = "PyYAML-6.0.1-cp37-cp37m-manylinux_2_17_s390x.manylinux2014_s390x.whl", hash = "sha256:596106435fa6ad000c2991a98fa58eeb8656ef2325d7e158344fb33864ed87e3"},
+ {file = "PyYAML-6.0.1-cp37-cp37m-manylinux_2_17_x86_64.manylinux2014_x86_64.whl", hash = "sha256:baa90d3f661d43131ca170712d903e6295d1f7a0f595074f151c0aed377c9b9c"},
+ {file = "PyYAML-6.0.1-cp37-cp37m-win32.whl", hash = "sha256:9046c58c4395dff28dd494285c82ba00b546adfc7ef001486fbf0324bc174fba"},
+ {file = "PyYAML-6.0.1-cp37-cp37m-win_amd64.whl", hash = "sha256:4fb147e7a67ef577a588a0e2c17b6db51dda102c71de36f8549b6816a96e1867"},
+ {file = "PyYAML-6.0.1-cp38-cp38-macosx_10_9_x86_64.whl", hash = "sha256:1d4c7e777c441b20e32f52bd377e0c409713e8bb1386e1099c2415f26e479595"},
+ {file = "PyYAML-6.0.1-cp38-cp38-manylinux_2_17_aarch64.manylinux2014_aarch64.whl", hash = "sha256:a0cd17c15d3bb3fa06978b4e8958dcdc6e0174ccea823003a106c7d4d7899ac5"},
+ {file = "PyYAML-6.0.1-cp38-cp38-manylinux_2_17_s390x.manylinux2014_s390x.whl", hash = "sha256:28c119d996beec18c05208a8bd78cbe4007878c6dd15091efb73a30e90539696"},
+ {file = "PyYAML-6.0.1-cp38-cp38-manylinux_2_17_x86_64.manylinux2014_x86_64.whl", hash = "sha256:7e07cbde391ba96ab58e532ff4803f79c4129397514e1413a7dc761ccd755735"},
+ {file = "PyYAML-6.0.1-cp38-cp38-musllinux_1_1_x86_64.whl", hash = "sha256:49a183be227561de579b4a36efbb21b3eab9651dd81b1858589f796549873dd6"},
+ {file = "PyYAML-6.0.1-cp38-cp38-win32.whl", hash = "sha256:184c5108a2aca3c5b3d3bf9395d50893a7ab82a38004c8f61c258d4428e80206"},
+ {file = "PyYAML-6.0.1-cp38-cp38-win_amd64.whl", hash = "sha256:1e2722cc9fbb45d9b87631ac70924c11d3a401b2d7f410cc0e3bbf249f2dca62"},
+ {file = "PyYAML-6.0.1-cp39-cp39-macosx_10_9_x86_64.whl", hash = "sha256:9eb6caa9a297fc2c2fb8862bc5370d0303ddba53ba97e71f08023b6cd73d16a8"},
+ {file = "PyYAML-6.0.1-cp39-cp39-macosx_11_0_arm64.whl", hash = "sha256:c8098ddcc2a85b61647b2590f825f3db38891662cfc2fc776415143f599bb859"},
+ {file = "PyYAML-6.0.1-cp39-cp39-manylinux_2_17_aarch64.manylinux2014_aarch64.whl", hash = "sha256:5773183b6446b2c99bb77e77595dd486303b4faab2b086e7b17bc6bef28865f6"},
+ {file = "PyYAML-6.0.1-cp39-cp39-manylinux_2_17_s390x.manylinux2014_s390x.whl", hash = "sha256:b786eecbdf8499b9ca1d697215862083bd6d2a99965554781d0d8d1ad31e13a0"},
+ {file = "PyYAML-6.0.1-cp39-cp39-manylinux_2_17_x86_64.manylinux2014_x86_64.whl", hash = "sha256:bc1bf2925a1ecd43da378f4db9e4f799775d6367bdb94671027b73b393a7c42c"},
+ {file = "PyYAML-6.0.1-cp39-cp39-musllinux_1_1_x86_64.whl", hash = "sha256:04ac92ad1925b2cff1db0cfebffb6ffc43457495c9b3c39d3fcae417d7125dc5"},
+ {file = "PyYAML-6.0.1-cp39-cp39-win32.whl", hash = "sha256:faca3bdcf85b2fc05d06ff3fbc1f83e1391b3e724afa3feba7d13eeab355484c"},
+ {file = "PyYAML-6.0.1-cp39-cp39-win_amd64.whl", hash = "sha256:510c9deebc5c0225e8c96813043e62b680ba2f9c50a08d3724c7f28a747d1486"},
+ {file = "PyYAML-6.0.1.tar.gz", hash = "sha256:bfdf460b1736c775f2ba9f6a92bca30bc2095067b8a9d77876d1fad6cc3b4a43"},
+]
+
+[[package]]
+name = "requests"
+version = "2.31.0"
+description = "Python HTTP for Humans."
+optional = false
+python-versions = ">=3.7"
+files = [
+ {file = "requests-2.31.0-py3-none-any.whl", hash = "sha256:58cd2187c01e70e6e26505bca751777aa9f2ee0b7f4300988b709f44e013003f"},
+ {file = "requests-2.31.0.tar.gz", hash = "sha256:942c5a758f98d790eaed1a29cb6eefc7ffb0d1cf7af05c3d2791656dbd6ad1e1"},
+]
+
+[package.dependencies]
+certifi = ">=2017.4.17"
+charset-normalizer = ">=2,<4"
+idna = ">=2.5,<4"
+urllib3 = ">=1.21.1,<3"
+
+[package.extras]
+socks = ["PySocks (>=1.5.6,!=1.5.7)"]
+use-chardet-on-py3 = ["chardet (>=3.0.2,<6)"]
+
+[[package]]
+name = "toml"
+version = "0.10.2"
+description = "Python Library for Tom's Obvious, Minimal Language"
+optional = false
+python-versions = ">=2.6, !=3.0.*, !=3.1.*, !=3.2.*"
+files = [
+ {file = "toml-0.10.2-py2.py3-none-any.whl", hash = "sha256:806143ae5bfb6a3c6e736a764057db0e6a0e05e338b5630894a5f779cabb4f9b"},
+ {file = "toml-0.10.2.tar.gz", hash = "sha256:b3bda1d108d5dd99f4a20d24d9c348e91c4db7ab1b749200bded2f839ccbe68f"},
+]
+
+[[package]]
+name = "tomli"
+version = "2.0.1"
+description = "A lil' TOML parser"
+optional = false
+python-versions = ">=3.7"
+files = [
+ {file = "tomli-2.0.1-py3-none-any.whl", hash = "sha256:939de3e7a6161af0c887ef91b7d41a53e7c5a1ca976325f429cb46ea9bc30ecc"},
+ {file = "tomli-2.0.1.tar.gz", hash = "sha256:de526c12914f0c550d15924c62d72abc48d6fe7364aa87328337a31007fe8a4f"},
+]
+
+[[package]]
+name = "tqdm"
+version = "4.66.1"
+description = "Fast, Extensible Progress Meter"
+optional = false
+python-versions = ">=3.7"
+files = [
+ {file = "tqdm-4.66.1-py3-none-any.whl", hash = "sha256:d302b3c5b53d47bce91fea46679d9c3c6508cf6332229aa1e7d8653723793386"},
+ {file = "tqdm-4.66.1.tar.gz", hash = "sha256:d88e651f9db8d8551a62556d3cff9e3034274ca5d66e93197cf2490e2dcb69c7"},
+]
+
+[package.dependencies]
+colorama = {version = "*", markers = "platform_system == \"Windows\""}
+
+[package.extras]
+dev = ["pytest (>=6)", "pytest-cov", "pytest-timeout", "pytest-xdist"]
+notebook = ["ipywidgets (>=6)"]
+slack = ["slack-sdk"]
+telegram = ["requests"]
+
+[[package]]
+name = "typing-extensions"
+version = "4.7.1"
+description = "Backported and Experimental Type Hints for Python 3.7+"
+optional = false
+python-versions = ">=3.7"
+files = [
+ {file = "typing_extensions-4.7.1-py3-none-any.whl", hash = "sha256:440d5dd3af93b060174bf433bccd69b0babc3b15b1a8dca43789fd7f61514b36"},
+ {file = "typing_extensions-4.7.1.tar.gz", hash = "sha256:b75ddc264f0ba5615db7ba217daeb99701ad295353c45f9e95963337ceeeffb2"},
+]
+
+[[package]]
+name = "urllib3"
+version = "2.0.5"
+description = "HTTP library with thread-safe connection pooling, file post, and more."
+optional = false
+python-versions = ">=3.7"
+files = [
+ {file = "urllib3-2.0.5-py3-none-any.whl", hash = "sha256:ef16afa8ba34a1f989db38e1dbbe0c302e4289a47856990d0682e374563ce35e"},
+ {file = "urllib3-2.0.5.tar.gz", hash = "sha256:13abf37382ea2ce6fb744d4dad67838eec857c9f4f57009891805e0b5e123594"},
+]
+
+[package.extras]
+brotli = ["brotli (>=1.0.9)", "brotlicffi (>=0.8.0)"]
+secure = ["certifi", "cryptography (>=1.9)", "idna (>=2.0.0)", "pyopenssl (>=17.1.0)", "urllib3-secure-extra"]
+socks = ["pysocks (>=1.5.6,!=1.5.7,<2.0)"]
+zstd = ["zstandard (>=0.18.0)"]
+
+[[package]]
+name = "yarl"
+version = "1.9.2"
+description = "Yet another URL library"
+optional = false
+python-versions = ">=3.7"
+files = [
+ {file = "yarl-1.9.2-cp310-cp310-macosx_10_9_universal2.whl", hash = "sha256:8c2ad583743d16ddbdf6bb14b5cd76bf43b0d0006e918809d5d4ddf7bde8dd82"},
+ {file = "yarl-1.9.2-cp310-cp310-macosx_10_9_x86_64.whl", hash = "sha256:82aa6264b36c50acfb2424ad5ca537a2060ab6de158a5bd2a72a032cc75b9eb8"},
+ {file = "yarl-1.9.2-cp310-cp310-macosx_11_0_arm64.whl", hash = "sha256:c0c77533b5ed4bcc38e943178ccae29b9bcf48ffd1063f5821192f23a1bd27b9"},
+ {file = "yarl-1.9.2-cp310-cp310-manylinux_2_17_aarch64.manylinux2014_aarch64.whl", hash = "sha256:ee4afac41415d52d53a9833ebae7e32b344be72835bbb589018c9e938045a560"},
+ {file = "yarl-1.9.2-cp310-cp310-manylinux_2_17_ppc64le.manylinux2014_ppc64le.whl", hash = "sha256:9bf345c3a4f5ba7f766430f97f9cc1320786f19584acc7086491f45524a551ac"},
+ {file = "yarl-1.9.2-cp310-cp310-manylinux_2_17_s390x.manylinux2014_s390x.whl", hash = "sha256:2a96c19c52ff442a808c105901d0bdfd2e28575b3d5f82e2f5fd67e20dc5f4ea"},
+ {file = "yarl-1.9.2-cp310-cp310-manylinux_2_17_x86_64.manylinux2014_x86_64.whl", hash = "sha256:891c0e3ec5ec881541f6c5113d8df0315ce5440e244a716b95f2525b7b9f3608"},
+ {file = "yarl-1.9.2-cp310-cp310-manylinux_2_5_i686.manylinux1_i686.manylinux_2_17_i686.manylinux2014_i686.whl", hash = "sha256:c3a53ba34a636a256d767c086ceb111358876e1fb6b50dfc4d3f4951d40133d5"},
+ {file = "yarl-1.9.2-cp310-cp310-musllinux_1_1_aarch64.whl", hash = "sha256:566185e8ebc0898b11f8026447eacd02e46226716229cea8db37496c8cdd26e0"},
+ {file = "yarl-1.9.2-cp310-cp310-musllinux_1_1_i686.whl", hash = "sha256:2b0738fb871812722a0ac2154be1f049c6223b9f6f22eec352996b69775b36d4"},
+ {file = "yarl-1.9.2-cp310-cp310-musllinux_1_1_ppc64le.whl", hash = "sha256:32f1d071b3f362c80f1a7d322bfd7b2d11e33d2adf395cc1dd4df36c9c243095"},
+ {file = "yarl-1.9.2-cp310-cp310-musllinux_1_1_s390x.whl", hash = "sha256:e9fdc7ac0d42bc3ea78818557fab03af6181e076a2944f43c38684b4b6bed8e3"},
+ {file = "yarl-1.9.2-cp310-cp310-musllinux_1_1_x86_64.whl", hash = "sha256:56ff08ab5df8429901ebdc5d15941b59f6253393cb5da07b4170beefcf1b2528"},
+ {file = "yarl-1.9.2-cp310-cp310-win32.whl", hash = "sha256:8ea48e0a2f931064469bdabca50c2f578b565fc446f302a79ba6cc0ee7f384d3"},
+ {file = "yarl-1.9.2-cp310-cp310-win_amd64.whl", hash = "sha256:50f33040f3836e912ed16d212f6cc1efb3231a8a60526a407aeb66c1c1956dde"},
+ {file = "yarl-1.9.2-cp311-cp311-macosx_10_9_universal2.whl", hash = "sha256:646d663eb2232d7909e6601f1a9107e66f9791f290a1b3dc7057818fe44fc2b6"},
+ {file = "yarl-1.9.2-cp311-cp311-macosx_10_9_x86_64.whl", hash = "sha256:aff634b15beff8902d1f918012fc2a42e0dbae6f469fce134c8a0dc51ca423bb"},
+ {file = "yarl-1.9.2-cp311-cp311-macosx_11_0_arm64.whl", hash = "sha256:a83503934c6273806aed765035716216cc9ab4e0364f7f066227e1aaea90b8d0"},
+ {file = "yarl-1.9.2-cp311-cp311-manylinux_2_17_aarch64.manylinux2014_aarch64.whl", hash = "sha256:b25322201585c69abc7b0e89e72790469f7dad90d26754717f3310bfe30331c2"},
+ {file = "yarl-1.9.2-cp311-cp311-manylinux_2_17_ppc64le.manylinux2014_ppc64le.whl", hash = "sha256:22a94666751778629f1ec4280b08eb11815783c63f52092a5953faf73be24191"},
+ {file = "yarl-1.9.2-cp311-cp311-manylinux_2_17_s390x.manylinux2014_s390x.whl", hash = "sha256:8ec53a0ea2a80c5cd1ab397925f94bff59222aa3cf9c6da938ce05c9ec20428d"},
+ {file = "yarl-1.9.2-cp311-cp311-manylinux_2_17_x86_64.manylinux2014_x86_64.whl", hash = "sha256:159d81f22d7a43e6eabc36d7194cb53f2f15f498dbbfa8edc8a3239350f59fe7"},
+ {file = "yarl-1.9.2-cp311-cp311-manylinux_2_5_i686.manylinux1_i686.manylinux_2_17_i686.manylinux2014_i686.whl", hash = "sha256:832b7e711027c114d79dffb92576acd1bd2decc467dec60e1cac96912602d0e6"},
+ {file = "yarl-1.9.2-cp311-cp311-musllinux_1_1_aarch64.whl", hash = "sha256:95d2ecefbcf4e744ea952d073c6922e72ee650ffc79028eb1e320e732898d7e8"},
+ {file = "yarl-1.9.2-cp311-cp311-musllinux_1_1_i686.whl", hash = "sha256:d4e2c6d555e77b37288eaf45b8f60f0737c9efa3452c6c44626a5455aeb250b9"},
+ {file = "yarl-1.9.2-cp311-cp311-musllinux_1_1_ppc64le.whl", hash = "sha256:783185c75c12a017cc345015ea359cc801c3b29a2966c2655cd12b233bf5a2be"},
+ {file = "yarl-1.9.2-cp311-cp311-musllinux_1_1_s390x.whl", hash = "sha256:b8cc1863402472f16c600e3e93d542b7e7542a540f95c30afd472e8e549fc3f7"},
+ {file = "yarl-1.9.2-cp311-cp311-musllinux_1_1_x86_64.whl", hash = "sha256:822b30a0f22e588b32d3120f6d41e4ed021806418b4c9f0bc3048b8c8cb3f92a"},
+ {file = "yarl-1.9.2-cp311-cp311-win32.whl", hash = "sha256:a60347f234c2212a9f0361955007fcf4033a75bf600a33c88a0a8e91af77c0e8"},
+ {file = "yarl-1.9.2-cp311-cp311-win_amd64.whl", hash = "sha256:be6b3fdec5c62f2a67cb3f8c6dbf56bbf3f61c0f046f84645cd1ca73532ea051"},
+ {file = "yarl-1.9.2-cp37-cp37m-macosx_10_9_x86_64.whl", hash = "sha256:38a3928ae37558bc1b559f67410df446d1fbfa87318b124bf5032c31e3447b74"},
+ {file = "yarl-1.9.2-cp37-cp37m-manylinux_2_17_aarch64.manylinux2014_aarch64.whl", hash = "sha256:ac9bb4c5ce3975aeac288cfcb5061ce60e0d14d92209e780c93954076c7c4367"},
+ {file = "yarl-1.9.2-cp37-cp37m-manylinux_2_17_ppc64le.manylinux2014_ppc64le.whl", hash = "sha256:3da8a678ca8b96c8606bbb8bfacd99a12ad5dd288bc6f7979baddd62f71c63ef"},
+ {file = "yarl-1.9.2-cp37-cp37m-manylinux_2_17_s390x.manylinux2014_s390x.whl", hash = "sha256:13414591ff516e04fcdee8dc051c13fd3db13b673c7a4cb1350e6b2ad9639ad3"},
+ {file = "yarl-1.9.2-cp37-cp37m-manylinux_2_17_x86_64.manylinux2014_x86_64.whl", hash = "sha256:bf74d08542c3a9ea97bb8f343d4fcbd4d8f91bba5ec9d5d7f792dbe727f88938"},
+ {file = "yarl-1.9.2-cp37-cp37m-manylinux_2_5_i686.manylinux1_i686.manylinux_2_17_i686.manylinux2014_i686.whl", hash = "sha256:6e7221580dc1db478464cfeef9b03b95c5852cc22894e418562997df0d074ccc"},
+ {file = "yarl-1.9.2-cp37-cp37m-musllinux_1_1_aarch64.whl", hash = "sha256:494053246b119b041960ddcd20fd76224149cfea8ed8777b687358727911dd33"},
+ {file = "yarl-1.9.2-cp37-cp37m-musllinux_1_1_i686.whl", hash = "sha256:52a25809fcbecfc63ac9ba0c0fb586f90837f5425edfd1ec9f3372b119585e45"},
+ {file = "yarl-1.9.2-cp37-cp37m-musllinux_1_1_ppc64le.whl", hash = "sha256:e65610c5792870d45d7b68c677681376fcf9cc1c289f23e8e8b39c1485384185"},
+ {file = "yarl-1.9.2-cp37-cp37m-musllinux_1_1_s390x.whl", hash = "sha256:1b1bba902cba32cdec51fca038fd53f8beee88b77efc373968d1ed021024cc04"},
+ {file = "yarl-1.9.2-cp37-cp37m-musllinux_1_1_x86_64.whl", hash = "sha256:662e6016409828ee910f5d9602a2729a8a57d74b163c89a837de3fea050c7582"},
+ {file = "yarl-1.9.2-cp37-cp37m-win32.whl", hash = "sha256:f364d3480bffd3aa566e886587eaca7c8c04d74f6e8933f3f2c996b7f09bee1b"},
+ {file = "yarl-1.9.2-cp37-cp37m-win_amd64.whl", hash = "sha256:6a5883464143ab3ae9ba68daae8e7c5c95b969462bbe42e2464d60e7e2698368"},
+ {file = "yarl-1.9.2-cp38-cp38-macosx_10_9_universal2.whl", hash = "sha256:5610f80cf43b6202e2c33ba3ec2ee0a2884f8f423c8f4f62906731d876ef4fac"},
+ {file = "yarl-1.9.2-cp38-cp38-macosx_10_9_x86_64.whl", hash = "sha256:b9a4e67ad7b646cd6f0938c7ebfd60e481b7410f574c560e455e938d2da8e0f4"},
+ {file = "yarl-1.9.2-cp38-cp38-macosx_11_0_arm64.whl", hash = "sha256:83fcc480d7549ccebe9415d96d9263e2d4226798c37ebd18c930fce43dfb9574"},
+ {file = "yarl-1.9.2-cp38-cp38-manylinux_2_17_aarch64.manylinux2014_aarch64.whl", hash = "sha256:5fcd436ea16fee7d4207c045b1e340020e58a2597301cfbcfdbe5abd2356c2fb"},
+ {file = "yarl-1.9.2-cp38-cp38-manylinux_2_17_ppc64le.manylinux2014_ppc64le.whl", hash = "sha256:84e0b1599334b1e1478db01b756e55937d4614f8654311eb26012091be109d59"},
+ {file = "yarl-1.9.2-cp38-cp38-manylinux_2_17_s390x.manylinux2014_s390x.whl", hash = "sha256:3458a24e4ea3fd8930e934c129b676c27452e4ebda80fbe47b56d8c6c7a63a9e"},
+ {file = "yarl-1.9.2-cp38-cp38-manylinux_2_17_x86_64.manylinux2014_x86_64.whl", hash = "sha256:838162460b3a08987546e881a2bfa573960bb559dfa739e7800ceeec92e64417"},
+ {file = "yarl-1.9.2-cp38-cp38-manylinux_2_5_i686.manylinux1_i686.manylinux_2_17_i686.manylinux2014_i686.whl", hash = "sha256:f4e2d08f07a3d7d3e12549052eb5ad3eab1c349c53ac51c209a0e5991bbada78"},
+ {file = "yarl-1.9.2-cp38-cp38-musllinux_1_1_aarch64.whl", hash = "sha256:de119f56f3c5f0e2fb4dee508531a32b069a5f2c6e827b272d1e0ff5ac040333"},
+ {file = "yarl-1.9.2-cp38-cp38-musllinux_1_1_i686.whl", hash = "sha256:149ddea5abf329752ea5051b61bd6c1d979e13fbf122d3a1f9f0c8be6cb6f63c"},
+ {file = "yarl-1.9.2-cp38-cp38-musllinux_1_1_ppc64le.whl", hash = "sha256:674ca19cbee4a82c9f54e0d1eee28116e63bc6fd1e96c43031d11cbab8b2afd5"},
+ {file = "yarl-1.9.2-cp38-cp38-musllinux_1_1_s390x.whl", hash = "sha256:9b3152f2f5677b997ae6c804b73da05a39daa6a9e85a512e0e6823d81cdad7cc"},
+ {file = "yarl-1.9.2-cp38-cp38-musllinux_1_1_x86_64.whl", hash = "sha256:5415d5a4b080dc9612b1b63cba008db84e908b95848369aa1da3686ae27b6d2b"},
+ {file = "yarl-1.9.2-cp38-cp38-win32.whl", hash = "sha256:f7a3d8146575e08c29ed1cd287068e6d02f1c7bdff8970db96683b9591b86ee7"},
+ {file = "yarl-1.9.2-cp38-cp38-win_amd64.whl", hash = "sha256:63c48f6cef34e6319a74c727376e95626f84ea091f92c0250a98e53e62c77c72"},
+ {file = "yarl-1.9.2-cp39-cp39-macosx_10_9_universal2.whl", hash = "sha256:75df5ef94c3fdc393c6b19d80e6ef1ecc9ae2f4263c09cacb178d871c02a5ba9"},
+ {file = "yarl-1.9.2-cp39-cp39-macosx_10_9_x86_64.whl", hash = "sha256:c027a6e96ef77d401d8d5a5c8d6bc478e8042f1e448272e8d9752cb0aff8b5c8"},
+ {file = "yarl-1.9.2-cp39-cp39-macosx_11_0_arm64.whl", hash = "sha256:f3b078dbe227f79be488ffcfc7a9edb3409d018e0952cf13f15fd6512847f3f7"},
+ {file = "yarl-1.9.2-cp39-cp39-manylinux_2_17_aarch64.manylinux2014_aarch64.whl", hash = "sha256:59723a029760079b7d991a401386390c4be5bfec1e7dd83e25a6a0881859e716"},
+ {file = "yarl-1.9.2-cp39-cp39-manylinux_2_17_ppc64le.manylinux2014_ppc64le.whl", hash = "sha256:b03917871bf859a81ccb180c9a2e6c1e04d2f6a51d953e6a5cdd70c93d4e5a2a"},
+ {file = "yarl-1.9.2-cp39-cp39-manylinux_2_17_s390x.manylinux2014_s390x.whl", hash = "sha256:c1012fa63eb6c032f3ce5d2171c267992ae0c00b9e164efe4d73db818465fac3"},
+ {file = "yarl-1.9.2-cp39-cp39-manylinux_2_17_x86_64.manylinux2014_x86_64.whl", hash = "sha256:a74dcbfe780e62f4b5a062714576f16c2f3493a0394e555ab141bf0d746bb955"},
+ {file = "yarl-1.9.2-cp39-cp39-manylinux_2_5_i686.manylinux1_i686.manylinux_2_17_i686.manylinux2014_i686.whl", hash = "sha256:8c56986609b057b4839968ba901944af91b8e92f1725d1a2d77cbac6972b9ed1"},
+ {file = "yarl-1.9.2-cp39-cp39-musllinux_1_1_aarch64.whl", hash = "sha256:2c315df3293cd521033533d242d15eab26583360b58f7ee5d9565f15fee1bef4"},
+ {file = "yarl-1.9.2-cp39-cp39-musllinux_1_1_i686.whl", hash = "sha256:b7232f8dfbd225d57340e441d8caf8652a6acd06b389ea2d3222b8bc89cbfca6"},
+ {file = "yarl-1.9.2-cp39-cp39-musllinux_1_1_ppc64le.whl", hash = "sha256:53338749febd28935d55b41bf0bcc79d634881195a39f6b2f767870b72514caf"},
+ {file = "yarl-1.9.2-cp39-cp39-musllinux_1_1_s390x.whl", hash = "sha256:066c163aec9d3d073dc9ffe5dd3ad05069bcb03fcaab8d221290ba99f9f69ee3"},
+ {file = "yarl-1.9.2-cp39-cp39-musllinux_1_1_x86_64.whl", hash = "sha256:8288d7cd28f8119b07dd49b7230d6b4562f9b61ee9a4ab02221060d21136be80"},
+ {file = "yarl-1.9.2-cp39-cp39-win32.whl", hash = "sha256:b124e2a6d223b65ba8768d5706d103280914d61f5cae3afbc50fc3dfcc016623"},
+ {file = "yarl-1.9.2-cp39-cp39-win_amd64.whl", hash = "sha256:61016e7d582bc46a5378ffdd02cd0314fb8ba52f40f9cf4d9a5e7dbef88dee18"},
+ {file = "yarl-1.9.2.tar.gz", hash = "sha256:04ab9d4b9f587c06d801c2abfe9317b77cdf996c65a90d5e84ecc45010823571"},
+]
+
+[package.dependencies]
+idna = ">=2.0"
+multidict = ">=4.0"
+typing-extensions = {version = ">=3.7.4", markers = "python_version < \"3.8\""}
+
+[[package]]
+name = "zipp"
+version = "3.15.0"
+description = "Backport of pathlib-compatible object wrapper for zip files"
+optional = false
+python-versions = ">=3.7"
+files = [
+ {file = "zipp-3.15.0-py3-none-any.whl", hash = "sha256:48904fc76a60e542af151aded95726c1a5c34ed43ab4134b597665c86d7ad556"},
+ {file = "zipp-3.15.0.tar.gz", hash = "sha256:112929ad649da941c23de50f356a2b5570c954b65150642bccdd66bf194d224b"},
+]
+
+[package.extras]
+docs = ["furo", "jaraco.packaging (>=9)", "jaraco.tidelift (>=1.4)", "rst.linker (>=1.9)", "sphinx (>=3.5)", "sphinx-lint"]
+testing = ["big-O", "flake8 (<5)", "jaraco.functools", "jaraco.itertools", "more-itertools", "pytest (>=6)", "pytest-black (>=0.3.7)", "pytest-checkdocs (>=2.4)", "pytest-cov", "pytest-enabler (>=1.3)", "pytest-flake8", "pytest-mypy (>=0.9.1)"]
+
+[metadata]
+lock-version = "2.0"
+python-versions = "^3.7"
+content-hash = "b7fab8703967f2616ea59a98a437cd30f97f0c8d2a06e399d688814a2a2c64f8"
diff --git a/clients/python/pyproject.toml b/clients/python/pyproject.toml
new file mode 100644
index 00000000..2925085b
--- /dev/null
+++ b/clients/python/pyproject.toml
@@ -0,0 +1,29 @@
+[tool.poetry]
+name = "text-generation"
+version = "0.7.0"
+description = "Hugging Face Text Generation Python Client"
+license = "Apache-2.0"
+authors = ["Olivier Dehaene "]
+maintainers = ["Olivier Dehaene "]
+readme = "README.md"
+homepage = "https://github.com/huggingface/text-generation-inference"
+repository = "https://github.com/huggingface/text-generation-inference"
+
+
+[tool.poetry.dependencies]
+python = "^3.7"
+pydantic = "> 2, < 3"
+aiohttp = "^3.8"
+huggingface-hub = ">= 0.12, < 1.0"
+
+[tool.poetry.dev-dependencies]
+pytest = "^6.2.5"
+pytest-asyncio = "^0.17.2"
+pytest-cov = "^3.0.0"
+
+[tool.pytest.ini_options]
+asyncio_mode = "auto"
+
+[build-system]
+requires = ["poetry-core>=1.0.0"]
+build-backend = "poetry.core.masonry.api"
diff --git a/clients/python/tests/conftest.py b/clients/python/tests/conftest.py
new file mode 100644
index 00000000..10a5b599
--- /dev/null
+++ b/clients/python/tests/conftest.py
@@ -0,0 +1,61 @@
+import pytest
+
+from text_generation import __version__
+from huggingface_hub.utils import build_hf_headers
+
+
+@pytest.fixture
+def flan_t5_xxl():
+ return "google/flan-t5-xxl"
+
+
+@pytest.fixture
+def llama_7b():
+ return "" # "meta-llama/Llama-2-7b-chat-hf"
+
+
+@pytest.fixture
+def fake_model():
+ return "fake/model"
+
+
+@pytest.fixture
+def unsupported_model():
+ return "gpt2"
+
+
+@pytest.fixture
+def base_url():
+ return "http://127.0.0.1:3000" # "https://api-inference.huggingface.co/models"
+
+
+@pytest.fixture
+def bloom_url(base_url, bloom_model):
+ return f"{base_url}/{bloom_model}"
+
+
+@pytest.fixture
+def flan_t5_xxl_url(base_url, flan_t5_xxl):
+ return f"{base_url}/{flan_t5_xxl}"
+
+
+@pytest.fixture
+def llama_7b_url(base_url, llama_7b):
+ return f"{base_url}/{llama_7b}"
+
+
+@pytest.fixture
+def fake_url(base_url, fake_model):
+ return f"{base_url}/{fake_model}"
+
+
+@pytest.fixture
+def unsupported_url(base_url, unsupported_model):
+ return f"{base_url}/{unsupported_model}"
+
+
+@pytest.fixture(scope="session")
+def hf_headers():
+ return build_hf_headers(
+ library_name="text-generation-tests", library_version=__version__
+ )
diff --git a/clients/python/tests/test_client.py b/clients/python/tests/test_client.py
new file mode 100644
index 00000000..2cfd019d
--- /dev/null
+++ b/clients/python/tests/test_client.py
@@ -0,0 +1,167 @@
+import pytest
+
+from text_generation import Client, AsyncClient
+from text_generation.errors import NotFoundError, ValidationError
+from text_generation.types import FinishReason, InputToken
+
+
+def test_generate_lora(llama_7b_url, hf_headers):
+ client = Client(llama_7b_url, hf_headers)
+ response = client.generate(
+ "test",
+ max_new_tokens=1,
+ decoder_input_details=True,
+ lora_id="abcdabcd987/gsm8k-llama2-7b-lora-16",
+ )
+ assert response.generated_text == "_"
+
+ response = client.download_lora_adapter("abcdabcd987/gsm8k-llama2-7b-lora-16")
+ assert response.status_code == 200
+
+
+def test_generate(llama_7b_url, hf_headers):
+ client = Client(llama_7b_url, hf_headers)
+ response = client.generate("test", max_new_tokens=1, decoder_input_details=True)
+
+ assert response.generated_text == "_"
+ assert response.details.finish_reason == FinishReason.Length
+ assert response.details.generated_tokens == 1
+ assert response.details.seed is None
+ assert len(response.details.prefill) == 2
+ assert response.details.prefill[0] == InputToken(id=1, text="", logprob=None)
+ assert len(response.details.tokens) == 1
+ assert response.details.tokens[0].id == 29918
+ assert response.details.tokens[0].text == "_"
+ assert not response.details.tokens[0].special
+
+
+def test_generate_best_of(llama_7b_url, hf_headers):
+ client = Client(llama_7b_url, hf_headers)
+ response = client.generate(
+ "test", max_new_tokens=1, best_of=2, do_sample=True, decoder_input_details=True
+ )
+
+ assert response.details.seed is not None
+ assert response.details.best_of_sequences is not None
+ assert len(response.details.best_of_sequences) == 1
+ assert response.details.best_of_sequences[0].seed is not None
+
+
+def test_generate_not_found(fake_url, hf_headers):
+ client = Client(fake_url, hf_headers)
+ with pytest.raises(NotFoundError):
+ client.generate("test")
+
+
+def test_generate_validation_error(llama_7b_url, hf_headers):
+ client = Client(llama_7b_url, hf_headers)
+ with pytest.raises(ValidationError):
+ client.generate("test", max_new_tokens=10_000)
+
+
+def test_generate_stream(llama_7b_url, hf_headers):
+ client = Client(llama_7b_url, hf_headers)
+ responses = [
+ response for response in client.generate_stream("test", max_new_tokens=1)
+ ]
+
+ assert len(responses) == 1
+ response = responses[0]
+
+ assert response.generated_text == "_"
+ assert response.details.finish_reason == FinishReason.Length
+ assert response.details.generated_tokens == 1
+ assert response.details.seed is None
+
+
+def test_generate_stream_not_found(fake_url, hf_headers):
+ client = Client(fake_url, hf_headers)
+ with pytest.raises(NotFoundError):
+ list(client.generate_stream("test"))
+
+
+def test_generate_stream_validation_error(llama_7b_url, hf_headers):
+ client = Client(llama_7b_url, hf_headers)
+ with pytest.raises(ValidationError):
+ list(client.generate_stream("test", max_new_tokens=10_000))
+
+
+@pytest.mark.asyncio
+async def test_generate_async(llama_7b_url, hf_headers):
+ client = AsyncClient(llama_7b_url, hf_headers)
+ response = await client.generate(
+ "test", max_new_tokens=1, decoder_input_details=True
+ )
+
+ assert response.generated_text == "_"
+ assert response.details.finish_reason == FinishReason.Length
+ assert response.details.generated_tokens == 1
+ assert response.details.seed is None
+ assert len(response.details.prefill) == 2
+ assert response.details.prefill[0] == InputToken(id=1, text="", logprob=None)
+ assert response.details.prefill[1] == InputToken(
+ id=1243, text="test", logprob=-10.96875
+ )
+ assert len(response.details.tokens) == 1
+ assert response.details.tokens[0].id == 29918
+ assert response.details.tokens[0].text == "_"
+ assert not response.details.tokens[0].special
+
+
+@pytest.mark.asyncio
+async def test_generate_async_best_of(llama_7b_url, hf_headers):
+ client = AsyncClient(llama_7b_url, hf_headers)
+ response = await client.generate(
+ "test", max_new_tokens=1, best_of=2, do_sample=True, decoder_input_details=True
+ )
+
+ assert response.details.seed is not None
+ assert response.details.best_of_sequences is not None
+ assert len(response.details.best_of_sequences) == 1
+ assert response.details.best_of_sequences[0].seed is not None
+
+
+@pytest.mark.asyncio
+async def test_generate_async_not_found(fake_url, hf_headers):
+ client = AsyncClient(fake_url, hf_headers)
+ with pytest.raises(NotFoundError):
+ await client.generate("test")
+
+
+@pytest.mark.asyncio
+async def test_generate_async_validation_error(llama_7b_url, hf_headers):
+ client = AsyncClient(llama_7b_url, hf_headers)
+ with pytest.raises(ValidationError):
+ await client.generate("test", max_new_tokens=10_000)
+
+
+@pytest.mark.asyncio
+async def test_generate_stream_async(llama_7b_url, hf_headers):
+ client = AsyncClient(llama_7b_url, hf_headers)
+ responses = [
+ response async for response in client.generate_stream("test", max_new_tokens=1)
+ ]
+
+ assert len(responses) == 1
+ response = responses[0]
+
+ assert response.generated_text == "_"
+ assert response.details.finish_reason == FinishReason.Length
+ assert response.details.generated_tokens == 1
+ assert response.details.seed is None
+
+
+@pytest.mark.asyncio
+async def test_generate_stream_async_not_found(fake_url, hf_headers):
+ client = AsyncClient(fake_url, hf_headers)
+ with pytest.raises(NotFoundError):
+ async for _ in client.generate_stream("test"):
+ pass
+
+
+@pytest.mark.asyncio
+async def test_generate_stream_async_validation_error(llama_7b_url, hf_headers):
+ client = AsyncClient(llama_7b_url, hf_headers)
+ with pytest.raises(ValidationError):
+ async for _ in client.generate_stream("test", max_new_tokens=10_000):
+ pass
diff --git a/clients/python/tests/test_errors.py b/clients/python/tests/test_errors.py
new file mode 100644
index 00000000..8389ed31
--- /dev/null
+++ b/clients/python/tests/test_errors.py
@@ -0,0 +1,64 @@
+from text_generation.errors import (
+ parse_error,
+ GenerationError,
+ IncompleteGenerationError,
+ OverloadedError,
+ ValidationError,
+ BadRequestError,
+ ShardNotReadyError,
+ ShardTimeoutError,
+ NotFoundError,
+ RateLimitExceededError,
+ UnknownError,
+)
+
+
+def test_generation_error():
+ payload = {"error_type": "generation", "error": "test"}
+ assert isinstance(parse_error(400, payload), GenerationError)
+
+
+def test_incomplete_generation_error():
+ payload = {"error_type": "incomplete_generation", "error": "test"}
+ assert isinstance(parse_error(400, payload), IncompleteGenerationError)
+
+
+def test_overloaded_error():
+ payload = {"error_type": "overloaded", "error": "test"}
+ assert isinstance(parse_error(400, payload), OverloadedError)
+
+
+def test_validation_error():
+ payload = {"error_type": "validation", "error": "test"}
+ assert isinstance(parse_error(400, payload), ValidationError)
+
+
+def test_bad_request_error():
+ payload = {"error": "test"}
+ assert isinstance(parse_error(400, payload), BadRequestError)
+
+
+def test_shard_not_ready_error():
+ payload = {"error": "test"}
+ assert isinstance(parse_error(403, payload), ShardNotReadyError)
+ assert isinstance(parse_error(424, payload), ShardNotReadyError)
+
+
+def test_shard_timeout_error():
+ payload = {"error": "test"}
+ assert isinstance(parse_error(504, payload), ShardTimeoutError)
+
+
+def test_not_found_error():
+ payload = {"error": "test"}
+ assert isinstance(parse_error(404, payload), NotFoundError)
+
+
+def test_rate_limit_exceeded_error():
+ payload = {"error": "test"}
+ assert isinstance(parse_error(429, payload), RateLimitExceededError)
+
+
+def test_unknown_error():
+ payload = {"error": "test"}
+ assert isinstance(parse_error(500, payload), UnknownError)
diff --git a/clients/python/tests/test_inference_api.py b/clients/python/tests/test_inference_api.py
new file mode 100644
index 00000000..59297c26
--- /dev/null
+++ b/clients/python/tests/test_inference_api.py
@@ -0,0 +1,42 @@
+import pytest
+
+from text_generation import (
+ InferenceAPIClient,
+ InferenceAPIAsyncClient,
+ Client,
+ AsyncClient,
+)
+from text_generation.errors import NotSupportedError, NotFoundError
+from text_generation.inference_api import check_model_support, deployed_models
+
+
+def test_check_model_support(flan_t5_xxl, unsupported_model, fake_model):
+ assert check_model_support(flan_t5_xxl)
+ assert not check_model_support(unsupported_model)
+
+ with pytest.raises(NotFoundError):
+ check_model_support(fake_model)
+
+
+def test_deployed_models():
+ deployed_models()
+
+
+def test_client(flan_t5_xxl):
+ client = InferenceAPIClient(flan_t5_xxl)
+ assert isinstance(client, Client)
+
+
+def test_client_unsupported_model(unsupported_model):
+ with pytest.raises(NotSupportedError):
+ InferenceAPIClient(unsupported_model)
+
+
+def test_async_client(flan_t5_xxl):
+ client = InferenceAPIAsyncClient(flan_t5_xxl)
+ assert isinstance(client, AsyncClient)
+
+
+def test_async_client_unsupported_model(unsupported_model):
+ with pytest.raises(NotSupportedError):
+ InferenceAPIAsyncClient(unsupported_model)
diff --git a/clients/python/tests/test_types.py b/clients/python/tests/test_types.py
new file mode 100644
index 00000000..77689ade
--- /dev/null
+++ b/clients/python/tests/test_types.py
@@ -0,0 +1,84 @@
+import pytest
+
+from text_generation.types import Parameters, Request
+from text_generation.errors import ValidationError
+
+
+def test_parameters_validation():
+ # Test best_of
+ Parameters(best_of=1)
+ with pytest.raises(ValidationError):
+ Parameters(best_of=0)
+ with pytest.raises(ValidationError):
+ Parameters(best_of=-1)
+ Parameters(best_of=2, do_sample=True)
+ with pytest.raises(ValidationError):
+ Parameters(best_of=2)
+ with pytest.raises(ValidationError):
+ Parameters(best_of=2, seed=1)
+
+ # Test repetition_penalty
+ Parameters(repetition_penalty=1)
+ with pytest.raises(ValidationError):
+ Parameters(repetition_penalty=0)
+ with pytest.raises(ValidationError):
+ Parameters(repetition_penalty=-1)
+
+ # Test seed
+ Parameters(seed=1)
+ with pytest.raises(ValidationError):
+ Parameters(seed=-1)
+
+ # Test temperature
+ Parameters(temperature=1)
+ with pytest.raises(ValidationError):
+ Parameters(temperature=0)
+ with pytest.raises(ValidationError):
+ Parameters(temperature=-1)
+
+ # Test top_k
+ Parameters(top_k=1)
+ with pytest.raises(ValidationError):
+ Parameters(top_k=0)
+ with pytest.raises(ValidationError):
+ Parameters(top_k=-1)
+
+ # Test top_p
+ Parameters(top_p=0.5)
+ with pytest.raises(ValidationError):
+ Parameters(top_p=0)
+ with pytest.raises(ValidationError):
+ Parameters(top_p=-1)
+ with pytest.raises(ValidationError):
+ Parameters(top_p=1)
+
+ # Test truncate
+ Parameters(truncate=1)
+ with pytest.raises(ValidationError):
+ Parameters(truncate=0)
+ with pytest.raises(ValidationError):
+ Parameters(truncate=-1)
+
+ # Test typical_p
+ Parameters(typical_p=0.5)
+ with pytest.raises(ValidationError):
+ Parameters(typical_p=0)
+ with pytest.raises(ValidationError):
+ Parameters(typical_p=-1)
+ with pytest.raises(ValidationError):
+ Parameters(typical_p=1)
+
+
+def test_request_validation():
+ Request(inputs="test")
+
+ with pytest.raises(ValidationError):
+ Request(inputs="")
+
+ Request(inputs="test", stream=True)
+ Request(inputs="test", parameters=Parameters(best_of=2, do_sample=True))
+
+ with pytest.raises(ValidationError):
+ Request(
+ inputs="test", parameters=Parameters(best_of=2, do_sample=True), stream=True
+ )
diff --git a/clients/python/text_generation/__init__.py b/clients/python/text_generation/__init__.py
new file mode 100644
index 00000000..a8e67071
--- /dev/null
+++ b/clients/python/text_generation/__init__.py
@@ -0,0 +1,23 @@
+# Copyright 2023 The HuggingFace Team. All rights reserved.
+#
+# Licensed under the Apache License, Version 2.0 (the "License");
+# you may not use this file except in compliance with the License.
+# You may obtain a copy of the License at
+#
+# http://www.apache.org/licenses/LICENSE-2.0
+#
+# Unless required by applicable law or agreed to in writing, software
+# distributed under the License is distributed on an "AS IS" BASIS,
+# WITHOUT WARRANTIES OR CONDITIONS OF ANY KIND, either express or implied.
+# See the License for the specific language governing permissions and
+# limitations under the License.
+
+__version__ = "0.6.0"
+
+DEPRECATION_WARNING = (
+ "`text_generation` clients are deprecated and will be removed in the near future. "
+ "Please use the `InferenceClient` from the `huggingface_hub` package instead."
+)
+
+from text_generation.client import Client, AsyncClient
+from text_generation.inference_api import InferenceAPIClient, InferenceAPIAsyncClient
diff --git a/clients/python/text_generation/client.py b/clients/python/text_generation/client.py
new file mode 100644
index 00000000..dbcb9cef
--- /dev/null
+++ b/clients/python/text_generation/client.py
@@ -0,0 +1,1043 @@
+import json
+import requests
+import warnings
+
+from aiohttp import ClientSession, ClientTimeout
+from pydantic import ValidationError
+from typing import Dict, Optional, List, AsyncIterator, Iterator, Union
+
+from text_generation import DEPRECATION_WARNING
+from text_generation.types import (
+ StreamResponse,
+ Response,
+ Request,
+ Parameters,
+ Grammar,
+ CompletionRequest,
+ Completion,
+ CompletionComplete,
+ ChatRequest,
+ ChatCompletionChunk,
+ ChatComplete,
+ Message,
+ Tool,
+)
+from text_generation.errors import parse_error
+
+# emit deprecation warnings
+warnings.simplefilter("always", DeprecationWarning)
+
+
+class Client:
+ """Client to make calls to a text-generation-inference instance
+
+ Example:
+
+ ```python
+ >>> from text_generation import Client
+
+ >>> client = Client("https://api-inference.huggingface.co/models/bigscience/bloomz")
+ >>> client.generate("Why is the sky blue?").generated_text
+ ' Rayleigh scattering'
+
+ >>> result = ""
+ >>> for response in client.generate_stream("Why is the sky blue?"):
+ >>> if not response.token.special:
+ >>> result += response.token.text
+ >>> result
+ ' Rayleigh scattering'
+ ```
+ """
+
+ def __init__(
+ self,
+ base_url: str,
+ headers: Optional[Dict[str, str]] = None,
+ cookies: Optional[Dict[str, str]] = None,
+ timeout: int = 10,
+ ):
+ """
+ Args:
+ base_url (`str`):
+ text-generation-inference instance base url
+ headers (`Optional[Dict[str, str]]`):
+ Additional headers
+ cookies (`Optional[Dict[str, str]]`):
+ Cookies to include in the requests
+ timeout (`int`):
+ Timeout in seconds
+ """
+ warnings.warn(DEPRECATION_WARNING, DeprecationWarning)
+ self.base_url = base_url
+ self.headers = headers
+ self.cookies = cookies
+ self.timeout = timeout
+
+ def completion(
+ self,
+ prompt: str,
+ frequency_penalty: Optional[float] = None,
+ max_tokens: Optional[int] = None,
+ repetition_penalty: Optional[float] = None,
+ seed: Optional[int] = None,
+ stream: bool = False,
+ temperature: Optional[float] = None,
+ top_p: Optional[float] = None,
+ stop: Optional[List[str]] = None,
+ lora_id: Optional[str] = None,
+ ):
+ """
+ Given a prompt, generate a response synchronously
+
+ Args:
+ prompt (`str`):
+ Prompt
+ frequency_penalty (`float`):
+ The parameter for frequency penalty. 0.0 means no penalty
+ Penalize new tokens based on their existing frequency in the text so far,
+ decreasing the model's likelihood to repeat the same line verbatim.
+ max_tokens (`int`):
+ Maximum number of generated tokens
+ repetition_penalty (`float`):
+ The parameter for frequency penalty. 0.0 means no penalty. See [this
+ paper](https://arxiv.org/pdf/1909.05858.pdf) for more details.
+ seed (`int`):
+ Random sampling seed
+ stream (`bool`):
+ Stream the response
+ temperature (`float`):
+ The value used to module the logits distribution.
+ top_p (`float`):
+ If set to < 1, only the smallest set of most probable tokens with probabilities that add up to `top_p` or
+ higher are kept for generation
+ stop (`List[str]`):
+ Stop generating tokens if a member of `stop` is generated
+ """
+ request = CompletionRequest(
+ model="tgi",
+ prompt=prompt,
+ frequency_penalty=frequency_penalty,
+ max_tokens=max_tokens,
+ repetition_penalty=repetition_penalty,
+ seed=seed,
+ stream=stream,
+ temperature=temperature,
+ top_p=top_p,
+ stop=stop,
+ lora_id=lora_id,
+ )
+ if not stream:
+ resp = requests.post(
+ f"{self.base_url}/v1/completions",
+ json=request.dict(),
+ headers=self.headers,
+ cookies=self.cookies,
+ timeout=self.timeout,
+ )
+ payload = resp.json()
+ if resp.status_code != 200:
+ raise parse_error(resp.status_code, payload)
+ return Completion(**payload)
+ else:
+ return self._completion_stream_response(request)
+
+ def _completion_stream_response(self, request):
+ resp = requests.post(
+ f"{self.base_url}/v1/completions",
+ json=request.dict(),
+ headers=self.headers,
+ cookies=self.cookies,
+ timeout=self.timeout,
+ stream=True,
+ )
+ # iterate and print stream
+ for byte_payload in resp.iter_lines():
+ if byte_payload == b"\n":
+ continue
+ payload = byte_payload.decode("utf-8")
+ if payload.startswith("data:"):
+ json_payload = json.loads(payload.lstrip("data:").rstrip("\n"))
+ try:
+ response = CompletionComplete(**json_payload)
+ yield response
+ except ValidationError:
+ raise parse_error(resp.status, json_payload)
+
+ def chat(
+ self,
+ messages: List[Message],
+ repetition_penalty: Optional[float] = None,
+ frequency_penalty: Optional[float] = None,
+ logit_bias: Optional[List[float]] = None,
+ logprobs: Optional[bool] = None,
+ top_logprobs: Optional[int] = None,
+ max_tokens: Optional[int] = None,
+ n: Optional[int] = None,
+ presence_penalty: Optional[float] = None,
+ stream: bool = False,
+ seed: Optional[int] = None,
+ temperature: Optional[float] = None,
+ top_p: Optional[float] = None,
+ tools: Optional[List[Tool]] = None,
+ tool_prompt: Optional[str] = None,
+ tool_choice: Optional[str] = None,
+ stop: Optional[List[str]] = None,
+ lora_id: Optional[str] = None,
+ ):
+ """
+ Given a list of messages, generate a response asynchronously
+
+ Args:
+ messages (`List[Message]`):
+ List of messages
+ repetition_penalty (`float`):
+ The parameter for repetition penalty. 0.0 means no penalty. See [this
+ paper](https://arxiv.org/pdf/1909.05858.pdf) for more details.
+ frequency_penalty (`float`):
+ The parameter for frequency penalty. 0.0 means no penalty
+ Penalize new tokens based on their existing frequency in the text so far,
+ decreasing the model's likelihood to repeat the same line verbatim.
+ logit_bias (`List[float]`):
+ Adjust the likelihood of specified tokens
+ logprobs (`bool`):
+ Include log probabilities in the response
+ top_logprobs (`int`):
+ Include the `n` most likely tokens at each step
+ max_tokens (`int`):
+ Maximum number of generated tokens
+ n (`int`):
+ Generate `n` completions
+ presence_penalty (`float`):
+ The parameter for presence penalty. 0.0 means no penalty. See [this
+ paper](https://arxiv.org/pdf/1909.05858.pdf) for more details.
+ stream (`bool`):
+ Stream the response
+ seed (`int`):
+ Random sampling seed
+ temperature (`float`):
+ The value used to module the logits distribution.
+ top_p (`float`):
+ If set to < 1, only the smallest set of most probable tokens with probabilities that add up to `top_p` or
+ higher are kept for generation
+ tools (`List[Tool]`):
+ List of tools to use
+ tool_prompt (`str`):
+ A prompt to be appended before the tools
+ tool_choice (`str`):
+ The tool to use
+ stop (`List[str]`):
+ Stop generating tokens if a member of `stop` is generated
+
+ """
+ request = ChatRequest(
+ model="tgi",
+ messages=messages,
+ repetition_penalty=repetition_penalty,
+ frequency_penalty=frequency_penalty,
+ logit_bias=logit_bias,
+ logprobs=logprobs,
+ top_logprobs=top_logprobs,
+ max_tokens=max_tokens,
+ n=n,
+ presence_penalty=presence_penalty,
+ stream=stream,
+ seed=seed,
+ temperature=temperature,
+ top_p=top_p,
+ tools=tools,
+ tool_prompt=tool_prompt,
+ tool_choice=tool_choice,
+ stop=stop,
+ lora_id=lora_id,
+ )
+ if not stream:
+ resp = requests.post(
+ f"{self.base_url}/v1/chat/completions",
+ json=request.dict(),
+ headers=self.headers,
+ cookies=self.cookies,
+ timeout=self.timeout,
+ )
+ payload = resp.json()
+ if resp.status_code != 200:
+ raise parse_error(resp.status_code, payload)
+ return ChatComplete(**payload)
+ else:
+ return self._chat_stream_response(request)
+
+ def _chat_stream_response(self, request):
+ resp = requests.post(
+ f"{self.base_url}/v1/chat/completions",
+ json=request.dict(),
+ headers=self.headers,
+ cookies=self.cookies,
+ timeout=self.timeout,
+ stream=True,
+ )
+ # iterate and print stream
+ for byte_payload in resp.iter_lines():
+ if byte_payload == b"\n":
+ continue
+ payload = byte_payload.decode("utf-8")
+ if payload.startswith("data:"):
+ json_payload = json.loads(payload.lstrip("data:").rstrip("\n"))
+ try:
+ response = ChatCompletionChunk(**json_payload)
+ yield response
+ except ValidationError:
+ raise parse_error(resp.status, json_payload)
+
+ def generate(
+ self,
+ prompt: str,
+ do_sample: bool = False,
+ max_new_tokens: int = 20,
+ best_of: Optional[int] = None,
+ repetition_penalty: Optional[float] = None,
+ frequency_penalty: Optional[float] = None,
+ return_full_text: bool = False,
+ seed: Optional[int] = None,
+ stop_sequences: Optional[List[str]] = None,
+ temperature: Optional[float] = None,
+ top_k: Optional[int] = None,
+ top_p: Optional[float] = None,
+ truncate: Optional[int] = None,
+ typical_p: Optional[float] = None,
+ watermark: bool = False,
+ decoder_input_details: bool = False,
+ top_n_tokens: Optional[int] = None,
+ grammar: Optional[Grammar] = None,
+ lora_id: Optional[str] = None,
+ ) -> Response:
+ """
+ Given a prompt, generate the following text
+
+ Args:
+ prompt (`str`):
+ Input text
+ do_sample (`bool`):
+ Activate logits sampling
+ max_new_tokens (`int`):
+ Maximum number of generated tokens
+ best_of (`int`):
+ Generate best_of sequences and return the one if the highest token logprobs
+ repetition_penalty (`float`):
+ The parameter for repetition penalty. 1.0 means no penalty. See [this
+ paper](https://arxiv.org/pdf/1909.05858.pdf) for more details.
+ frequency_penalty (`float`):
+ The parameter for frequency penalty. 1.0 means no penalty
+ Penalize new tokens based on their existing frequency in the text so far,
+ decreasing the model's likelihood to repeat the same line verbatim.
+ return_full_text (`bool`):
+ Whether to prepend the prompt to the generated text
+ seed (`int`):
+ Random sampling seed
+ stop_sequences (`List[str]`):
+ Stop generating tokens if a member of `stop_sequences` is generated
+ temperature (`float`):
+ The value used to module the logits distribution.
+ top_k (`int`):
+ The number of highest probability vocabulary tokens to keep for top-k-filtering.
+ top_p (`float`):
+ If set to < 1, only the smallest set of most probable tokens with probabilities that add up to `top_p` or
+ higher are kept for generation.
+ truncate (`int`):
+ Truncate inputs tokens to the given size
+ typical_p (`float`):
+ Typical Decoding mass
+ See [Typical Decoding for Natural Language Generation](https://arxiv.org/abs/2202.00666) for more information
+ watermark (`bool`):
+ Watermarking with [A Watermark for Large Language Models](https://arxiv.org/abs/2301.10226)
+ decoder_input_details (`bool`):
+ Return the decoder input token logprobs and ids
+ top_n_tokens (`int`):
+ Return the `n` most likely tokens at each step
+ grammar (`Grammar`):
+ Whether to use a grammar for the generation and the grammar to use. Grammars will constrain the generation
+ of the text to match a regular expression or JSON schema.
+
+ Returns:
+ Response: generated response
+ """
+ # Validate parameters
+ parameters = Parameters(
+ best_of=best_of,
+ details=True,
+ do_sample=do_sample,
+ max_new_tokens=max_new_tokens,
+ repetition_penalty=repetition_penalty,
+ frequency_penalty=frequency_penalty,
+ return_full_text=return_full_text,
+ seed=seed,
+ stop=stop_sequences if stop_sequences is not None else [],
+ temperature=temperature,
+ top_k=top_k,
+ top_p=top_p,
+ truncate=truncate,
+ typical_p=typical_p,
+ watermark=watermark,
+ decoder_input_details=decoder_input_details,
+ top_n_tokens=top_n_tokens,
+ grammar=grammar,
+ )
+ request = Request(
+ inputs=prompt, stream=False, parameters=parameters, lora_id=lora_id
+ )
+
+ resp = requests.post(
+ self.base_url,
+ json=request.dict(),
+ headers=self.headers,
+ cookies=self.cookies,
+ timeout=self.timeout,
+ )
+ if resp.status_code == 404:
+ raise parse_error(
+ resp.status_code,
+ {"error": "Service not found.", "errory_type": "generation"},
+ )
+ payload = resp.json()
+ if resp.status_code != 200:
+ raise parse_error(resp.status_code, payload)
+ return Response(**payload[0])
+
+ def generate_stream(
+ self,
+ prompt: str,
+ do_sample: bool = False,
+ max_new_tokens: int = 20,
+ repetition_penalty: Optional[float] = None,
+ frequency_penalty: Optional[float] = None,
+ return_full_text: bool = False,
+ seed: Optional[int] = None,
+ stop_sequences: Optional[List[str]] = None,
+ temperature: Optional[float] = None,
+ top_k: Optional[int] = None,
+ top_p: Optional[float] = None,
+ truncate: Optional[int] = None,
+ typical_p: Optional[float] = None,
+ watermark: bool = False,
+ top_n_tokens: Optional[int] = None,
+ grammar: Optional[Grammar] = None,
+ lora_id: Optional[str] = None,
+ ) -> Iterator[StreamResponse]:
+ """
+ Given a prompt, generate the following stream of tokens
+
+ Args:
+ prompt (`str`):
+ Input text
+ do_sample (`bool`):
+ Activate logits sampling
+ max_new_tokens (`int`):
+ Maximum number of generated tokens
+ repetition_penalty (`float`):
+ The parameter for repetition penalty. 1.0 means no penalty. See [this
+ paper](https://arxiv.org/pdf/1909.05858.pdf) for more details.
+ frequency_penalty (`float`):
+ The parameter for frequency penalty. 1.0 means no penalty
+ Penalize new tokens based on their existing frequency in the text so far,
+ decreasing the model's likelihood to repeat the same line verbatim.
+ return_full_text (`bool`):
+ Whether to prepend the prompt to the generated text
+ seed (`int`):
+ Random sampling seed
+ stop_sequences (`List[str]`):
+ Stop generating tokens if a member of `stop_sequences` is generated
+ temperature (`float`):
+ The value used to module the logits distribution.
+ top_k (`int`):
+ The number of highest probability vocabulary tokens to keep for top-k-filtering.
+ top_p (`float`):
+ If set to < 1, only the smallest set of most probable tokens with probabilities that add up to `top_p` or
+ higher are kept for generation.
+ truncate (`int`):
+ Truncate inputs tokens to the given size
+ typical_p (`float`):
+ Typical Decoding mass
+ See [Typical Decoding for Natural Language Generation](https://arxiv.org/abs/2202.00666) for more information
+ watermark (`bool`):
+ Watermarking with [A Watermark for Large Language Models](https://arxiv.org/abs/2301.10226)
+ top_n_tokens (`int`):
+ Return the `n` most likely tokens at each step
+ grammar (`Grammar`):
+ Whether to use a grammar for the generation and the grammar to use. Grammars will constrain the generation
+ of the text to match a regular expression or JSON schema.
+
+ Returns:
+ Iterator[StreamResponse]: stream of generated tokens
+ """
+ # Validate parameters
+ parameters = Parameters(
+ best_of=None,
+ details=True,
+ decoder_input_details=False,
+ do_sample=do_sample,
+ max_new_tokens=max_new_tokens,
+ repetition_penalty=repetition_penalty,
+ frequency_penalty=frequency_penalty,
+ return_full_text=return_full_text,
+ seed=seed,
+ stop=stop_sequences if stop_sequences is not None else [],
+ temperature=temperature,
+ top_k=top_k,
+ top_p=top_p,
+ truncate=truncate,
+ typical_p=typical_p,
+ watermark=watermark,
+ top_n_tokens=top_n_tokens,
+ grammar=grammar,
+ )
+ request = Request(
+ inputs=prompt, stream=True, parameters=parameters, lora_id=lora_id
+ )
+
+ resp = requests.post(
+ self.base_url,
+ json=request.dict(),
+ headers=self.headers,
+ cookies=self.cookies,
+ timeout=self.timeout,
+ stream=True,
+ )
+
+ if resp.status_code == 404:
+ raise parse_error(
+ resp.status_code,
+ {"error": "Service not found.", "errory_type": "generation"},
+ )
+ if resp.status_code != 200:
+ raise parse_error(resp.status_code, resp.json())
+
+ # Parse ServerSentEvents
+ for byte_payload in resp.iter_lines():
+ # Skip line
+ if byte_payload == b"\n":
+ continue
+
+ payload = byte_payload.decode("utf-8")
+
+ # Event data
+ if payload.startswith("data:"):
+ # Decode payload
+ json_payload = json.loads(payload.lstrip("data:").rstrip("/n"))
+ # Parse payload
+ try:
+ response = StreamResponse(**json_payload)
+ except ValidationError:
+ # If we failed to parse the payload, then it is an error payload
+ raise parse_error(resp.status_code, json_payload)
+ yield response
+
+ def download_lora_adapter(self, lora_id: str, hf_api_token: Optional[str] = None):
+ req = {}
+ req["lora_id"] = lora_id
+ req["hf_api_token"] = hf_api_token
+ resp = requests.post(
+ f"{self.base_url}/download_lora_adapter",
+ json=req,
+ headers=self.headers,
+ cookies=self.cookies,
+ timeout=self.timeout,
+ stream=True,
+ )
+
+ if resp.status_code != 200:
+ raise parse_error(resp.status_code, resp.json())
+ return resp
+
+
+class AsyncClient:
+ """Asynchronous Client to make calls to a text-generation-inference instance
+
+ Example:
+
+ ```python
+ >>> from text_generation import AsyncClient
+
+ >>> client = AsyncClient("https://api-inference.huggingface.co/models/bigscience/bloomz")
+ >>> response = await client.generate("Why is the sky blue?")
+ >>> response.generated_text
+ ' Rayleigh scattering'
+
+ >>> result = ""
+ >>> async for response in client.generate_stream("Why is the sky blue?"):
+ >>> if not response.token.special:
+ >>> result += response.token.text
+ >>> result
+ ' Rayleigh scattering'
+ ```
+ """
+
+ def __init__(
+ self,
+ base_url: str,
+ headers: Optional[Dict[str, str]] = None,
+ cookies: Optional[Dict[str, str]] = None,
+ timeout: int = 10,
+ ):
+ """
+ Args:
+ base_url (`str`):
+ text-generation-inference instance base url
+ headers (`Optional[Dict[str, str]]`):
+ Additional headers
+ cookies (`Optional[Dict[str, str]]`):
+ Cookies to include in the requests
+ timeout (`int`):
+ Timeout in seconds
+ """
+ warnings.warn(DEPRECATION_WARNING, DeprecationWarning)
+ self.base_url = base_url
+ self.headers = headers
+ self.cookies = cookies
+ self.timeout = ClientTimeout(timeout)
+
+ async def completion(
+ self,
+ prompt: str,
+ frequency_penalty: Optional[float] = None,
+ max_tokens: Optional[int] = None,
+ repetition_penalty: Optional[float] = None,
+ seed: Optional[int] = None,
+ stream: bool = False,
+ temperature: Optional[float] = None,
+ top_p: Optional[float] = None,
+ stop: Optional[List[str]] = None,
+ lora_id: Optional[str] = None,
+ ) -> Union[Completion, AsyncIterator[CompletionComplete]]:
+ """
+ Given a prompt, generate a response asynchronously
+
+ Args:
+ prompt (`str`):
+ Prompt
+ frequency_penalty (`float`):
+ The parameter for frequency penalty. 0.0 means no penalty
+ Penalize new tokens based on their existing frequency in the text so far,
+ decreasing the model's likelihood to repeat the same line verbatim.
+ max_tokens (`int`):
+ Maximum number of generated tokens
+ repetition_penalty (`float`):
+ The parameter for frequency penalty. 0.0 means no penalty. See [this
+ paper](https://arxiv.org/pdf/1909.05858.pdf) for more details.
+ seed (`int`):
+ Random sampling seed
+ stream (`bool`):
+ Stream the response
+ temperature (`float`):
+ The value used to module the logits distribution.
+ top_p (`float`):
+ If set to < 1, only the smallest set of most probable tokens with probabilities that add up to `top_p` or
+ higher are kept for generation
+ stop (`List[str]`):
+ Stop generating tokens if a member of `stop` is generated
+ """
+ request = CompletionRequest(
+ model="tgi",
+ prompt=prompt,
+ frequency_penalty=frequency_penalty,
+ max_tokens=max_tokens,
+ repetition_penalty=repetition_penalty,
+ seed=seed,
+ stream=stream,
+ temperature=temperature,
+ top_p=top_p,
+ stop=stop,
+ lora_id=lora_id,
+ )
+ if not stream:
+ return await self._completion_single_response(request)
+ else:
+ return self._completion_stream_response(request)
+
+ async def _completion_single_response(self, request):
+ async with ClientSession(
+ headers=self.headers, cookies=self.cookies, timeout=self.timeout
+ ) as session:
+ async with session.post(
+ f"{self.base_url}/v1/completions", json=request.dict()
+ ) as resp:
+ payload = await resp.json()
+ if resp.status != 200:
+ raise parse_error(resp.status, payload)
+ return Completion(**payload)
+
+ async def _completion_stream_response(self, request):
+ async with ClientSession(
+ headers=self.headers, cookies=self.cookies, timeout=self.timeout
+ ) as session:
+ async with session.post(
+ f"{self.base_url}/v1/completions", json=request.dict()
+ ) as resp:
+ async for byte_payload in resp.content:
+ if byte_payload == b"\n":
+ continue
+ payload = byte_payload.decode("utf-8")
+ if payload.startswith("data:"):
+ json_payload = json.loads(payload.lstrip("data:").rstrip("\n"))
+ try:
+ response = CompletionComplete(**json_payload)
+ yield response
+ except ValidationError:
+ raise parse_error(resp.status, json_payload)
+
+ async def chat(
+ self,
+ messages: List[Message],
+ repetition_penalty: Optional[float] = None,
+ frequency_penalty: Optional[float] = None,
+ logit_bias: Optional[List[float]] = None,
+ logprobs: Optional[bool] = None,
+ top_logprobs: Optional[int] = None,
+ max_tokens: Optional[int] = None,
+ n: Optional[int] = None,
+ presence_penalty: Optional[float] = None,
+ stream: bool = False,
+ seed: Optional[int] = None,
+ temperature: Optional[float] = None,
+ top_p: Optional[float] = None,
+ tools: Optional[List[Tool]] = None,
+ tool_prompt: Optional[str] = None,
+ tool_choice: Optional[str] = None,
+ stop: Optional[List[str]] = None,
+ lora_id: Optional[str] = None,
+ ) -> Union[ChatComplete, AsyncIterator[ChatCompletionChunk]]:
+ """
+ Given a list of messages, generate a response asynchronously
+
+ Args:
+ messages (`List[Message]`):
+ List of messages
+ repetition_penalty (`float`):
+ The parameter for frequency penalty. 0.0 means no penalty. See [this
+ paper](https://arxiv.org/pdf/1909.05858.pdf) for more details.
+ frequency_penalty (`float`):
+ The parameter for frequency penalty. 0.0 means no penalty
+ Penalize new tokens based on their existing frequency in the text so far,
+ decreasing the model's likelihood to repeat the same line verbatim.
+ logit_bias (`List[float]`):
+ Adjust the likelihood of specified tokens
+ logprobs (`bool`):
+ Include log probabilities in the response
+ top_logprobs (`int`):
+ Include the `n` most likely tokens at each step
+ max_tokens (`int`):
+ Maximum number of generated tokens
+ n (`int`):
+ Generate `n` completions
+ presence_penalty (`float`):
+ The parameter for presence penalty. 0.0 means no penalty. See [this
+ paper](https://arxiv.org/pdf/1909.05858.pdf) for more details.
+ stream (`bool`):
+ Stream the response
+ seed (`int`):
+ Random sampling seed
+ temperature (`float`):
+ The value used to module the logits distribution.
+ top_p (`float`):
+ If set to < 1, only the smallest set of most probable tokens with probabilities that add up to `top_p` or
+ higher are kept for generation
+ tools (`List[Tool]`):
+ List of tools to use
+ tool_prompt (`str`):
+ A prompt to be appended before the tools
+ tool_choice (`str`):
+ The tool to use
+ stop (`List[str]`):
+ Stop generating tokens if a member of `stop` is generated
+
+ """
+ request = ChatRequest(
+ model="tgi",
+ messages=messages,
+ repetition_penalty=repetition_penalty,
+ frequency_penalty=frequency_penalty,
+ logit_bias=logit_bias,
+ logprobs=logprobs,
+ top_logprobs=top_logprobs,
+ max_tokens=max_tokens,
+ n=n,
+ presence_penalty=presence_penalty,
+ stream=stream,
+ seed=seed,
+ temperature=temperature,
+ top_p=top_p,
+ tools=tools,
+ tool_prompt=tool_prompt,
+ tool_choice=tool_choice,
+ stop=stop,
+ lora_id=lora_id,
+ )
+ if not stream:
+ return await self._chat_single_response(request)
+ else:
+ return self._chat_stream_response(request)
+
+ async def _chat_single_response(self, request):
+ async with ClientSession(
+ headers=self.headers, cookies=self.cookies, timeout=self.timeout
+ ) as session:
+ async with session.post(
+ f"{self.base_url}/v1/chat/completions", json=request.dict()
+ ) as resp:
+ payload = await resp.json()
+ if resp.status != 200:
+ raise parse_error(resp.status, payload)
+ return ChatComplete(**payload)
+
+ async def _chat_stream_response(self, request):
+ async with ClientSession(
+ headers=self.headers, cookies=self.cookies, timeout=self.timeout
+ ) as session:
+ async with session.post(
+ f"{self.base_url}/v1/chat/completions", json=request.dict()
+ ) as resp:
+ async for byte_payload in resp.content:
+ if byte_payload == b"\n":
+ continue
+ payload = byte_payload.decode("utf-8")
+ if payload.startswith("data:"):
+ json_payload = json.loads(payload.lstrip("data:").rstrip("\n"))
+ try:
+ response = ChatCompletionChunk(**json_payload)
+ yield response
+ except ValidationError:
+ raise parse_error(resp.status, json_payload)
+
+ async def generate(
+ self,
+ prompt: str,
+ do_sample: bool = False,
+ max_new_tokens: int = 20,
+ best_of: Optional[int] = None,
+ repetition_penalty: Optional[float] = None,
+ frequency_penalty: Optional[float] = None,
+ return_full_text: bool = False,
+ seed: Optional[int] = None,
+ stop_sequences: Optional[List[str]] = None,
+ temperature: Optional[float] = None,
+ top_k: Optional[int] = None,
+ top_p: Optional[float] = None,
+ truncate: Optional[int] = None,
+ typical_p: Optional[float] = None,
+ watermark: bool = False,
+ decoder_input_details: bool = False,
+ top_n_tokens: Optional[int] = None,
+ grammar: Optional[Grammar] = None,
+ lora_id: Optional[str] = None,
+ ) -> Response:
+ """
+ Given a prompt, generate the following text asynchronously
+
+ Args:
+ prompt (`str`):
+ Input text
+ do_sample (`bool`):
+ Activate logits sampling
+ max_new_tokens (`int`):
+ Maximum number of generated tokens
+ best_of (`int`):
+ Generate best_of sequences and return the one if the highest token logprobs
+ repetition_penalty (`float`):
+ The parameter for repetition penalty. 1.0 means no penalty. See [this
+ paper](https://arxiv.org/pdf/1909.05858.pdf) for more details.
+ frequency_penalty (`float`):
+ The parameter for frequency penalty. 1.0 means no penalty
+ Penalize new tokens based on their existing frequency in the text so far,
+ decreasing the model's likelihood to repeat the same line verbatim.
+ return_full_text (`bool`):
+ Whether to prepend the prompt to the generated text
+ seed (`int`):
+ Random sampling seed
+ stop_sequences (`List[str]`):
+ Stop generating tokens if a member of `stop_sequences` is generated
+ temperature (`float`):
+ The value used to module the logits distribution.
+ top_k (`int`):
+ The number of highest probability vocabulary tokens to keep for top-k-filtering.
+ top_p (`float`):
+ If set to < 1, only the smallest set of most probable tokens with probabilities that add up to `top_p` or
+ higher are kept for generation.
+ truncate (`int`):
+ Truncate inputs tokens to the given size
+ typical_p (`float`):
+ Typical Decoding mass
+ See [Typical Decoding for Natural Language Generation](https://arxiv.org/abs/2202.00666) for more information
+ watermark (`bool`):
+ Watermarking with [A Watermark for Large Language Models](https://arxiv.org/abs/2301.10226)
+ decoder_input_details (`bool`):
+ Return the decoder input token logprobs and ids
+ top_n_tokens (`int`):
+ Return the `n` most likely tokens at each step
+ grammar (`Grammar`):
+ Whether to use a grammar for the generation and the grammar to use. Grammars will constrain the generation
+ of the text to match a regular expression or JSON schema.
+
+ Returns:
+ Response: generated response
+ """
+
+ # Validate parameters
+ parameters = Parameters(
+ best_of=best_of,
+ details=True,
+ decoder_input_details=decoder_input_details,
+ do_sample=do_sample,
+ max_new_tokens=max_new_tokens,
+ repetition_penalty=repetition_penalty,
+ frequency_penalty=frequency_penalty,
+ return_full_text=return_full_text,
+ seed=seed,
+ stop=stop_sequences if stop_sequences is not None else [],
+ temperature=temperature,
+ top_k=top_k,
+ top_p=top_p,
+ truncate=truncate,
+ typical_p=typical_p,
+ watermark=watermark,
+ top_n_tokens=top_n_tokens,
+ grammar=grammar,
+ )
+ request = Request(
+ inputs=prompt, stream=False, parameters=parameters, lora_id=lora_id
+ )
+
+ async with ClientSession(
+ headers=self.headers, cookies=self.cookies, timeout=self.timeout
+ ) as session:
+ async with session.post(self.base_url, json=request.dict()) as resp:
+ if resp.status == 404:
+ raise parse_error(
+ resp.status,
+ {"error": "Service not found.", "errory_type": "generation"},
+ )
+
+ payload = await resp.json()
+
+ if resp.status != 200:
+ raise parse_error(resp.status, payload)
+ return Response(**payload[0])
+
+ async def generate_stream(
+ self,
+ prompt: str,
+ do_sample: bool = False,
+ max_new_tokens: int = 20,
+ repetition_penalty: Optional[float] = None,
+ frequency_penalty: Optional[float] = None,
+ return_full_text: bool = False,
+ seed: Optional[int] = None,
+ stop_sequences: Optional[List[str]] = None,
+ temperature: Optional[float] = None,
+ top_k: Optional[int] = None,
+ top_p: Optional[float] = None,
+ truncate: Optional[int] = None,
+ typical_p: Optional[float] = None,
+ watermark: bool = False,
+ top_n_tokens: Optional[int] = None,
+ grammar: Optional[Grammar] = None,
+ lora_id: Optional[str] = None,
+ ) -> AsyncIterator[StreamResponse]:
+ """
+ Given a prompt, generate the following stream of tokens asynchronously
+
+ Args:
+ prompt (`str`):
+ Input text
+ do_sample (`bool`):
+ Activate logits sampling
+ max_new_tokens (`int`):
+ Maximum number of generated tokens
+ repetition_penalty (`float`):
+ The parameter for repetition penalty. 1.0 means no penalty. See [this
+ paper](https://arxiv.org/pdf/1909.05858.pdf) for more details.
+ frequency_penalty (`float`):
+ The parameter for frequency penalty. 1.0 means no penalty
+ Penalize new tokens based on their existing frequency in the text so far,
+ decreasing the model's likelihood to repeat the same line verbatim.
+ return_full_text (`bool`):
+ Whether to prepend the prompt to the generated text
+ seed (`int`):
+ Random sampling seed
+ stop_sequences (`List[str]`):
+ Stop generating tokens if a member of `stop_sequences` is generated
+ temperature (`float`):
+ The value used to module the logits distribution.
+ top_k (`int`):
+ The number of highest probability vocabulary tokens to keep for top-k-filtering.
+ top_p (`float`):
+ If set to < 1, only the smallest set of most probable tokens with probabilities that add up to `top_p` or
+ higher are kept for generation.
+ truncate (`int`):
+ Truncate inputs tokens to the given size
+ typical_p (`float`):
+ Typical Decoding mass
+ See [Typical Decoding for Natural Language Generation](https://arxiv.org/abs/2202.00666) for more information
+ watermark (`bool`):
+ Watermarking with [A Watermark for Large Language Models](https://arxiv.org/abs/2301.10226)
+ top_n_tokens (`int`):
+ Return the `n` most likely tokens at each step
+ grammar (`Grammar`):
+ Whether to use a grammar for the generation and the grammar to use. Grammars will constrain the generation
+ of the text to match a regular expression or JSON schema.
+
+ Returns:
+ AsyncIterator[StreamResponse]: stream of generated tokens
+ """
+ # Validate parameters
+ parameters = Parameters(
+ best_of=None,
+ details=True,
+ decoder_input_details=False,
+ do_sample=do_sample,
+ max_new_tokens=max_new_tokens,
+ repetition_penalty=repetition_penalty,
+ frequency_penalty=frequency_penalty,
+ return_full_text=return_full_text,
+ seed=seed,
+ stop=stop_sequences if stop_sequences is not None else [],
+ temperature=temperature,
+ top_k=top_k,
+ top_p=top_p,
+ truncate=truncate,
+ typical_p=typical_p,
+ watermark=watermark,
+ top_n_tokens=top_n_tokens,
+ grammar=grammar,
+ )
+ request = Request(
+ inputs=prompt, stream=True, parameters=parameters, lora_id=lora_id
+ )
+
+ async with ClientSession(
+ headers=self.headers, cookies=self.cookies, timeout=self.timeout
+ ) as session:
+ async with session.post(self.base_url, json=request.dict()) as resp:
+ if resp.status == 404:
+ raise parse_error(
+ resp.status,
+ {"error": "Service not found.", "errory_type": "generation"},
+ )
+ if resp.status != 200:
+ raise parse_error(resp.status, await resp.json())
+
+ # Parse ServerSentEvents
+ async for byte_payload in resp.content:
+ # Skip line
+ if byte_payload == b"\n":
+ continue
+
+ payload = byte_payload.decode("utf-8")
+
+ # Event data
+ if payload.startswith("data:"):
+ # Decode payload
+ json_payload = json.loads(payload.lstrip("data:").rstrip("/n"))
+ # Parse payload
+ try:
+ response = StreamResponse(**json_payload)
+ except ValidationError:
+ # If we failed to parse the payload, then it is an error payload
+ raise parse_error(resp.status, json_payload)
+ yield response
diff --git a/clients/python/text_generation/errors.py b/clients/python/text_generation/errors.py
new file mode 100644
index 00000000..dbf0b761
--- /dev/null
+++ b/clients/python/text_generation/errors.py
@@ -0,0 +1,106 @@
+from typing import Dict
+
+
+# Text Generation Inference Errors
+class ValidationError(Exception):
+ def __init__(self, message: str):
+ super().__init__(message)
+
+
+class GenerationError(Exception):
+ def __init__(self, message: str):
+ super().__init__(message)
+
+
+class OverloadedError(Exception):
+ def __init__(self, message: str):
+ super().__init__(message)
+
+
+class IncompleteGenerationError(Exception):
+ def __init__(self, message: str):
+ super().__init__(message)
+
+
+# API Inference Errors
+class BadRequestError(Exception):
+ def __init__(self, message: str):
+ super().__init__(message)
+
+
+class ShardNotReadyError(Exception):
+ def __init__(self, message: str):
+ super().__init__(message)
+
+
+class ShardTimeoutError(Exception):
+ def __init__(self, message: str):
+ super().__init__(message)
+
+
+class NotFoundError(Exception):
+ def __init__(self, message: str):
+ super().__init__(message)
+
+
+class RateLimitExceededError(Exception):
+ def __init__(self, message: str):
+ super().__init__(message)
+
+
+class NotSupportedError(Exception):
+ def __init__(self, model_id: str):
+ message = (
+ f"Model `{model_id}` is not available for inference with this client. \n"
+ "Use `huggingface_hub.inference_api.InferenceApi` instead."
+ )
+ super(NotSupportedError, self).__init__(message)
+
+
+# Unknown error
+class UnknownError(Exception):
+ def __init__(self, message: str):
+ super().__init__(message)
+
+
+def parse_error(status_code: int, payload: Dict[str, str]) -> Exception:
+ """
+ Parse error given an HTTP status code and a json payload
+
+ Args:
+ status_code (`int`):
+ HTTP status code
+ payload (`Dict[str, str]`):
+ Json payload
+
+ Returns:
+ Exception: parsed exception
+
+ """
+ # Try to parse a Text Generation Inference error
+ message = payload["error"]
+ if "error_type" in payload:
+ error_type = payload["error_type"]
+ if error_type == "generation":
+ return GenerationError(message)
+ if error_type == "incomplete_generation":
+ return IncompleteGenerationError(message)
+ if error_type == "overloaded":
+ return OverloadedError(message)
+ if error_type == "validation":
+ return ValidationError(message)
+
+ # Try to parse a APIInference error
+ if status_code == 400:
+ return BadRequestError(message)
+ if status_code == 403 or status_code == 424:
+ return ShardNotReadyError(message)
+ if status_code == 504:
+ return ShardTimeoutError(message)
+ if status_code == 404:
+ return NotFoundError(message)
+ if status_code == 429:
+ return RateLimitExceededError(message)
+
+ # Fallback to an unknown error
+ return UnknownError(message)
diff --git a/clients/python/text_generation/inference_api.py b/clients/python/text_generation/inference_api.py
new file mode 100644
index 00000000..93b0de8d
--- /dev/null
+++ b/clients/python/text_generation/inference_api.py
@@ -0,0 +1,168 @@
+import os
+import requests
+
+from typing import Dict, Optional, List
+from huggingface_hub.utils import build_hf_headers
+
+from text_generation import Client, AsyncClient, __version__
+from text_generation.types import DeployedModel
+from text_generation.errors import NotSupportedError, parse_error
+
+INFERENCE_ENDPOINT = os.environ.get(
+ "HF_INFERENCE_ENDPOINT", "https://api-inference.huggingface.co"
+)
+
+
+def deployed_models(headers: Optional[Dict] = None) -> List[DeployedModel]:
+ """
+ Get all currently deployed models with text-generation-inference-support
+
+ Returns:
+ List[DeployedModel]: list of all currently deployed models
+ """
+ resp = requests.get(
+ f"https://api-inference.huggingface.co/framework/text-generation-inference",
+ headers=headers,
+ timeout=5,
+ )
+
+ payload = resp.json()
+ if resp.status_code != 200:
+ raise parse_error(resp.status_code, payload)
+
+ models = [DeployedModel(**raw_deployed_model) for raw_deployed_model in payload]
+ return models
+
+
+def check_model_support(repo_id: str, headers: Optional[Dict] = None) -> bool:
+ """
+ Check if a given model is supported by text-generation-inference
+
+ Returns:
+ bool: whether the model is supported by this client
+ """
+ resp = requests.get(
+ f"https://api-inference.huggingface.co/status/{repo_id}",
+ headers=headers,
+ timeout=5,
+ )
+
+ payload = resp.json()
+ if resp.status_code != 200:
+ raise parse_error(resp.status_code, payload)
+
+ framework = payload["framework"]
+ supported = framework == "text-generation-inference"
+ return supported
+
+
+class InferenceAPIClient(Client):
+ """Client to make calls to the HuggingFace Inference API.
+
+ Only supports a subset of the available text-generation or text2text-generation models that are served using
+ text-generation-inference
+
+ Example:
+
+ ```python
+ >>> from text_generation import InferenceAPIClient
+
+ >>> client = InferenceAPIClient("bigscience/bloomz")
+ >>> client.generate("Why is the sky blue?").generated_text
+ ' Rayleigh scattering'
+
+ >>> result = ""
+ >>> for response in client.generate_stream("Why is the sky blue?"):
+ >>> if not response.token.special:
+ >>> result += response.token.text
+ >>> result
+ ' Rayleigh scattering'
+ ```
+ """
+
+ def __init__(self, repo_id: str, token: Optional[str] = None, timeout: int = 10):
+ """
+ Init headers and API information
+
+ Args:
+ repo_id (`str`):
+ Id of repository (e.g. `bigscience/bloom`).
+ token (`str`, `optional`):
+ The API token to use as HTTP bearer authorization. This is not
+ the authentication token. You can find the token in
+ https://huggingface.co/settings/token. Alternatively, you can
+ find both your organizations and personal API tokens using
+ `HfApi().whoami(token)`.
+ timeout (`int`):
+ Timeout in seconds
+ """
+
+ headers = build_hf_headers(
+ token=token, library_name="text-generation", library_version=__version__
+ )
+
+ # Text Generation Inference client only supports a subset of the available hub models
+ if not check_model_support(repo_id, headers):
+ raise NotSupportedError(repo_id)
+
+ base_url = f"{INFERENCE_ENDPOINT}/models/{repo_id}"
+
+ super(InferenceAPIClient, self).__init__(
+ base_url, headers=headers, timeout=timeout
+ )
+
+
+class InferenceAPIAsyncClient(AsyncClient):
+ """Aynschronous Client to make calls to the HuggingFace Inference API.
+
+ Only supports a subset of the available text-generation or text2text-generation models that are served using
+ text-generation-inference
+
+ Example:
+
+ ```python
+ >>> from text_generation import InferenceAPIAsyncClient
+
+ >>> client = InferenceAPIAsyncClient("bigscience/bloomz")
+ >>> response = await client.generate("Why is the sky blue?")
+ >>> response.generated_text
+ ' Rayleigh scattering'
+
+ >>> result = ""
+ >>> async for response in client.generate_stream("Why is the sky blue?"):
+ >>> if not response.token.special:
+ >>> result += response.token.text
+ >>> result
+ ' Rayleigh scattering'
+ ```
+ """
+
+ def __init__(self, repo_id: str, token: Optional[str] = None, timeout: int = 10):
+ """
+ Init headers and API information
+
+ Args:
+ repo_id (`str`):
+ Id of repository (e.g. `bigscience/bloom`).
+ token (`str`, `optional`):
+ The API token to use as HTTP bearer authorization. This is not
+ the authentication token. You can find the token in
+ https://huggingface.co/settings/token. Alternatively, you can
+ find both your organizations and personal API tokens using
+ `HfApi().whoami(token)`.
+ timeout (`int`):
+ Timeout in seconds
+ """
+ headers = build_hf_headers(
+ token=token, library_name="text-generation", library_version=__version__
+ )
+
+ # Text Generation Inference client only supports a subset of the available hub models
+ if not check_model_support(repo_id, headers):
+ raise NotSupportedError(repo_id)
+
+ base_url = f"{INFERENCE_ENDPOINT}/models/{repo_id}"
+
+ super(InferenceAPIAsyncClient, self).__init__(
+ base_url, headers=headers, timeout=timeout
+ )
diff --git a/clients/python/text_generation/types.py b/clients/python/text_generation/types.py
new file mode 100644
index 00000000..8195a08b
--- /dev/null
+++ b/clients/python/text_generation/types.py
@@ -0,0 +1,462 @@
+from enum import Enum
+from pydantic import BaseModel, field_validator
+from typing import Optional, List, Union, Any
+
+from text_generation.errors import ValidationError
+
+
+# enum for grammar type
+class GrammarType(str, Enum):
+ Json = "json"
+ Regex = "regex"
+
+
+# Grammar type and value
+class Grammar(BaseModel):
+ # Grammar type
+ type: GrammarType
+ # Grammar value
+ value: Union[str, dict]
+
+
+class ToolCall(BaseModel):
+ # Id of the tool call
+ id: int
+ # Type of the tool call
+ type: str
+ # Function details of the tool call
+ function: dict
+
+
+class Message(BaseModel):
+ # Role of the message sender
+ role: str
+ # Content of the message
+ content: Optional[str] = None
+ # Optional name of the message sender
+ name: Optional[str] = None
+ # Tool calls associated with the chat completion
+ tool_calls: Optional[Any] = None
+
+
+class Tool(BaseModel):
+ # Type of the tool
+ type: str
+ # Function details of the tool
+ function: dict
+
+
+class Function(BaseModel):
+ name: Optional[str]
+ arguments: str
+
+
+class ChoiceDeltaToolCall(BaseModel):
+ index: int
+ id: str
+ type: str
+ function: Function
+
+
+class ChoiceDelta(BaseModel):
+ role: str
+ content: Optional[str] = None
+ tool_calls: Optional[ChoiceDeltaToolCall]
+
+
+class Choice(BaseModel):
+ index: int
+ delta: ChoiceDelta
+ logprobs: Optional[dict] = None
+ finish_reason: Optional[str] = None
+
+
+class CompletionRequest(BaseModel):
+ # Model identifier
+ model: str
+ # Prompt
+ prompt: str
+ # The parameter for repetition penalty. 1.0 means no penalty.
+ # See [this paper](https://arxiv.org/pdf/1909.05858.pdf) for more details.
+ repetition_penalty: Optional[float] = None
+ # The parameter for frequency penalty. 1.0 means no penalty
+ # Penalize new tokens based on their existing frequency in the text so far,
+ # decreasing the model's likelihood to repeat the same line verbatim.
+ frequency_penalty: Optional[float] = None
+ # Maximum number of tokens to generate
+ max_tokens: Optional[int] = None
+ # Flag to indicate streaming response
+ stream: bool = False
+ # Random sampling seed
+ seed: Optional[int] = None
+ # Sampling temperature
+ temperature: Optional[float] = None
+ # Top-p value for nucleus sampling
+ top_p: Optional[float] = None
+ # Stop generating tokens if a member of `stop` is generated
+ stop: Optional[List[str]] = None
+ # LoRA id
+ lora_id: Optional[str] = None
+
+
+class CompletionComplete(BaseModel):
+ # Index of the chat completion
+ index: int
+ # Message associated with the chat completion
+ text: str
+ # Log probabilities for the chat completion
+ logprobs: Optional[Any]
+ # Reason for completion
+ finish_reason: str
+
+
+class Completion(BaseModel):
+ # Completion details
+ id: str
+ object: str
+ created: int
+ model: str
+ system_fingerprint: str
+ choices: List[CompletionComplete]
+
+
+class ChatRequest(BaseModel):
+ # Model identifier
+ model: str
+ # List of messages in the conversation
+ messages: List[Message]
+ # The parameter for repetition penalty. 1.0 means no penalty.
+ # See [this paper](https://arxiv.org/pdf/1909.05858.pdf) for more details.
+ repetition_penalty: Optional[float] = None
+ # The parameter for frequency penalty. 1.0 means no penalty
+ # Penalize new tokens based on their existing frequency in the text so far,
+ # decreasing the model's likelihood to repeat the same line verbatim.
+ frequency_penalty: Optional[float] = None
+ # Bias values for token selection
+ logit_bias: Optional[List[float]] = None
+ # Whether to return log probabilities
+ logprobs: Optional[bool] = None
+ # Number of most likely tokens to return at each position
+ top_logprobs: Optional[int] = None
+ # Maximum number of tokens to generate
+ max_tokens: Optional[int] = None
+ # Number of chat completion choices to generate
+ n: Optional[int] = None
+ # Penalty for presence of new tokens
+ presence_penalty: Optional[float] = None
+ # Flag to indicate streaming response
+ stream: bool = False
+ # Random sampling seed
+ seed: Optional[int] = None
+ # Sampling temperature
+ temperature: Optional[float] = None
+ # Top-p value for nucleus sampling
+ top_p: Optional[float] = None
+ # List of tools to be used
+ tools: Optional[List[Tool]] = None
+ # A prompt to be appended before the tools
+ tool_prompt: Optional[str] = None
+ # Choice of tool to be used
+ tool_choice: Optional[str] = None
+ # Stop generating tokens if a member of `stop` is generated
+ stop: Optional[List[str]] = None
+ # LoRA id
+ lora_id: Optional[str] = None
+
+
+class ChatCompletionComplete(BaseModel):
+ # Index of the chat completion
+ index: int
+ # Message associated with the chat completion
+ message: Message
+ # Log probabilities for the chat completion
+ logprobs: Optional[Any]
+ # Reason for completion
+ finish_reason: str
+ # Usage details of the chat completion
+ usage: Optional[Any] = None
+
+
+class ChatComplete(BaseModel):
+ # Chat completion details
+ id: str
+ object: str
+ created: int
+ model: str
+ system_fingerprint: str
+ choices: List[ChatCompletionComplete]
+ usage: Any
+
+
+class ChatCompletionChunk(BaseModel):
+ id: str
+ object: str
+ created: int
+ model: str
+ system_fingerprint: str
+ choices: List[Choice]
+
+
+class Parameters(BaseModel):
+ # Activate logits sampling
+ do_sample: bool = False
+ # Maximum number of generated tokens
+ max_new_tokens: int = 20
+ # The parameter for repetition penalty. 1.0 means no penalty.
+ # See [this paper](https://arxiv.org/pdf/1909.05858.pdf) for more details.
+ repetition_penalty: Optional[float] = None
+ # The parameter for frequency penalty. 1.0 means no penalty
+ # Penalize new tokens based on their existing frequency in the text so far,
+ # decreasing the model's likelihood to repeat the same line verbatim.
+ frequency_penalty: Optional[float] = None
+ # Whether to prepend the prompt to the generated text
+ return_full_text: bool = False
+ # Stop generating tokens if a member of `stop_sequences` is generated
+ stop: List[str] = []
+ # Random sampling seed
+ seed: Optional[int] = None
+ # The value used to module the logits distribution.
+ temperature: Optional[float] = None
+ # The number of highest probability vocabulary tokens to keep for top-k-filtering.
+ top_k: Optional[int] = None
+ # If set to < 1, only the smallest set of most probable tokens with probabilities that add up to `top_p` or
+ # higher are kept for generation.
+ top_p: Optional[float] = None
+ # truncate inputs tokens to the given size
+ truncate: Optional[int] = None
+ # Typical Decoding mass
+ # See [Typical Decoding for Natural Language Generation](https://arxiv.org/abs/2202.00666) for more information
+ typical_p: Optional[float] = None
+ # Generate best_of sequences and return the one if the highest token logprobs
+ best_of: Optional[int] = None
+ # Watermarking with [A Watermark for Large Language Models](https://arxiv.org/abs/2301.10226)
+ watermark: bool = False
+ # Get generation details
+ details: bool = False
+ # Get decoder input token logprobs and ids
+ decoder_input_details: bool = False
+ # Return the N most likely tokens at each step
+ top_n_tokens: Optional[int] = None
+ # grammar to use for generation
+ grammar: Optional[Grammar] = None
+
+ @field_validator("best_of")
+ def valid_best_of(cls, field_value, values):
+ if field_value is not None:
+ if field_value <= 0:
+ raise ValidationError("`best_of` must be strictly positive")
+ if field_value > 1 and values.data["seed"] is not None:
+ raise ValidationError("`seed` must not be set when `best_of` is > 1")
+ sampling = (
+ values.data["do_sample"]
+ | (values.data["temperature"] is not None)
+ | (values.data["top_k"] is not None)
+ | (values.data["top_p"] is not None)
+ | (values.data["typical_p"] is not None)
+ )
+ if field_value > 1 and not sampling:
+ raise ValidationError("you must use sampling when `best_of` is > 1")
+
+ return field_value
+
+ @field_validator("repetition_penalty")
+ def valid_repetition_penalty(cls, v):
+ if v is not None and v <= 0:
+ raise ValidationError("`repetition_penalty` must be strictly positive")
+ return v
+
+ @field_validator("frequency_penalty")
+ def valid_frequency_penalty(cls, v):
+ if v is not None and v <= 0:
+ raise ValidationError("`frequency_penalty` must be strictly positive")
+ return v
+
+ @field_validator("seed")
+ def valid_seed(cls, v):
+ if v is not None and v < 0:
+ raise ValidationError("`seed` must be positive")
+ return v
+
+ @field_validator("temperature")
+ def valid_temp(cls, v):
+ if v is not None and v <= 0:
+ raise ValidationError("`temperature` must be strictly positive")
+ return v
+
+ @field_validator("top_k")
+ def valid_top_k(cls, v):
+ if v is not None and v <= 0:
+ raise ValidationError("`top_k` must be strictly positive")
+ return v
+
+ @field_validator("top_p")
+ def valid_top_p(cls, v):
+ if v is not None and (v <= 0 or v >= 1.0):
+ raise ValidationError("`top_p` must be > 0.0 and < 1.0")
+ return v
+
+ @field_validator("truncate")
+ def valid_truncate(cls, v):
+ if v is not None and v <= 0:
+ raise ValidationError("`truncate` must be strictly positive")
+ return v
+
+ @field_validator("typical_p")
+ def valid_typical_p(cls, v):
+ if v is not None and (v <= 0 or v >= 1.0):
+ raise ValidationError("`typical_p` must be > 0.0 and < 1.0")
+ return v
+
+ @field_validator("top_n_tokens")
+ def valid_top_n_tokens(cls, v):
+ if v is not None and v <= 0:
+ raise ValidationError("`top_n_tokens` must be strictly positive")
+ return v
+
+ @field_validator("grammar")
+ def valid_grammar(cls, v):
+ if v is not None:
+ if v.type == GrammarType.Regex and not v.value:
+ raise ValidationError("`value` cannot be empty for `regex` grammar")
+ if v.type == GrammarType.Json and not v.value:
+ raise ValidationError("`value` cannot be empty for `json` grammar")
+ return v
+
+
+class Request(BaseModel):
+ # Prompt
+ inputs: str
+ # Generation parameters
+ parameters: Optional[Parameters] = None
+ # Whether to stream output tokens
+ stream: bool = False
+ # LoRA id
+ lora_id: Optional[str] = None
+
+ @field_validator("inputs")
+ def valid_input(cls, v):
+ if not v:
+ raise ValidationError("`inputs` cannot be empty")
+ return v
+
+ @field_validator("stream")
+ def valid_best_of_stream(cls, field_value, values):
+ parameters = values.data["parameters"]
+ if (
+ parameters is not None
+ and parameters.best_of is not None
+ and parameters.best_of > 1
+ and field_value
+ ):
+ raise ValidationError(
+ "`best_of` != 1 is not supported when `stream` == True"
+ )
+ return field_value
+
+
+# Decoder input tokens
+class InputToken(BaseModel):
+ # Token ID from the model tokenizer
+ id: int
+ # Token text
+ text: str
+ # Logprob
+ # Optional since the logprob of the first token cannot be computed
+ logprob: Optional[float] = None
+
+
+# Generated tokens
+class Token(BaseModel):
+ # Token ID from the model tokenizer
+ id: int
+ # Token text
+ text: str
+ # Logprob
+ logprob: Optional[float] = None
+ # Is the token a special token
+ # Can be used to ignore tokens when concatenating
+ special: bool
+
+
+# Generation finish reason
+class FinishReason(str, Enum):
+ # number of generated tokens == `max_new_tokens`
+ Length = "length"
+ # the model generated its end of sequence token
+ EndOfSequenceToken = "eos_token"
+ # the model generated a text included in `stop_sequences`
+ StopSequence = "stop_sequence"
+
+
+# Additional sequences when using the `best_of` parameter
+class BestOfSequence(BaseModel):
+ # Generated text
+ generated_text: str
+ # Generation finish reason
+ finish_reason: FinishReason
+ # Number of generated tokens
+ generated_tokens: int
+ # Sampling seed if sampling was activated
+ seed: Optional[int] = None
+ # Decoder input tokens, empty if decoder_input_details is False
+ prefill: List[InputToken]
+ # Generated tokens
+ tokens: List[Token]
+ # Most likely tokens
+ top_tokens: Optional[List[List[Token]]] = None
+
+
+# `generate` details
+class Details(BaseModel):
+ # Generation finish reason
+ finish_reason: FinishReason
+ # Number of generated tokens
+ generated_tokens: int
+ # Sampling seed if sampling was activated
+ seed: Optional[int] = None
+ # Decoder input tokens, empty if decoder_input_details is False
+ prefill: List[InputToken]
+ # Generated tokens
+ tokens: List[Token]
+ # Most likely tokens
+ top_tokens: Optional[List[List[Token]]] = None
+ # Additional sequences when using the `best_of` parameter
+ best_of_sequences: Optional[List[BestOfSequence]] = None
+
+
+# `generate` return value
+class Response(BaseModel):
+ # Generated text
+ generated_text: str
+ # Generation details
+ details: Details
+
+
+# `generate_stream` details
+class StreamDetails(BaseModel):
+ # Generation finish reason
+ finish_reason: FinishReason
+ # Number of generated tokens
+ generated_tokens: int
+ # Sampling seed if sampling was activated
+ seed: Optional[int] = None
+
+
+# `generate_stream` return value
+class StreamResponse(BaseModel):
+ # Generated token
+ token: Token
+ # Most likely tokens
+ top_tokens: Optional[List[Token]] = None
+ # Complete generated text
+ # Only available when the generation is finished
+ generated_text: Optional[str] = None
+ # Generation details
+ # Only available when the generation is finished
+ details: Optional[StreamDetails] = None
+
+
+# Inference API currently deployed model
+class DeployedModel(BaseModel):
+ model_id: str
+ sha: str
diff --git a/copy_back.py b/copy_back.py
deleted file mode 100644
index 520a9533..00000000
--- a/copy_back.py
+++ /dev/null
@@ -1,34 +0,0 @@
-from __future__ import print_function
-import filecmp
-import os.path
-import sys
-import shutil
-import os
-
-compare_file_data = True
-
-files = []
-
-def compare_dir_trees(dir1, dir2, compare_file_data, output):
- def compare_dirs(dir1, dir2):
- dirs_cmp = filecmp.dircmp(dir1, dir2)
- if compare_file_data and dirs_cmp.diff_files:
- for f in dirs_cmp.diff_files:
- files.append(dir1+'/' + f)
- for common_dir in dirs_cmp.common_dirs:
- new_dir1 = os.path.join(dir1, common_dir)
- new_dir2 = os.path.join(dir2, common_dir)
- compare_dirs(new_dir1, new_dir2)
- compare_dirs(dir1, dir2)
-
-dirs = ['server', 'clients', 'launcher', 'benchmark', 'integration-tests', 'load_tests', 'proto', 'router']
-for dir in dirs:
- if os.path.exists(dir):
- dir_a = 'build/' + dir
- dir_b = dir
- compare_dir_trees(dir_a, dir_b, compare_file_data, sys.stdout)
-
-for file in files:
- print(file + " -> " + file.replace('build/', ''))
- os.remove(file.replace('build/', ''))
- shutil.copy(file, file.replace('build/', ''))
diff --git a/docs/README.md b/docs/README.md
new file mode 100644
index 00000000..fb2ff198
--- /dev/null
+++ b/docs/README.md
@@ -0,0 +1,10 @@
+Documentation available at: https://huggingface.co/docs/text-generation-inference
+
+## Release
+
+When making a release, please update the latest version in the documentation with:
+```
+export OLD_VERSION="2\.0\.3"
+export NEW_VERSION="2\.0\.4"
+find . -name '*.md' -exec sed -i -e "s/$OLD_VERSION/$NEW_VERSION/g" {} \;
+```
diff --git a/docs/index.html b/docs/index.html
new file mode 100644
index 00000000..f582d3ce
--- /dev/null
+++ b/docs/index.html
@@ -0,0 +1,30 @@
+
+
+
+
+
+ Text Generation Inference API
+
+
+
+
+
+
diff --git a/docs/openapi.json b/docs/openapi.json
new file mode 100644
index 00000000..79c3b80f
--- /dev/null
+++ b/docs/openapi.json
@@ -0,0 +1,1883 @@
+{
+ "openapi": "3.0.3",
+ "info": {
+ "title": "Text Generation Inference",
+ "description": "Text Generation Webserver",
+ "contact": {
+ "name": "Olivier Dehaene"
+ },
+ "license": {
+ "name": "Apache 2.0",
+ "url": "https://www.apache.org/licenses/LICENSE-2.0"
+ },
+ "version": "2.0.1"
+ },
+ "paths": {
+ "/": {
+ "post": {
+ "tags": [
+ "Text Generation Inference"
+ ],
+ "summary": "Generate tokens if `stream == false` or a stream of token if `stream == true`",
+ "description": "Generate tokens if `stream == false` or a stream of token if `stream == true`",
+ "operationId": "compat_generate",
+ "requestBody": {
+ "content": {
+ "application/json": {
+ "schema": {
+ "$ref": "#/components/schemas/CompatGenerateRequest"
+ }
+ }
+ },
+ "required": true
+ },
+ "responses": {
+ "200": {
+ "description": "Generated Text",
+ "content": {
+ "application/json": {
+ "schema": {
+ "$ref": "#/components/schemas/GenerateResponse"
+ }
+ },
+ "text/event-stream": {
+ "schema": {
+ "$ref": "#/components/schemas/StreamResponse"
+ }
+ }
+ }
+ },
+ "422": {
+ "description": "Input validation error",
+ "content": {
+ "application/json": {
+ "schema": {
+ "$ref": "#/components/schemas/ErrorResponse"
+ },
+ "example": {
+ "error": "Input validation error"
+ }
+ }
+ }
+ },
+ "424": {
+ "description": "Generation Error",
+ "content": {
+ "application/json": {
+ "schema": {
+ "$ref": "#/components/schemas/ErrorResponse"
+ },
+ "example": {
+ "error": "Request failed during generation"
+ }
+ }
+ }
+ },
+ "429": {
+ "description": "Model is overloaded",
+ "content": {
+ "application/json": {
+ "schema": {
+ "$ref": "#/components/schemas/ErrorResponse"
+ },
+ "example": {
+ "error": "Model is overloaded"
+ }
+ }
+ }
+ },
+ "500": {
+ "description": "Incomplete generation",
+ "content": {
+ "application/json": {
+ "schema": {
+ "$ref": "#/components/schemas/ErrorResponse"
+ },
+ "example": {
+ "error": "Incomplete generation"
+ }
+ }
+ }
+ }
+ }
+ }
+ },
+ "/generate": {
+ "post": {
+ "tags": [
+ "Text Generation Inference"
+ ],
+ "summary": "Generate tokens",
+ "description": "Generate tokens",
+ "operationId": "generate",
+ "requestBody": {
+ "content": {
+ "application/json": {
+ "schema": {
+ "$ref": "#/components/schemas/GenerateRequest"
+ }
+ }
+ },
+ "required": true
+ },
+ "responses": {
+ "200": {
+ "description": "Generated Text",
+ "content": {
+ "application/json": {
+ "schema": {
+ "$ref": "#/components/schemas/GenerateResponse"
+ }
+ }
+ }
+ },
+ "422": {
+ "description": "Input validation error",
+ "content": {
+ "application/json": {
+ "schema": {
+ "$ref": "#/components/schemas/ErrorResponse"
+ },
+ "example": {
+ "error": "Input validation error"
+ }
+ }
+ }
+ },
+ "424": {
+ "description": "Generation Error",
+ "content": {
+ "application/json": {
+ "schema": {
+ "$ref": "#/components/schemas/ErrorResponse"
+ },
+ "example": {
+ "error": "Request failed during generation"
+ }
+ }
+ }
+ },
+ "429": {
+ "description": "Model is overloaded",
+ "content": {
+ "application/json": {
+ "schema": {
+ "$ref": "#/components/schemas/ErrorResponse"
+ },
+ "example": {
+ "error": "Model is overloaded"
+ }
+ }
+ }
+ },
+ "500": {
+ "description": "Incomplete generation",
+ "content": {
+ "application/json": {
+ "schema": {
+ "$ref": "#/components/schemas/ErrorResponse"
+ },
+ "example": {
+ "error": "Incomplete generation"
+ }
+ }
+ }
+ }
+ }
+ }
+ },
+ "/generate_stream": {
+ "post": {
+ "tags": [
+ "Text Generation Inference"
+ ],
+ "summary": "Generate a stream of token using Server-Sent Events",
+ "description": "Generate a stream of token using Server-Sent Events",
+ "operationId": "generate_stream",
+ "requestBody": {
+ "content": {
+ "application/json": {
+ "schema": {
+ "$ref": "#/components/schemas/GenerateRequest"
+ }
+ }
+ },
+ "required": true
+ },
+ "responses": {
+ "200": {
+ "description": "Generated Text",
+ "content": {
+ "text/event-stream": {
+ "schema": {
+ "$ref": "#/components/schemas/StreamResponse"
+ }
+ }
+ }
+ },
+ "422": {
+ "description": "Input validation error",
+ "content": {
+ "text/event-stream": {
+ "schema": {
+ "$ref": "#/components/schemas/ErrorResponse"
+ },
+ "example": {
+ "error": "Input validation error"
+ }
+ }
+ }
+ },
+ "424": {
+ "description": "Generation Error",
+ "content": {
+ "text/event-stream": {
+ "schema": {
+ "$ref": "#/components/schemas/ErrorResponse"
+ },
+ "example": {
+ "error": "Request failed during generation"
+ }
+ }
+ }
+ },
+ "429": {
+ "description": "Model is overloaded",
+ "content": {
+ "text/event-stream": {
+ "schema": {
+ "$ref": "#/components/schemas/ErrorResponse"
+ },
+ "example": {
+ "error": "Model is overloaded"
+ }
+ }
+ }
+ },
+ "500": {
+ "description": "Incomplete generation",
+ "content": {
+ "text/event-stream": {
+ "schema": {
+ "$ref": "#/components/schemas/ErrorResponse"
+ },
+ "example": {
+ "error": "Incomplete generation"
+ }
+ }
+ }
+ }
+ }
+ }
+ },
+ "/health": {
+ "get": {
+ "tags": [
+ "Text Generation Inference"
+ ],
+ "summary": "Health check method",
+ "description": "Health check method",
+ "operationId": "health",
+ "responses": {
+ "200": {
+ "description": "Everything is working fine"
+ },
+ "503": {
+ "description": "Text generation inference is down",
+ "content": {
+ "application/json": {
+ "schema": {
+ "$ref": "#/components/schemas/ErrorResponse"
+ },
+ "example": {
+ "error": "unhealthy",
+ "error_type": "healthcheck"
+ }
+ }
+ }
+ }
+ }
+ }
+ },
+ "/info": {
+ "get": {
+ "tags": [
+ "Text Generation Inference"
+ ],
+ "summary": "Text Generation Inference endpoint info",
+ "description": "Text Generation Inference endpoint info",
+ "operationId": "get_model_info",
+ "responses": {
+ "200": {
+ "description": "Served model info",
+ "content": {
+ "application/json": {
+ "schema": {
+ "$ref": "#/components/schemas/Info"
+ }
+ }
+ }
+ }
+ }
+ }
+ },
+ "/metrics": {
+ "get": {
+ "tags": [
+ "Text Generation Inference"
+ ],
+ "summary": "Prometheus metrics scrape endpoint",
+ "description": "Prometheus metrics scrape endpoint",
+ "operationId": "metrics",
+ "responses": {
+ "200": {
+ "description": "Prometheus Metrics",
+ "content": {
+ "text/plain": {
+ "schema": {
+ "type": "string"
+ }
+ }
+ }
+ }
+ }
+ }
+ },
+ "/tokenize": {
+ "post": {
+ "tags": [
+ "Text Generation Inference"
+ ],
+ "summary": "Tokenize inputs",
+ "description": "Tokenize inputs",
+ "operationId": "tokenize",
+ "requestBody": {
+ "content": {
+ "application/json": {
+ "schema": {
+ "$ref": "#/components/schemas/GenerateRequest"
+ }
+ }
+ },
+ "required": true
+ },
+ "responses": {
+ "200": {
+ "description": "Tokenized ids",
+ "content": {
+ "application/json": {
+ "schema": {
+ "$ref": "#/components/schemas/TokenizeResponse"
+ }
+ }
+ }
+ },
+ "404": {
+ "description": "No tokenizer found",
+ "content": {
+ "application/json": {
+ "schema": {
+ "$ref": "#/components/schemas/ErrorResponse"
+ },
+ "example": {
+ "error": "No fast tokenizer available"
+ }
+ }
+ }
+ }
+ }
+ }
+ },
+ "/v1/chat/completions": {
+ "post": {
+ "tags": [
+ "Text Generation Inference"
+ ],
+ "summary": "Generate tokens",
+ "description": "Generate tokens",
+ "operationId": "chat_completions",
+ "requestBody": {
+ "content": {
+ "application/json": {
+ "schema": {
+ "$ref": "#/components/schemas/ChatRequest"
+ }
+ }
+ },
+ "required": true
+ },
+ "responses": {
+ "200": {
+ "description": "Generated Chat Completion",
+ "content": {
+ "application/json": {
+ "schema": {
+ "$ref": "#/components/schemas/ChatCompletion"
+ }
+ },
+ "text/event-stream": {
+ "schema": {
+ "$ref": "#/components/schemas/ChatCompletionChunk"
+ }
+ }
+ }
+ },
+ "422": {
+ "description": "Input validation error",
+ "content": {
+ "application/json": {
+ "schema": {
+ "$ref": "#/components/schemas/ErrorResponse"
+ },
+ "example": {
+ "error": "Input validation error"
+ }
+ }
+ }
+ },
+ "424": {
+ "description": "Generation Error",
+ "content": {
+ "application/json": {
+ "schema": {
+ "$ref": "#/components/schemas/ErrorResponse"
+ },
+ "example": {
+ "error": "Request failed during generation"
+ }
+ }
+ }
+ },
+ "429": {
+ "description": "Model is overloaded",
+ "content": {
+ "application/json": {
+ "schema": {
+ "$ref": "#/components/schemas/ErrorResponse"
+ },
+ "example": {
+ "error": "Model is overloaded"
+ }
+ }
+ }
+ },
+ "500": {
+ "description": "Incomplete generation",
+ "content": {
+ "application/json": {
+ "schema": {
+ "$ref": "#/components/schemas/ErrorResponse"
+ },
+ "example": {
+ "error": "Incomplete generation"
+ }
+ }
+ }
+ }
+ }
+ }
+ },
+ "/v1/completions": {
+ "post": {
+ "tags": [
+ "Text Generation Inference"
+ ],
+ "summary": "Generate tokens",
+ "description": "Generate tokens",
+ "operationId": "completions",
+ "requestBody": {
+ "content": {
+ "application/json": {
+ "schema": {
+ "$ref": "#/components/schemas/CompletionRequest"
+ }
+ }
+ },
+ "required": true
+ },
+ "responses": {
+ "200": {
+ "description": "Generated Chat Completion",
+ "content": {
+ "application/json": {
+ "schema": {
+ "$ref": "#/components/schemas/Completion"
+ }
+ },
+ "text/event-stream": {
+ "schema": {
+ "$ref": "#/components/schemas/CompletionCompleteChunk"
+ }
+ }
+ }
+ },
+ "422": {
+ "description": "Input validation error",
+ "content": {
+ "application/json": {
+ "schema": {
+ "$ref": "#/components/schemas/ErrorResponse"
+ },
+ "example": {
+ "error": "Input validation error"
+ }
+ }
+ }
+ },
+ "424": {
+ "description": "Generation Error",
+ "content": {
+ "application/json": {
+ "schema": {
+ "$ref": "#/components/schemas/ErrorResponse"
+ },
+ "example": {
+ "error": "Request failed during generation"
+ }
+ }
+ }
+ },
+ "429": {
+ "description": "Model is overloaded",
+ "content": {
+ "application/json": {
+ "schema": {
+ "$ref": "#/components/schemas/ErrorResponse"
+ },
+ "example": {
+ "error": "Model is overloaded"
+ }
+ }
+ }
+ },
+ "500": {
+ "description": "Incomplete generation",
+ "content": {
+ "application/json": {
+ "schema": {
+ "$ref": "#/components/schemas/ErrorResponse"
+ },
+ "example": {
+ "error": "Incomplete generation"
+ }
+ }
+ }
+ }
+ }
+ }
+ }
+ },
+ "components": {
+ "schemas": {
+ "BestOfSequence": {
+ "type": "object",
+ "required": [
+ "generated_text",
+ "finish_reason",
+ "generated_tokens",
+ "prefill",
+ "tokens"
+ ],
+ "properties": {
+ "finish_reason": {
+ "$ref": "#/components/schemas/FinishReason"
+ },
+ "generated_text": {
+ "type": "string",
+ "example": "test"
+ },
+ "generated_tokens": {
+ "type": "integer",
+ "format": "int32",
+ "example": 1,
+ "minimum": 0
+ },
+ "prefill": {
+ "type": "array",
+ "items": {
+ "$ref": "#/components/schemas/PrefillToken"
+ }
+ },
+ "seed": {
+ "type": "integer",
+ "format": "int64",
+ "example": 42,
+ "nullable": true,
+ "minimum": 0
+ },
+ "tokens": {
+ "type": "array",
+ "items": {
+ "$ref": "#/components/schemas/Token"
+ }
+ },
+ "top_tokens": {
+ "type": "array",
+ "items": {
+ "type": "array",
+ "items": {
+ "$ref": "#/components/schemas/Token"
+ }
+ }
+ }
+ }
+ },
+ "ChatCompletion": {
+ "type": "object",
+ "required": [
+ "id",
+ "object",
+ "created",
+ "model",
+ "system_fingerprint",
+ "choices",
+ "usage"
+ ],
+ "properties": {
+ "choices": {
+ "type": "array",
+ "items": {
+ "$ref": "#/components/schemas/ChatCompletionComplete"
+ }
+ },
+ "created": {
+ "type": "integer",
+ "format": "int64",
+ "example": "1706270835",
+ "minimum": 0
+ },
+ "id": {
+ "type": "string"
+ },
+ "model": {
+ "type": "string",
+ "example": "mistralai/Mistral-7B-Instruct-v0.2"
+ },
+ "object": {
+ "type": "string"
+ },
+ "system_fingerprint": {
+ "type": "string"
+ },
+ "usage": {
+ "$ref": "#/components/schemas/Usage"
+ }
+ }
+ },
+ "ChatCompletionChoice": {
+ "type": "object",
+ "required": [
+ "index",
+ "delta"
+ ],
+ "properties": {
+ "delta": {
+ "$ref": "#/components/schemas/ChatCompletionDelta"
+ },
+ "finish_reason": {
+ "type": "string",
+ "nullable": true
+ },
+ "index": {
+ "type": "integer",
+ "format": "int32",
+ "minimum": 0
+ },
+ "logprobs": {
+ "allOf": [
+ {
+ "$ref": "#/components/schemas/ChatCompletionLogprobs"
+ }
+ ],
+ "nullable": true
+ }
+ }
+ },
+ "ChatCompletionChunk": {
+ "type": "object",
+ "required": [
+ "id",
+ "object",
+ "created",
+ "model",
+ "system_fingerprint",
+ "choices"
+ ],
+ "properties": {
+ "choices": {
+ "type": "array",
+ "items": {
+ "$ref": "#/components/schemas/ChatCompletionChoice"
+ }
+ },
+ "created": {
+ "type": "integer",
+ "format": "int64",
+ "example": "1706270978",
+ "minimum": 0
+ },
+ "id": {
+ "type": "string"
+ },
+ "model": {
+ "type": "string",
+ "example": "mistralai/Mistral-7B-Instruct-v0.2"
+ },
+ "object": {
+ "type": "string"
+ },
+ "system_fingerprint": {
+ "type": "string"
+ }
+ }
+ },
+ "ChatCompletionComplete": {
+ "type": "object",
+ "required": [
+ "index",
+ "message",
+ "finish_reason"
+ ],
+ "properties": {
+ "finish_reason": {
+ "type": "string"
+ },
+ "index": {
+ "type": "integer",
+ "format": "int32",
+ "minimum": 0
+ },
+ "logprobs": {
+ "allOf": [
+ {
+ "$ref": "#/components/schemas/ChatCompletionLogprobs"
+ }
+ ],
+ "nullable": true
+ },
+ "message": {
+ "$ref": "#/components/schemas/Message"
+ }
+ }
+ },
+ "ChatCompletionDelta": {
+ "type": "object",
+ "required": [
+ "role"
+ ],
+ "properties": {
+ "content": {
+ "type": "string",
+ "example": "What is Deep Learning?",
+ "nullable": true
+ },
+ "role": {
+ "type": "string",
+ "example": "user"
+ },
+ "tool_calls": {
+ "allOf": [
+ {
+ "$ref": "#/components/schemas/DeltaToolCall"
+ }
+ ],
+ "nullable": true
+ }
+ }
+ },
+ "ChatCompletionLogprob": {
+ "type": "object",
+ "required": [
+ "token",
+ "logprob",
+ "top_logprobs"
+ ],
+ "properties": {
+ "logprob": {
+ "type": "number",
+ "format": "float"
+ },
+ "token": {
+ "type": "string"
+ },
+ "top_logprobs": {
+ "type": "array",
+ "items": {
+ "$ref": "#/components/schemas/ChatCompletionTopLogprob"
+ }
+ }
+ }
+ },
+ "ChatCompletionLogprobs": {
+ "type": "object",
+ "required": [
+ "content"
+ ],
+ "properties": {
+ "content": {
+ "type": "array",
+ "items": {
+ "$ref": "#/components/schemas/ChatCompletionLogprob"
+ }
+ }
+ }
+ },
+ "ChatCompletionTopLogprob": {
+ "type": "object",
+ "required": [
+ "token",
+ "logprob"
+ ],
+ "properties": {
+ "logprob": {
+ "type": "number",
+ "format": "float"
+ },
+ "token": {
+ "type": "string"
+ }
+ }
+ },
+ "ChatRequest": {
+ "type": "object",
+ "required": [
+ "model",
+ "messages"
+ ],
+ "properties": {
+ "frequency_penalty": {
+ "type": "number",
+ "format": "float",
+ "description": "Number between -2.0 and 2.0. Positive values penalize new tokens based on their existing frequency in the text so far,\ndecreasing the model's likelihood to repeat the same line verbatim.",
+ "example": "1.0",
+ "nullable": true
+ },
+ "logit_bias": {
+ "type": "array",
+ "items": {
+ "type": "number",
+ "format": "float"
+ },
+ "description": "UNUSED\nModify the likelihood of specified tokens appearing in the completion. Accepts a JSON object that maps tokens\n(specified by their token ID in the tokenizer) to an associated bias value from -100 to 100. Mathematically,\nthe bias is added to the logits generated by the model prior to sampling. The exact effect will vary per model,\nbut values between -1 and 1 should decrease or increase likelihood of selection; values like -100 or 100 should\nresult in a ban or exclusive selection of the relevant token.",
+ "nullable": true
+ },
+ "logprobs": {
+ "type": "boolean",
+ "description": "Whether to return log probabilities of the output tokens or not. If true, returns the log probabilities of each\noutput token returned in the content of message.",
+ "example": "false",
+ "nullable": true
+ },
+ "max_tokens": {
+ "type": "integer",
+ "format": "int32",
+ "description": "The maximum number of tokens that can be generated in the chat completion.",
+ "example": "32",
+ "nullable": true,
+ "minimum": 0
+ },
+ "messages": {
+ "type": "array",
+ "items": {
+ "$ref": "#/components/schemas/Message"
+ },
+ "description": "A list of messages comprising the conversation so far.",
+ "example": "[{\"role\": \"user\", \"content\": \"What is Deep Learning?\"}]"
+ },
+ "model": {
+ "type": "string",
+ "description": "[UNUSED] ID of the model to use. See the model endpoint compatibility table for details on which models work with the Chat API.",
+ "example": "mistralai/Mistral-7B-Instruct-v0.2"
+ },
+ "n": {
+ "type": "integer",
+ "format": "int32",
+ "description": "UNUSED\nHow many chat completion choices to generate for each input message. Note that you will be charged based on the\nnumber of generated tokens across all of the choices. Keep n as 1 to minimize costs.",
+ "example": "2",
+ "nullable": true,
+ "minimum": 0
+ },
+ "presence_penalty": {
+ "type": "number",
+ "format": "float",
+ "description": "Number between -2.0 and 2.0. Positive values penalize new tokens based on whether they appear in the text so far,\nincreasing the model's likelihood to talk about new topics",
+ "example": 0.1,
+ "nullable": true
+ },
+ "seed": {
+ "type": "integer",
+ "format": "int64",
+ "example": 42,
+ "nullable": true,
+ "minimum": 0
+ },
+ "stop": {
+ "type": "array",
+ "items": {
+ "type": "string"
+ },
+ "description": "Up to 4 sequences where the API will stop generating further tokens.",
+ "example": "null",
+ "nullable": true
+ },
+ "stream": {
+ "type": "boolean"
+ },
+ "temperature": {
+ "type": "number",
+ "format": "float",
+ "description": "What sampling temperature to use, between 0 and 2. Higher values like 0.8 will make the output more random, while\nlower values like 0.2 will make it more focused and deterministic.\n\nWe generally recommend altering this or `top_p` but not both.",
+ "example": 1.0,
+ "nullable": true
+ },
+ "tool_choice": {
+ "allOf": [
+ {
+ "$ref": "#/components/schemas/ToolType"
+ }
+ ],
+ "nullable": true
+ },
+ "tool_prompt": {
+ "type": "string",
+ "description": "A prompt to be appended before the tools",
+ "example": "\"You will be presented with a JSON schema representing a set of tools.\nIf the user request lacks of sufficient information to make a precise tool selection: Do not invent any tool's properties, instead notify with an error message.\n\nJSON Schema:\n\"",
+ "nullable": true
+ },
+ "tools": {
+ "type": "array",
+ "items": {
+ "$ref": "#/components/schemas/Tool"
+ },
+ "description": "A list of tools the model may call. Currently, only functions are supported as a tool. Use this to provide a list of\nfunctions the model may generate JSON inputs for.",
+ "example": "null",
+ "nullable": true
+ },
+ "top_logprobs": {
+ "type": "integer",
+ "format": "int32",
+ "description": "An integer between 0 and 5 specifying the number of most likely tokens to return at each token position, each with\nan associated log probability. logprobs must be set to true if this parameter is used.",
+ "example": "5",
+ "nullable": true,
+ "minimum": 0
+ },
+ "top_p": {
+ "type": "number",
+ "format": "float",
+ "description": "An alternative to sampling with temperature, called nucleus sampling, where the model considers the results of the\ntokens with top_p probability mass. So 0.1 means only the tokens comprising the top 10% probability mass are considered.",
+ "example": 0.95,
+ "nullable": true
+ }
+ }
+ },
+ "CompatGenerateRequest": {
+ "type": "object",
+ "required": [
+ "inputs"
+ ],
+ "properties": {
+ "inputs": {
+ "type": "string",
+ "example": "My name is Olivier and I"
+ },
+ "parameters": {
+ "$ref": "#/components/schemas/GenerateParameters"
+ },
+ "stream": {
+ "type": "boolean",
+ "default": "false"
+ }
+ }
+ },
+ "CompletionComplete": {
+ "type": "object",
+ "required": [
+ "index",
+ "text",
+ "finish_reason"
+ ],
+ "properties": {
+ "finish_reason": {
+ "type": "string"
+ },
+ "index": {
+ "type": "integer",
+ "format": "int32",
+ "minimum": 0
+ },
+ "logprobs": {
+ "type": "array",
+ "items": {
+ "type": "number",
+ "format": "float"
+ },
+ "nullable": true
+ },
+ "text": {
+ "type": "string"
+ }
+ }
+ },
+ "CompletionCompleteChunk": {
+ "type": "object",
+ "required": [
+ "id",
+ "object",
+ "created",
+ "choices",
+ "model",
+ "system_fingerprint"
+ ],
+ "properties": {
+ "choices": {
+ "type": "array",
+ "items": {
+ "$ref": "#/components/schemas/CompletionComplete"
+ }
+ },
+ "created": {
+ "type": "integer",
+ "format": "int64",
+ "minimum": 0
+ },
+ "id": {
+ "type": "string"
+ },
+ "model": {
+ "type": "string"
+ },
+ "object": {
+ "type": "string"
+ },
+ "system_fingerprint": {
+ "type": "string"
+ }
+ }
+ },
+ "CompletionRequest": {
+ "type": "object",
+ "required": [
+ "model",
+ "prompt"
+ ],
+ "properties": {
+ "frequency_penalty": {
+ "type": "number",
+ "format": "float",
+ "description": "Number between -2.0 and 2.0. Positive values penalize new tokens based on their existing frequency in the text so far,\ndecreasing the model's likelihood to repeat the same line verbatim.",
+ "example": "1.0",
+ "nullable": true
+ },
+ "max_tokens": {
+ "type": "integer",
+ "format": "int32",
+ "description": "The maximum number of tokens that can be generated in the chat completion.",
+ "default": "32",
+ "nullable": true,
+ "minimum": 0
+ },
+ "model": {
+ "type": "string",
+ "description": "UNUSED\nID of the model to use. See the model endpoint compatibility table for details on which models work with the Chat API.",
+ "example": "mistralai/Mistral-7B-Instruct-v0.2"
+ },
+ "prompt": {
+ "type": "array",
+ "items": {
+ "type": "string"
+ },
+ "description": "The prompt to generate completions for.",
+ "example": "What is Deep Learning?"
+ },
+ "repetition_penalty": {
+ "type": "number",
+ "format": "float",
+ "nullable": true
+ },
+ "seed": {
+ "type": "integer",
+ "format": "int64",
+ "example": 42,
+ "nullable": true,
+ "minimum": 0
+ },
+ "stream": {
+ "type": "boolean"
+ },
+ "suffix": {
+ "type": "string",
+ "description": "The text to append to the prompt. This is useful for completing sentences or generating a paragraph of text.\nplease see the completion_template field in the model's tokenizer_config.json file for completion template.",
+ "nullable": true
+ },
+ "temperature": {
+ "type": "number",
+ "format": "float",
+ "description": "What sampling temperature to use, between 0 and 2. Higher values like 0.8 will make the output more random, while\nlower values like 0.2 will make it more focused and deterministic. We generally recommend altering this or `top_p` but not both.",
+ "example": 1.0,
+ "nullable": true
+ },
+ "top_p": {
+ "type": "number",
+ "format": "float",
+ "description": "An alternative to sampling with temperature, called nucleus sampling, where the model considers the results of the\ntokens with top_p probability mass. So 0.1 means only the tokens comprising the top 10% probability mass are considered.",
+ "example": 0.95,
+ "nullable": true
+ },
+ "stop": {
+ "type": "array",
+ "items": {
+ "type": "string"
+ },
+ "description": "Up to 4 sequences where the API will stop generating further tokens.",
+ "example": "null",
+ "nullable": true
+ }
+ }
+ },
+ "DeltaToolCall": {
+ "type": "object",
+ "required": [
+ "index",
+ "id",
+ "type",
+ "function"
+ ],
+ "properties": {
+ "function": {
+ "$ref": "#/components/schemas/Function"
+ },
+ "id": {
+ "type": "string"
+ },
+ "index": {
+ "type": "integer",
+ "format": "int32",
+ "minimum": 0
+ },
+ "type": {
+ "type": "string"
+ }
+ }
+ },
+ "Details": {
+ "type": "object",
+ "required": [
+ "finish_reason",
+ "generated_tokens",
+ "prefill",
+ "tokens"
+ ],
+ "properties": {
+ "best_of_sequences": {
+ "type": "array",
+ "items": {
+ "$ref": "#/components/schemas/BestOfSequence"
+ },
+ "nullable": true
+ },
+ "finish_reason": {
+ "$ref": "#/components/schemas/FinishReason"
+ },
+ "generated_tokens": {
+ "type": "integer",
+ "format": "int32",
+ "example": 1,
+ "minimum": 0
+ },
+ "prefill": {
+ "type": "array",
+ "items": {
+ "$ref": "#/components/schemas/PrefillToken"
+ }
+ },
+ "seed": {
+ "type": "integer",
+ "format": "int64",
+ "example": 42,
+ "nullable": true,
+ "minimum": 0
+ },
+ "tokens": {
+ "type": "array",
+ "items": {
+ "$ref": "#/components/schemas/Token"
+ }
+ },
+ "top_tokens": {
+ "type": "array",
+ "items": {
+ "type": "array",
+ "items": {
+ "$ref": "#/components/schemas/Token"
+ }
+ }
+ }
+ }
+ },
+ "ErrorResponse": {
+ "type": "object",
+ "required": [
+ "error",
+ "error_type"
+ ],
+ "properties": {
+ "error": {
+ "type": "string"
+ },
+ "error_type": {
+ "type": "string"
+ }
+ }
+ },
+ "FinishReason": {
+ "type": "string",
+ "enum": [
+ "length",
+ "eos_token",
+ "stop_sequence"
+ ],
+ "example": "Length"
+ },
+ "Function": {
+ "type": "object",
+ "required": [
+ "arguments"
+ ],
+ "properties": {
+ "arguments": {
+ "type": "string"
+ },
+ "name": {
+ "type": "string",
+ "nullable": true
+ }
+ }
+ },
+ "FunctionDefinition": {
+ "type": "object",
+ "required": [
+ "name",
+ "arguments"
+ ],
+ "properties": {
+ "arguments": {},
+ "description": {
+ "type": "string",
+ "nullable": true
+ },
+ "name": {
+ "type": "string"
+ }
+ }
+ },
+ "GenerateParameters": {
+ "type": "object",
+ "properties": {
+ "best_of": {
+ "type": "integer",
+ "default": "null",
+ "example": 1,
+ "nullable": true,
+ "minimum": 0,
+ "exclusiveMinimum": 0
+ },
+ "decoder_input_details": {
+ "type": "boolean",
+ "default": "false"
+ },
+ "details": {
+ "type": "boolean",
+ "default": "true"
+ },
+ "do_sample": {
+ "type": "boolean",
+ "default": "false",
+ "example": true
+ },
+ "frequency_penalty": {
+ "type": "number",
+ "format": "float",
+ "default": "null",
+ "example": 0.1,
+ "nullable": true,
+ "exclusiveMinimum": -2
+ },
+ "grammar": {
+ "allOf": [
+ {
+ "$ref": "#/components/schemas/GrammarType"
+ }
+ ],
+ "default": "null",
+ "nullable": true
+ },
+ "max_new_tokens": {
+ "type": "integer",
+ "format": "int32",
+ "default": "100",
+ "example": "20",
+ "nullable": true,
+ "minimum": 0
+ },
+ "repetition_penalty": {
+ "type": "number",
+ "format": "float",
+ "default": "null",
+ "example": 1.03,
+ "nullable": true,
+ "exclusiveMinimum": 0
+ },
+ "return_full_text": {
+ "type": "boolean",
+ "default": "null",
+ "example": false,
+ "nullable": true
+ },
+ "seed": {
+ "type": "integer",
+ "format": "int64",
+ "default": "null",
+ "example": "null",
+ "nullable": true,
+ "minimum": 0,
+ "exclusiveMinimum": 0
+ },
+ "stop": {
+ "type": "array",
+ "items": {
+ "type": "string"
+ },
+ "example": [
+ "photographer"
+ ],
+ "maxItems": 4
+ },
+ "temperature": {
+ "type": "number",
+ "format": "float",
+ "default": "null",
+ "example": 0.5,
+ "nullable": true,
+ "exclusiveMinimum": 0
+ },
+ "top_k": {
+ "type": "integer",
+ "format": "int32",
+ "default": "null",
+ "example": 10,
+ "nullable": true,
+ "exclusiveMinimum": 0
+ },
+ "top_n_tokens": {
+ "type": "integer",
+ "format": "int32",
+ "default": "null",
+ "example": 5,
+ "nullable": true,
+ "minimum": 0,
+ "exclusiveMinimum": 0
+ },
+ "top_p": {
+ "type": "number",
+ "format": "float",
+ "default": "null",
+ "example": 0.95,
+ "nullable": true,
+ "maximum": 1,
+ "exclusiveMinimum": 0
+ },
+ "truncate": {
+ "type": "integer",
+ "default": "null",
+ "example": "null",
+ "nullable": true,
+ "minimum": 0
+ },
+ "typical_p": {
+ "type": "number",
+ "format": "float",
+ "default": "null",
+ "example": 0.95,
+ "nullable": true,
+ "maximum": 1,
+ "exclusiveMinimum": 0
+ },
+ "watermark": {
+ "type": "boolean",
+ "default": "false",
+ "example": true
+ }
+ }
+ },
+ "GenerateRequest": {
+ "type": "object",
+ "required": [
+ "inputs"
+ ],
+ "properties": {
+ "inputs": {
+ "type": "string",
+ "example": "My name is Olivier and I"
+ },
+ "parameters": {
+ "$ref": "#/components/schemas/GenerateParameters"
+ }
+ }
+ },
+ "GenerateResponse": {
+ "type": "object",
+ "required": [
+ "generated_text"
+ ],
+ "properties": {
+ "details": {
+ "allOf": [
+ {
+ "$ref": "#/components/schemas/Details"
+ }
+ ],
+ "nullable": true
+ },
+ "generated_text": {
+ "type": "string",
+ "example": "test"
+ }
+ }
+ },
+ "GrammarType": {
+ "oneOf": [
+ {
+ "type": "object",
+ "required": [
+ "type",
+ "value"
+ ],
+ "properties": {
+ "type": {
+ "type": "string",
+ "enum": [
+ "json"
+ ]
+ },
+ "value": {
+ "description": "A string that represents a [JSON Schema](https://json-schema.org/).\n\nJSON Schema is a declarative language that allows to annotate JSON documents\nwith types and descriptions."
+ }
+ }
+ },
+ {
+ "type": "object",
+ "required": [
+ "type",
+ "value"
+ ],
+ "properties": {
+ "type": {
+ "type": "string",
+ "enum": [
+ "regex"
+ ]
+ },
+ "value": {
+ "type": "string"
+ }
+ }
+ }
+ ],
+ "discriminator": {
+ "propertyName": "type"
+ }
+ },
+ "Info": {
+ "type": "object",
+ "required": [
+ "model_id",
+ "model_dtype",
+ "model_device_type",
+ "max_concurrent_requests",
+ "max_best_of",
+ "max_stop_sequences",
+ "max_input_length",
+ "max_total_tokens",
+ "waiting_served_ratio",
+ "max_batch_total_tokens",
+ "max_waiting_tokens",
+ "validation_workers",
+ "max_client_batch_size",
+ "version"
+ ],
+ "properties": {
+ "docker_label": {
+ "type": "string",
+ "example": "null",
+ "nullable": true
+ },
+ "max_batch_size": {
+ "type": "integer",
+ "example": "null",
+ "nullable": true,
+ "minimum": 0
+ },
+ "max_batch_total_tokens": {
+ "type": "integer",
+ "format": "int32",
+ "example": "32000",
+ "minimum": 0
+ },
+ "max_best_of": {
+ "type": "integer",
+ "example": "2",
+ "minimum": 0
+ },
+ "max_client_batch_size": {
+ "type": "integer",
+ "example": "32",
+ "minimum": 0
+ },
+ "max_concurrent_requests": {
+ "type": "integer",
+ "description": "Router Parameters",
+ "example": "128",
+ "minimum": 0
+ },
+ "max_input_length": {
+ "type": "integer",
+ "example": "1024",
+ "minimum": 0
+ },
+ "max_stop_sequences": {
+ "type": "integer",
+ "example": "4",
+ "minimum": 0
+ },
+ "max_total_tokens": {
+ "type": "integer",
+ "example": "2048",
+ "minimum": 0
+ },
+ "max_waiting_tokens": {
+ "type": "integer",
+ "example": "20",
+ "minimum": 0
+ },
+ "model_device_type": {
+ "type": "string",
+ "example": "cuda"
+ },
+ "model_dtype": {
+ "type": "string",
+ "example": "torch.float16"
+ },
+ "model_id": {
+ "type": "string",
+ "description": "Model info",
+ "example": "bigscience/blomm-560m"
+ },
+ "model_pipeline_tag": {
+ "type": "string",
+ "example": "text-generation",
+ "nullable": true
+ },
+ "model_sha": {
+ "type": "string",
+ "example": "e985a63cdc139290c5f700ff1929f0b5942cced2",
+ "nullable": true
+ },
+ "sha": {
+ "type": "string",
+ "example": "null",
+ "nullable": true
+ },
+ "validation_workers": {
+ "type": "integer",
+ "example": "2",
+ "minimum": 0
+ },
+ "version": {
+ "type": "string",
+ "description": "Router Info",
+ "example": "0.5.0"
+ },
+ "waiting_served_ratio": {
+ "type": "number",
+ "format": "float",
+ "example": "1.2"
+ }
+ }
+ },
+ "Message": {
+ "type": "object",
+ "required": [
+ "role"
+ ],
+ "properties": {
+ "content": {
+ "type": "string",
+ "example": "My name is David and I",
+ "nullable": true
+ },
+ "name": {
+ "type": "string",
+ "example": "\"David\"",
+ "nullable": true
+ },
+ "role": {
+ "type": "string",
+ "example": "user"
+ },
+ "tool_calls": {
+ "type": "array",
+ "items": {
+ "$ref": "#/components/schemas/ToolCall"
+ },
+ "nullable": true
+ }
+ }
+ },
+ "PrefillToken": {
+ "type": "object",
+ "required": [
+ "id",
+ "text",
+ "logprob"
+ ],
+ "properties": {
+ "id": {
+ "type": "integer",
+ "format": "int32",
+ "example": 0,
+ "minimum": 0
+ },
+ "logprob": {
+ "type": "number",
+ "format": "float",
+ "example": -0.34,
+ "nullable": true
+ },
+ "text": {
+ "type": "string",
+ "example": "test"
+ }
+ }
+ },
+ "SimpleToken": {
+ "type": "object",
+ "required": [
+ "id",
+ "text",
+ "start",
+ "stop"
+ ],
+ "properties": {
+ "id": {
+ "type": "integer",
+ "format": "int32",
+ "example": 0,
+ "minimum": 0
+ },
+ "start": {
+ "type": "integer",
+ "example": 0,
+ "minimum": 0
+ },
+ "stop": {
+ "type": "integer",
+ "example": 2,
+ "minimum": 0
+ },
+ "text": {
+ "type": "string",
+ "example": "test"
+ }
+ }
+ },
+ "StreamDetails": {
+ "type": "object",
+ "required": [
+ "finish_reason",
+ "generated_tokens"
+ ],
+ "properties": {
+ "finish_reason": {
+ "$ref": "#/components/schemas/FinishReason"
+ },
+ "generated_tokens": {
+ "type": "integer",
+ "format": "int32",
+ "example": 1,
+ "minimum": 0
+ },
+ "seed": {
+ "type": "integer",
+ "format": "int64",
+ "example": 42,
+ "nullable": true,
+ "minimum": 0
+ }
+ }
+ },
+ "StreamResponse": {
+ "type": "object",
+ "required": [
+ "index",
+ "token"
+ ],
+ "properties": {
+ "details": {
+ "allOf": [
+ {
+ "$ref": "#/components/schemas/StreamDetails"
+ }
+ ],
+ "default": "null",
+ "nullable": true
+ },
+ "generated_text": {
+ "type": "string",
+ "default": "null",
+ "example": "test",
+ "nullable": true
+ },
+ "index": {
+ "type": "integer",
+ "format": "int32",
+ "minimum": 0
+ },
+ "token": {
+ "$ref": "#/components/schemas/Token"
+ },
+ "top_tokens": {
+ "type": "array",
+ "items": {
+ "$ref": "#/components/schemas/Token"
+ }
+ }
+ }
+ },
+ "Token": {
+ "type": "object",
+ "required": [
+ "id",
+ "text",
+ "logprob",
+ "special"
+ ],
+ "properties": {
+ "id": {
+ "type": "integer",
+ "format": "int32",
+ "example": 0,
+ "minimum": 0
+ },
+ "logprob": {
+ "type": "number",
+ "format": "float",
+ "example": -0.34,
+ "nullable": true
+ },
+ "special": {
+ "type": "boolean",
+ "example": "false"
+ },
+ "text": {
+ "type": "string",
+ "example": "test"
+ }
+ }
+ },
+ "TokenizeResponse": {
+ "type": "array",
+ "items": {
+ "$ref": "#/components/schemas/SimpleToken"
+ }
+ },
+ "Tool": {
+ "type": "object",
+ "required": [
+ "type",
+ "function"
+ ],
+ "properties": {
+ "function": {
+ "$ref": "#/components/schemas/FunctionDefinition"
+ },
+ "type": {
+ "type": "string",
+ "example": "function"
+ }
+ }
+ },
+ "ToolCall": {
+ "type": "object",
+ "required": [
+ "id",
+ "type",
+ "function"
+ ],
+ "properties": {
+ "function": {
+ "$ref": "#/components/schemas/FunctionDefinition"
+ },
+ "id": {
+ "type": "integer",
+ "format": "int32",
+ "minimum": 0
+ },
+ "type": {
+ "type": "string"
+ }
+ }
+ },
+ "ToolType": {
+ "oneOf": [
+ {
+ "type": "object",
+ "required": [
+ "FunctionName"
+ ],
+ "properties": {
+ "FunctionName": {
+ "type": "string"
+ }
+ }
+ },
+ {
+ "type": "string",
+ "enum": [
+ "OneOf"
+ ]
+ }
+ ]
+ },
+ "Usage": {
+ "type": "object",
+ "required": [
+ "prompt_tokens",
+ "completion_tokens",
+ "total_tokens"
+ ],
+ "properties": {
+ "completion_tokens": {
+ "type": "integer",
+ "format": "int32",
+ "minimum": 0
+ },
+ "prompt_tokens": {
+ "type": "integer",
+ "format": "int32",
+ "minimum": 0
+ },
+ "total_tokens": {
+ "type": "integer",
+ "format": "int32",
+ "minimum": 0
+ }
+ }
+ }
+ }
+ },
+ "tags": [
+ {
+ "name": "Text Generation Inference",
+ "description": "Hugging Face Text Generation Inference API"
+ }
+ ]
+}
diff --git a/docs/source/_toctree.yml b/docs/source/_toctree.yml
new file mode 100644
index 00000000..a7351a33
--- /dev/null
+++ b/docs/source/_toctree.yml
@@ -0,0 +1,63 @@
+- sections:
+ - local: index
+ title: Text Generation Inference
+ - local: quicktour
+ title: Quick Tour
+ - local: installation_nvidia
+ title: Using TGI with Nvidia GPUs
+ - local: installation_amd
+ title: Using TGI with AMD GPUs
+ - local: installation_gaudi
+ title: Using TGI with Intel Gaudi
+ - local: installation_inferentia
+ title: Using TGI with AWS Inferentia
+ - local: installation
+ title: Installation from source
+ - local: supported_models
+ title: Supported Models and Hardware
+ - local: messages_api
+ title: Messages API
+ title: Getting started
+- sections:
+ - local: basic_tutorials/consuming_tgi
+ title: Consuming TGI
+ - local: basic_tutorials/preparing_model
+ title: Preparing Model for Serving
+ - local: basic_tutorials/gated_model_access
+ title: Serving Private & Gated Models
+ - local: basic_tutorials/using_cli
+ title: Using TGI CLI
+ - local: basic_tutorials/launcher
+ title: All TGI CLI options
+ - local: basic_tutorials/non_core_models
+ title: Non-core Model Serving
+ - local: basic_tutorials/safety
+ title: Safety
+ - local: basic_tutorials/using_guidance
+ title: Using Guidance, JSON, tools
+ - local: basic_tutorials/visual_language_models
+ title: Visual Language Models
+ - local: basic_tutorials/monitoring
+ title: Monitoring TGI with Prometheus and Grafana
+ - local: basic_tutorials/train_medusa
+ title: Train Medusa
+ title: Tutorials
+- sections:
+ - local: conceptual/streaming
+ title: Streaming
+ - local: conceptual/quantization
+ title: Quantization
+ - local: conceptual/tensor_parallelism
+ title: Tensor Parallelism
+ - local: conceptual/paged_attention
+ title: PagedAttention
+ - local: conceptual/safetensors
+ title: Safetensors
+ - local: conceptual/flash_attention
+ title: Flash Attention
+ - local: conceptual/speculation
+ title: Speculation (Medusa, ngram)
+ - local: conceptual/guidance
+ title: How Guidance Works (via outlines)
+
+ title: Conceptual Guides
diff --git a/docs/source/basic_tutorials/consuming_tgi.md b/docs/source/basic_tutorials/consuming_tgi.md
new file mode 100644
index 00000000..4829ec7c
--- /dev/null
+++ b/docs/source/basic_tutorials/consuming_tgi.md
@@ -0,0 +1,155 @@
+# Consuming Text Generation Inference
+
+There are many ways you can consume Text Generation Inference server in your applications. After launching, you can use the `/generate` route and make a `POST` request to get results from the server. You can also use the `/generate_stream` route if you want TGI to return a stream of tokens. You can make the requests using the tool of your preference, such as curl, Python or TypeScrpt. For a final end-to-end experience, we also open-sourced ChatUI, a chat interface for open-source models.
+
+## curl
+
+After the launch, you can query the model using either the `/generate` or `/generate_stream` routes:
+
+```bash
+curl 127.0.0.1:8080/generate \
+ -X POST \
+ -d '{"inputs":"What is Deep Learning?","parameters":{"max_new_tokens":20}}' \
+ -H 'Content-Type: application/json'
+```
+
+
+## Inference Client
+
+[`huggingface-hub`](https://huggingface.co/docs/huggingface_hub/main/en/index) is a Python library to interact with the Hugging Face Hub, including its endpoints. It provides a nice high-level class, [`~huggingface_hub.InferenceClient`], which makes it easy to make calls to a TGI endpoint. `InferenceClient` also takes care of parameter validation and provides a simple to-use interface.
+You can simply install `huggingface-hub` package with pip.
+
+```bash
+pip install huggingface-hub
+```
+
+Once you start the TGI server, instantiate `InferenceClient()` with the URL to the endpoint serving the model. You can then call `text_generation()` to hit the endpoint through Python.
+
+```python
+from huggingface_hub import InferenceClient
+
+client = InferenceClient(model="http://127.0.0.1:8080")
+client.text_generation(prompt="Write a code for snake game")
+```
+
+You can do streaming with `InferenceClient` by passing `stream=True`. Streaming will return tokens as they are being generated in the server. To use streaming, you can do as follows:
+
+```python
+for token in client.text_generation("How do you make cheese?", max_new_tokens=12, stream=True):
+ print(token)
+```
+
+Another parameter you can use with TGI backend is `details`. You can get more details on generation (tokens, probabilities, etc.) by setting `details` to `True`. When it's specified, TGI will return a `TextGenerationResponse` or `TextGenerationStreamResponse` rather than a string or stream.
+
+```python
+output = client.text_generation(prompt="Meaning of life is", details=True)
+print(output)
+
+# TextGenerationResponse(generated_text=' a complex concept that is not always clear to the individual. It is a concept that is not always', details=Details(finish_reason=, generated_tokens=20, seed=None, prefill=[], tokens=[Token(id=267, text=' a', logprob=-2.0723474, special=False), Token(id=11235, text=' complex', logprob=-3.1272552, special=False), Token(id=17908, text=' concept', logprob=-1.3632495, special=False),..))
+```
+
+You can see how to stream below.
+
+```python
+output = client.text_generation(prompt="Meaning of life is", stream=True, details=True)
+print(next(iter(output)))
+
+# TextGenerationStreamResponse(token=Token(id=267, text=' a', logprob=-2.0723474, special=False), generated_text=None, details=None)
+```
+
+You can check out the details of the function [here](https://huggingface.co/docs/huggingface_hub/main/en/package_reference/inference_client#huggingface_hub.InferenceClient.text_generation). There is also an async version of the client, `AsyncInferenceClient`, based on `asyncio` and `aiohttp`. You can find docs for it [here](https://huggingface.co/docs/huggingface_hub/package_reference/inference_client#huggingface_hub.AsyncInferenceClient)
+
+
+## ChatUI
+
+ChatUI is an open-source interface built for LLM serving. It offers many customization options, such as web search with SERP API and more. ChatUI can automatically consume the TGI server and even provides an option to switch between different TGI endpoints. You can try it out at [Hugging Chat](https://huggingface.co/chat/), or use the [ChatUI Docker Space](https://huggingface.co/new-space?template=huggingchat/chat-ui-template) to deploy your own Hugging Chat to Spaces.
+
+To serve both ChatUI and TGI in same environment, simply add your own endpoints to the `MODELS` variable in `.env.local` file inside the `chat-ui` repository. Provide the endpoints pointing to where TGI is served.
+
+```
+{
+// rest of the model config here
+"endpoints": [{"url": "https://HOST:PORT/generate_stream"}]
+}
+```
+
+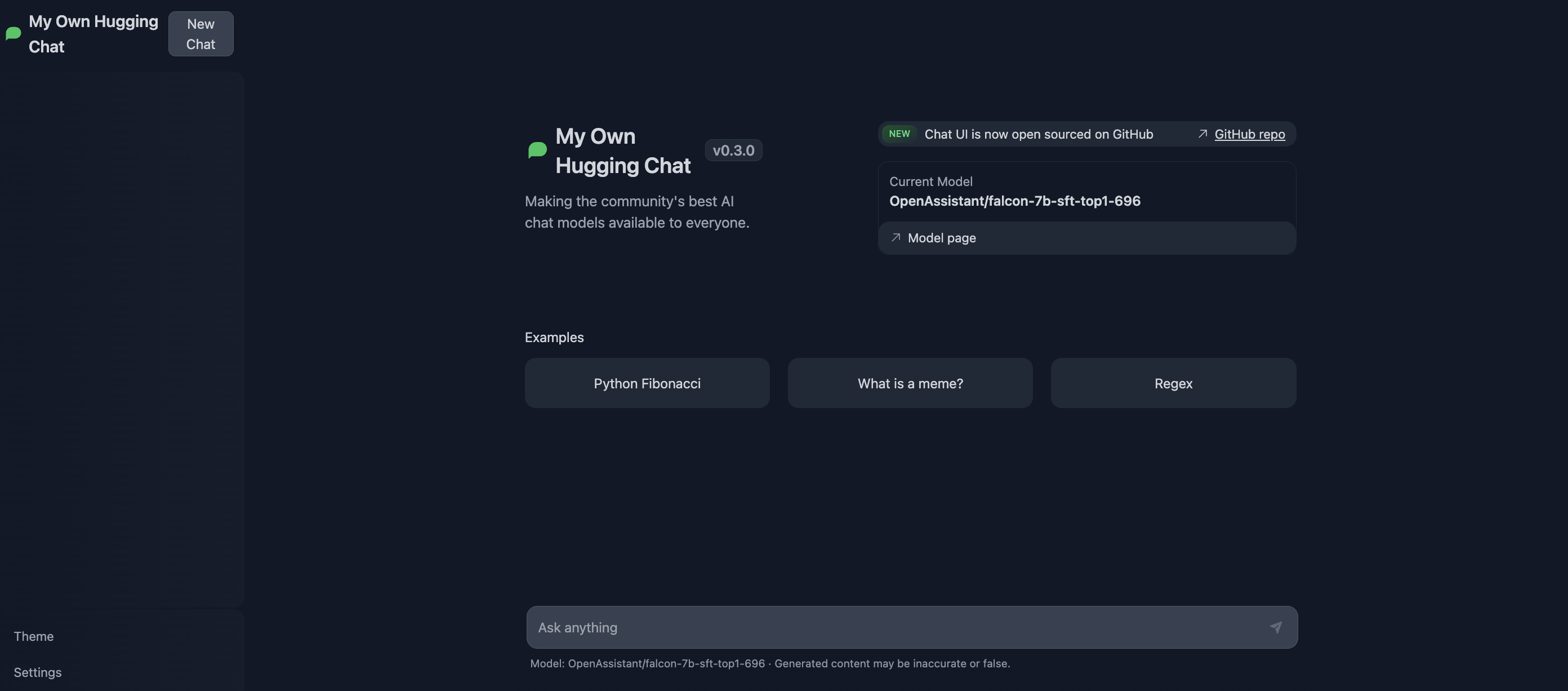
+
+## Gradio
+
+Gradio is a Python library that helps you build web applications for your machine learning models with a few lines of code. It has a `ChatInterface` wrapper that helps create neat UIs for chatbots. Let's take a look at how to create a chatbot with streaming mode using TGI and Gradio. Let's install Gradio and Hub Python library first.
+
+```bash
+pip install huggingface-hub gradio
+```
+
+Assume you are serving your model on port 8080, we will query through [InferenceClient](consuming_tgi#inference-client).
+
+```python
+import gradio as gr
+from huggingface_hub import InferenceClient
+
+client = InferenceClient(model="http://127.0.0.1:8080")
+
+def inference(message, history):
+ partial_message = ""
+ for token in client.text_generation(message, max_new_tokens=20, stream=True):
+ partial_message += token
+ yield partial_message
+
+gr.ChatInterface(
+ inference,
+ chatbot=gr.Chatbot(height=300),
+ textbox=gr.Textbox(placeholder="Chat with me!", container=False, scale=7),
+ description="This is the demo for Gradio UI consuming TGI endpoint with LLaMA 7B-Chat model.",
+ title="Gradio 🤝 TGI",
+ examples=["Are tomatoes vegetables?"],
+ retry_btn="Retry",
+ undo_btn="Undo",
+ clear_btn="Clear",
+).queue().launch()
+```
+
+The UI looks like this 👇
+
+
+
+
+
+
+You can try the demo directly here 👇
+
+
+
+
+
+
+
+
+
+You can disable streaming mode using `return` instead of `yield` in your inference function, like below.
+
+```python
+def inference(message, history):
+ return client.text_generation(message, max_new_tokens=20)
+```
+
+You can read more about how to customize a `ChatInterface` [here](https://www.gradio.app/guides/creating-a-chatbot-fast).
+
+## API documentation
+
+You can consult the OpenAPI documentation of the `text-generation-inference` REST API using the `/docs` route. The Swagger UI is also available [here](https://huggingface.github.io/text-generation-inference).
diff --git a/docs/source/basic_tutorials/gated_model_access.md b/docs/source/basic_tutorials/gated_model_access.md
new file mode 100644
index 00000000..b49c59c9
--- /dev/null
+++ b/docs/source/basic_tutorials/gated_model_access.md
@@ -0,0 +1,24 @@
+# Serving Private & Gated Models
+
+If the model you wish to serve is behind gated access or the model repository on Hugging Face Hub is private, and you have access to the model, you can provide your Hugging Face Hub access token. You can generate and copy a read token from [Hugging Face Hub tokens page](https://huggingface.co/settings/tokens)
+
+If you're using the CLI, set the `HUGGING_FACE_HUB_TOKEN` environment variable. For example:
+
+```
+export HUGGING_FACE_HUB_TOKEN=
+```
+
+If you would like to do it through Docker, you can provide your token by specifying `HUGGING_FACE_HUB_TOKEN` as shown below.
+
+```bash
+model=meta-llama/Llama-2-7b-chat-hf
+volume=$PWD/data
+token=
+
+docker run --gpus all \
+ --shm-size 1g \
+ -e HUGGING_FACE_HUB_TOKEN=$token \
+ -p 8080:80 \
+ -v $volume:/data ghcr.io/huggingface/text-generation-inference:2.0.4 \
+ --model-id $model
+```
diff --git a/docs/source/basic_tutorials/launcher.md b/docs/source/basic_tutorials/launcher.md
new file mode 100644
index 00000000..08a03d0d
--- /dev/null
+++ b/docs/source/basic_tutorials/launcher.md
@@ -0,0 +1,432 @@
+# Text-generation-launcher arguments
+
+
+
+```shell
+Text Generation Launcher
+
+Usage: text-generation-launcher [OPTIONS]
+
+Options:
+```
+## MODEL_ID
+```shell
+ --model-id
+ The name of the model to load. Can be a MODEL_ID as listed on like `gpt2` or `OpenAssistant/oasst-sft-1-pythia-12b`. Or it can be a local directory containing the necessary files as saved by `save_pretrained(...)` methods of transformers
+
+ [env: MODEL_ID=]
+ [default: bigscience/bloom-560m]
+
+```
+## REVISION
+```shell
+ --revision
+ The actual revision of the model if you're referring to a model on the hub. You can use a specific commit id or a branch like `refs/pr/2`
+
+ [env: REVISION=]
+
+```
+## VALIDATION_WORKERS
+```shell
+ --validation-workers
+ The number of tokenizer workers used for payload validation and truncation inside the router
+
+ [env: VALIDATION_WORKERS=]
+ [default: 2]
+
+```
+## SHARDED
+```shell
+ --sharded
+ Whether to shard the model across multiple GPUs By default text-generation-inference will use all available GPUs to run the model. Setting it to `false` deactivates `num_shard`
+
+ [env: SHARDED=]
+ [possible values: true, false]
+
+```
+## NUM_SHARD
+```shell
+ --num-shard
+ The number of shards to use if you don't want to use all GPUs on a given machine. You can use `CUDA_VISIBLE_DEVICES=0,1 text-generation-launcher... --num_shard 2` and `CUDA_VISIBLE_DEVICES=2,3 text-generation-launcher... --num_shard 2` to launch 2 copies with 2 shard each on a given machine with 4 GPUs for instance
+
+ [env: NUM_SHARD=]
+
+```
+## QUANTIZE
+```shell
+ --quantize
+ Whether you want the model to be quantized
+
+ [env: QUANTIZE=]
+
+ Possible values:
+ - awq: 4 bit quantization. Requires a specific AWQ quantized model: . Should replace GPTQ models wherever possible because of the better latency
+ - eetq: 8 bit quantization, doesn't require specific model. Should be a drop-in replacement to bitsandbytes with much better performance. Kernels are from
+ - exl2: Variable bit quantization. Requires a specific EXL2 quantized model: . Requires exllama2 kernels and does not support tensor parallelism (num_shard > 1)
+ - gptq: 4 bit quantization. Requires a specific GTPQ quantized model: . text-generation-inference will use exllama (faster) kernels wherever possible, and use triton kernel (wider support) when it's not. AWQ has faster kernels
+ - bitsandbytes: Bitsandbytes 8bit. Can be applied on any model, will cut the memory requirement in half, but it is known that the model will be much slower to run than the native f16
+ - bitsandbytes-nf4: Bitsandbytes 4bit. Can be applied on any model, will cut the memory requirement by 4x, but it is known that the model will be much slower to run than the native f16
+ - bitsandbytes-fp4: Bitsandbytes 4bit. nf4 should be preferred in most cases but maybe this one has better perplexity performance for you model
+ - fp8: [FP8](https://developer.nvidia.com/blog/nvidia-arm-and-intel-publish-fp8-specification-for-standardization-as-an-interchange-format-for-ai/) (e4m3) works on H100 and above This dtype has native ops should be the fastest if available. This is currently not the fastest because of local unpacking + padding to satisfy matrix multiplication limitations
+
+```
+## SPECULATE
+```shell
+ --speculate
+ The number of input_ids to speculate on If using a medusa model, the heads will be picked up automatically Other wise, it will use n-gram speculation which is relatively free in terms of compute, but the speedup heavily depends on the task
+
+ [env: SPECULATE=]
+
+```
+## DTYPE
+```shell
+ --dtype
+ The dtype to be forced upon the model. This option cannot be used with `--quantize`
+
+ [env: DTYPE=]
+ [possible values: float16, bfloat16]
+
+```
+## TRUST_REMOTE_CODE
+```shell
+ --trust-remote-code
+ Whether you want to execute hub modelling code. Explicitly passing a `revision` is encouraged when loading a model with custom code to ensure no malicious code has been contributed in a newer revision
+
+ [env: TRUST_REMOTE_CODE=]
+
+```
+## MAX_CONCURRENT_REQUESTS
+```shell
+ --max-concurrent-requests
+ The maximum amount of concurrent requests for this particular deployment. Having a low limit will refuse clients requests instead of having them wait for too long and is usually good to handle backpressure correctly
+
+ [env: MAX_CONCURRENT_REQUESTS=]
+ [default: 128]
+
+```
+## MAX_BEST_OF
+```shell
+ --max-best-of
+ This is the maximum allowed value for clients to set `best_of`. Best of makes `n` generations at the same time, and return the best in terms of overall log probability over the entire generated sequence
+
+ [env: MAX_BEST_OF=]
+ [default: 2]
+
+```
+## MAX_STOP_SEQUENCES
+```shell
+ --max-stop-sequences
+ This is the maximum allowed value for clients to set `stop_sequences`. Stop sequences are used to allow the model to stop on more than just the EOS token, and enable more complex "prompting" where users can preprompt the model in a specific way and define their "own" stop token aligned with their prompt
+
+ [env: MAX_STOP_SEQUENCES=]
+ [default: 4]
+
+```
+## MAX_TOP_N_TOKENS
+```shell
+ --max-top-n-tokens
+ This is the maximum allowed value for clients to set `top_n_tokens`. `top_n_tokens` is used to return information about the the `n` most likely tokens at each generation step, instead of just the sampled token. This information can be used for downstream tasks like for classification or ranking
+
+ [env: MAX_TOP_N_TOKENS=]
+ [default: 5]
+
+```
+## MAX_INPUT_TOKENS
+```shell
+ --max-input-tokens
+ This is the maximum allowed input length (expressed in number of tokens) for users. The larger this value, the longer prompt users can send which can impact the overall memory required to handle the load. Please note that some models have a finite range of sequence they can handle. Default to min(max_position_embeddings - 1, 4095)
+
+ [env: MAX_INPUT_TOKENS=]
+
+```
+## MAX_INPUT_LENGTH
+```shell
+ --max-input-length
+ Legacy version of [`Args::max_input_tokens`]
+
+ [env: MAX_INPUT_LENGTH=]
+
+```
+## MAX_TOTAL_TOKENS
+```shell
+ --max-total-tokens
+ This is the most important value to set as it defines the "memory budget" of running clients requests. Clients will send input sequences and ask to generate `max_new_tokens` on top. with a value of `1512` users can send either a prompt of `1000` and ask for `512` new tokens, or send a prompt of `1` and ask for `1511` max_new_tokens. The larger this value, the larger amount each request will be in your RAM and the less effective batching can be. Default to min(max_position_embeddings, 4096)
+
+ [env: MAX_TOTAL_TOKENS=]
+
+```
+## WAITING_SERVED_RATIO
+```shell
+ --waiting-served-ratio
+ This represents the ratio of waiting queries vs running queries where you want to start considering pausing the running queries to include the waiting ones into the same batch. `waiting_served_ratio=1.2` Means when 12 queries are waiting and there's only 10 queries left in the current batch we check if we can fit those 12 waiting queries into the batching strategy, and if yes, then batching happens delaying the 10 running queries by a `prefill` run.
+
+ This setting is only applied if there is room in the batch as defined by `max_batch_total_tokens`.
+
+ [env: WAITING_SERVED_RATIO=]
+ [default: 0.3]
+
+```
+## MAX_BATCH_PREFILL_TOKENS
+```shell
+ --max-batch-prefill-tokens
+ Limits the number of tokens for the prefill operation. Since this operation take the most memory and is compute bound, it is interesting to limit the number of requests that can be sent. Default to `max_input_tokens + 50` to give a bit of room
+
+ [env: MAX_BATCH_PREFILL_TOKENS=]
+
+```
+## MAX_BATCH_TOTAL_TOKENS
+```shell
+ --max-batch-total-tokens
+ **IMPORTANT** This is one critical control to allow maximum usage of the available hardware.
+
+ This represents the total amount of potential tokens within a batch. When using padding (not recommended) this would be equivalent of `batch_size` * `max_total_tokens`.
+
+ However in the non-padded (flash attention) version this can be much finer.
+
+ For `max_batch_total_tokens=1000`, you could fit `10` queries of `total_tokens=100` or a single query of `1000` tokens.
+
+ Overall this number should be the largest possible amount that fits the remaining memory (after the model is loaded). Since the actual memory overhead depends on other parameters like if you're using quantization, flash attention or the model implementation, text-generation-inference cannot infer this number automatically.
+
+ [env: MAX_BATCH_TOTAL_TOKENS=]
+
+```
+## MAX_WAITING_TOKENS
+```shell
+ --max-waiting-tokens
+ This setting defines how many tokens can be passed before forcing the waiting queries to be put on the batch (if the size of the batch allows for it). New queries require 1 `prefill` forward, which is different from `decode` and therefore you need to pause the running batch in order to run `prefill` to create the correct values for the waiting queries to be able to join the batch.
+
+ With a value too small, queries will always "steal" the compute to run `prefill` and running queries will be delayed by a lot.
+
+ With a value too big, waiting queries could wait for a very long time before being allowed a slot in the running batch. If your server is busy that means that requests that could run in ~2s on an empty server could end up running in ~20s because the query had to wait for 18s.
+
+ This number is expressed in number of tokens to make it a bit more "model" agnostic, but what should really matter is the overall latency for end users.
+
+ [env: MAX_WAITING_TOKENS=]
+ [default: 20]
+
+```
+## MAX_BATCH_SIZE
+```shell
+ --max-batch-size
+ Enforce a maximum number of requests per batch Specific flag for hardware targets that do not support unpadded inference
+
+ [env: MAX_BATCH_SIZE=]
+
+```
+## CUDA_GRAPHS
+```shell
+ --cuda-graphs
+ Specify the batch sizes to compute cuda graphs for. Use "0" to disable. Default = "1,2,4,8,16,32"
+
+ [env: CUDA_GRAPHS=]
+
+```
+## HOSTNAME
+```shell
+ --hostname
+ The IP address to listen on
+
+ [env: HOSTNAME=]
+ [default: 0.0.0.0]
+
+```
+## PORT
+```shell
+ -p, --port
+ The port to listen on
+
+ [env: PORT=]
+ [default: 3000]
+
+```
+## SHARD_UDS_PATH
+```shell
+ --shard-uds-path
+ The name of the socket for gRPC communication between the webserver and the shards
+
+ [env: SHARD_UDS_PATH=]
+ [default: /tmp/text-generation-server]
+
+```
+## MASTER_ADDR
+```shell
+ --master-addr
+ The address the master shard will listen on. (setting used by torch distributed)
+
+ [env: MASTER_ADDR=]
+ [default: localhost]
+
+```
+## MASTER_PORT
+```shell
+ --master-port
+ The address the master port will listen on. (setting used by torch distributed)
+
+ [env: MASTER_PORT=]
+ [default: 29500]
+
+```
+## HUGGINGFACE_HUB_CACHE
+```shell
+ --huggingface-hub-cache
+ The location of the huggingface hub cache. Used to override the location if you want to provide a mounted disk for instance
+
+ [env: HUGGINGFACE_HUB_CACHE=]
+
+```
+## WEIGHTS_CACHE_OVERRIDE
+```shell
+ --weights-cache-override
+ The location of the huggingface hub cache. Used to override the location if you want to provide a mounted disk for instance
+
+ [env: WEIGHTS_CACHE_OVERRIDE=]
+
+```
+## DISABLE_CUSTOM_KERNELS
+```shell
+ --disable-custom-kernels
+ For some models (like bloom), text-generation-inference implemented custom cuda kernels to speed up inference. Those kernels were only tested on A100. Use this flag to disable them if you're running on different hardware and encounter issues
+
+ [env: DISABLE_CUSTOM_KERNELS=]
+
+```
+## CUDA_MEMORY_FRACTION
+```shell
+ --cuda-memory-fraction
+ Limit the CUDA available memory. The allowed value equals the total visible memory multiplied by cuda-memory-fraction
+
+ [env: CUDA_MEMORY_FRACTION=]
+ [default: 1.0]
+
+```
+## ROPE_SCALING
+```shell
+ --rope-scaling
+ Rope scaling will only be used for RoPE models and allow rescaling the position rotary to accomodate for larger prompts.
+
+ Goes together with `rope_factor`.
+
+ `--rope-factor 2.0` gives linear scaling with a factor of 2.0 `--rope-scaling dynamic` gives dynamic scaling with a factor of 1.0 `--rope-scaling linear` gives linear scaling with a factor of 1.0 (Nothing will be changed basically)
+
+ `--rope-scaling linear --rope-factor` fully describes the scaling you want
+
+ [env: ROPE_SCALING=]
+ [possible values: linear, dynamic]
+
+```
+## ROPE_FACTOR
+```shell
+ --rope-factor
+ Rope scaling will only be used for RoPE models See `rope_scaling`
+
+ [env: ROPE_FACTOR=]
+
+```
+## JSON_OUTPUT
+```shell
+ --json-output
+ Outputs the logs in JSON format (useful for telemetry)
+
+ [env: JSON_OUTPUT=]
+
+```
+## OTLP_ENDPOINT
+```shell
+ --otlp-endpoint
+ [env: OTLP_ENDPOINT=]
+
+```
+## CORS_ALLOW_ORIGIN
+```shell
+ --cors-allow-origin
+ [env: CORS_ALLOW_ORIGIN=]
+
+```
+## WATERMARK_GAMMA
+```shell
+ --watermark-gamma
+ [env: WATERMARK_GAMMA=]
+
+```
+## WATERMARK_DELTA
+```shell
+ --watermark-delta
+ [env: WATERMARK_DELTA=]
+
+```
+## NGROK
+```shell
+ --ngrok
+ Enable ngrok tunneling
+
+ [env: NGROK=]
+
+```
+## NGROK_AUTHTOKEN
+```shell
+ --ngrok-authtoken
+ ngrok authentication token
+
+ [env: NGROK_AUTHTOKEN=]
+
+```
+## NGROK_EDGE
+```shell
+ --ngrok-edge
+ ngrok edge
+
+ [env: NGROK_EDGE=]
+
+```
+## TOKENIZER_CONFIG_PATH
+```shell
+ --tokenizer-config-path
+ The path to the tokenizer config file. This path is used to load the tokenizer configuration which may include a `chat_template`. If not provided, the default config will be used from the model hub
+
+ [env: TOKENIZER_CONFIG_PATH=]
+
+```
+## DISABLE_GRAMMAR_SUPPORT
+```shell
+ --disable-grammar-support
+ Disable outlines grammar constrained generation. This is a feature that allows you to generate text that follows a specific grammar
+
+ [env: DISABLE_GRAMMAR_SUPPORT=]
+
+```
+## ENV
+```shell
+ -e, --env
+ Display a lot of information about your runtime environment
+
+```
+## MAX_CLIENT_BATCH_SIZE
+```shell
+ --max-client-batch-size
+ Control the maximum number of inputs that a client can send in a single request
+
+ [env: MAX_CLIENT_BATCH_SIZE=]
+ [default: 4]
+
+```
+## LORA_IDS
+```shell
+ --lora-ids
+ Specify LoRA adapters
+
+ [env: LORA_IDS=]
+ [default: empty]
+
+```
+## HELP
+```shell
+ -h, --help
+ Print help (see a summary with '-h')
+
+```
+## VERSION
+```shell
+ -V, --version
+ Print version
+
+```
diff --git a/docs/source/basic_tutorials/monitoring.md b/docs/source/basic_tutorials/monitoring.md
new file mode 100644
index 00000000..509b0aff
--- /dev/null
+++ b/docs/source/basic_tutorials/monitoring.md
@@ -0,0 +1,75 @@
+# Monitoring TGI server with Prometheus and Grafana dashboard
+
+TGI server deployment can easily be monitored through a Grafana dashboard, consuming a Prometheus data collection. Example of inspectable metrics are statistics on the effective batch sizes used by TGI, prefill/decode latencies, number of generated tokens, etc.
+
+In this tutorial, we look at how to set up a local Grafana dashboard to monitor TGI usage.
+
+
+
+## Setup on the server machine
+
+First, on your server machine, TGI needs to be launched as usual. TGI exposes [multiple](https://github.com/huggingface/text-generation-inference/discussions/1127#discussioncomment-7240527) metrics that can be collected by Prometheus monitoring server.
+
+In the rest of this tutorial, we assume that TGI was launched through Docker with `--network host`.
+
+On the server where TGI is hosted, a Prometheus server needs to be installed and launched. To do so, please follow [Prometheus installation instructions](https://prometheus.io/download/#prometheus). For example, at the time of writing on a Linux machine:
+
+```
+wget https://github.com/prometheus/prometheus/releases/download/v2.52.0/prometheus-2.52.0.linux-amd64.tar.gz
+tar -xvzf prometheus-2.52.0.linux-amd64.tar.gz
+cd prometheus
+```
+
+Prometheus needs to be configured to listen on TGI's port. To do so, in Prometheus configuration file `prometheus.yml`, one needs to edit the lines:
+```
+ static_configs:
+ - targets: ["0.0.0.0:80"]
+```
+to use the correct IP address and port.
+
+We suggest to try `curl 0.0.0.0:80/generate -X POST -d '{"inputs":"hey chatbot, how are","parameters":{"max_new_tokens":15}}' -H 'Content-Type: application/json'` on the server side to make sure to configure the correct IP and port.
+
+Once Prometheus is configured, Prometheus server can be launched on the same machine where TGI is launched:
+```
+./prometheus --config.file="prometheus.yml"
+```
+
+In this guide, Prometheus monitoring data will be consumed on a local computer. Hence, we need to forward Prometheus port (by default 9090) to the local computer. To do so, we can for example:
+* Use ssh [local port forwarding](https://www.ssh.com/academy/ssh/tunneling-example)
+* Use ngrok port tunneling
+
+For simplicity, we will use [Ngrok](https://ngrok.com/docs/) in this guide to tunnel Prometheus port from the TGI server to the outside word.
+
+For that, you should follow the steps at https://dashboard.ngrok.com/get-started/setup/linux, and once Ngrok is installed, use:
+```bash
+ngrok http http://0.0.0.0:9090
+```
+
+As a sanity check, one can make sure that Prometheus server can be accessed at the URL given by Ngrok (in the style of https://d661-4-223-164-145.ngrok-free.app) from a local machine.
+
+## Setup on the monitoring machine
+
+Monitoring is typically done on an other machine than the server one. We use a Grafana dashboard to monitor TGI's server usage.
+
+Two options are available:
+* Use Grafana Cloud for an hosted dashboard solution (https://grafana.com/products/cloud/).
+* Self-host a grafana dashboard.
+
+In this tutorial, for simplicity, we will self host the dashbard. We recommend installing Grafana Open-source edition following [the official install instructions](https://grafana.com/grafana/download?platform=linux&edition=oss), using the available Linux binaries. For example:
+
+```bash
+wget https://dl.grafana.com/oss/release/grafana-11.0.0.linux-amd64.tar.gz
+tar -zxvf grafana-11.0.0.linux-amd64.tar.gz
+cd grafana-11.0.0
+./bin/grafana-server
+```
+
+Once the Grafana server is launched, the Grafana interface is available at http://localhost:3000. One needs to log in with the `admin` username and `admin` password.
+
+Once logged in, the Prometheus data source for Grafana needs to be configured, in the option `Add your first data source`. There, a Prometheus data source needs to be added with the Ngrok address we got earlier, that exposes Prometheus port (example: https://d661-4-223-164-145.ngrok-free.app).
+
+Once Prometheus data source is configured, we can finally create our dashboard! From home, go to `Create your first dashboard` and then `Import dashboard`. There, we will use the recommended dashboard template [tgi_grafana.json](https://github.com/huggingface/text-generation-inference/blob/main/assets/tgi_grafana.json) for a dashboard ready to be used, but you may configure your own dashboard as you like.
+
+Community contributed dashboard templates are also available, for example [here](https://grafana.com/grafana/dashboards/19831-text-generation-inference-dashboard/) or [here](https://grafana.com/grafana/dashboards/20246-text-generation-inference/).
+
+Load your dashboard configuration, and your TGI dashboard should be ready to go!
diff --git a/docs/source/basic_tutorials/non_core_models.md b/docs/source/basic_tutorials/non_core_models.md
new file mode 100644
index 00000000..2badaff0
--- /dev/null
+++ b/docs/source/basic_tutorials/non_core_models.md
@@ -0,0 +1,24 @@
+# Non-core Model Serving
+
+TGI supports various LLM architectures (see full list [here](../supported_models)). If you wish to serve a model that is not one of the supported models, TGI will fallback to the `transformers` implementation of that model. This means you will be unable to use some of the features introduced by TGI, such as tensor-parallel sharding or flash attention. However, you can still get many benefits of TGI, such as continuous batching or streaming outputs.
+
+You can serve these models using the same Docker command-line invocation as with fully supported models 👇
+
+```bash
+docker run --gpus all --shm-size 1g -p 8080:80 -v $volume:/data ghcr.io/huggingface/text-generation-inference:latest --model-id gpt2
+```
+
+If the model you wish to serve is a custom transformers model, and its weights and implementation are available in the Hub, you can still serve the model by passing the `--trust-remote-code` flag to the `docker run` command like below 👇
+
+```bash
+docker run --gpus all --shm-size 1g -p 8080:80 -v $volume:/data ghcr.io/huggingface/text-generation-inference:latest --model-id --trust-remote-code
+```
+
+Finally, if the model is not on Hugging Face Hub but on your local, you can pass the path to the folder that contains your model like below 👇
+
+```bash
+# Make sure your model is in the $volume directory
+docker run --shm-size 1g -p 8080:80 -v $volume:/data ghcr.io/huggingface/text-generation-inference:latest --model-id /data/
+```
+
+You can refer to [transformers docs on custom models](https://huggingface.co/docs/transformers/main/en/custom_models) for more information.
diff --git a/docs/source/basic_tutorials/preparing_model.md b/docs/source/basic_tutorials/preparing_model.md
new file mode 100644
index 00000000..71ca5598
--- /dev/null
+++ b/docs/source/basic_tutorials/preparing_model.md
@@ -0,0 +1,22 @@
+# Preparing the Model
+
+Text Generation Inference improves the model in several aspects.
+
+## Quantization
+
+TGI supports [bits-and-bytes](https://github.com/TimDettmers/bitsandbytes#bitsandbytes), [GPT-Q](https://arxiv.org/abs/2210.17323) and [AWQ](https://arxiv.org/abs/2306.00978) quantization. To speed up inference with quantization, simply set `quantize` flag to `bitsandbytes`, `gptq` or `awq` depending on the quantization technique you wish to use. When using GPT-Q quantization, you need to point to one of the models [here](https://huggingface.co/models?search=gptq) when using AWQ quantization, you need to point to one of the models [here](https://huggingface.co/models?search=awq). To get more information about quantization, please refer to [quantization guide](./../conceptual/quantization)
+
+
+## RoPE Scaling
+
+RoPE scaling can be used to increase the sequence length of the model during the inference time without necessarily fine-tuning it. To enable RoPE scaling, simply pass `--rope-scaling`, `--max-input-length` and `--rope-factors` flags when running through CLI. `--rope-scaling` can take the values `linear` or `dynamic`. If your model is not fine-tuned to a longer sequence length, use `dynamic`. `--rope-factor` is the ratio between the intended max sequence length and the model's original max sequence length. Make sure to pass `--max-input-length` to provide maximum input length for extension.
+
+
+
+We recommend using `dynamic` RoPE scaling.
+
+
+
+## Safetensors
+
+[Safetensors](https://github.com/huggingface/safetensors) is a fast and safe persistence format for deep learning models, and is required for tensor parallelism. TGI supports `safetensors` model loading under the hood. By default, given a repository with `safetensors` and `pytorch` weights, TGI will always load `safetensors`. If there's no `pytorch` weights, TGI will convert the weights to `safetensors` format.
diff --git a/docs/source/basic_tutorials/safety.md b/docs/source/basic_tutorials/safety.md
new file mode 100644
index 00000000..0b865db4
--- /dev/null
+++ b/docs/source/basic_tutorials/safety.md
@@ -0,0 +1,31 @@
+# Model safety.
+
+[Pytorch uses pickle](https://pytorch.org/docs/master/generated/torch.load.html) by default meaning that for quite a long while
+*Every* model using that format is potentially executing unintended code while purely loading the model.
+
+There is a big red warning on Python's page for pickle [link](https://docs.python.org/3/library/pickle.html) but for quite a while
+this was ignored by the community. Now that AI/ML is getting used much more ubiquitously we need to switch away from this format.
+
+HuggingFace is leading the effort here by creating a new format which contains pure data ([safetensors](https://github.com/huggingface/safetensors))
+and moving slowly but surely all the libs to make use of it by default.
+The move is intentionnally slow in order to make breaking changes as little impact as possible on users throughout.
+
+
+# TGI 2.0
+
+Since the release of TGI 2.0, we take the opportunity of this major version increase to break backward compatibility for these pytorch
+models (since they are a huge security risk for anyone deploying them).
+
+
+From now on, TGI will not convert automatically pickle files without having `--trust-remote-code` flag or `TRUST_REMOTE_CODE=true` in the environment variables.
+This flag is already used for community defined inference code, and is therefore quite representative of the level of confidence you are giving the model providers.
+
+
+If you want to use a model that uses pickle, but you still do not want to trust the authors entirely we recommend making a convertion on our space made for that.
+
+https://huggingface.co/spaces/safetensors/convert
+
+This space will create a PR on the original model, which you are use directly regardless of merge status from the original authors. Just use
+```
+docker run .... --revision refs/pr/#ID # Or use REVISION=refs/pr/#ID in the environment
+```
diff --git a/docs/source/basic_tutorials/train_medusa.md b/docs/source/basic_tutorials/train_medusa.md
new file mode 100644
index 00000000..ba2e43b7
--- /dev/null
+++ b/docs/source/basic_tutorials/train_medusa.md
@@ -0,0 +1,208 @@
+# Train Medusa
+
+This tutorial will show you how to train a Medusa model on a dataset of your choice. Please check out the [speculation documentation](../conceptual/speculation) for more information on how Medusa works and speculation in general.
+
+## What are the benefits of training a Medusa model?
+
+Training Medusa heads can greatly improve the speed of generation. Medusa adds extra "heads" to LLMs to predict multiple future tokens simultaneously. When augmenting a model with Medusa, the original model stays untouched, and only the new heads are fine-tuned during training.
+
+One of the most important things is to have a good dataset (with similar data to what will be used in production) because Medusa has a much higher hit-rate when the generation is in-domain.
+
+If you train Medusa on a dataset that is very different from the one you will use in production then the model will not be able to predict the future tokens accurately and consequently the speedup will be minimal or non-existent.
+
+## Self-distillation (Generating data for training)
+
+There are many methods for preparing data for training, but one of the easiest and most effective ways is to "self-distill" the data. This means that you can use the same model to generate the data that you will use to train the model.
+
+Essentially, you prompt the model with a similar input to what you will use in production and the model will generate the output.
+
+We'll use this output to help train the medusa heads to predict the `n+1`, `n+2`, `n+3`, etc tokens in the sequence.
+
+## Training
+
+The original implementation of Medusa is available at [https://github.com/FasterDecoding/Medusa](https://github.com/FasterDecoding/Medusa) and we'll follow a very similar process to train the model as described on the original repository.
+
+### Getting Started
+
+There are two methods for training the model:
+
+- `torchrun` that is a wrapper around `torch.distributed.launch`
+- a forked version of `axlotl` that supports Medusa
+
+In this tutorial we'll use `torchrun` to train the model as it is the most straightforward way to train the model but similar steps can be followed to train the model using `axlotl` if you prefer.
+
+### Training with `torchrun`
+
+```bash
+mkdir medusa-training
+cd medusa-training
+
+pyenv install 3.10
+pyenv local 3.10
+
+uv venv -p 3.10
+source .venv/bin/activate
+```
+
+Now lets clone the original `Medusa` repository and install the library.
+
+```bash
+git clone https://github.com/FasterDecoding/Medusa.git
+cd Medusa
+pip install -e .
+```
+
+Next we'll need some data to train on, we can use the `ShareGPT_Vicuna_unfiltered` dataset that is available on the Hugging Face Hub.
+
+```bash
+apt install git-lfs
+git lfs install
+git clone https://huggingface.co/datasets/Aeala/ShareGPT_Vicuna_unfiltered
+```
+
+Currently our directory structure looks like this:
+
+```bash
+.
+├── assets
+├── CITATION.cff
+├── create_data.py
+├── data_generation
+├── deepspeed.json
+├── last_run_prepared
+├── LICENSE
+├── llm_judge
+├── medusa
+├── medusa_llm.egg-info
+├── mistral.json
+├── notebooks
+├── pyproject.toml
+├── README.md
+├── ROADMAP.md
+├── scripts
+├── ShareGPT_Vicuna_unfiltered
+│ ├── README.md
+│ ├── ShareGPT_2023.05.04v0_Wasteland_Edition.json
+│ └── ShareGPT_V4.3_unfiltered_cleaned_split.json
+├── simple_gradio_interface.py
+├── tiny-llama.json
+└── vicuna_7b_qlora_stage1
+```
+
+## Start Training
+
+Now the lets generate the data and start training the model. This process will take a while since we are generating data from the model.
+
+First make sure you have an instance of TGI running with the model you want to use for self-distillation.
+
+```bash
+model=HuggingFaceH4/zephyr-7b-beta
+volume=/home/ubuntu/.cache/huggingface/hub/
+
+docker run --gpus all --shm-size 1g -p 8080:80 -v $volume:/data ghcr.io/huggingface/text-generation-inference:latest --model-id $model
+```
+
+Now we can generate the data using the `create_data.py` script.
+
+```bash
+python create_data.py \
+ --input-filename ShareGPT_Vicuna_unfiltered/ShareGPT_V4.3_unfiltered_cleaned_split.json \
+ --output-filename zephyr_self_distill.json
+```
+
+At this point our terminal should look like this:
+
+
+
+
+
+> Note: In the screen shot above we are only using a the first 500 examples from the dataset to speed up the process, you should have a much larger dataset for training.
+
+Now we can finally get to the fun part and start training the model!
+
+Using `torchrun` we can easily launch the `medusa` training script with the `zephyr_self_distill.json` configuration file.
+
+> NOTE: If you just self-distilled you may still have the model running, make sure to stop it before starting the training in order to allow all of the resources to be used for training.
+
+```bash
+WANDB_MODE=offline torchrun --nproc_per_node=4 medusa/train/train_legacy.py \
+ --model_name_or_path HuggingFaceH4/zephyr-7b-beta \
+ --data_path zephyr_self_distill.json \
+ --bf16 True \
+ --output_dir zephyr_out \
+ --num_train_epochs 5 \
+ --per_device_train_batch_size 4 \
+ --per_device_eval_batch_size 4 \
+ --gradient_accumulation_steps 4 \
+ --evaluation_strategy "no" \
+ --save_strategy "no" \
+ --learning_rate 1e-3 \
+ --weight_decay 0.0 \
+ --warmup_ratio 0.1 \
+ --lr_scheduler_type "cosine" \
+ --logging_steps 1 \
+ --tf32 True \
+ --model_max_length 2048 \
+ --lazy_preprocess True \
+ --medusa_num_heads 3 \
+ --medusa_num_layers 1 \
+ --deepspeed deepspeed.json
+```
+
+
+
+
+
+If successful, you should see the similar output to the one below:
+
+```bash
+wandb: Run history:
+wandb: train/epoch ▁▁▁▁▁▂▂▂▂▂▃▃▃▃▃▄▄▄▄▄▅▅▅▅▅▅▅▆▆▆▆▆▇▇▇▇▇███
+wandb: train/global_step ▁▁▁▁▁▂▂▂▂▂▃▃▃▃▃▄▄▄▄▄▅▅▅▅▅▅▅▆▆▆▆▆▇▇▇▇▇███
+wandb: train/learning_rate ▅███▇▇▆▅▅▄▃▂▂▁▁▁
+wandb: train/loss ██▆▄▄▃▃▂▂▃▁▁▂▁▁▁
+wandb: train/medusa0_loss ▆▆▇▆▆▅▄▅▃▃▃▃▂▂▂▂▂▃▂▂▂▁▁▁▂▁▁▁▁▁█▁▁▁▂▁▁▁▁▁
+wandb: train/medusa0_top1 ▁▁▁▁▁▁▁▁▃▂▃▃▄▄▄▃▄▃▄▄▅▅▆▅▆▆▇▅▇▇▄▇█▇▅▇█▆▇▇
+wandb: train/medusa1_loss ▇▇█▇▇▆▅▅▃▄▃▃▃▃▃▃▃▃▃▃▂▁▂▂▂▁▁▂▁▁▇▁▁▁▂▁▁▁▁▁
+wandb: train/medusa1_top1 ▁▁▁▁▁▁▁▁▃▂▃▃▃▄▄▃▃▂▃▃▅▅▆▄█▆▇▅▇▇▅█▇▇▅▇█▆▆▇
+wandb: train/medusa2_loss ▃▃▄▄▄▃▃▃▂▂▂▂▂▂▂▂▂▂▂▂▁▁▁▁▁▁▁▁▁▁█▁▁▁▂▁▁▁▁▁
+wandb: train/medusa2_top1 ▁▁▁▂▁▁▁▁▂▂▃▃▃▄▄▃▃▂▃▃▅▆▅▄█▆▆▅▆▆▄█▇▇▄▇█▆▆▇
+wandb: train/total_flos ▁
+wandb: train/train_loss ▁
+wandb: train/train_runtime ▁
+wandb: train/train_samples_per_second ▁
+wandb: train/train_steps_per_second ▁
+wandb:
+wandb: Run summary:
+wandb: train/epoch 2.0
+wandb: train/global_step 16
+wandb: train/learning_rate 0.0
+wandb: train/loss 14.8906
+wandb: train/medusa0_loss 4.25
+wandb: train/medusa0_top1 0.28809
+wandb: train/medusa1_loss 4.8125
+wandb: train/medusa1_top1 0.22727
+wandb: train/medusa2_loss 5.5
+wandb: train/medusa2_top1 0.17293
+wandb: train/total_flos 0.0
+wandb: train/train_loss 23.98242
+wandb: train/train_runtime 396.9266
+wandb: train/train_samples_per_second 2.519
+wandb: train/train_steps_per_second 0.04
+```
+
+Last but most importantly, don't forget to push this model to the Hugging Face Hub so you can use it in your projects.
+
+```bash
+python -m medusa.hf_utils \
+ --folder zephyr_out_medusa_mlp_zephyr-7b-beta_medusa_3_lr_0.001_layers_1 \
+ --repo drbh/zephyr_medusa_demo
+```
+
+Woo, we've successfully trained a Medusa model and pushed it to the Hugging Face Hub! 🎉
diff --git a/docs/source/basic_tutorials/using_cli.md b/docs/source/basic_tutorials/using_cli.md
new file mode 100644
index 00000000..64554069
--- /dev/null
+++ b/docs/source/basic_tutorials/using_cli.md
@@ -0,0 +1,35 @@
+# Using TGI CLI
+
+You can use TGI command-line interface (CLI) to download weights, serve and quantize models, or get information on serving parameters. To install the CLI, please refer to [the installation section](../installation#install-cli).
+
+`text-generation-server` lets you download the model with `download-weights` command like below 👇
+
+```bash
+text-generation-server download-weights MODEL_HUB_ID
+```
+
+You can also use it to quantize models like below 👇
+
+```bash
+text-generation-server quantize MODEL_HUB_ID OUTPUT_DIR
+```
+
+You can use `text-generation-launcher` to serve models.
+
+```bash
+text-generation-launcher --model-id MODEL_HUB_ID --port 8080
+```
+
+There are many options and parameters you can pass to `text-generation-launcher`. The documentation for CLI is kept minimal and intended to rely on self-generating documentation, which can be found by running
+
+```bash
+text-generation-launcher --help
+```
+
+You can also find it hosted in this [Swagger UI](https://huggingface.github.io/text-generation-inference/).
+
+Same documentation can be found for `text-generation-server`.
+
+```bash
+text-generation-server --help
+```
diff --git a/docs/source/basic_tutorials/using_guidance.md b/docs/source/basic_tutorials/using_guidance.md
new file mode 100644
index 00000000..d0008fdb
--- /dev/null
+++ b/docs/source/basic_tutorials/using_guidance.md
@@ -0,0 +1,359 @@
+# Guidance
+
+Text Generation Inference (TGI) now supports [JSON and regex grammars](#grammar-and-constraints) and [tools and functions](#tools-and-functions) to help developers guide LLM responses to fit their needs.
+
+These feature are available starting from version `1.4.3`. They are accessible via the [`huggingface_hub`](https://pypi.org/project/huggingface-hub/) library. The tool support is compatible with OpenAI's client libraries. The following guide will walk you through the new features and how to use them!
+
+_note: guidance is supported as grammar in the `/generate` endpoint and as tools in the `/chat/completions` endpoint._
+
+## How it works
+
+TGI leverages the [outlines](https://github.com/outlines-dev/outlines) library to efficiently parse and compile the grammatical structures and tools specified by users. This integration transforms the defined grammars into an intermediate representation that acts as a framework to guide and constrain content generation, ensuring that outputs adhere to the specified grammatical rules.
+
+If you are interested in the technical details on how outlines is used in TGI, you can check out the [conceptual guidance documentation](../conceptual/guidance).
+
+## Table of Contents 📚
+
+### Grammar and Constraints
+
+- [The Grammar Parameter](#the-grammar-parameter): Shape your AI's responses with precision.
+- [Constrain with Pydantic](#constrain-with-pydantic): Define a grammar using Pydantic models.
+- [JSON Schema Integration](#json-schema-integration): Fine-grained control over your requests via JSON schema.
+- [Using the client](#using-the-client): Use TGI's client libraries to shape the AI's responses.
+
+### Tools and Functions
+
+- [The Tools Parameter](#the-tools-parameter): Enhance the AI's capabilities with predefined functions.
+- [Via the client](#text-generation-inference-client): Use TGI's client libraries to interact with the Messages API and Tool functions.
+- [OpenAI integration](#openai-integration): Use OpenAI's client libraries to interact with TGI's Messages API and Tool functions.
+
+## Grammar and Constraints 🛣️
+
+### The Grammar Parameter
+
+In TGI `1.4.3`, we've introduced the grammar parameter, which allows you to specify the format of the response you want from the LLM.
+
+Using curl, you can make a request to TGI's Messages API with the grammar parameter. This is the most primitive way to interact with the API and using [Pydantic](#constrain-with-pydantic) is recommended for ease of use and readability.
+
+```json
+curl localhost:3000/generate \
+ -X POST \
+ -H 'Content-Type: application/json' \
+ -d '{
+ "inputs": "I saw a puppy a cat and a raccoon during my bike ride in the park",
+ "parameters": {
+ "repetition_penalty": 1.3,
+ "grammar": {
+ "type": "json",
+ "value": {
+ "properties": {
+ "location": {
+ "type": "string"
+ },
+ "activity": {
+ "type": "string"
+ },
+ "animals_seen": {
+ "type": "integer",
+ "minimum": 1,
+ "maximum": 5
+ },
+ "animals": {
+ "type": "array",
+ "items": {
+ "type": "string"
+ }
+ }
+ },
+ "required": ["location", "activity", "animals_seen", "animals"]
+ }
+ }
+ }
+}'
+// {"generated_text":"{ \n\n\"activity\": \"biking\",\n\"animals\": [\"puppy\",\"cat\",\"raccoon\"],\n\"animals_seen\": 3,\n\"location\": \"park\"\n}"}
+
+```
+
+### Hugging Face Hub Python Library
+
+The Hugging Face Hub Python library provides a client that makes it easy to interact with the Messages API. Here's an example of how to use the client to send a request with a grammar parameter.
+
+```python
+from huggingface_hub import InferenceClient
+
+client = InferenceClient("http://localhost:3000")
+
+schema = {
+ "properties": {
+ "location": {"title": "Location", "type": "string"},
+ "activity": {"title": "Activity", "type": "string"},
+ "animals_seen": {
+ "maximum": 5,
+ "minimum": 1,
+ "title": "Animals Seen",
+ "type": "integer",
+ },
+ "animals": {"items": {"type": "string"}, "title": "Animals", "type": "array"},
+ },
+ "required": ["location", "activity", "animals_seen", "animals"],
+ "title": "Animals",
+ "type": "object",
+}
+
+user_input = "I saw a puppy a cat and a raccoon during my bike ride in the park"
+resp = client.text_generation(
+ f"convert to JSON: 'f{user_input}'. please use the following schema: {schema}",
+ max_new_tokens=100,
+ seed=42,
+ grammar={"type": "json", "value": schema},
+)
+
+print(resp)
+# { "activity": "bike ride", "animals": ["puppy", "cat", "raccoon"], "animals_seen": 3, "location": "park" }
+
+```
+
+A grammar can be defined using Pydantic models, JSON schemas, or regular expressions. The LLM will then generate a response that conforms to the specified grammar.
+
+> Note: A grammar must compile to an intermediate representation to constrain the output. Grammar compilation is a computationally expensive and may take a few seconds to complete on the first request. Subsequent requests will use the cached grammar and will be much faster.
+
+### Constrain with Pydantic
+
+Using Pydantic models we can define a similar grammar as the previous example in a shorter and more readable way.
+
+```python
+from huggingface_hub import InferenceClient
+from pydantic import BaseModel, conint
+from typing import List
+
+
+class Animals(BaseModel):
+ location: str
+ activity: str
+ animals_seen: conint(ge=1, le=5) # Constrained integer type
+ animals: List[str]
+
+
+client = InferenceClient("http://localhost:3000")
+
+user_input = "I saw a puppy a cat and a raccoon during my bike ride in the park"
+resp = client.text_generation(
+ f"convert to JSON: 'f{user_input}'. please use the following schema: {Animals.schema()}",
+ max_new_tokens=100,
+ seed=42,
+ grammar={"type": "json", "value": Animals.schema()},
+)
+
+print(resp)
+# { "activity": "bike ride", "animals": ["puppy", "cat", "raccoon"], "animals_seen": 3, "location": "park" }
+
+
+```
+
+defining a grammar as regular expressions
+
+```python
+from huggingface_hub import InferenceClient
+
+client = InferenceClient("http://localhost:3000")
+
+regexp = "((25[0-5]|2[0-4]\\d|[01]?\\d\\d?)\\.){3}(25[0-5]|2[0-4]\\d|[01]?\\d\\d?)"
+
+resp = client.text_generation(
+ f"Whats Googles DNS? Please use the following regex: {regexp}",
+ seed=42,
+ grammar={
+ "type": "regex",
+ "value": regexp,
+ },
+)
+
+
+print(resp)
+# 7.1.1.1
+
+```
+
+## Tools and Functions 🛠️
+
+### The Tools Parameter
+
+In addition to the grammar parameter, we've also introduced a set of tools and functions to help you get the most out of the Messages API.
+
+Tools are a set of user defined functions that can be used in tandem with the chat functionality to enhance the LLM's capabilities. Functions, similar to grammar are defined as JSON schema and can be passed as part of the parameters to the Messages API.
+
+Functions, similar to grammar are defined as JSON schema and can be passed as part of the parameters to the Messages API.
+
+```json
+curl localhost:3000/v1/chat/completions \
+ -X POST \
+ -H 'Content-Type: application/json' \
+ -d '{
+ "model": "tgi",
+ "messages": [
+ {
+ "role": "user",
+ "content": "What is the weather like in New York?"
+ }
+ ],
+ "tools": [
+ {
+ "type": "function",
+ "function": {
+ "name": "get_current_weather",
+ "description": "Get the current weather",
+ "parameters": {
+ "type": "object",
+ "properties": {
+ "location": {
+ "type": "string",
+ "description": "The city and state, e.g. San Francisco, CA"
+ },
+ "format": {
+ "type": "string",
+ "enum": ["celsius", "fahrenheit"],
+ "description": "The temperature unit to use. Infer this from the users location."
+ }
+ },
+ "required": ["location", "format"]
+ }
+ }
+ }
+ ],
+ "tool_choice": "get_current_weather"
+}'
+// {"id":"","object":"text_completion","created":1709051640,"model":"HuggingFaceH4/zephyr-7b-beta","system_fingerprint":"1.4.3-native","choices":[{"index":0,"message":{"role":"assistant","tool_calls":{"id":0,"type":"function","function":{"description":null,"name":"tools","parameters":{"format":"celsius","location":"New York"}}}},"logprobs":null,"finish_reason":"eos_token"}],"usage":{"prompt_tokens":157,"completion_tokens":19,"total_tokens":176}}
+```
+
+### Chat Completion with Tools
+
+Grammars are supported in the `/generate` endpoint, while tools are supported in the `/chat/completions` endpoint. Here's an example of how to use the client to send a request with a tool parameter.
+
+```python
+from huggingface_hub import InferenceClient
+
+client = InferenceClient("http://localhost:3000")
+
+tools = [
+ {
+ "type": "function",
+ "function": {
+ "name": "get_current_weather",
+ "description": "Get the current weather",
+ "parameters": {
+ "type": "object",
+ "properties": {
+ "location": {
+ "type": "string",
+ "description": "The city and state, e.g. San Francisco, CA",
+ },
+ "format": {
+ "type": "string",
+ "enum": ["celsius", "fahrenheit"],
+ "description": "The temperature unit to use. Infer this from the users location.",
+ },
+ },
+ "required": ["location", "format"],
+ },
+ },
+ },
+ {
+ "type": "function",
+ "function": {
+ "name": "get_n_day_weather_forecast",
+ "description": "Get an N-day weather forecast",
+ "parameters": {
+ "type": "object",
+ "properties": {
+ "location": {
+ "type": "string",
+ "description": "The city and state, e.g. San Francisco, CA",
+ },
+ "format": {
+ "type": "string",
+ "enum": ["celsius", "fahrenheit"],
+ "description": "The temperature unit to use. Infer this from the users location.",
+ },
+ "num_days": {
+ "type": "integer",
+ "description": "The number of days to forecast",
+ },
+ },
+ "required": ["location", "format", "num_days"],
+ },
+ },
+ },
+]
+
+chat = client.chat_completion(
+ messages=[
+ {
+ "role": "system",
+ "content": "You're a helpful assistant! Answer the users question best you can.",
+ },
+ {
+ "role": "user",
+ "content": "What is the weather like in Brooklyn, New York?",
+ },
+ ],
+ tools=tools,
+ seed=42,
+ max_tokens=100,
+)
+
+print(chat.choices[0].message.tool_calls)
+# [ChatCompletionOutputToolCall(function=ChatCompletionOutputFunctionDefinition(arguments={'format': 'fahrenheit', 'location': 'Brooklyn, New York', 'num_days': 7}, name='get_n_day_weather_forecast', description=None), id=0, type='function')]
+
+```
+
+### OpenAI integration
+
+TGI exposes an OpenAI-compatible API, which means you can use OpenAI's client libraries to interact with TGI's Messages API and Tool functions.
+
+However there are some minor differences in the API, for example `tool_choice="auto"` will ALWAYS choose the tool for you. This is different from OpenAI's API where `tool_choice="auto"` will choose a tool if the model thinks it's necessary.
+
+```python
+from openai import OpenAI
+
+# Initialize the client, pointing it to one of the available models
+client = OpenAI(
+ base_url="http://localhost:3000/v1",
+ api_key="_",
+)
+
+# NOTE: tools defined above and removed for brevity
+
+chat_completion = client.chat.completions.create(
+ model="tgi",
+ messages=[
+ {
+ "role": "system",
+ "content": "Don't make assumptions about what values to plug into functions. Ask for clarification if a user request is ambiguous.",
+ },
+ {
+ "role": "user",
+ "content": "What's the weather like the next 3 days in San Francisco, CA?",
+ },
+ ],
+ tools=tools,
+ tool_choice="auto", # tool selected by model
+ max_tokens=500,
+)
+
+
+called = chat_completion.choices[0].message.tool_calls
+print(called)
+# {
+# "id": 0,
+# "type": "function",
+# "function": {
+# "description": None,
+# "name": "tools",
+# "parameters": {
+# "format": "celsius",
+# "location": "San Francisco, CA",
+# "num_days": 3,
+# },
+# },
+# }
+```
diff --git a/docs/source/basic_tutorials/visual_language_models.md b/docs/source/basic_tutorials/visual_language_models.md
new file mode 100644
index 00000000..3770db0b
--- /dev/null
+++ b/docs/source/basic_tutorials/visual_language_models.md
@@ -0,0 +1,230 @@
+# Vision Language Model Inference in TGI
+
+Visual Language Model (VLM) are models that consume both image and text inputs to generate text.
+
+VLM's are trained on a combination of image and text data and can handle a wide range of tasks, such as image captioning, visual question answering, and visual dialog.
+
+> What distinguishes VLMs from other text and image models is their ability to handle long context and generate text that is coherent and relevant to the image even after multiple turns or in some cases, multiple images.
+
+Below are couple of common use cases for vision language models:
+
+- **Image Captioning**: Given an image, generate a caption that describes the image.
+- **Visual Question Answering (VQA)**: Given an image and a question about the image, generate an answer to the question.
+- **Mulimodal Dialog**: Generate response to multiple turns of images and conversations.
+- **Image Information Retrieval**: Given an image, retrieve information from the image.
+
+## How to Use a Vision Language Model?
+
+### Hugging Face Hub Python Library
+
+To infer with vision language models through Python, you can use the [`huggingface_hub`](https://pypi.org/project/huggingface-hub/) library. The `InferenceClient` class provides a simple way to interact with the [Inference API](https://huggingface.co/docs/api-inference/index). Images can be passed as URLs or base64-encoded strings. The `InferenceClient` will automatically detect the image format.
+
+```python
+from huggingface_hub import InferenceClient
+
+client = InferenceClient("http://127.0.0.1:3000")
+image = "https://huggingface.co/datasets/huggingface/documentation-images/resolve/main/transformers/rabbit.png"
+prompt = f"What is this a picture of?\n\n"
+for token in client.text_generation(prompt, max_new_tokens=16, stream=True):
+ print(token)
+
+# This is a picture of an anthropomorphic rabbit in a space suit.
+```
+
+```python
+from huggingface_hub import InferenceClient
+import base64
+import requests
+import io
+
+client = InferenceClient("http://127.0.0.1:3000")
+
+# read image from local file
+image_path = "rabbit.png"
+with open(image_path, "rb") as f:
+ image = base64.b64encode(f.read()).decode("utf-8")
+
+image = f"data:image/png;base64,{image}"
+prompt = f"What is this a picture of?\n\n"
+
+for token in client.text_generation(prompt, max_new_tokens=10, stream=True):
+ print(token)
+
+# This is a picture of an anthropomorphic rabbit in a space suit.
+```
+
+or via the `chat_completion` endpoint:
+
+```python
+from huggingface_hub import InferenceClient
+
+client = InferenceClient("http://127.0.0.1:3000")
+
+chat = client.chat_completion(
+ messages=[
+ {
+ "role": "user",
+ "content": [
+ {"type": "text", "text": "Whats in this image?"},
+ {
+ "type": "image_url",
+ "image_url": {
+ "url": "https://huggingface.co/datasets/huggingface/documentation-images/resolve/main/transformers/rabbit.png"
+ },
+ },
+ ],
+ },
+ ],
+ seed=42,
+ max_tokens=100,
+)
+
+print(chat)
+# ChatCompletionOutput(choices=[ChatCompletionOutputComplete(finish_reason='length', index=0, message=ChatCompletionOutputMessage(role='assistant', content=" The image you've provided features an anthropomorphic rabbit in spacesuit attire. This rabbit is depicted with human-like posture and movement, standing on a rocky terrain with a vast, reddish-brown landscape in the background. The spacesuit is detailed with mission patches, circuitry, and a helmet that covers the rabbit's face and ear, with an illuminated red light on the chest area.\n\nThe artwork style is that of a", name=None, tool_calls=None), logprobs=None)], created=1714589614, id='', model='llava-hf/llava-v1.6-mistral-7b-hf', object='text_completion', system_fingerprint='2.0.2-native', usage=ChatCompletionOutputUsage(completion_tokens=100, prompt_tokens=2943, total_tokens=3043))
+
+```
+
+or with OpenAi's library:
+
+```python
+from openai import OpenAI
+
+# init the client but point it to TGI
+client = OpenAI(base_url="http://localhost:3000/v1", api_key="-")
+
+chat_completion = client.chat.completions.create(
+ model="tgi",
+ messages=[
+ {
+ "role": "user",
+ "content": [
+ {"type": "text", "text": "Whats in this image?"},
+ {
+ "type": "image_url",
+ "image_url": {
+ "url": "https://huggingface.co/datasets/huggingface/documentation-images/resolve/main/transformers/rabbit.png"
+ },
+ },
+ ],
+ },
+ ],
+ stream=False,
+)
+
+print(chat_completion)
+# ChatCompletion(id='', choices=[Choice(finish_reason='eos_token', index=0, logprobs=None, message=ChatCompletionMessage(content=' The image depicts an anthropomorphic rabbit dressed in a space suit with gear that resembles NASA attire. The setting appears to be a solar eclipse with dramatic mountain peaks and a partial celestial body in the sky. The artwork is detailed and vivid, with a warm color palette and a sense of an adventurous bunny exploring or preparing for a journey beyond Earth. ', role='assistant', function_call=None, tool_calls=None))], created=1714589732, model='llava-hf/llava-v1.6-mistral-7b-hf', object='text_completion', system_fingerprint='2.0.2-native', usage=CompletionUsage(completion_tokens=84, prompt_tokens=2943, total_tokens=3027))
+```
+
+### Inference Through Sending `cURL` Requests
+
+To use the `generate_stream` endpoint with curl, you can add the `-N` flag. This flag disables curl default buffering and shows data as it arrives from the server.
+
+```bash
+curl -N 127.0.0.1:3000/generate_stream \
+ -X POST \
+ -d '{"inputs":"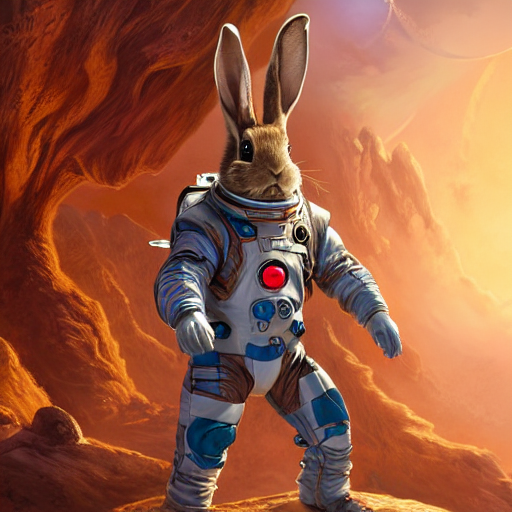What is this a picture of?\n\n","parameters":{"max_new_tokens":16, "seed": 42}}' \
+ -H 'Content-Type: application/json'
+
+# ...
+# data:{"index":16,"token":{"id":28723,"text":".","logprob":-0.6196289,"special":false},"generated_text":"This is a picture of an anthropomorphic rabbit in a space suit.","details":null}
+```
+
+### Inference Through JavaScript
+
+First, we need to install the `@huggingface/inference` library.
+
+```bash
+npm install @huggingface/inference
+```
+
+If you're using the free Inference API, you can use [Huggingface.js](https://huggingface.co/docs/huggingface.js/inference/README)'s `HfInference`. If you're using inference endpoints, you can use `HfInferenceEndpoint` class to easily interact with the Inference API.
+
+We can create a `HfInferenceEndpoint` providing our endpoint URL and We can create a `HfInferenceEndpoint` providing our endpoint URL and [Hugging Face access token](https://huggingface.co/settings/tokens).
+
+```js
+import { HfInferenceEndpoint } from "@huggingface/inference";
+
+const hf = new HfInferenceEndpoint("http://127.0.0.1:3000", "HF_TOKEN");
+
+const prompt =
+ "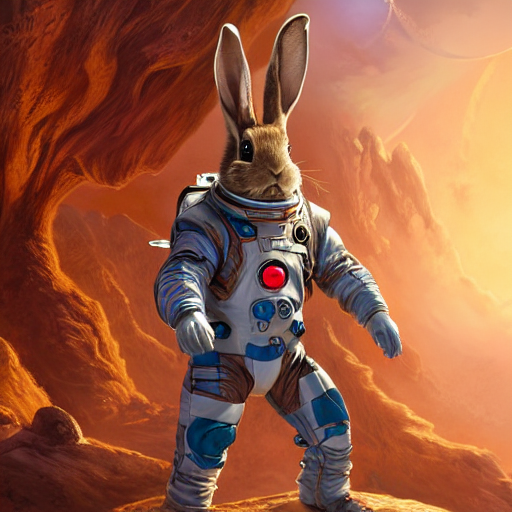What is this a picture of?\n\n";
+
+const stream = hf.textGenerationStream({
+ inputs: prompt,
+ parameters: { max_new_tokens: 16, seed: 42 },
+});
+for await (const r of stream) {
+ // yield the generated token
+ process.stdout.write(r.token.text);
+}
+
+// This is a picture of an anthropomorphic rabbit in a space suit.
+```
+
+## Combining Vision Language Models with Other Features
+
+VLMs in TGI have several advantages, for example these models can be used in tandem with other features for more complex tasks. For example, you can use VLMs with [Guided Generation](/docs/conceptual/guided-generation) to generate specific JSON data from an image.
+
+
+
+
+
+For example we can extract information from the rabbit image and generate a JSON object with the location, activity, number of animals seen, and the animals seen. That would look like this:
+
+```json
+{
+ "activity": "Standing",
+ "animals": ["Rabbit"],
+ "animals_seen": 1,
+ "location": "Rocky surface with mountains in the background and a red light on the rabbit's chest"
+}
+```
+
+All we need to do is provide a JSON schema to the VLM model and it will generate the JSON object for us.
+
+```bash
+curl localhost:3000/generate \
+ -X POST \
+ -H 'Content-Type: application/json' \
+ -d '{
+ "inputs":"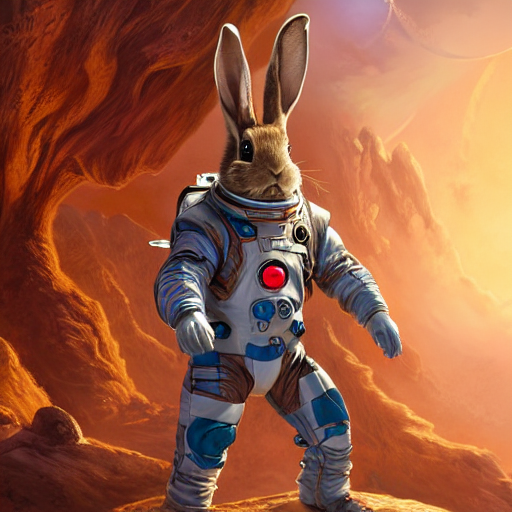What is this a picture of?\n\n",
+ "parameters": {
+ "max_new_tokens": 100,
+ "seed": 42,
+ "grammar": {
+ "type": "json",
+ "value": {
+ "properties": {
+ "location": {
+ "type": "string"
+ },
+ "activity": {
+ "type": "string"
+ },
+ "animals_seen": {
+ "type": "integer",
+ "minimum": 1,
+ "maximum": 5
+ },
+ "animals": {
+ "type": "array",
+ "items": {
+ "type": "string"
+ }
+ }
+ },
+ "required": ["location", "activity", "animals_seen", "animals"]
+ }
+ }
+ }
+}'
+
+# {
+# "generated_text": "{ \"activity\": \"Standing\", \"animals\": [ \"Rabbit\" ], \"animals_seen\": 1, \"location\": \"Rocky surface with mountains in the background and a red light on the rabbit's chest\" }"
+# }
+```
+
+Want to learn more about how Vision Language Models work? Check out the [awesome blog post on the topic](https://huggingface.co/blog/vlms).
diff --git a/docs/source/conceptual/flash_attention.md b/docs/source/conceptual/flash_attention.md
new file mode 100644
index 00000000..6b13cd13
--- /dev/null
+++ b/docs/source/conceptual/flash_attention.md
@@ -0,0 +1,11 @@
+# Flash Attention
+
+Scaling the transformer architecture is heavily bottlenecked by the self-attention mechanism, which has quadratic time and memory complexity. Recent developments in accelerator hardware mainly focus on enhancing compute capacities and not memory and transferring data between hardware. This results in attention operation having a memory bottleneck. **Flash Attention** is an attention algorithm used to reduce this problem and scale transformer-based models more efficiently, enabling faster training and inference.
+
+Standard attention mechanism uses High Bandwidth Memory (HBM) to store, read and write keys, queries and values. HBM is large in memory, but slow in processing, meanwhile SRAM is smaller in memory, but faster in operations. In the standard attention implementation, the cost of loading and writing keys, queries, and values from HBM is high. It loads keys, queries, and values from HBM to GPU on-chip SRAM, performs a single step of the attention mechanism, writes it back to HBM, and repeats this for every single attention step. Instead, Flash Attention loads keys, queries, and values once, fuses the operations of the attention mechanism, and writes them back.
+
+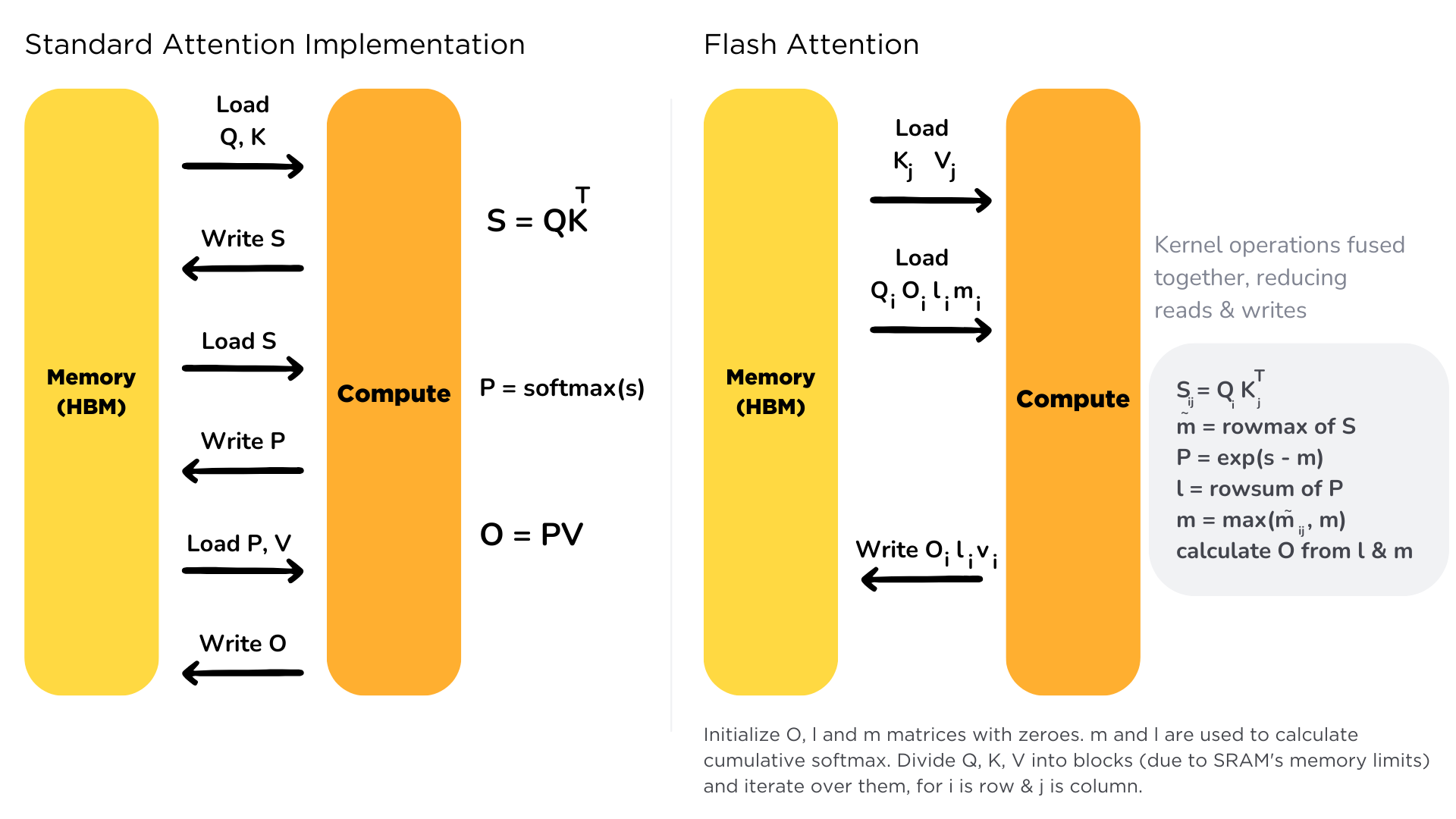
+
+It is implemented for supported models. You can check out the complete list of models that support Flash Attention [here](https://github.com/huggingface/text-generation-inference/tree/main/server/text_generation_server/models), for models with flash prefix.
+
+You can learn more about Flash Attention by reading the paper in this [link](https://arxiv.org/abs/2205.14135).
diff --git a/docs/source/conceptual/guidance.md b/docs/source/conceptual/guidance.md
new file mode 100644
index 00000000..3059e3de
--- /dev/null
+++ b/docs/source/conceptual/guidance.md
@@ -0,0 +1,86 @@
+# Guidance
+
+## What is Guidance?
+
+Guidance is a feature that allows users to constrain the generation of a large language model with a specified grammar. This feature is particularly useful when you want to generate text that follows a specific structure or uses a specific set of words or produce output in a specific format. A prominent example is JSON grammar, where the model is forced to output valid JSON.
+
+## How is it used?
+
+Guidance can be implemented in many ways and the community is always finding new ways to use it. Here are some examples of how you can use guidance:
+
+Technically, guidance can be used to generate:
+
+- a specific JSON object
+- a function signature
+- typed output like a list of integers
+
+However these use cases can span a wide range of applications, such as:
+
+- extracting structured data from unstructured text
+- summarizing text into a specific format
+- limit output to specific classes of words (act as a LLM powered classifier)
+- generate the input to specific APIs or services
+- provide reliable and consistent output for downstream tasks
+- extract data from multimodal inputs
+
+## How it works?
+
+Diving into the details, guidance is enabled by including a grammar with a generation request that is compiled, and used to modify the chosen tokens.
+
+This process can be broken down into the following steps:
+
+1. A request is sent to the backend, it is processed and placed in batch. Processing includes compiling the grammar into a finite state machine and a grammar state.
+
+
+
+
+
+
+2. The model does a forward pass over the batch. This returns probabilities for each token in the vocabulary for each request in the batch.
+
+3. The process of choosing one of those tokens is called `sampling`. The model samples from the distribution of probabilities to choose the next token. In TGI all of the steps before sampling are called `processor`. Grammars are applied as a processor that masks out tokens that are not allowed by the grammar.
+
+
+
+
+
+
+4. The grammar mask is applied and the model samples from the remaining tokens. Once a token is chosen, we update the grammar state with the new token, to prepare it for the next pass.
+
+
+
+
+
+
+## How to use Guidance?
+
+There are two main ways to use guidance; you can either use the `/generate` endpoint with a grammar or use the `/chat/completion` endpoint with tools.
+
+Under the hood tools are a special case of grammars that allows the model to choose one or none of the provided tools.
+
+Please refer to [using guidance](../basic_tutorials/using_guidance) for more examples and details on how to use guidance in Python, JavaScript, and cURL.
+
+### Getting the most out of guidance
+
+Depending on how you are using guidance, you may want to make use of different features. Here are some tips to get the most out of guidance:
+
+- If you are using the `/generate` with a `grammar` it is recommended to include the grammar in the prompt prefixed by something like `Please use the following JSON schema to generate the output:`. This will help the model understand the context of the grammar and generate the output accordingly.
+- If you are getting a response with many repeated tokens, please use the `frequency_penalty` or `repetition_penalty` to reduce the number of repeated tokens in the output.
diff --git a/docs/source/conceptual/paged_attention.md b/docs/source/conceptual/paged_attention.md
new file mode 100644
index 00000000..3fb2dcd8
--- /dev/null
+++ b/docs/source/conceptual/paged_attention.md
@@ -0,0 +1,9 @@
+# PagedAttention
+
+LLMs struggle with memory limitations during generation. In the decoding part of generation, all the attention keys and values generated for previous tokens are stored in GPU memory for reuse. This is called _KV cache_, and it may take up a large amount of memory for large models and long sequences.
+
+PagedAttention attempts to optimize memory use by partitioning the KV cache into blocks that are accessed through a lookup table. Thus, the KV cache does not need to be stored in contiguous memory, and blocks are allocated as needed. The memory efficiency can increase GPU utilization on memory-bound workloads, so more inference batches can be supported.
+
+The use of a lookup table to access the memory blocks can also help with KV sharing across multiple generations. This is helpful for techniques such as _parallel sampling_, where multiple outputs are generated simultaneously for the same prompt. In this case, the cached KV blocks can be shared among the generations.
+
+TGI's PagedAttention implementation leverages the custom cuda kernels developed by the [vLLM Project](https://github.com/vllm-project/vllm). You can learn more about this technique in the [project's page](https://vllm.ai/).
diff --git a/docs/source/conceptual/quantization.md b/docs/source/conceptual/quantization.md
new file mode 100644
index 00000000..8f26fdba
--- /dev/null
+++ b/docs/source/conceptual/quantization.md
@@ -0,0 +1,59 @@
+# Quantization
+
+TGI offers GPTQ and bits-and-bytes quantization to quantize large language models.
+
+## Quantization with GPTQ
+
+GPTQ is a post-training quantization method to make the model smaller. It quantizes the layers by finding a compressed version of that weight, that will yield a minimum mean squared error like below 👇
+
+Given a layer \\(l\\) with weight matrix \\(W_{l}\\) and layer input \\(X_{l}\\), find quantized weight \\(\\hat{W}_{l}\\):
+
+$$({\hat{W}_{l}}^{*} = argmin_{\hat{W_{l}}} ||W_{l}X-\hat{W}_{l}X||^{2}_{2})$$
+
+
+TGI allows you to both run an already GPTQ quantized model (see available models [here](https://huggingface.co/models?search=gptq)) or quantize a model of your choice using quantization script. You can run a quantized model by simply passing --quantize like below 👇
+
+```bash
+docker run --gpus all --shm-size 1g -p 8080:80 -v $volume:/data ghcr.io/huggingface/text-generation-inference:latest --model-id $model --quantize gptq
+```
+
+Note that TGI's GPTQ implementation doesn't use [AutoGPTQ](https://github.com/PanQiWei/AutoGPTQ) under the hood. However, models quantized using AutoGPTQ or Optimum can still be served by TGI.
+
+To quantize a given model using GPTQ with a calibration dataset, simply run
+
+```bash
+text-generation-server quantize tiiuae/falcon-40b /data/falcon-40b-gptq
+# Add --upload-to-model-id MYUSERNAME/falcon-40b to push the created model to the hub directly
+```
+
+This will create a new directory with the quantized files which you can use with,
+
+```bash
+text-generation-launcher --model-id /data/falcon-40b-gptq/ --sharded true --num-shard 2 --quantize gptq
+```
+
+You can learn more about the quantization options by running `text-generation-server quantize --help`.
+
+If you wish to do more with GPTQ models (e.g. train an adapter on top), you can read about transformers GPTQ integration [here](https://huggingface.co/blog/gptq-integration).
+You can learn more about GPTQ from the [paper](https://arxiv.org/pdf/2210.17323.pdf).
+
+## Quantization with bitsandbytes
+
+bitsandbytes is a library used to apply 8-bit and 4-bit quantization to models. Unlike GPTQ quantization, bitsandbytes doesn't require a calibration dataset or any post-processing – weights are automatically quantized on load. However, inference with bitsandbytes is slower than GPTQ or FP16 precision.
+
+8-bit quantization enables multi-billion parameter scale models to fit in smaller hardware without degrading performance too much.
+In TGI, you can use 8-bit quantization by adding `--quantize bitsandbytes` like below 👇
+
+```bash
+docker run --gpus all --shm-size 1g -p 8080:80 -v $volume:/data ghcr.io/huggingface/text-generation-inference:latest --model-id $model --quantize bitsandbytes
+```
+
+4-bit quantization is also possible with bitsandbytes. You can choose one of the following 4-bit data types: 4-bit float (`fp4`), or 4-bit `NormalFloat` (`nf4`). These data types were introduced in the context of parameter-efficient fine-tuning, but you can apply them for inference by automatically converting the model weights on load.
+
+In TGI, you can use 4-bit quantization by adding `--quantize bitsandbytes-nf4` or `--quantize bitsandbytes-fp4` like below 👇
+
+```bash
+docker run --gpus all --shm-size 1g -p 8080:80 -v $volume:/data ghcr.io/huggingface/text-generation-inference:latest --model-id $model --quantize bitsandbytes-nf4
+```
+
+You can get more information about 8-bit quantization by reading this [blog post](https://huggingface.co/blog/hf-bitsandbytes-integration), and 4-bit quantization by reading [this blog post](https://huggingface.co/blog/4bit-transformers-bitsandbytes).
diff --git a/docs/source/conceptual/safetensors.md b/docs/source/conceptual/safetensors.md
new file mode 100644
index 00000000..8ede20fe
--- /dev/null
+++ b/docs/source/conceptual/safetensors.md
@@ -0,0 +1,7 @@
+# Safetensors
+
+Safetensors is a model serialization format for deep learning models. It is [faster](https://huggingface.co/docs/safetensors/speed) and safer compared to other serialization formats like pickle (which is used under the hood in many deep learning libraries).
+
+TGI depends on safetensors format mainly to enable [tensor parallelism sharding](./tensor_parallelism). For a given model repository during serving, TGI looks for safetensors weights. If there are no safetensors weights, TGI converts the PyTorch weights to safetensors format.
+
+You can learn more about safetensors by reading the [safetensors documentation](https://huggingface.co/docs/safetensors/index).
diff --git a/docs/source/conceptual/speculation.md b/docs/source/conceptual/speculation.md
new file mode 100644
index 00000000..45618ae3
--- /dev/null
+++ b/docs/source/conceptual/speculation.md
@@ -0,0 +1,49 @@
+## Speculation
+
+
+Speculative decoding, assisted generation, Medusa, and others are a few different names for the same idea.
+The idea is to generate tokens *before* the large model actually runs, and only *check* if those tokens where valid.
+
+So you are making *more* computations on your LLM, but if you are correct you produce 1, 2, 3 etc.. tokens on a single LLM pass. Since LLMs are usually memory bound (and not compute bound), provided your guesses are correct enough, this is a 2-3x faster inference (It can be much more for code oriented tasks for instance).
+
+You can check a more [detailed explanation](https://huggingface.co/blog/assisted-generation).
+
+Text-generation inference supports 2 main speculative methods:
+
+- Medusa
+- N-gram
+
+
+### Medusa
+
+
+Medusa is a [simple method](https://arxiv.org/abs/2401.10774) to create many tokens in a single pass using fine-tuned LM heads in addition to your existing models.
+
+
+You can check a few existing fine-tunes for popular models:
+
+- [text-generation-inference/gemma-7b-it-medusa](https://huggingface.co/text-generation-inference/gemma-7b-it-medusa)
+- [text-generation-inference/Mixtral-8x7B-Instruct-v0.1-medusa](https://huggingface.co/text-generation-inference/Mixtral-8x7B-Instruct-v0.1-medusa)
+- [text-generation-inference/Mistral-7B-Instruct-v0.2-medusa](https://huggingface.co/text-generation-inference/Mistral-7B-Instruct-v0.2-medusa)
+
+
+In order to create your own medusa heads for your own finetune, you should check own the original medusa repo. [../basic_tutorials/train_medusa.md](../basic_tutorials/train_medusa.md)
+
+
+In order to use medusa models in TGI, simply point to a medusa enabled model, and everything will load automatically.
+
+
+### N-gram
+
+
+If you don't have a medusa model, or don't have the resource to fine-tune, you can try to use `n-gram`.
+N-gram works by trying to find matching tokens in the previous sequence, and use those as speculation for generating new tokens. For example, if the tokens "np.mean" appear multiple times in the sequence, the model can speculate that the next continuation of the tokens "np." is probably also "mean".
+
+This is an extremely simple method, which works best for code, or highly repetitive text. This might not be beneficial, if the speculation misses too much.
+
+
+In order to enable n-gram speculation simply use
+
+`--speculate 2` in your flags.
+
+[Details about the flag](https://huggingface.co/docs/text-generation-inference/basic_tutorials/launcher#speculate)
diff --git a/docs/source/conceptual/streaming.md b/docs/source/conceptual/streaming.md
new file mode 100644
index 00000000..71ec9b25
--- /dev/null
+++ b/docs/source/conceptual/streaming.md
@@ -0,0 +1,146 @@
+# Streaming
+
+## What is Streaming?
+
+Token streaming is the mode in which the server returns the tokens one by one as the model generates them. This enables showing progressive generations to the user rather than waiting for the whole generation. Streaming is an essential aspect of the end-user experience as it reduces latency, one of the most critical aspects of a smooth experience.
+
+
+
+
+
+
+With token streaming, the server can start returning the tokens one by one before having to generate the whole response. Users can have a sense of the generation's quality before the end of the generation. This has different positive effects:
+
+* Users can get results orders of magnitude earlier for extremely long queries.
+* Seeing something in progress allows users to stop the generation if it's not going in the direction they expect.
+* Perceived latency is lower when results are shown in the early stages.
+* When used in conversational UIs, the experience feels more natural.
+
+For example, a system can generate 100 tokens per second. If the system generates 1000 tokens, with the non-streaming setup, users need to wait 10 seconds to get results. On the other hand, with the streaming setup, users get initial results immediately, and although end-to-end latency will be the same, they can see half of the generation after five seconds. Below you can see an interactive demo that shows non-streaming vs streaming side-by-side. Click **generate** below.
+
+