diff --git a/.dev_scripts/github/update_model_index.py b/.dev_scripts/github/update_model_index.py
index 3c24055060..f6721f7790 100755
--- a/.dev_scripts/github/update_model_index.py
+++ b/.dev_scripts/github/update_model_index.py
@@ -151,6 +151,7 @@ def parse_config_path(path):
'3d_kpt_mview_rgb_img': '3D Keypoint',
'3d_kpt_sview_rgb_vid': '3D Keypoint',
'3d_mesh_sview_rgb_img': '3D Mesh',
+ 'gesture_sview_rgbd_vid': 'Gesture',
None: None
}
task_readable = task2readable.get(task)
diff --git a/configs/_base_/datasets/nvgesture.py b/configs/_base_/datasets/nvgesture.py
new file mode 100644
index 0000000000..7d5a3df7b9
--- /dev/null
+++ b/configs/_base_/datasets/nvgesture.py
@@ -0,0 +1,42 @@
+dataset_info = dict(
+ dataset_name='nvgesture',
+ paper_info=dict(
+ author='Pavlo Molchanov and Xiaodong Yang and Shalini Gupta '
+ 'and Kihwan Kim and Stephen Tyree and Jan Kautz',
+ title='Online Detection and Classification of Dynamic Hand Gestures '
+ 'with Recurrent 3D Convolutional Neural Networks',
+ container='Proceedings of the IEEE Conference on '
+ 'Computer Vision and Pattern Recognition',
+ year='2016',
+ homepage='https://research.nvidia.com/publication/2016-06_online-'
+ 'detection-and-classification-dynamic-hand-gestures-recurrent-3d',
+ ),
+ category_info={
+ 0: 'five fingers move right',
+ 1: 'five fingers move left',
+ 2: 'five fingers move up',
+ 3: 'five fingers move down',
+ 4: 'two fingers move right',
+ 5: 'two fingers move left',
+ 6: 'two fingers move up',
+ 7: 'two fingers move down',
+ 8: 'click',
+ 9: 'beckoned',
+ 10: 'stretch hand',
+ 11: 'shake hand',
+ 12: 'one',
+ 13: 'two',
+ 14: 'three',
+ 15: 'lift up',
+ 16: 'press down',
+ 17: 'push',
+ 18: 'shrink',
+ 19: 'levorotation',
+ 20: 'dextrorotation',
+ 21: 'two fingers prod',
+ 22: 'grab',
+ 23: 'thumbs up',
+ 24: 'OK'
+ },
+ flip_pairs=[(0, 1), (4, 5), (19, 20)],
+ fps=30)
diff --git a/configs/hand/gesture_sview_rgbd_vid/README.md b/configs/hand/gesture_sview_rgbd_vid/README.md
new file mode 100644
index 0000000000..fb5ce51f9a
--- /dev/null
+++ b/configs/hand/gesture_sview_rgbd_vid/README.md
@@ -0,0 +1,7 @@
+# Gesture Recognition
+
+Gesture recognition aims to recognize the hand gestures in the video, such as thumbs up.
+
+## Data preparation
+
+Please follow [DATA Preparation](/docs/en/tasks/2d_hand_gesture.md) to prepare data.
diff --git a/configs/hand/gesture_sview_rgbd_vid/mtut/README.md b/configs/hand/gesture_sview_rgbd_vid/mtut/README.md
new file mode 100644
index 0000000000..80e0e8f0b0
--- /dev/null
+++ b/configs/hand/gesture_sview_rgbd_vid/mtut/README.md
@@ -0,0 +1,8 @@
+# Multi-modal Training and Uni-modal Testing (MTUT) for gesture recognition
+
+MTUT method uses multi-modal data in the training phase, such as RGB videos and depth videos.
+For each modality, an I3D network is trained to conduct gesture recognition. The property
+of spatial-temporal semantic alignment across multi-modal data is utilized to supervise the
+learning, in order to improve the performance of each I3D network for a single modality.
+
+In the testing phase, uni-modal data, generally RGB video, is used.
diff --git a/configs/hand/gesture_sview_rgbd_vid/mtut/nvgesture/i3d_nvgesture.md b/configs/hand/gesture_sview_rgbd_vid/mtut/nvgesture/i3d_nvgesture.md
new file mode 100644
index 0000000000..297c6b95c6
--- /dev/null
+++ b/configs/hand/gesture_sview_rgbd_vid/mtut/nvgesture/i3d_nvgesture.md
@@ -0,0 +1,60 @@
+
+
+
+MTUT (CVPR'2019)
+
+```bibtex
+@InProceedings{Abavisani_2019_CVPR,
+ author = {Abavisani, Mahdi and Joze, Hamid Reza Vaezi and Patel, Vishal M.},
+ title = {Improving the Performance of Unimodal Dynamic Hand-Gesture Recognition With Multimodal Training},
+ booktitle = {Proceedings of the IEEE/CVF Conference on Computer Vision and Pattern Recognition (CVPR)},
+ month = {June},
+ year = {2019}
+}
+```
+
+
+
+
+
+
+I3D (CVPR'2017)
+
+```bibtex
+@InProceedings{Carreira_2017_CVPR,
+ author = {Carreira, Joao and Zisserman, Andrew},
+ title = {Quo Vadis, Action Recognition? A New Model and the Kinetics Dataset},
+ booktitle = {Proceedings of the IEEE Conference on Computer Vision and Pattern Recognition (CVPR)},
+ month = {July},
+ year = {2017}
+}
+```
+
+
+
+
+
+
+NVGesture (CVPR'2016)
+
+```bibtex
+@InProceedings{Molchanov_2016_CVPR,
+ author = {Molchanov, Pavlo and Yang, Xiaodong and Gupta, Shalini and Kim, Kihwan and Tyree, Stephen and Kautz, Jan},
+ title = {Online Detection and Classification of Dynamic Hand Gestures With Recurrent 3D Convolutional Neural Network},
+ booktitle = {Proceedings of the IEEE Conference on Computer Vision and Pattern Recognition (CVPR)},
+ month = {June},
+ year = {2016}
+}
+```
+
+
+
+Results on NVGesture test set
+
+| Arch | Input Size | fps | bbox | AP_rgb | AP_depth | ckpt | log |
+| :------------------------------------------------------ | :--------: | :-: | :-------: | :----: | :------: | :-----------------------------------------------------: | :----------------------------------------------------: |
+| [I3D+MTUT](/configs/hand/gesture_sview_rgbd_vid/mtut/nvgesture/i3d_nvgesture_bbox_112x112_fps15.py)$^\*$ | 112x112 | 15 | $\\surd$ | 0.725 | 0.730 | [ckpt](https://download.openmmlab.com/mmpose/gesture/mtut/i3d_nvgesture/i3d_nvgesture_bbox_112x112_fps15-363b5956_20220530.pth) | [log](https://download.openmmlab.com/mmpose/gesture/mtut/i3d_nvgesture/i3d_nvgesture_bbox_112x112_fps15-20220530.log.json) |
+| [I3D+MTUT](/configs/hand/gesture_sview_rgbd_vid/mtut/nvgesture/i3d_nvgesture_bbox_224x224_fps30.py) | 224x224 | 30 | $\\surd$ | 0.782 | 0.811 | [ckpt](https://download.openmmlab.com/mmpose/gesture/mtut/i3d_nvgesture/i3d_nvgesture_bbox_224x224_fps30-98a8f288_20220530.pthh) | [log](https://download.openmmlab.com/mmpose/gesture/mtut/i3d_nvgesture/i3d_nvgesture_bbox_224x224_fps30-20220530.log.json) |
+| [I3D+MTUT](/configs/hand/gesture_sview_rgbd_vid/mtut/nvgesture/i3d_nvgesture_224x224_fps30.py) | 224x224 | 30 | $\\times$ | 0.739 | 0.809 | [ckpt](https://download.openmmlab.com/mmpose/gesture/mtut/i3d_nvgesture/i3d_nvgesture_224x224_fps30-b7abf574_20220530.pth) | [log](https://download.openmmlab.com/mmpose/gesture/mtut/i3d_nvgesture/i3d_nvgesture_224x224_fps30-20220530.log.json) |
+
+$^\*$: MTUT supports multi-modal training and uni-modal testing. Model trained with this config can be used to recognize gestures in rgb videos with [inference config](/configs/hand/gesture_sview_rgbd_vid/mtut/nvgesture/i3d_nvgesture_bbox_112x112_fps15_rgb.py).
diff --git a/configs/hand/gesture_sview_rgbd_vid/mtut/nvgesture/i3d_nvgesture.yml b/configs/hand/gesture_sview_rgbd_vid/mtut/nvgesture/i3d_nvgesture.yml
new file mode 100644
index 0000000000..26e6f58f78
--- /dev/null
+++ b/configs/hand/gesture_sview_rgbd_vid/mtut/nvgesture/i3d_nvgesture.yml
@@ -0,0 +1,49 @@
+Collections:
+- Name: MTUT
+ Paper:
+ Title: Improving the Performance of Unimodal Dynamic Hand-Gesture Recognition
+ With Multimodal Training
+ URL: https://openaccess.thecvf.com/content_CVPR_2019/html/Abavisani_Improving_the_Performance_of_Unimodal_Dynamic_Hand-Gesture_Recognition_With_Multimodal_CVPR_2019_paper.html
+ README: https://github.com/open-mmlab/mmpose/blob/master/docs/en/papers/algorithms/mtut.md
+Models:
+- Config: configs/hand/gesture_sview_rgbd_vid/mtut/nvgesture/i3d_nvgesture_bbox_112x112_fps15.py
+ In Collection: MTUT
+ Metadata:
+ Architecture: &id001
+ - MTUT
+ - I3D
+ Training Data: NVGesture
+ Name: mtut_i3d_nvgesture_bbox_112x112_fps15
+ Results:
+ - Dataset: NVGesture
+ Metrics:
+ AP depth: 0.73
+ AP rgb: 0.725
+ Task: Hand Gesture
+ Weights: https://download.openmmlab.com/mmpose/gesture/mtut/i3d_nvgesture/i3d_nvgesture_bbox_112x112_fps15-363b5956_20220530.pth
+- Config: configs/hand/gesture_sview_rgbd_vid/mtut/nvgesture/i3d_nvgesture_bbox_224x224_fps30.py
+ In Collection: MTUT
+ Metadata:
+ Architecture: *id001
+ Training Data: NVGesture
+ Name: mtut_i3d_nvgesture_bbox_224x224_fps30
+ Results:
+ - Dataset: NVGesture
+ Metrics:
+ AP depth: 0.811
+ AP rgb: 0.782
+ Task: Hand Gesture
+ Weights: https://download.openmmlab.com/mmpose/gesture/mtut/i3d_nvgesture/i3d_nvgesture_bbox_224x224_fps30-98a8f288_20220530.pthh
+- Config: configs/hand/gesture_sview_rgbd_vid/mtut/nvgesture/i3d_nvgesture_224x224_fps30.py
+ In Collection: MTUT
+ Metadata:
+ Architecture: *id001
+ Training Data: NVGesture
+ Name: mtut_i3d_nvgesture_224x224_fps30
+ Results:
+ - Dataset: NVGesture
+ Metrics:
+ AP depth: 0.809
+ AP rgb: 0.739
+ Task: Hand Gesture
+ Weights: https://download.openmmlab.com/mmpose/gesture/mtut/i3d_nvgesture/i3d_nvgesture_224x224_fps30-b7abf574_20220530.pth
diff --git a/configs/hand/gesture_sview_rgbd_vid/mtut/nvgesture/i3d_nvgesture_224x224_fps30.py b/configs/hand/gesture_sview_rgbd_vid/mtut/nvgesture/i3d_nvgesture_224x224_fps30.py
new file mode 100644
index 0000000000..4c2e2b400f
--- /dev/null
+++ b/configs/hand/gesture_sview_rgbd_vid/mtut/nvgesture/i3d_nvgesture_224x224_fps30.py
@@ -0,0 +1,128 @@
+_base_ = [
+ '../../../../_base_/default_runtime.py',
+ '../../../../_base_/datasets/nvgesture.py'
+]
+
+checkpoint_config = dict(interval=5)
+evaluation = dict(interval=5, metric='AP', save_best='AP_rgb')
+
+optimizer = dict(
+ type='SGD',
+ lr=1e-2,
+ momentum=0.9,
+)
+optimizer_config = dict(grad_clip=None)
+# learning policy
+lr_config = dict(policy='step', gamma=0.1, step=[30, 50])
+total_epochs = 75
+log_config = dict(interval=10)
+
+custom_hooks_config = [dict(type='ModelSetEpochHook')]
+
+model = dict(
+ type='GestureRecognizer',
+ modality=['rgb', 'depth'],
+ pretrained=dict(
+ rgb='https://github.com/hassony2/kinetics_i3d_pytorch/'
+ 'raw/master/model/model_rgb.pth',
+ depth='https://github.com/hassony2/kinetics_i3d_pytorch/'
+ 'raw/master/model/model_rgb.pth',
+ ),
+ backbone=dict(
+ rgb=dict(
+ type='I3D',
+ in_channels=3,
+ expansion=1,
+ ),
+ depth=dict(
+ type='I3D',
+ in_channels=1,
+ expansion=1,
+ ),
+ ),
+ cls_head=dict(
+ type='MultiModalSSAHead',
+ num_classes=25,
+ ),
+ train_cfg=dict(
+ beta=2,
+ lambda_=5e-3,
+ ssa_start_epoch=61,
+ ),
+ test_cfg=dict(),
+)
+
+data_cfg = dict(
+ video_size=[320, 240],
+ modality=['rgb', 'depth'],
+)
+
+train_pipeline = [
+ dict(type='LoadVideoFromFile'),
+ dict(type='ModalWiseChannelProcess'),
+ dict(type='CropValidClip'),
+ dict(type='TemporalPooling', length=64, ref_fps=30),
+ dict(type='ResizeGivenShortEdge', length=256),
+ dict(type='RandomAlignedSpatialCrop', length=224),
+ dict(type='GestureRandomFlip'),
+ dict(type='MultiModalVideoToTensor'),
+ dict(
+ type='VideoNormalizeTensor',
+ mean=[0.485, 0.456, 0.406],
+ std=[0.229, 0.224, 0.225]),
+ dict(
+ type='Collect', keys=['video', 'label'], meta_keys=['fps',
+ 'modality']),
+]
+
+val_pipeline = [
+ dict(type='LoadVideoFromFile'),
+ dict(type='ModalWiseChannelProcess'),
+ dict(type='CropValidClip'),
+ dict(type='TemporalPooling', length=-1, ref_fps=30),
+ dict(type='ResizeGivenShortEdge', length=256),
+ dict(type='CenterSpatialCrop', length=224),
+ dict(type='MultiModalVideoToTensor'),
+ dict(
+ type='VideoNormalizeTensor',
+ mean=[0.485, 0.456, 0.406],
+ std=[0.229, 0.224, 0.225]),
+ dict(
+ type='Collect', keys=['video', 'label'], meta_keys=['fps',
+ 'modality']),
+]
+
+test_pipeline = val_pipeline
+
+data_root = 'data/nvgesture'
+data = dict(
+ samples_per_gpu=6,
+ workers_per_gpu=2,
+ val_dataloader=dict(samples_per_gpu=6),
+ test_dataloader=dict(samples_per_gpu=6),
+ train=dict(
+ type='NVGestureDataset',
+ ann_file=f'{data_root}/annotations/'
+ 'nvgesture_train_correct_cvpr2016_v2.lst',
+ vid_prefix=f'{data_root}/',
+ data_cfg=data_cfg,
+ pipeline=train_pipeline,
+ dataset_info={{_base_.dataset_info}}),
+ val=dict(
+ type='NVGestureDataset',
+ ann_file=f'{data_root}/annotations/'
+ 'nvgesture_test_correct_cvpr2016_v2.lst',
+ vid_prefix=f'{data_root}/',
+ data_cfg=data_cfg,
+ pipeline=val_pipeline,
+ test_mode=True,
+ dataset_info={{_base_.dataset_info}}),
+ test=dict(
+ type='NVGestureDataset',
+ ann_file=f'{data_root}/annotations/'
+ 'nvgesture_test_correct_cvpr2016_v2.lst',
+ vid_prefix=f'{data_root}/',
+ data_cfg=data_cfg,
+ pipeline=test_pipeline,
+ test_mode=True,
+ dataset_info={{_base_.dataset_info}}))
diff --git a/configs/hand/gesture_sview_rgbd_vid/mtut/nvgesture/i3d_nvgesture_bbox_112x112_fps15.py b/configs/hand/gesture_sview_rgbd_vid/mtut/nvgesture/i3d_nvgesture_bbox_112x112_fps15.py
new file mode 100644
index 0000000000..ae2fe2e960
--- /dev/null
+++ b/configs/hand/gesture_sview_rgbd_vid/mtut/nvgesture/i3d_nvgesture_bbox_112x112_fps15.py
@@ -0,0 +1,136 @@
+_base_ = [
+ '../../../../_base_/default_runtime.py',
+ '../../../../_base_/datasets/nvgesture.py'
+]
+
+checkpoint_config = dict(interval=5)
+evaluation = dict(interval=5, metric='AP', save_best='AP_rgb')
+
+optimizer = dict(
+ type='SGD',
+ lr=1e-1,
+ momentum=0.9,
+)
+optimizer_config = dict(grad_clip=None)
+# learning policy
+lr_config = dict(policy='step', gamma=0.1, step=[30, 60, 90, 110])
+total_epochs = 130
+log_config = dict(interval=10)
+
+custom_hooks_config = [dict(type='ModelSetEpochHook')]
+
+model = dict(
+ type='GestureRecognizer',
+ modality=['rgb', 'depth'],
+ pretrained=dict(
+ rgb='https://github.com/hassony2/kinetics_i3d_pytorch/'
+ 'raw/master/model/model_rgb.pth',
+ depth='https://github.com/hassony2/kinetics_i3d_pytorch/'
+ 'raw/master/model/model_rgb.pth',
+ ),
+ backbone=dict(
+ rgb=dict(
+ type='I3D',
+ in_channels=3,
+ expansion=1,
+ ),
+ depth=dict(
+ type='I3D',
+ in_channels=1,
+ expansion=1,
+ ),
+ ),
+ cls_head=dict(
+ type='MultiModalSSAHead',
+ num_classes=25,
+ avg_pool_kernel=(1, 2, 2),
+ ),
+ train_cfg=dict(
+ beta=2,
+ lambda_=1e-3,
+ ssa_start_epoch=111,
+ ),
+ test_cfg=dict(),
+)
+
+data_root = 'data/nvgesture'
+data_cfg = dict(
+ video_size=[320, 240],
+ modality=['rgb', 'depth'],
+ bbox_file=f'{data_root}/annotations/bboxes.json',
+)
+
+train_pipeline = [
+ dict(type='LoadVideoFromFile'),
+ dict(type='ModalWiseChannelProcess'),
+ dict(type='CropValidClip'),
+ dict(type='TemporalPooling', length=16, ref_fps=15),
+ dict(type='MultiFrameBBoxMerge'),
+ dict(
+ type='ResizedCropByBBox',
+ size=112,
+ scale=(0.8, 1.25),
+ ratio=(0.75, 1.33),
+ shift=0.3),
+ dict(type='GestureRandomFlip'),
+ dict(type='VideoColorJitter', brightness=0.4, contrast=0.3),
+ dict(type='MultiModalVideoToTensor'),
+ dict(
+ type='VideoNormalizeTensor',
+ mean=[0.485, 0.456, 0.406],
+ std=[0.229, 0.224, 0.225]),
+ dict(
+ type='Collect', keys=['video', 'label'], meta_keys=['fps',
+ 'modality']),
+]
+
+val_pipeline = [
+ dict(type='LoadVideoFromFile'),
+ dict(type='ModalWiseChannelProcess'),
+ dict(type='CropValidClip'),
+ dict(type='TemporalPooling', length=-1, ref_fps=15),
+ dict(type='MultiFrameBBoxMerge'),
+ dict(type='ResizedCropByBBox', size=112),
+ dict(type='MultiModalVideoToTensor'),
+ dict(
+ type='VideoNormalizeTensor',
+ mean=[0.485, 0.456, 0.406],
+ std=[0.229, 0.224, 0.225]),
+ dict(
+ type='Collect', keys=['video', 'label'], meta_keys=['fps',
+ 'modality']),
+]
+
+test_pipeline = val_pipeline
+
+data = dict(
+ samples_per_gpu=6,
+ workers_per_gpu=2,
+ val_dataloader=dict(samples_per_gpu=6),
+ test_dataloader=dict(samples_per_gpu=6),
+ train=dict(
+ type='NVGestureDataset',
+ ann_file=f'{data_root}/annotations/'
+ 'nvgesture_train_correct_cvpr2016_v2.lst',
+ vid_prefix=f'{data_root}/',
+ data_cfg=data_cfg,
+ pipeline=train_pipeline,
+ dataset_info={{_base_.dataset_info}}),
+ val=dict(
+ type='NVGestureDataset',
+ ann_file=f'{data_root}/annotations/'
+ 'nvgesture_test_correct_cvpr2016_v2.lst',
+ vid_prefix=f'{data_root}/',
+ data_cfg=data_cfg,
+ pipeline=val_pipeline,
+ test_mode=True,
+ dataset_info={{_base_.dataset_info}}),
+ test=dict(
+ type='NVGestureDataset',
+ ann_file=f'{data_root}/annotations/'
+ 'nvgesture_test_correct_cvpr2016_v2.lst',
+ vid_prefix=f'{data_root}/',
+ data_cfg=data_cfg,
+ pipeline=test_pipeline,
+ test_mode=True,
+ dataset_info={{_base_.dataset_info}}))
diff --git a/configs/hand/gesture_sview_rgbd_vid/mtut/nvgesture/i3d_nvgesture_bbox_112x112_fps15_rgb.py b/configs/hand/gesture_sview_rgbd_vid/mtut/nvgesture/i3d_nvgesture_bbox_112x112_fps15_rgb.py
new file mode 100644
index 0000000000..9777dda67e
--- /dev/null
+++ b/configs/hand/gesture_sview_rgbd_vid/mtut/nvgesture/i3d_nvgesture_bbox_112x112_fps15_rgb.py
@@ -0,0 +1,124 @@
+# Copyright (c) OpenMMLab. All rights reserved.
+_base_ = [
+ '../../../../_base_/default_runtime.py',
+ '../../../../_base_/datasets/nvgesture.py'
+]
+
+checkpoint_config = dict(interval=5)
+evaluation = dict(interval=5, metric='AP', save_best='AP_rgb')
+
+optimizer = dict(
+ type='SGD',
+ lr=1e-1,
+ momentum=0.9,
+)
+optimizer_config = dict(grad_clip=None)
+# learning policy
+lr_config = dict(policy='step', gamma=0.1, step=[30, 60, 90, 110])
+total_epochs = 130
+log_config = dict(interval=10)
+
+custom_hooks_config = [dict(type='ModelSetEpochHook')]
+
+model = dict(
+ type='GestureRecognizer',
+ modality=['rgb'],
+ backbone=dict(rgb=dict(
+ type='I3D',
+ in_channels=3,
+ expansion=1,
+ ), ),
+ cls_head=dict(
+ type='MultiModalSSAHead',
+ num_classes=25,
+ avg_pool_kernel=(1, 2, 2),
+ ),
+ train_cfg=dict(
+ beta=2,
+ lambda_=1e-3,
+ ssa_start_epoch=111,
+ ),
+ test_cfg=dict(),
+)
+
+data_root = 'data/nvgesture'
+data_cfg = dict(
+ video_size=[320, 240],
+ modality=['rgb'],
+ bbox_file=f'{data_root}/annotations/bboxes.json',
+)
+
+train_pipeline = [
+ dict(type='LoadVideoFromFile'),
+ dict(type='ModalWiseChannelProcess'),
+ dict(type='CropValidClip'),
+ dict(type='TemporalPooling', length=16, ref_fps=15),
+ dict(type='MultiFrameBBoxMerge'),
+ dict(
+ type='ResizedCropByBBox',
+ size=112,
+ scale=(0.8, 1.25),
+ ratio=(0.75, 1.33),
+ shift=0.3),
+ dict(type='GestureRandomFlip'),
+ dict(type='VideoColorJitter', brightness=0.4, contrast=0.3),
+ dict(type='MultiModalVideoToTensor'),
+ dict(
+ type='VideoNormalizeTensor',
+ mean=[0.485, 0.456, 0.406],
+ std=[0.229, 0.224, 0.225]),
+ dict(
+ type='Collect', keys=['video', 'label'], meta_keys=['fps',
+ 'modality']),
+]
+
+val_pipeline = [
+ dict(type='LoadVideoFromFile'),
+ dict(type='ModalWiseChannelProcess'),
+ dict(type='CropValidClip'),
+ dict(type='TemporalPooling', length=-1, ref_fps=15),
+ dict(type='MultiFrameBBoxMerge'),
+ dict(type='ResizedCropByBBox', size=112),
+ dict(type='MultiModalVideoToTensor'),
+ dict(
+ type='VideoNormalizeTensor',
+ mean=[0.485, 0.456, 0.406],
+ std=[0.229, 0.224, 0.225]),
+ dict(
+ type='Collect', keys=['video', 'label'], meta_keys=['fps',
+ 'modality']),
+]
+
+test_pipeline = val_pipeline
+
+data = dict(
+ samples_per_gpu=6,
+ workers_per_gpu=2,
+ val_dataloader=dict(samples_per_gpu=6),
+ test_dataloader=dict(samples_per_gpu=6),
+ train=dict(
+ type='NVGestureDataset',
+ ann_file=f'{data_root}/annotations/'
+ 'nvgesture_train_correct_cvpr2016_v2.lst',
+ vid_prefix=f'{data_root}/',
+ data_cfg=data_cfg,
+ pipeline=train_pipeline,
+ dataset_info={{_base_.dataset_info}}),
+ val=dict(
+ type='NVGestureDataset',
+ ann_file=f'{data_root}/annotations/'
+ 'nvgesture_test_correct_cvpr2016_v2.lst',
+ vid_prefix=f'{data_root}/',
+ data_cfg=data_cfg,
+ pipeline=val_pipeline,
+ test_mode=True,
+ dataset_info={{_base_.dataset_info}}),
+ test=dict(
+ type='NVGestureDataset',
+ ann_file=f'{data_root}/annotations/'
+ 'nvgesture_test_correct_cvpr2016_v2.lst',
+ vid_prefix=f'{data_root}/',
+ data_cfg=data_cfg,
+ pipeline=test_pipeline,
+ test_mode=True,
+ dataset_info={{_base_.dataset_info}}))
diff --git a/configs/hand/gesture_sview_rgbd_vid/mtut/nvgesture/i3d_nvgesture_bbox_224x224_fps30.py b/configs/hand/gesture_sview_rgbd_vid/mtut/nvgesture/i3d_nvgesture_bbox_224x224_fps30.py
new file mode 100644
index 0000000000..8a00d1e9f4
--- /dev/null
+++ b/configs/hand/gesture_sview_rgbd_vid/mtut/nvgesture/i3d_nvgesture_bbox_224x224_fps30.py
@@ -0,0 +1,134 @@
+_base_ = [
+ '../../../../_base_/default_runtime.py',
+ '../../../../_base_/datasets/nvgesture.py'
+]
+
+checkpoint_config = dict(interval=5)
+evaluation = dict(interval=5, metric='AP', save_best='AP_rgb')
+
+optimizer = dict(
+ type='SGD',
+ lr=1e-2,
+ momentum=0.9,
+)
+optimizer_config = dict(grad_clip=None)
+# learning policy
+lr_config = dict(policy='step', gamma=0.1, step=[30, 50])
+total_epochs = 75
+log_config = dict(interval=10)
+
+custom_hooks_config = [dict(type='ModelSetEpochHook')]
+
+model = dict(
+ type='GestureRecognizer',
+ modality=['rgb', 'depth'],
+ pretrained=dict(
+ rgb='https://github.com/hassony2/kinetics_i3d_pytorch/'
+ 'raw/master/model/model_rgb.pth',
+ depth='https://github.com/hassony2/kinetics_i3d_pytorch/'
+ 'raw/master/model/model_rgb.pth',
+ ),
+ backbone=dict(
+ rgb=dict(
+ type='I3D',
+ in_channels=3,
+ expansion=1,
+ ),
+ depth=dict(
+ type='I3D',
+ in_channels=1,
+ expansion=1,
+ ),
+ ),
+ cls_head=dict(
+ type='MultiModalSSAHead',
+ num_classes=25,
+ ),
+ train_cfg=dict(
+ beta=2,
+ lambda_=5e-3,
+ ssa_start_epoch=61,
+ ),
+ test_cfg=dict(),
+)
+
+data_root = 'data/nvgesture'
+data_cfg = dict(
+ video_size=[320, 240],
+ modality=['rgb', 'depth'],
+ bbox_file=f'{data_root}/annotations/bboxes.json',
+)
+
+train_pipeline = [
+ dict(type='LoadVideoFromFile'),
+ dict(type='ModalWiseChannelProcess'),
+ dict(type='CropValidClip'),
+ dict(type='TemporalPooling', length=64, ref_fps=30),
+ dict(type='MultiFrameBBoxMerge'),
+ dict(
+ type='ResizedCropByBBox',
+ size=224,
+ scale=(0.8, 1.25),
+ ratio=(0.75, 1.33),
+ shift=0.3),
+ dict(type='GestureRandomFlip'),
+ dict(type='MultiModalVideoToTensor'),
+ dict(
+ type='VideoNormalizeTensor',
+ mean=[0.485, 0.456, 0.406],
+ std=[0.229, 0.224, 0.225]),
+ dict(
+ type='Collect', keys=['video', 'label'], meta_keys=['fps',
+ 'modality']),
+]
+
+val_pipeline = [
+ dict(type='LoadVideoFromFile'),
+ dict(type='ModalWiseChannelProcess'),
+ dict(type='CropValidClip'),
+ dict(type='TemporalPooling', length=-1, ref_fps=30),
+ dict(type='MultiFrameBBoxMerge'),
+ dict(type='ResizedCropByBBox', size=224),
+ dict(type='MultiModalVideoToTensor'),
+ dict(
+ type='VideoNormalizeTensor',
+ mean=[0.485, 0.456, 0.406],
+ std=[0.229, 0.224, 0.225]),
+ dict(
+ type='Collect', keys=['video', 'label'], meta_keys=['fps',
+ 'modality']),
+]
+
+test_pipeline = val_pipeline
+
+data = dict(
+ samples_per_gpu=6,
+ workers_per_gpu=2,
+ val_dataloader=dict(samples_per_gpu=6),
+ test_dataloader=dict(samples_per_gpu=6),
+ train=dict(
+ type='NVGestureDataset',
+ ann_file=f'{data_root}/annotations/'
+ 'nvgesture_train_correct_cvpr2016_v2.lst',
+ vid_prefix=f'{data_root}/',
+ data_cfg=data_cfg,
+ pipeline=train_pipeline,
+ dataset_info={{_base_.dataset_info}}),
+ val=dict(
+ type='NVGestureDataset',
+ ann_file=f'{data_root}/annotations/'
+ 'nvgesture_test_correct_cvpr2016_v2.lst',
+ vid_prefix=f'{data_root}/',
+ data_cfg=data_cfg,
+ pipeline=val_pipeline,
+ test_mode=True,
+ dataset_info={{_base_.dataset_info}}),
+ test=dict(
+ type='NVGestureDataset',
+ ann_file=f'{data_root}/annotations/'
+ 'nvgesture_test_correct_cvpr2016_v2.lst',
+ vid_prefix=f'{data_root}/',
+ data_cfg=data_cfg,
+ pipeline=test_pipeline,
+ test_mode=True,
+ dataset_info={{_base_.dataset_info}}))
diff --git a/demo/docs/mmdet_modelzoo.md b/demo/docs/mmdet_modelzoo.md
index a1d9a025aa..ae6b24df28 100644
--- a/demo/docs/mmdet_modelzoo.md
+++ b/demo/docs/mmdet_modelzoo.md
@@ -14,6 +14,7 @@ For hand bounding box detection, we simply train our hand box models on onehand1
| Arch | Box AP | ckpt | log |
| :---------------------------------------------------------------- | :----: | :---------------------------------------------------------------: | :--------------------------------------------------------------: |
| [Cascade_R-CNN X-101-64x4d-FPN-1class](/demo/mmdetection_cfg/cascade_rcnn_x101_64x4d_fpn_1class.py) | 0.817 | [ckpt](https://download.openmmlab.com/mmpose/mmdet_pretrained/cascade_rcnn_x101_64x4d_fpn_20e_onehand10k-dac19597_20201030.pth) | [log](https://download.openmmlab.com/mmpose/mmdet_pretrained/cascade_rcnn_x101_64x4d_fpn_20e_onehand10k_20201030.log.json) |
+| [ssdlite_mobilenetv2-1class](/demo/mmdetection_cfg/ssdlite_mobilenetv2_scratch_600e_onehand.py) | 0.779 | [ckpt](https://download.openmmlab.com/mmpose/mmdet_pretrained/ssdlite_mobilenetv2_scratch_600e_onehand-4f9f8686_20220523.pth) | [log](https://download.openmmlab.com/mmpose/mmdet_pretrainedssdlite_mobilenetv2_scratch_600e_onehand_20220523.log.json) |
### Animal Bounding Box Detection Models
diff --git a/demo/mmdetection_cfg/ssdlite_mobilenetv2_scratch_600e_onehand.py b/demo/mmdetection_cfg/ssdlite_mobilenetv2_scratch_600e_onehand.py
new file mode 100644
index 0000000000..b7e37964cf
--- /dev/null
+++ b/demo/mmdetection_cfg/ssdlite_mobilenetv2_scratch_600e_onehand.py
@@ -0,0 +1,240 @@
+# =========================================================
+# from 'mmdetection/configs/_base_/default_runtime.py'
+# =========================================================
+checkpoint_config = dict(interval=1)
+# yapf:disable
+log_config = dict(
+ interval=50,
+ hooks=[
+ dict(type='TextLoggerHook'),
+ # dict(type='TensorboardLoggerHook')
+ ])
+# yapf:enable
+custom_hooks = [dict(type='NumClassCheckHook')]
+# =========================================================
+
+# =========================================================
+# from 'mmdetection/configs/_base_/datasets/coco_detection.py'
+# =========================================================
+# dataset settings
+dataset_type = 'CocoDataset'
+data_root = 'data/coco/'
+img_norm_cfg = dict(
+ mean=[123.675, 116.28, 103.53], std=[58.395, 57.12, 57.375], to_rgb=True)
+train_pipeline = [
+ dict(type='LoadImageFromFile'),
+ dict(type='LoadAnnotations', with_bbox=True),
+ dict(type='Resize', img_scale=(1333, 800), keep_ratio=True),
+ dict(type='RandomFlip', flip_ratio=0.5),
+ dict(type='Normalize', **img_norm_cfg),
+ dict(type='Pad', size_divisor=32),
+ dict(type='DefaultFormatBundle'),
+ dict(type='Collect', keys=['img', 'gt_bboxes', 'gt_labels']),
+]
+test_pipeline = [
+ dict(type='LoadImageFromFile'),
+ dict(
+ type='MultiScaleFlipAug',
+ img_scale=(1333, 800),
+ flip=False,
+ transforms=[
+ dict(type='Resize', keep_ratio=True),
+ dict(type='RandomFlip'),
+ dict(type='Normalize', **img_norm_cfg),
+ dict(type='Pad', size_divisor=32),
+ dict(type='ImageToTensor', keys=['img']),
+ dict(type='Collect', keys=['img']),
+ ])
+]
+data = dict(
+ samples_per_gpu=2,
+ workers_per_gpu=2,
+ train=dict(
+ type=dataset_type,
+ ann_file=data_root + 'annotations/instances_train2017.json',
+ img_prefix=data_root + 'train2017/',
+ pipeline=train_pipeline),
+ val=dict(
+ type=dataset_type,
+ ann_file=data_root + 'annotations/instances_val2017.json',
+ img_prefix=data_root + 'val2017/',
+ pipeline=test_pipeline),
+ test=dict(
+ type=dataset_type,
+ ann_file=data_root + 'annotations/instances_val2017.json',
+ img_prefix=data_root + 'val2017/',
+ pipeline=test_pipeline))
+evaluation = dict(interval=1, metric='bbox')
+# =========================================================
+
+model = dict(
+ type='SingleStageDetector',
+ backbone=dict(
+ type='MobileNetV2',
+ out_indices=(4, 7),
+ norm_cfg=dict(type='BN', eps=0.001, momentum=0.03),
+ init_cfg=dict(type='TruncNormal', layer='Conv2d', std=0.03)),
+ neck=dict(
+ type='SSDNeck',
+ in_channels=(96, 1280),
+ out_channels=(96, 1280, 512, 256, 256, 128),
+ level_strides=(2, 2, 2, 2),
+ level_paddings=(1, 1, 1, 1),
+ l2_norm_scale=None,
+ use_depthwise=True,
+ norm_cfg=dict(type='BN', eps=0.001, momentum=0.03),
+ act_cfg=dict(type='ReLU6'),
+ init_cfg=dict(type='TruncNormal', layer='Conv2d', std=0.03)),
+ bbox_head=dict(
+ type='SSDHead',
+ in_channels=(96, 1280, 512, 256, 256, 128),
+ num_classes=1,
+ use_depthwise=True,
+ norm_cfg=dict(type='BN', eps=0.001, momentum=0.03),
+ act_cfg=dict(type='ReLU6'),
+ init_cfg=dict(type='Normal', layer='Conv2d', std=0.001),
+
+ # set anchor size manually instead of using the predefined
+ # SSD300 setting.
+ anchor_generator=dict(
+ type='SSDAnchorGenerator',
+ scale_major=False,
+ strides=[16, 32, 64, 107, 160, 320],
+ ratios=[[2, 3], [2, 3], [2, 3], [2, 3], [2, 3], [2, 3]],
+ min_sizes=[48, 100, 150, 202, 253, 304],
+ max_sizes=[100, 150, 202, 253, 304, 320]),
+ bbox_coder=dict(
+ type='DeltaXYWHBBoxCoder',
+ target_means=[.0, .0, .0, .0],
+ target_stds=[0.1, 0.1, 0.2, 0.2])),
+ # model training and testing settings
+ train_cfg=dict(
+ assigner=dict(
+ type='MaxIoUAssigner',
+ pos_iou_thr=0.5,
+ neg_iou_thr=0.5,
+ min_pos_iou=0.,
+ ignore_iof_thr=-1,
+ gt_max_assign_all=False),
+ smoothl1_beta=1.,
+ allowed_border=-1,
+ pos_weight=-1,
+ neg_pos_ratio=3,
+ debug=False),
+ test_cfg=dict(
+ nms_pre=1000,
+ nms=dict(type='nms', iou_threshold=0.45),
+ min_bbox_size=0,
+ score_thr=0.02,
+ max_per_img=200))
+cudnn_benchmark = True
+
+# dataset settings
+file_client_args = dict(
+ backend='petrel',
+ path_mapping=dict({
+ '.data/onehand10k/':
+ 'openmmlab:s3://openmmlab/datasets/pose/OneHand10K/',
+ 'data/onehand10k/':
+ 'openmmlab:s3://openmmlab/datasets/pose/OneHand10K/'
+ }))
+
+dataset_type = 'CocoDataset'
+data_root = 'data/onehand10k/'
+classes = ('hand', )
+img_norm_cfg = dict(
+ mean=[123.675, 116.28, 103.53], std=[58.395, 57.12, 57.375], to_rgb=True)
+train_pipeline = [
+ dict(type='LoadImageFromFile', file_client_args=file_client_args),
+ dict(type='LoadAnnotations', with_bbox=True),
+ dict(
+ type='Expand',
+ mean=img_norm_cfg['mean'],
+ to_rgb=img_norm_cfg['to_rgb'],
+ ratio_range=(1, 4)),
+ dict(
+ type='MinIoURandomCrop',
+ min_ious=(0.1, 0.3, 0.5, 0.7, 0.9),
+ min_crop_size=0.3),
+ dict(type='Resize', img_scale=(320, 320), keep_ratio=False),
+ dict(type='RandomFlip', flip_ratio=0.5),
+ dict(
+ type='PhotoMetricDistortion',
+ brightness_delta=32,
+ contrast_range=(0.5, 1.5),
+ saturation_range=(0.5, 1.5),
+ hue_delta=18),
+ dict(type='Normalize', **img_norm_cfg),
+ dict(type='Pad', size_divisor=320),
+ dict(type='DefaultFormatBundle'),
+ dict(type='Collect', keys=['img', 'gt_bboxes', 'gt_labels']),
+]
+test_pipeline = [
+ dict(type='LoadImageFromFile', file_client_args=file_client_args),
+ dict(
+ type='MultiScaleFlipAug',
+ img_scale=(320, 320),
+ flip=False,
+ transforms=[
+ dict(type='Resize', keep_ratio=False),
+ dict(type='Normalize', **img_norm_cfg),
+ dict(type='Pad', size_divisor=320),
+ dict(type='ImageToTensor', keys=['img']),
+ dict(type='Collect', keys=['img']),
+ ])
+]
+data = dict(
+ samples_per_gpu=24,
+ workers_per_gpu=4,
+ train=dict(
+ _delete_=True,
+ type='RepeatDataset', # use RepeatDataset to speed up training
+ times=5,
+ dataset=dict(
+ type=dataset_type,
+ ann_file=data_root + 'annotations/onehand10k_train.json',
+ img_prefix=data_root,
+ classes=classes,
+ pipeline=train_pipeline)),
+ val=dict(
+ ann_file=data_root + 'annotations/onehand10k_test.json',
+ img_prefix=data_root,
+ classes=classes,
+ pipeline=test_pipeline),
+ test=dict(
+ ann_file=data_root + 'annotations/onehand10k_test.json',
+ img_prefix=data_root,
+ classes=classes,
+ pipeline=test_pipeline))
+
+# optimizer
+optimizer = dict(type='SGD', lr=0.015, momentum=0.9, weight_decay=4.0e-5)
+optimizer_config = dict(grad_clip=None)
+
+# learning policy
+lr_config = dict(
+ policy='CosineAnnealing',
+ warmup='linear',
+ warmup_iters=500,
+ warmup_ratio=0.001,
+ min_lr=0)
+runner = dict(type='EpochBasedRunner', max_epochs=120)
+
+# Avoid evaluation and saving weights too frequently
+evaluation = dict(interval=5, metric='bbox')
+checkpoint_config = dict(interval=5)
+custom_hooks = [
+ dict(type='NumClassCheckHook'),
+ dict(type='CheckInvalidLossHook', interval=50, priority='VERY_LOW')
+]
+
+log_config = dict(interval=5)
+
+# NOTE: `auto_scale_lr` is for automatically scaling LR,
+# USER SHOULD NOT CHANGE ITS VALUES.
+# base_batch_size = (8 GPUs) x (24 samples per GPU)
+auto_scale_lr = dict(base_batch_size=192)
+
+load_from = 'https://download.openmmlab.com/mmdetection/'
+'v2.0/ssd/ssdlite_mobilenetv2_scratch_600e_coco/'
+'ssdlite_mobilenetv2_scratch_600e_coco_20210629_110627-974d9307.pth'
diff --git a/docs/en/papers/algorithms/mtut.md b/docs/en/papers/algorithms/mtut.md
new file mode 100644
index 0000000000..7cfefeef2f
--- /dev/null
+++ b/docs/en/papers/algorithms/mtut.md
@@ -0,0 +1,30 @@
+# Improving the Performance of Unimodal Dynamic Hand-Gesture Recognition with Multimodal Training
+
+
+
+
+MTUT (CVPR'2019)
+
+```bibtex
+@InProceedings{Abavisani_2019_CVPR,
+author = {Abavisani, Mahdi and Joze, Hamid Reza Vaezi and Patel, Vishal M.},
+title = {Improving the Performance of Unimodal Dynamic Hand-Gesture Recognition With Multimodal Training},
+booktitle = {Proceedings of the IEEE/CVF Conference on Computer Vision and Pattern Recognition (CVPR)},
+month = {June},
+year = {2019}
+}
+```
+
+
+
+## Abstract
+
+
+
+We present an efficient approach for leveraging the knowledge from multiple modalities in training unimodal 3D convolutional neural networks (3D-CNNs) for the task of dynamic hand gesture recognition. Instead of explicitly combining multimodal information, which is commonplace in many state-of-the-art methods, we propose a different framework in which we embed the knowledge of multiple modalities in individual networks so that each unimodal network can achieve an improved performance. In particular, we dedicate separate networks per available modality and enforce them to collaborate and learn to develop networks with common semantics and better representations. We introduce a "spatiotemporal semantic alignment" loss (SSA) to align the content of the features from different networks. In addition, we regularize this loss with our proposed "focal regularization parameter" to avoid negative knowledge transfer. Experimental results show that our framework improves the test time recognition accuracy of unimodal networks, and provides the state-of-the-art performance on various dynamic hand gesture recognition datasets.
+
+
+
+
+
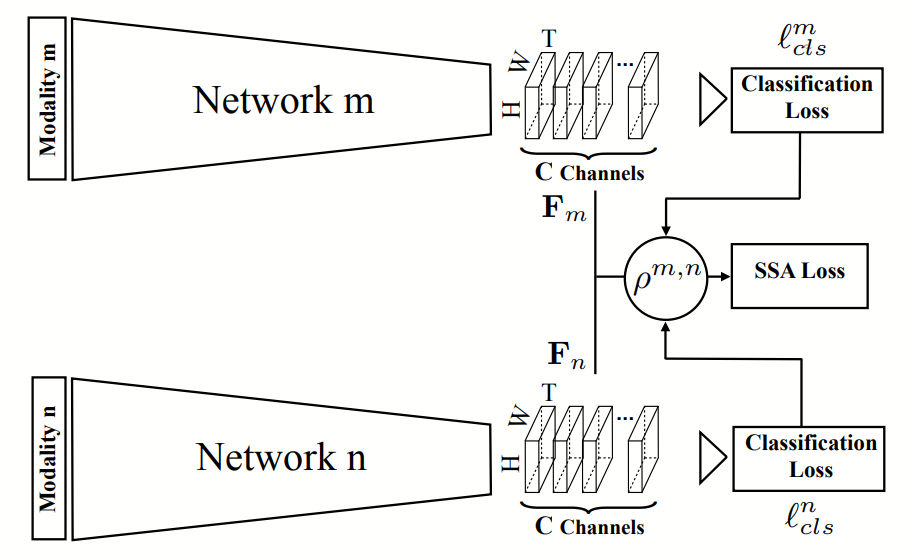
+
diff --git a/docs/en/papers/backbones/i3d.md b/docs/en/papers/backbones/i3d.md
new file mode 100644
index 0000000000..b1a51d1400
--- /dev/null
+++ b/docs/en/papers/backbones/i3d.md
@@ -0,0 +1,30 @@
+# Quo Vadis, Action Recognition? A New Model and the Kinetics Dataset
+
+
+
+
+I3D (CVPR'2017)
+
+```bibtex
+@InProceedings{Carreira_2017_CVPR,
+ author = {Carreira, Joao and Zisserman, Andrew},
+ title = {Quo Vadis, Action Recognition? A New Model and the Kinetics Dataset},
+ booktitle = {Proceedings of the IEEE Conference on Computer Vision and Pattern Recognition (CVPR)},
+ month = {July},
+ year = {2017}
+}
+```
+
+
+
+## Abstract
+
+
+
+The paucity of videos in current action classification datasets (UCF-101 and HMDB-51) has made it difficult to identify good video architectures, as most methods obtain similar performance on existing small-scale benchmarks. This paper re-evaluates state-of-the-art architectures in light of the new Kinetics Human Action Video dataset. Kinetics has two orders of magnitude more data, with 400 human action classes and over 400 clips per class, and is collected from realistic, challenging YouTube videos. We provide an analysis on how current architectures fare on the task of action classification on this dataset and how much performance improves on the smaller benchmark datasets after pre-training on Kinetics. We also introduce a new Two-Stream Inflated 3D ConvNet (I3D) that is based on 2D ConvNet inflation: filters and pooling kernels of very deep image classification ConvNets are expanded into 3D, making it possible to learn seamless spatio-temporal feature extractors from video while leveraging successful ImageNet architecture designs and even their parameters. We show that, after pre-training on Kinetics, I3D models considerably improve upon the state-of-the-art in action classification, reaching 80.2% on HMDB-51 and 97.9% on UCF-101.
+
+
+
+
+
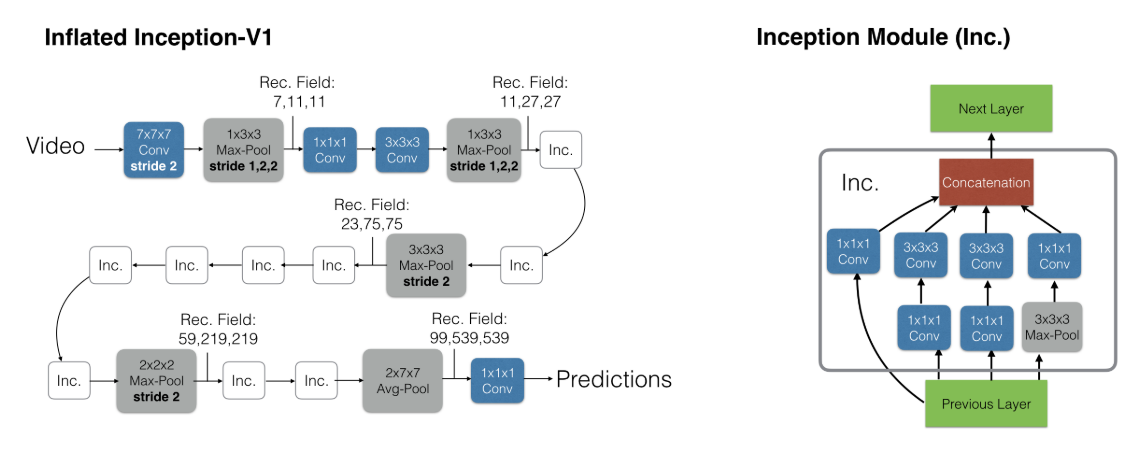
+
diff --git a/docs/en/papers/datasets/nvgesture.md b/docs/en/papers/datasets/nvgesture.md
new file mode 100644
index 0000000000..772cda9483
--- /dev/null
+++ b/docs/en/papers/datasets/nvgesture.md
@@ -0,0 +1,18 @@
+# Online Detection and Classification of Dynamic Hand Gestures With Recurrent 3D Convolutional Neural Network
+
+
+
+
+NVGesture (CVPR'2016)
+
+```bibtex
+@InProceedings{Molchanov_2016_CVPR,
+ author = {Molchanov, Pavlo and Yang, Xiaodong and Gupta, Shalini and Kim, Kihwan and Tyree, Stephen and Kautz, Jan},
+ title = {Online Detection and Classification of Dynamic Hand Gestures With Recurrent 3D Convolutional Neural Network},
+ booktitle = {Proceedings of the IEEE Conference on Computer Vision and Pattern Recognition (CVPR)},
+ month = {June},
+ year = {2016}
+}
+```
+
+
diff --git a/docs/en/tasks/2d_hand_gesture.md b/docs/en/tasks/2d_hand_gesture.md
new file mode 100644
index 0000000000..6161b401f7
--- /dev/null
+++ b/docs/en/tasks/2d_hand_gesture.md
@@ -0,0 +1,60 @@
+# 2D Hand Keypoint Datasets
+
+It is recommended to symlink the dataset root to `$MMPOSE/data`.
+If your folder structure is different, you may need to change the corresponding paths in config files.
+
+MMPose supported datasets:
+
+- [NVGesture](#nvgesture) \[ [Homepage](https://www.v7labs.com/open-datasets/nvgesture) \]
+
+## NVGesture
+
+
+
+
+OneHand10K (CVPR'2016)
+
+```bibtex
+@inproceedings{molchanov2016online,
+ title={Online detection and classification of dynamic hand gestures with recurrent 3d convolutional neural network},
+ author={Molchanov, Pavlo and Yang, Xiaodong and Gupta, Shalini and Kim, Kihwan and Tyree, Stephen and Kautz, Jan},
+ booktitle={Proceedings of the IEEE conference on computer vision and pattern recognition},
+ pages={4207--4215},
+ year={2016}
+}
+```
+
+
+
+For [NVGesture](https://www.v7labs.com/open-datasets/nvgesture) data and annotation, please download from [NVGesture Dataset](https://drive.google.com/drive/folders/0ByhYoRYACz9cMUk0QkRRMHM3enc?resourcekey=0-cJe9M3PZy2qCbfGmgpFrHQ&usp=sharing).
+Extract them under {MMPose}/data, and make them look like this:
+
+```text
+mmpose
+├── mmpose
+├── docs
+├── tests
+├── tools
+├── configs
+`── data
+ │── nvgesture
+ |── annotations
+ | |── nvgesture_train_correct_cvpr2016_v2.lst
+ | |── nvgesture_test_correct_cvpr2016_v2.lst
+ | ...
+ `── Video_data
+ |── class_01
+ | |── subject1_r0
+ | | |── sk_color.avi
+ | | |── sk_depth.avi
+ | | ...
+ | |── subject1_r1
+ | |── subject2_r0
+ | ...
+ |── class_02
+ |── class_03
+ ...
+
+```
+
+The hand bounding box is computed by the hand detection model described in [det model zoo](/demo/docs/mmdet_modelzoo.md). The detected bounding box can be downloaded from [GoogleDrive](https://drive.google.com/drive/folders/1AGOeX0iHhaigBVRicjetieNRC7Zctuz4?usp=sharing). It is recommended to place it at `data/nvgesture/annotations/bboxes.json`.
diff --git a/mmpose/apis/__init__.py b/mmpose/apis/__init__.py
index 772341fb02..2a7f70fbec 100644
--- a/mmpose/apis/__init__.py
+++ b/mmpose/apis/__init__.py
@@ -1,7 +1,8 @@
# Copyright (c) OpenMMLab. All rights reserved.
from .inference import (collect_multi_frames, inference_bottom_up_pose_model,
- inference_top_down_pose_model, init_pose_model,
- process_mmdet_results, vis_pose_result)
+ inference_gesture_model, inference_top_down_pose_model,
+ init_pose_model, process_mmdet_results,
+ vis_pose_result)
from .inference_3d import (extract_pose_sequence, inference_interhand_3d_model,
inference_mesh_model, inference_pose_lifter_model,
vis_3d_mesh_result, vis_3d_pose_result)
@@ -10,11 +11,23 @@
from .train import init_random_seed, train_model
__all__ = [
- 'train_model', 'init_pose_model', 'inference_top_down_pose_model',
- 'inference_bottom_up_pose_model', 'multi_gpu_test', 'single_gpu_test',
- 'vis_pose_result', 'get_track_id', 'vis_pose_tracking_result',
- 'inference_pose_lifter_model', 'vis_3d_pose_result',
- 'inference_interhand_3d_model', 'extract_pose_sequence',
- 'inference_mesh_model', 'vis_3d_mesh_result', 'process_mmdet_results',
- 'init_random_seed', 'collect_multi_frames'
+ 'train_model',
+ 'init_pose_model',
+ 'inference_top_down_pose_model',
+ 'inference_bottom_up_pose_model',
+ 'multi_gpu_test',
+ 'single_gpu_test',
+ 'vis_pose_result',
+ 'get_track_id',
+ 'vis_pose_tracking_result',
+ 'inference_pose_lifter_model',
+ 'vis_3d_pose_result',
+ 'inference_interhand_3d_model',
+ 'extract_pose_sequence',
+ 'inference_mesh_model',
+ 'vis_3d_mesh_result',
+ 'process_mmdet_results',
+ 'init_random_seed',
+ 'collect_multi_frames',
+ 'inference_gesture_model',
]
diff --git a/mmpose/apis/inference.py b/mmpose/apis/inference.py
index 6d7f4dacaf..20c6d0d007 100644
--- a/mmpose/apis/inference.py
+++ b/mmpose/apis/inference.py
@@ -2,6 +2,7 @@
import copy
import os
import warnings
+from collections import defaultdict
import mmcv
import numpy as np
@@ -800,6 +801,61 @@ def vis_pose_result(model,
return img
+def inference_gesture_model(
+ model,
+ videos_or_paths,
+ bboxes=None,
+ dataset_info=None,
+):
+
+ cfg = model.cfg
+ device = next(model.parameters()).device
+ if device.type == 'cpu':
+ device = -1
+
+ # build the data pipeline
+ test_pipeline = Compose(cfg.test_pipeline)
+ _pipeline_gpu_speedup(test_pipeline, next(model.parameters()).device)
+
+ # data preprocessing
+ data = defaultdict(list)
+ data['label'] = -1
+
+ if not isinstance(videos_or_paths, (tuple, list)):
+ videos_or_paths = [videos_or_paths]
+ if isinstance(videos_or_paths[0], str):
+ data['video_file'] = videos_or_paths
+ else:
+ data['video'] = videos_or_paths
+
+ if bboxes is not None:
+ data['bbox'] = bboxes
+
+ if isinstance(dataset_info, dict):
+ data['modality'] = dataset_info.get('modality', ['rgb'])
+ data['fps'] = dataset_info.get('fps', None)
+ if not isinstance(data['fps'], (tuple, list)):
+ data['fps'] = [data['fps']]
+
+ data = test_pipeline(data)
+ batch_data = collate([data], samples_per_gpu=1)
+ batch_data = scatter(batch_data, [device])[0]
+
+ # inference
+ with torch.no_grad():
+ output = model.forward(return_loss=False, **batch_data)
+ scores = []
+ for modal, logit in output['logits'].items():
+ while logit.ndim > 2:
+ logit = logit.mean(dim=2)
+ score = torch.softmax(logit, dim=1)
+ scores.append(score)
+ score = torch.stack(scores, dim=2).mean(dim=2)
+ pred_score, pred_label = torch.max(score, dim=1)
+
+ return pred_label, pred_score
+
+
def process_mmdet_results(mmdet_results, cat_id=1):
"""Process mmdet results, and return a list of bboxes.
diff --git a/mmpose/core/utils/__init__.py b/mmpose/core/utils/__init__.py
index d059d21422..512e7680bc 100644
--- a/mmpose/core/utils/__init__.py
+++ b/mmpose/core/utils/__init__.py
@@ -1,5 +1,9 @@
# Copyright (c) OpenMMLab. All rights reserved.
from .dist_utils import allreduce_grads, sync_random_seed
+from .model_util_hooks import ModelSetEpochHook
from .regularizations import WeightNormClipHook
-__all__ = ['allreduce_grads', 'WeightNormClipHook', 'sync_random_seed']
+__all__ = [
+ 'allreduce_grads', 'WeightNormClipHook', 'sync_random_seed',
+ 'ModelSetEpochHook'
+]
diff --git a/mmpose/core/utils/model_util_hooks.py b/mmpose/core/utils/model_util_hooks.py
new file mode 100644
index 0000000000..d308a8a57a
--- /dev/null
+++ b/mmpose/core/utils/model_util_hooks.py
@@ -0,0 +1,13 @@
+# Copyright (c) OpenMMLab. All rights reserved.
+from mmcv.runner import HOOKS, Hook
+
+
+@HOOKS.register_module()
+class ModelSetEpochHook(Hook):
+ """The hook that tells model the current epoch in training."""
+
+ def __init__(self):
+ pass
+
+ def before_epoch(self, runner):
+ runner.model.module.set_train_epoch(runner.epoch + 1)
diff --git a/mmpose/datasets/__init__.py b/mmpose/datasets/__init__.py
index 650d7e9480..fd58f3ea6d 100644
--- a/mmpose/datasets/__init__.py
+++ b/mmpose/datasets/__init__.py
@@ -21,7 +21,7 @@
TopDownOCHumanDataset, TopDownOneHand10KDataset, TopDownPanopticDataset,
TopDownPoseTrack18Dataset, TopDownPoseTrack18VideoDataset,
Body3DMviewDirectPanopticDataset, Body3DMviewDirectShelfDataset,
- Body3DMviewDirectCampusDataset)
+ Body3DMviewDirectCampusDataset, NVGestureDataset)
__all__ = [
'TopDownCocoDataset', 'BottomUpCocoDataset', 'BottomUpMhpDataset',
@@ -42,5 +42,5 @@
'TopDownPoseTrack18VideoDataset', 'build_dataloader', 'build_dataset',
'Compose', 'DistributedSampler', 'DATASETS', 'PIPELINES', 'DatasetInfo',
'Body3DMviewDirectPanopticDataset', 'Body3DMviewDirectShelfDataset',
- 'Body3DMviewDirectCampusDataset'
+ 'Body3DMviewDirectCampusDataset', 'NVGestureDataset'
]
diff --git a/mmpose/datasets/datasets/__init__.py b/mmpose/datasets/datasets/__init__.py
index 603f840206..f44fc8e198 100644
--- a/mmpose/datasets/datasets/__init__.py
+++ b/mmpose/datasets/datasets/__init__.py
@@ -13,6 +13,7 @@
from .face import (Face300WDataset, FaceAFLWDataset, FaceCocoWholeBodyDataset,
FaceCOFWDataset, FaceWFLWDataset)
from .fashion import DeepFashionDataset
+from .gesture import NVGestureDataset
from .hand import (FreiHandDataset, HandCocoWholeBodyDataset,
InterHand2DDataset, InterHand3DDataset, OneHand10KDataset,
PanopticDataset)
@@ -44,5 +45,5 @@
'AnimalATRWDataset', 'AnimalPoseDataset', 'TopDownH36MDataset',
'TopDownHalpeDataset', 'TopDownPoseTrack18VideoDataset',
'Body3DMviewDirectPanopticDataset', 'Body3DMviewDirectShelfDataset',
- 'Body3DMviewDirectCampusDataset'
+ 'Body3DMviewDirectCampusDataset', 'NVGestureDataset'
]
diff --git a/mmpose/datasets/datasets/gesture/__init__.py b/mmpose/datasets/datasets/gesture/__init__.py
new file mode 100644
index 0000000000..22c85afd7c
--- /dev/null
+++ b/mmpose/datasets/datasets/gesture/__init__.py
@@ -0,0 +1,4 @@
+# Copyright (c) OpenMMLab. All rights reserved.
+from .nvgesture_dataset import NVGestureDataset
+
+__all__ = ['NVGestureDataset']
diff --git a/mmpose/datasets/datasets/gesture/gesture_base_dataset.py b/mmpose/datasets/datasets/gesture/gesture_base_dataset.py
new file mode 100644
index 0000000000..e81d972ece
--- /dev/null
+++ b/mmpose/datasets/datasets/gesture/gesture_base_dataset.py
@@ -0,0 +1,86 @@
+# Copyright (c) OpenMMLab. All rights reserved.
+import copy
+from abc import ABCMeta, abstractmethod
+
+import numpy as np
+from torch.utils.data import Dataset
+
+from mmpose.datasets.pipelines import Compose
+
+
+class GestureBaseDataset(Dataset, metaclass=ABCMeta):
+ """Base class for gesture recognition datasets with Multi-Modal video as
+ the input.
+
+ All gesture datasets should subclass it.
+ All subclasses should overwrite:
+ Methods:`_get_single`, 'evaluate'
+
+ Args:
+ ann_file (str): Path to the annotation file.
+ vid_prefix (str): Path to a directory where videos are held.
+ data_cfg (dict): config
+ pipeline (list[dict | callable]): A sequence of data transforms.
+ dataset_info (DatasetInfo): A class containing all dataset info.
+ test_mode (bool): Store True when building test or
+ validation dataset. Default: False.
+ """
+
+ def __init__(self,
+ ann_file,
+ vid_prefix,
+ data_cfg,
+ pipeline,
+ dataset_info=None,
+ test_mode=False):
+
+ self.video_info = {}
+ self.ann_info = {}
+
+ self.ann_file = ann_file
+ self.vid_prefix = vid_prefix
+ self.pipeline = pipeline
+ self.test_mode = test_mode
+
+ self.ann_info['video_size'] = np.array(data_cfg['video_size'])
+ self.ann_info['flip_pairs'] = dataset_info.flip_pairs
+ self.modality = data_cfg['modality']
+ if isinstance(self.modality, (list, tuple)):
+ self.modality = self.modality
+ else:
+ self.modality = (self.modality, )
+ self.bbox_file = data_cfg.get('bbox_file', None)
+ self.dataset_name = dataset_info.dataset_name
+ self.pipeline = Compose(self.pipeline)
+
+ @abstractmethod
+ def _get_single(self, idx):
+ """Get anno for a single video."""
+ raise NotImplementedError
+
+ @abstractmethod
+ def evaluate(self, results, *args, **kwargs):
+ """Evaluate recognition results."""
+
+ def prepare_train_vid(self, idx):
+ """Prepare video for training given the index."""
+ results = copy.deepcopy(self._get_single(idx))
+ results['ann_info'] = self.ann_info
+ return self.pipeline(results)
+
+ def prepare_test_vid(self, idx):
+ """Prepare video for testing given the index."""
+ results = copy.deepcopy(self._get_single(idx))
+ results['ann_info'] = self.ann_info
+ return self.pipeline(results)
+
+ def __len__(self):
+ """Get dataset length."""
+ return len(self.vid_ids)
+
+ def __getitem__(self, idx):
+ """Get the sample for either training or testing given index."""
+ if self.test_mode:
+ return self.prepare_test_vid(idx)
+
+ return self.prepare_train_vid(idx)
diff --git a/mmpose/datasets/datasets/gesture/nvgesture_dataset.py b/mmpose/datasets/datasets/gesture/nvgesture_dataset.py
new file mode 100644
index 0000000000..83f5e0df06
--- /dev/null
+++ b/mmpose/datasets/datasets/gesture/nvgesture_dataset.py
@@ -0,0 +1,185 @@
+# Copyright (c) OpenMMLab. All rights reserved.
+import os
+import os.path as osp
+import tempfile
+import warnings
+from collections import defaultdict
+
+import json_tricks as json
+import numpy as np
+from mmcv import Config
+
+from ...builder import DATASETS
+from .gesture_base_dataset import GestureBaseDataset
+
+
+@DATASETS.register_module()
+class NVGestureDataset(GestureBaseDataset):
+ """NVGesture dataset for gesture recognition.
+
+ "Online Detection and Classification of Dynamic Hand Gestures
+ With Recurrent 3D Convolutional Neural Network",
+ Conference on Computer Vision and Pattern Recognition (CVPR) 2016.
+
+ The dataset loads raw videos and apply specified transforms
+ to return a dict containing the image tensors and other information.
+
+ Args:
+ ann_file (str): Path to the annotation file.
+ vid_prefix (str): Path to a directory where videos are held.
+ data_cfg (dict): config
+ pipeline (list[dict | callable]): A sequence of data transforms.
+ dataset_info (DatasetInfo): A class containing all dataset info.
+ test_mode (bool): Store True when building test or
+ validation dataset. Default: False.
+ """
+
+ def __init__(self,
+ ann_file,
+ vid_prefix,
+ data_cfg,
+ pipeline,
+ dataset_info=None,
+ test_mode=False):
+
+ if dataset_info is None:
+ warnings.warn(
+ 'dataset_info is missing. '
+ 'Check https://github.com/open-mmlab/mmpose/pull/663 '
+ 'for details.', DeprecationWarning)
+ cfg = Config.fromfile('configs/_base_/datasets/nvgesture.py')
+ dataset_info = cfg._cfg_dict['dataset_info']
+
+ super().__init__(
+ ann_file,
+ vid_prefix,
+ data_cfg,
+ pipeline,
+ dataset_info=dataset_info,
+ test_mode=test_mode)
+
+ self.db = self._get_db()
+ self.vid_ids = list(range(len(self.db)))
+ print(f'=> load {len(self.db)} samples')
+
+ def _get_db(self):
+ """Load dataset."""
+ db = []
+ with open(self.ann_file, 'r') as f:
+ samples = f.readlines()
+
+ use_bbox = bool(self.bbox_file)
+ if use_bbox:
+ with open(self.bbox_file, 'r') as f:
+ bboxes = json.load(f)
+
+ for sample in samples:
+ sample = sample.strip().split()
+ sample = {
+ item.split(':', 1)[0]: item.split(':', 1)[1]
+ for item in sample
+ }
+ path = sample['path'][2:]
+ for key in ('depth', 'color'):
+ fname, start, end = sample[key].split(':')
+ sample[key] = {
+ 'path': os.path.join(path, fname + '.avi'),
+ 'valid_frames': (eval(start), eval(end))
+ }
+ sample['flow'] = {
+ 'path': sample['color']['path'].replace('color', 'flow'),
+ 'valid_frames': sample['color']['valid_frames']
+ }
+ sample['rgb'] = sample['color']
+ sample['label'] = eval(sample['label']) - 1
+
+ if use_bbox:
+ sample['bbox'] = bboxes[path]
+
+ del sample['path'], sample['duo_left'], sample['color']
+ db.append(sample)
+
+ return db
+
+ def _get_single(self, idx):
+ """Get anno for a single video."""
+ anno = defaultdict(list)
+ sample = self.db[self.vid_ids[idx]]
+
+ anno['label'] = sample['label']
+ anno['modality'] = self.modality
+ if 'bbox' in sample:
+ anno['bbox'] = sample['bbox']
+
+ for modal in self.modality:
+ anno['video_file'].append(
+ os.path.join(self.vid_prefix, sample[modal]['path']))
+ anno['valid_frames'].append(sample[modal]['valid_frames'])
+
+ return anno
+
+ def evaluate(self, results, res_folder=None, metric='AP', **kwargs):
+ """Evaluate nvgesture recognition results. The gesture prediction
+ results will be saved in ``${res_folder}/result_gesture.json``.
+
+ Note:
+ - batch_size: N
+ - heatmap length: L
+
+ Args:
+ results (dict): Testing results containing the following
+ items:
+ - logits (dict[str, torch.tensor[N,25,L]]): For each item, \
+ the key represents the modality of input video, while \
+ the value represents the prediction of gesture. Three \
+ dimensions represent batch, category and temporal \
+ length, respectively.
+ - label (np.ndarray[N]): [center[0], center[1], scale[0], \
+ scale[1],area, score]
+ res_folder (str, optional): The folder to save the testing
+ results. If not specified, a temp folder will be created.
+ Default: None.
+ metric (str | list[str]): Metric to be performed.
+ Options: 'AP'.
+
+ Returns:
+ dict: Evaluation results for evaluation metric.
+ """
+ metrics = metric if isinstance(metric, list) else [metric]
+ allowed_metrics = ['AP']
+ for metric in metrics:
+ if metric not in allowed_metrics:
+ raise KeyError(f'metric {metric} is not supported')
+
+ if res_folder is not None:
+ tmp_folder = None
+ res_file = osp.join(res_folder, 'result_gesture.json')
+ else:
+ tmp_folder = tempfile.TemporaryDirectory()
+ res_file = osp.join(tmp_folder.name, 'result_gesture.json')
+
+ predictions = defaultdict(list)
+ label = []
+ for result in results:
+ label.append(result['label'].cpu().numpy())
+ for modal in result['logits']:
+ logit = result['logits'][modal].mean(dim=2)
+ pred = logit.argmax(dim=1).cpu().numpy()
+ predictions[modal].append(pred)
+
+ label = np.concatenate(label, axis=0)
+ for modal in predictions:
+ predictions[modal] = np.concatenate(predictions[modal], axis=0)
+
+ with open(res_file, 'w') as f:
+ json.dump(predictions, f, indent=4)
+
+ results = dict()
+ if 'AP' in metrics:
+ APs = []
+ for modal in predictions:
+ results[f'AP_{modal}'] = (predictions[modal] == label).mean()
+ APs.append(results[f'AP_{modal}'])
+ results['AP_mean'] = sum(APs) / len(APs)
+
+ return results
diff --git a/mmpose/datasets/pipelines/__init__.py b/mmpose/datasets/pipelines/__init__.py
index cf06db1c9d..e619b339f6 100644
--- a/mmpose/datasets/pipelines/__init__.py
+++ b/mmpose/datasets/pipelines/__init__.py
@@ -1,7 +1,8 @@
# Copyright (c) OpenMMLab. All rights reserved.
from .bottom_up_transform import * # noqa
+from .gesture_transform import * # noqa
from .hand_transform import * # noqa
-from .loading import LoadImageFromFile # noqa
+from .loading import * # noqa
from .mesh_transform import * # noqa
from .pose3d_transform import * # noqa
from .shared_transform import * # noqa
diff --git a/mmpose/datasets/pipelines/gesture_transform.py b/mmpose/datasets/pipelines/gesture_transform.py
new file mode 100644
index 0000000000..28a3e568cc
--- /dev/null
+++ b/mmpose/datasets/pipelines/gesture_transform.py
@@ -0,0 +1,414 @@
+# Copyright (c) OpenMMLab. All rights reserved.
+import mmcv
+import numpy as np
+import torch
+
+from mmpose.core import bbox_xywh2xyxy, bbox_xyxy2xywh
+from mmpose.datasets.builder import PIPELINES
+
+
+@PIPELINES.register_module()
+class CropValidClip:
+ """Generate the clip from complete video with valid frames.
+
+ Required keys: 'video', 'modality', 'valid_frames', 'num_frames'.
+
+ Modified keys: 'video', 'valid_frames', 'num_frames'.
+ """
+
+ def __init__(self):
+ pass
+
+ def __call__(self, results):
+ """Crop the valid part from the video."""
+ if 'valid_frames' not in results:
+ results['valid_frames'] = [[0, n - 1]
+ for n in results['num_frames']]
+ lengths = [(end - start) for start, end in results['valid_frames']]
+ length = min(lengths)
+ for i, modal in enumerate(results['modality']):
+ start = results['valid_frames'][i][0]
+ results['video'][i] = results['video'][i][start:start + length]
+ results['num_frames'] = length
+ del results['valid_frames']
+ if 'bbox' in results:
+ results['bbox'] = results['bbox'][start:start + length]
+ return results
+
+
+@PIPELINES.register_module()
+class TemporalPooling:
+ """Pick frames according to either stride or reference fps.
+
+ Required keys: 'video', 'modality', 'num_frames', 'fps'.
+
+ Modified keys: 'video', 'num_frames'.
+
+ Args:
+ length (int): output video length. If unset, the entire video will
+ be pooled.
+ stride (int): temporal pooling stride. If unset, the stride will be
+ computed with video fps and `ref_fps`. If both `stride` and
+ `ref_fps` are unset, the stride will be 1.
+ ref_fps (int): expected fps of output video. If unset, the video will
+ be pooling with `stride`.
+ """
+
+ def __init__(self, length: int = -1, stride: int = -1, ref_fps: int = -1):
+ self.length = length
+ if stride == -1 and ref_fps == -1:
+ stride = 1
+ elif stride != -1 and ref_fps != -1:
+ raise ValueError('`stride` and `ref_fps` can not be assigned '
+ 'simultaneously, as they might conflict.')
+ self.stride = stride
+ self.ref_fps = ref_fps
+
+ def __call__(self, results):
+ """Implement data aumentation with random temporal crop."""
+
+ if self.ref_fps > 0 and 'fps' in results:
+ assert len(set(results['fps'])) == 1, 'Videos of different '
+ 'modality have different rate. May be misaligned after pooling.'
+ stride = results['fps'][0] // self.ref_fps
+ if stride < 1:
+ raise ValueError(f'`ref_fps` must be smaller than video '
+ f"fps {results['fps'][0]}")
+ else:
+ stride = self.stride
+
+ if self.length < 0:
+ length = results['num_frames']
+ num_frames = (results['num_frames'] - 1) // stride + 1
+ else:
+ length = (self.length - 1) * stride + 1
+ num_frames = self.length
+
+ diff = length - results['num_frames']
+ start = np.random.randint(max(1 - diff, 1))
+
+ for i, modal in enumerate(results['modality']):
+ video = results['video'][i]
+ if diff > 0:
+ video = np.pad(video, ((diff // 2, diff - (diff // 2)),
+ *(((0, 0), ) * (video.ndim - 1))),
+ 'edge')
+ results['video'][i] = video[start:start + length:stride]
+ assert results['video'][i].shape[0] == num_frames
+
+ results['num_frames'] = num_frames
+ if 'bbox' in results:
+ results['bbox'] = results['bbox'][start:start + length:stride]
+ return results
+
+
+@PIPELINES.register_module()
+class ResizeGivenShortEdge:
+ """Resize the video to make its short edge have given length.
+
+ Required keys: 'video', 'modality', 'width', 'height'.
+
+ Modified keys: 'video', 'width', 'height'.
+ """
+
+ def __init__(self, length: int = 256):
+ self.length = length
+
+ def __call__(self, results):
+ """Implement data processing with resize given short edge."""
+ for i, modal in enumerate(results['modality']):
+ width, height = results['width'][i], results['height'][i]
+ video = results['video'][i].transpose(1, 2, 3, 0)
+ num_frames = video.shape[-1]
+ video = video.reshape(height, width, -1)
+ if width < height:
+ width, height = self.length, int(self.length * height / width)
+ else:
+ width, height = int(self.length * width / height), self.length
+ video = mmcv.imresize(video,
+ (width,
+ height)).reshape(height, width, -1,
+ num_frames)
+ results['video'][i] = video.transpose(3, 0, 1, 2)
+ results['width'][i], results['height'][i] = width, height
+ return results
+
+
+@PIPELINES.register_module()
+class MultiFrameBBoxMerge:
+ """Compute the union of bboxes in selected frames.
+
+ Required keys: 'bbox'.
+
+ Modified keys: 'bbox'.
+ """
+
+ def __init__(self):
+ pass
+
+ def __call__(self, results):
+ if 'bbox' not in results:
+ return results
+
+ bboxes = list(filter(lambda x: len(x), results['bbox']))
+ if len(bboxes) == 0:
+ bbox_xyxy = np.array(
+ (0, 0, results['width'][0] - 1, results['height'][0] - 1))
+ else:
+ bboxes_xyxy = np.stack([b[0]['bbox'] for b in bboxes])
+ bbox_xyxy = np.array((
+ bboxes_xyxy[:, 0].min(),
+ bboxes_xyxy[:, 1].min(),
+ bboxes_xyxy[:, 2].max(),
+ bboxes_xyxy[:, 3].max(),
+ ))
+ results['bbox'] = bbox_xyxy
+ return results
+
+
+@PIPELINES.register_module()
+class ResizedCropByBBox:
+ """Spatial crop for spatially aligned videos by bounding box.
+
+ Required keys: 'video', 'modality', 'width', 'height', 'bbox'.
+
+ Modified keys: 'video', 'width', 'height'.
+ """
+
+ def __init__(self, size, scale=(1, 1), ratio=(1, 1), shift=0):
+ self.size = size if isinstance(size, (tuple, list)) else (size, size)
+ self.scale = scale
+ self.ratio = ratio
+ self.shift = shift
+
+ def __call__(self, results):
+ bbox_xywh = bbox_xyxy2xywh(results['bbox'][None, :])[0]
+ length = bbox_xywh[2:].max()
+ length = length * np.random.uniform(*self.scale)
+ x = bbox_xywh[0] + np.random.uniform(-self.shift, self.shift) * length
+ y = bbox_xywh[1] + np.random.uniform(-self.shift, self.shift) * length
+ w, h = length, length * np.random.uniform(*self.ratio)
+
+ bbox_xyxy = bbox_xywh2xyxy(np.array([[x, y, w, h]]))[0]
+ bbox_xyxy = bbox_xyxy.clip(min=0)
+ bbox_xyxy[2] = min(bbox_xyxy[2], results['width'][0])
+ bbox_xyxy[3] = min(bbox_xyxy[3], results['height'][0])
+ bbox_xyxy = bbox_xyxy.astype(np.int32)
+
+ for i in range(len(results['video'])):
+ video = results['video'][i].transpose(1, 2, 3, 0)
+ num_frames = video.shape[-1]
+ video = video.reshape(video.shape[0], video.shape[1], -1)
+ video = mmcv.imcrop(video, bbox_xyxy)
+ video = mmcv.imresize(video, self.size)
+
+ results['video'][i] = video.reshape(video.shape[0], video.shape[1],
+ -1, num_frames)
+ results['video'][i] = results['video'][i].transpose(3, 0, 1, 2)
+ results['width'][i], results['height'][i] = video.shape[
+ 1], video.shape[0]
+
+ return results
+
+
+@PIPELINES.register_module()
+class GestureRandomFlip:
+ """Data augmentation by randomly horizontal flip the video. The label will
+ be alternated simultaneously.
+
+ Required keys: 'video', 'label', 'ann_info'.
+
+ Modified keys: 'video', 'label'.
+ """
+
+ def __init__(self, prob=0.5):
+ self.flip_prob = prob
+
+ def __call__(self, results):
+ flip = np.random.rand() < self.flip_prob
+ if flip:
+ for i in range(len(results['video'])):
+ results['video'][i] = results['video'][i][:, :, ::-1, :]
+ for flip_pairs in results['ann_info']['flip_pairs']:
+ if results['label'] in flip_pairs:
+ results['label'] = sum(flip_pairs) - results['label']
+ break
+
+ results['flipped'] = flip
+ return results
+
+
+@PIPELINES.register_module()
+class VideoColorJitter:
+ """Data augmentation with random color transformations.
+
+ Required keys: 'video', 'modality'.
+
+ Modified keys: 'video'.
+ """
+
+ def __init__(self, brightness=0, contrast=0):
+ self.brightness = brightness
+ self.contrast = contrast
+
+ def __call__(self, results):
+ for i, modal in enumerate(results['modality']):
+ if modal == 'rgb':
+ video = results['video'][i]
+ bright = np.random.uniform(
+ max(0, 1 - self.brightness), 1 + self.brightness)
+ contrast = np.random.uniform(
+ max(0, 1 - self.contrast), 1 + self.contrast)
+ video = mmcv.adjust_brightness(video.astype(np.int32), bright)
+ num_frames = video.shape[0]
+ video = video.astype(np.uint8).reshape(-1, video.shape[2], 3)
+ video = mmcv.adjust_contrast(video, contrast).reshape(
+ num_frames, -1, video.shape[1], 3)
+ results['video'][i] = video
+ return results
+
+
+@PIPELINES.register_module()
+class RandomAlignedSpatialCrop:
+ """Data augmentation with random spatial crop for spatially aligned videos.
+
+ Required keys: 'video', 'modality', 'width', 'height'.
+
+ Modified keys: 'video', 'width', 'height'.
+ """
+
+ def __init__(self, length: int = 224):
+ self.length = length
+
+ def __call__(self, results):
+ """Implement data augmentation with random spatial crop."""
+ assert len(set(results['height'])) == 1, \
+ f"the heights {results['height']} are not identical."
+ assert len(set(results['width'])) == 1, \
+ f"the widths {results['width']} are not identical."
+ height, width = results['height'][0], results['width'][0]
+ for i, modal in enumerate(results['modality']):
+ video = results['video'][i].transpose(1, 2, 3, 0)
+ num_frames = video.shape[-1]
+ video = video.reshape(height, width, -1)
+ start_h, start_w = np.random.randint(
+ height - self.length + 1), np.random.randint(width -
+ self.length + 1)
+ video = mmcv.imcrop(
+ video,
+ np.array((start_w, start_h, start_w + self.length - 1,
+ start_h + self.length - 1)))
+ results['video'][i] = video.reshape(self.length, self.length, -1,
+ num_frames).transpose(
+ 3, 0, 1, 2)
+ results['width'][i], results['height'][
+ i] = self.length, self.length
+ return results
+
+
+@PIPELINES.register_module()
+class CenterSpatialCrop:
+ """Data processing by crop the center region of a video.
+
+ Required keys: 'video', 'modality', 'width', 'height'.
+
+ Modified keys: 'video', 'width', 'height'.
+ """
+
+ def __init__(self, length: int = 224):
+ self.length = length
+
+ def __call__(self, results):
+ """Implement data processing with center crop."""
+ for i, modal in enumerate(results['modality']):
+ height, width = results['height'][i], results['width'][i]
+ video = results['video'][i].transpose(1, 2, 3, 0)
+ num_frames = video.shape[-1]
+ video = video.reshape(height, width, -1)
+ start_h, start_w = (height - self.length) // 2, (width -
+ self.length) // 2
+ video = mmcv.imcrop(
+ video,
+ np.array((start_w, start_h, start_w + self.length - 1,
+ start_h + self.length - 1)))
+ results['video'][i] = video.reshape(self.length, self.length, -1,
+ num_frames).transpose(
+ 3, 0, 1, 2)
+ results['width'][i], results['height'][
+ i] = self.length, self.length
+ return results
+
+
+@PIPELINES.register_module()
+class ModalWiseChannelProcess:
+ """Video channel processing according to modality.
+
+ Required keys: 'video', 'modality'.
+
+ Modified keys: 'video'.
+ """
+
+ def __init__(self):
+ pass
+
+ def __call__(self, results):
+ """Implement channel processing for video array."""
+ for i, modal in enumerate(results['modality']):
+ if modal == 'rgb':
+ results['video'][i] = results['video'][i][..., ::-1]
+ elif modal == 'depth':
+ if results['video'][i].ndim == 4:
+ results['video'][i] = results['video'][i][..., :1]
+ elif results['video'][i].ndim == 3:
+ results['video'][i] = results['video'][i][..., None]
+ elif modal == 'flow':
+ results['video'][i] = results['video'][i][..., :2]
+ else:
+ raise ValueError(f'modality {modal} is invalid.')
+ return results
+
+
+@PIPELINES.register_module()
+class MultiModalVideoToTensor:
+ """Data processing by converting video arrays to pytorch tensors.
+
+ Required keys: 'video', 'modality'.
+
+ Modified keys: 'video'.
+ """
+
+ def __init__(self):
+ pass
+
+ def __call__(self, results):
+ """Implement data processing similar to ToTensor."""
+ for i, modal in enumerate(results['modality']):
+ video = results['video'][i].transpose(3, 0, 1, 2)
+ results['video'][i] = torch.tensor(
+ np.ascontiguousarray(video), dtype=torch.float) / 255.0
+ return results
+
+
+@PIPELINES.register_module()
+class VideoNormalizeTensor:
+ """Data processing by normalizing video tensors with mean and std.
+
+ Required keys: 'video', 'modality'.
+
+ Modified keys: 'video'.
+ """
+
+ def __init__(self, mean, std):
+ self.mean = torch.tensor(mean)
+ self.std = torch.tensor(std)
+
+ def __call__(self, results):
+ """Implement data normalization."""
+ for i, modal in enumerate(results['modality']):
+ if modal == 'rgb':
+ video = results['video'][i]
+ dim = video.ndim - 1
+ video = video - self.mean.view(3, *((1, ) * dim))
+ video = video / self.std.view(3, *((1, ) * dim))
+ results['video'][i] = video
+ return results
diff --git a/mmpose/datasets/pipelines/loading.py b/mmpose/datasets/pipelines/loading.py
index d6374274ad..a19d220cc9 100644
--- a/mmpose/datasets/pipelines/loading.py
+++ b/mmpose/datasets/pipelines/loading.py
@@ -99,3 +99,81 @@ def __repr__(self):
f"color_type='{self.color_type}', "
f'file_client_args={self.file_client_args})')
return repr_str
+
+
+@PIPELINES.register_module()
+class LoadVideoFromFile:
+ """Loading video(s) from file.
+
+ Required key: "video_file".
+
+ Added key: "video".
+
+ Args:
+ to_float32 (bool): Whether to convert the loaded video to a float32
+ numpy array. If set to False, the loaded video is an uint8 array.
+ Defaults to False.
+ file_client_args (dict): Arguments to instantiate a FileClient.
+ See :class:`mmcv.fileio.FileClient` for details.
+ Defaults to ``dict(backend='disk')``.
+ """
+
+ def __init__(self,
+ to_float32=False,
+ file_client_args=dict(backend='disk')):
+ self.to_float32 = to_float32
+ self.file_client_args = file_client_args.copy()
+ self.file_client = None
+
+ def _read_video(self, path):
+ container = mmcv.VideoReader(path)
+ sample = dict(
+ height=int(container.height),
+ width=int(container.width),
+ fps=int(container.fps),
+ num_frames=int(container.frame_cnt),
+ video=[])
+ for _ in range(container.frame_cnt):
+ sample['video'].append(container.read())
+ sample['video'] = np.stack(sample['video'], axis=0)
+ return sample
+
+ def __call__(self, results):
+ """Loading video(s) from file."""
+ if self.file_client is None:
+ self.file_client = mmcv.FileClient(**self.file_client_args)
+
+ video_file = results.get('video_file', None)
+
+ if isinstance(video_file, (list, tuple)):
+ # Load videos from a list of paths
+ for path in video_file:
+ video = self._read_video(path)
+ for key in video:
+ results[key].append(video[key])
+ elif video_file is not None:
+ # Load single video from path
+ results.update(self._read_video(video_file))
+ else:
+ if 'video' not in results:
+ # If `video_file`` is not in results, check the `video` exists
+ # and format the image. This for compatibility when the image
+ # is manually set outside the pipeline.
+ raise KeyError('Either `video_file` or `video` should exist '
+ 'in results.')
+ if isinstance(results['video'], (list, tuple)):
+ assert isinstance(results['video'][0], np.ndarray)
+ else:
+ assert isinstance(results['video'], np.ndarray)
+ results['video'] = [results['video']]
+
+ results['num_frames'] = [v.shape[0] for v in results['video']]
+ results['height'] = [v.shape[1] for v in results['video']]
+ results['width'] = [v.shape[2] for v in results['video']]
+ return results
+
+ def __repr__(self):
+ repr_str = (f'{self.__class__.__name__}('
+ f'to_float32={self.to_float32}, '
+ f'file_client_args={self.file_client_args})')
+ return repr_str
diff --git a/mmpose/models/backbones/__init__.py b/mmpose/models/backbones/__init__.py
index cb2498560a..09745d443c 100644
--- a/mmpose/models/backbones/__init__.py
+++ b/mmpose/models/backbones/__init__.py
@@ -5,6 +5,7 @@
from .hourglass_ae import HourglassAENet
from .hrformer import HRFormer
from .hrnet import HRNet
+from .i3d import I3D
from .litehrnet import LiteHRNet
from .mobilenet_v2 import MobileNetV2
from .mobilenet_v3 import MobileNetV3
@@ -33,5 +34,5 @@
'SEResNet', 'SEResNeXt', 'ShuffleNetV1', 'ShuffleNetV2', 'CPM', 'RSN',
'MSPN', 'ResNeSt', 'VGG', 'TCN', 'ViPNAS_ResNet', 'ViPNAS_MobileNetV3',
'LiteHRNet', 'V2VNet', 'HRFormer', 'PyramidVisionTransformer',
- 'PyramidVisionTransformerV2', 'SwinTransformer'
+ 'PyramidVisionTransformerV2', 'SwinTransformer', 'I3D'
]
diff --git a/mmpose/models/backbones/i3d.py b/mmpose/models/backbones/i3d.py
new file mode 100644
index 0000000000..64f330abac
--- /dev/null
+++ b/mmpose/models/backbones/i3d.py
@@ -0,0 +1,215 @@
+# Copyright (c) OpenMMLab. All rights reserved.
+# Code is modified from `Third-party pytorch implementation of i3d
+# `.
+
+import torch
+import torch.nn as nn
+
+from ..builder import BACKBONES
+from .base_backbone import BaseBackbone
+
+
+class Conv3dBlock(nn.Module):
+ """Basic 3d convolution block for I3D.
+
+ Args:
+ in_channels (int): Input channels of this block.
+ out_channels (int): Output channels of this block.
+ expansion (float): The multiplier of in_channels and out_channels.
+ Default: 1.
+ kernel_size (tuple[int]): kernel size of the 3d convolution layer.
+ Default: (1, 1, 1).
+ stride (tuple[int]): stride of the block. Default: (1, 1, 1)
+ padding (tuple[int]): padding of the input tensor. Default: (0, 0, 0)
+ use_bias (bool): whether to enable bias in 3d convolution layer.
+ Default: False
+ use_bn (bool): whether to use Batch Normalization after 3d convolution
+ layer. Default: True
+ use_relu (bool): whether to use ReLU after Batch Normalization layer.
+ Default: True
+ """
+
+ def __init__(self,
+ in_channels,
+ out_channels,
+ expansion=1.0,
+ kernel_size=(1, 1, 1),
+ stride=(1, 1, 1),
+ padding=(0, 0, 0),
+ use_bias=False,
+ use_bn=True,
+ use_relu=True):
+ super().__init__()
+
+ in_channels = int(in_channels * expansion)
+ out_channels = int(out_channels * expansion)
+
+ self.conv3d = nn.Conv3d(
+ in_channels,
+ out_channels,
+ kernel_size,
+ padding=padding,
+ stride=stride,
+ bias=use_bias)
+
+ self.use_bn = use_bn
+ self.use_relu = use_relu
+
+ if self.use_bn:
+ self.batch3d = nn.BatchNorm3d(out_channels)
+
+ if self.use_relu:
+ self.activation = nn.ReLU(inplace=True)
+
+ def forward(self, x):
+ """Forward function."""
+ out = self.conv3d(x)
+ if self.use_bn:
+ out = self.batch3d(out)
+ if self.use_relu:
+ out = self.activation(out)
+ return out
+
+
+class Mixed(nn.Module):
+ """Inception block for I3D.
+
+ Args:
+ in_channels (int): Input channels of this block.
+ out_channels (int): Output channels of this block.
+ expansion (float): The multiplier of in_channels and out_channels.
+ Default: 1.
+ """
+
+ def __init__(self, in_channels, out_channels, expansion=1.0):
+ super(Mixed, self).__init__()
+ # Branch 0
+ self.branch_0 = Conv3dBlock(
+ in_channels, out_channels[0], expansion, kernel_size=(1, 1, 1))
+
+ # Branch 1
+ branch_1_conv1 = Conv3dBlock(
+ in_channels, out_channels[1], expansion, kernel_size=(1, 1, 1))
+ branch_1_conv2 = Conv3dBlock(
+ out_channels[1],
+ out_channels[2],
+ expansion,
+ kernel_size=(3, 3, 3),
+ padding=(1, 1, 1))
+ self.branch_1 = nn.Sequential(branch_1_conv1, branch_1_conv2)
+
+ # Branch 2
+ branch_2_conv1 = Conv3dBlock(
+ in_channels, out_channels[3], expansion, kernel_size=(1, 1, 1))
+ branch_2_conv2 = Conv3dBlock(
+ out_channels[3],
+ out_channels[4],
+ expansion,
+ kernel_size=(3, 3, 3),
+ padding=(1, 1, 1))
+ self.branch_2 = nn.Sequential(branch_2_conv1, branch_2_conv2)
+
+ # Branch3
+ branch_3_pool = nn.MaxPool3d(
+ kernel_size=(3, 3, 3),
+ stride=(1, 1, 1),
+ padding=(1, 1, 1),
+ ceil_mode=True)
+ branch_3_conv2 = Conv3dBlock(
+ in_channels, out_channels[5], expansion, kernel_size=(1, 1, 1))
+ self.branch_3 = nn.Sequential(branch_3_pool, branch_3_conv2)
+
+ def forward(self, x):
+ """Forward function."""
+ out_0 = self.branch_0(x)
+ out_1 = self.branch_1(x)
+ out_2 = self.branch_2(x)
+ out_3 = self.branch_3(x)
+ out = torch.cat((out_0, out_1, out_2, out_3), 1)
+ return out
+
+
+@BACKBONES.register_module()
+class I3D(BaseBackbone):
+ """I3D backbone.
+
+ Please refer to the `paper `__ for
+ details.
+
+ Args:
+ in_channels (int): Input channels of the backbone, which is decided
+ on the input modality.
+ expansion (float): The multiplier of in_channels and out_channels.
+ Default: 1.
+ """
+
+ def __init__(self, in_channels=3, expansion=1.0):
+ super(I3D, self).__init__()
+
+ # expansion must be an integer multiple of 1/8
+ expansion = round(8 * expansion) / 8.0
+
+ # xut Layer
+ self.conv3d_1a_7x7 = Conv3dBlock(
+ out_channels=64,
+ in_channels=in_channels / expansion,
+ expansion=expansion,
+ kernel_size=(7, 7, 7),
+ stride=(2, 2, 2),
+ padding=(2, 3, 3))
+ self.maxPool3d_2a_3x3 = nn.MaxPool3d(
+ kernel_size=(1, 3, 3), stride=(1, 2, 2), padding=(0, 1, 1))
+
+ # Layer 2
+ self.conv3d_2b_1x1 = Conv3dBlock(
+ out_channels=64,
+ in_channels=64,
+ expansion=expansion,
+ kernel_size=(1, 1, 1))
+ self.conv3d_2c_3x3 = Conv3dBlock(
+ out_channels=192,
+ in_channels=64,
+ expansion=expansion,
+ kernel_size=(3, 3, 3),
+ padding=(1, 1, 1))
+ self.maxPool3d_3a_3x3 = nn.MaxPool3d(
+ kernel_size=(1, 3, 3), stride=(1, 2, 2), padding=(0, 1, 1))
+
+ # Mixed_3b
+ self.mixed_3b = Mixed(192, [64, 96, 128, 16, 32, 32], expansion)
+ self.mixed_3c = Mixed(256, [128, 128, 192, 32, 96, 64], expansion)
+ self.maxPool3d_4a_3x3 = nn.MaxPool3d(
+ kernel_size=(3, 3, 3), stride=(2, 2, 2), padding=(1, 1, 1))
+
+ # Mixed 4
+ self.mixed_4b = Mixed(480, [192, 96, 208, 16, 48, 64], expansion)
+ self.mixed_4c = Mixed(512, [160, 112, 224, 24, 64, 64], expansion)
+ self.mixed_4d = Mixed(512, [128, 128, 256, 24, 64, 64], expansion)
+ self.mixed_4e = Mixed(512, [112, 144, 288, 32, 64, 64], expansion)
+ self.mixed_4f = Mixed(528, [256, 160, 320, 32, 128, 128], expansion)
+
+ self.maxPool3d_5a_2x2 = nn.MaxPool3d(
+ kernel_size=(2, 2, 2), stride=(2, 2, 2), padding=(0, 0, 0))
+
+ # Mixed 5
+ self.mixed_5b = Mixed(832, [256, 160, 320, 32, 128, 128], expansion)
+ self.mixed_5c = Mixed(832, [384, 192, 384, 48, 128, 128], expansion)
+
+ def forward(self, x):
+ out = self.conv3d_1a_7x7(x)
+ out = self.maxPool3d_2a_3x3(out)
+ out = self.conv3d_2b_1x1(out)
+ out = self.conv3d_2c_3x3(out)
+ out = self.maxPool3d_3a_3x3(out)
+ out = self.mixed_3b(out)
+ out = self.mixed_3c(out)
+ out = self.maxPool3d_4a_3x3(out)
+ out = self.mixed_4b(out)
+ out = self.mixed_4c(out)
+ out = self.mixed_4d(out)
+ out = self.mixed_4e(out)
+ out = self.mixed_4f(out)
+ out = self.maxPool3d_5a_2x2(out)
+ out = self.mixed_5b(out)
+ out = self.mixed_5c(out)
+ return out
diff --git a/mmpose/models/detectors/__init__.py b/mmpose/models/detectors/__init__.py
index 66e575e2f2..d94d8b8aab 100644
--- a/mmpose/models/detectors/__init__.py
+++ b/mmpose/models/detectors/__init__.py
@@ -1,5 +1,6 @@
# Copyright (c) OpenMMLab. All rights reserved.
from .associative_embedding import AssociativeEmbedding
+from .gesture_recognizer import GestureRecognizer
from .interhand_3d import Interhand3D
from .mesh import ParametricMesh
from .multi_task import MultiTask
@@ -12,5 +13,5 @@
__all__ = [
'TopDown', 'AssociativeEmbedding', 'ParametricMesh', 'MultiTask',
'PoseLifter', 'Interhand3D', 'PoseWarper', 'DetectAndRegress',
- 'VoxelCenterDetector', 'VoxelSinglePose'
+ 'VoxelCenterDetector', 'VoxelSinglePose', 'GestureRecognizer'
]
diff --git a/mmpose/models/detectors/gesture_recognizer.py b/mmpose/models/detectors/gesture_recognizer.py
new file mode 100644
index 0000000000..f99cd04f30
--- /dev/null
+++ b/mmpose/models/detectors/gesture_recognizer.py
@@ -0,0 +1,188 @@
+# Copyright (c) OpenMMLab. All rights reserved.
+import warnings
+
+import torch.nn as nn
+
+from .. import builder
+from ..builder import POSENETS
+from .base import BasePose
+
+try:
+ from mmcv.runner import auto_fp16
+except ImportError:
+ warnings.warn('auto_fp16 from mmpose will be deprecated from v0.15.0'
+ 'Please install mmcv>=1.1.4')
+ from mmpose.core import auto_fp16
+
+
+@POSENETS.register_module()
+class GestureRecognizer(BasePose):
+ """Hand gesture recognizer.
+
+ Args:
+ backbone (dict): Backbone modules to extract feature.
+ neck (dict): Neck Modules to process feature.
+ cls_head (dict): Classification head to process feature.
+ train_cfg (dict): Config for training. Default: None.
+ test_cfg (dict): Config for testing. Default: None.
+ modality (str or list or tuple): Data modality. Default: None.
+ pretrained (str): Path to the pretrained models.
+ """
+
+ def __init__(self,
+ backbone,
+ neck=None,
+ cls_head=None,
+ train_cfg=None,
+ test_cfg=None,
+ modality='rgb',
+ pretrained=None):
+ super().__init__()
+
+ self.train_cfg = train_cfg
+ self.test_cfg = test_cfg
+
+ if isinstance(modality, (tuple, list)):
+ self.modality = modality
+ else:
+ self.modality = (modality, )
+ backbone = {modality: backbone}
+ pretrained = {modality: pretrained}
+
+ # build backbone
+ self.backbone = nn.Module()
+ for modal in self.modality:
+ setattr(self.backbone, modal,
+ builder.build_backbone(backbone[modal]))
+
+ # build neck
+ if neck is not None:
+ self.neck = builder.build_neck(neck)
+
+ # build head
+ cls_head['train_cfg'] = train_cfg
+ cls_head['test_cfg'] = test_cfg
+ cls_head['modality'] = self.modality
+ self.cls_head = builder.build_head(cls_head)
+
+ self.pretrained = dict() if pretrained is None else pretrained
+ self.init_weights()
+
+ def init_weights(self, pretrained=None):
+ """Weight initialization for model."""
+ if pretrained is not None:
+ self.pretrained = pretrained
+ for modal in self.modality:
+ getattr(self.backbone,
+ modal).init_weights(self.pretrained.get(modal, None))
+ if hasattr(self, 'neck'):
+ self.neck.init_weights()
+ if hasattr(self, 'cls_head'):
+ self.cls_head.init_weights()
+
+ @auto_fp16(apply_to=('video', ))
+ def forward(self,
+ video,
+ label=None,
+ img_metas=None,
+ return_loss=True,
+ **kwargs):
+ """Calls either forward_train or forward_test depending on whether
+ return_loss=True. Note this setting will change the expected inputs.
+
+ Note:
+ - batch_size: N
+ - num_vid_channel: C (Default: 3)
+ - video height: vidH
+ - video width: vidW
+ - video length: vidL
+
+ Args:
+ video (list[torch.Tensor[NxCxvidLxvidHxvidW]]): Input videos.
+ label (torch.Tensor[N]): Category label of videos.
+ img_metas (list(dict)): Information about data.
+ By default this includes:
+ - "fps: video frame rate
+ - "modality": modality of input videos
+ return_loss (bool): Option to `return loss`. `return loss=True`
+ for training, `return loss=False` for validation & test.
+
+ Returns:
+ dict|tuple: if `return loss` is true, then return losses. \
+ Otherwise, return predicted gestures for clips with \
+ a certain length. \
+ .
+ """
+ if not isinstance(img_metas, (tuple, list)):
+ img_metas = [img_metas.data]
+ if return_loss:
+ return self.forward_train(video, label, img_metas[0], **kwargs)
+ return self.forward_test(video, label, img_metas[0], **kwargs)
+
+ def _feed_forward(self, video, img_metas):
+ """Feed videos into network to compute feature maps and logits.
+
+ Note:
+ - batch_size: N
+ - num_vid_channel: C (Default: 3)
+ - video height: vidH
+ - video width: vidW
+ - video length: vidL
+
+ Args:
+ video (list[torch.Tensor[NxCxvidLxvidHxvidW]]): Input videos.
+ img_metas (list(dict)): Information about data.
+ By default this includes:
+ - "fps: video frame rate
+ - "modality": modality of input videos
+
+ Returns:
+ tuple[Tensor, Tensor]: output logit and feature map.
+ """
+ fmaps = []
+ for i, modal in enumerate(img_metas['modality']):
+ fmaps.append(getattr(self.backbone, modal)(video[i]))
+
+ if hasattr(self, 'neck'):
+ fmaps = [self.neck(fmap) for fmap in fmaps]
+
+ if hasattr(self, 'cls_head'):
+ logits = self.cls_head(fmaps, img_metas)
+ else:
+ return None, fmaps
+
+ return logits, fmaps
+
+ def forward_train(self, video, label, img_metas, **kwargs):
+ """Defines the computation performed at every call when training."""
+ logits, fmaps = self._feed_forward(video, img_metas)
+
+ # if return loss
+ losses = dict()
+ if hasattr(self, 'cls_head'):
+ cls_losses = self.cls_head.get_loss(logits, label, fmaps=fmaps)
+ losses.update(cls_losses)
+ cls_accuracy = self.cls_head.get_accuracy(logits, label, img_metas)
+ losses.update(cls_accuracy)
+
+ return losses
+
+ def forward_test(self, video, label, img_metas, **kwargs):
+ """Defines the computation performed at every call when testing."""
+ results = dict(logits=dict())
+ logits, _ = self._feed_forward(video, img_metas)
+ for i, modal in enumerate(img_metas['modality']):
+ results['logits'][modal] = logits[i]
+ results['label'] = label
+ return results
+
+ def set_train_epoch(self, epoch: int):
+ """set the training epoch of heads to support customized behaviour."""
+ if hasattr(self, 'cls_head'):
+ self.cls_head.set_train_epoch(epoch)
+
+ def forward_dummy(self, video):
+ raise NotImplementedError
+
+ def show_result(self, video, result, **kwargs):
+ raise NotImplementedError
diff --git a/mmpose/models/heads/__init__.py b/mmpose/models/heads/__init__.py
index a98e91140e..459c20b8bd 100644
--- a/mmpose/models/heads/__init__.py
+++ b/mmpose/models/heads/__init__.py
@@ -6,6 +6,7 @@
from .deeppose_regression_head import DeepposeRegressionHead
from .hmr_head import HMRMeshHead
from .interhand_3d_head import Interhand3DHead
+from .mtut_head import MultiModalSSAHead
from .temporal_regression_head import TemporalRegressionHead
from .topdown_heatmap_base_head import TopdownHeatmapBaseHead
from .topdown_heatmap_multi_stage_head import (TopdownHeatmapMSMUHead,
@@ -20,5 +21,5 @@
'AEHigherResolutionHead', 'AESimpleHead', 'AEMultiStageHead',
'DeepposeRegressionHead', 'TemporalRegressionHead', 'Interhand3DHead',
'HMRMeshHead', 'DeconvHead', 'ViPNASHeatmapSimpleHead', 'CuboidCenterHead',
- 'CuboidPoseHead'
+ 'CuboidPoseHead', 'MultiModalSSAHead'
]
diff --git a/mmpose/models/heads/mtut_head.py b/mmpose/models/heads/mtut_head.py
new file mode 100644
index 0000000000..4931d6d436
--- /dev/null
+++ b/mmpose/models/heads/mtut_head.py
@@ -0,0 +1,155 @@
+# Copyright (c) OpenMMLab. All rights reserved.
+import itertools
+
+import torch
+import torch.nn as nn
+from mmcv.cnn import xavier_init
+
+from ..builder import HEADS
+
+
+@HEADS.register_module()
+class MultiModalSSAHead(nn.Module):
+ """Sparial-temporal Semantic Alignment Head proposed in "Improving the
+ performance of unimodal dynamic hand-gesture recognition with multimodal
+ training",
+
+ Please refer to the `paper `__ for
+ details.
+
+ Args:
+ num_classes (int): number of classes.
+ modality (list[str]): modalities of input videos for backbone.
+ in_channels (int): number of channels of feature maps. Default: 1024
+ avg_pool_kernel (tuple[int]): kernel size of pooling layer.
+ Default: (1, 7, 7)
+ dropout_prob (float): probablity to use dropout on input feature map.
+ Default: 0
+ train_cfg (dict): training config.
+ test_cfg (dict): testing config.
+ """
+
+ def __init__(self,
+ num_classes,
+ modality,
+ in_channels=1024,
+ avg_pool_kernel=(1, 7, 7),
+ dropout_prob=0.0,
+ train_cfg=None,
+ test_cfg=None,
+ **kwargs):
+ super().__init__()
+
+ self.modality = modality
+ self.train_cfg = train_cfg
+ self.test_cfg = test_cfg
+
+ # build sub modules
+ self.avg_pool = nn.AvgPool3d(avg_pool_kernel, (1, 1, 1))
+ self.dropout = nn.Dropout(dropout_prob)
+ self.output_conv = nn.Module()
+ for modal in self.modality:
+ conv3d = nn.Conv3d(in_channels, num_classes, (1, 1, 1))
+ setattr(self.output_conv, modal, conv3d)
+ self.loss = nn.CrossEntropyLoss(reduction='none')
+
+ # parameters for ssa loss
+ self.beta = self.train_cfg.get('beta', 2.0)
+ self.lambda_ = self.train_cfg.get('lambda_', 5e-3)
+ self.start_epoch = self.train_cfg.get('ssa_start_epoch', 1e6)
+ self._train_epoch = 0
+
+ def init_weights(self):
+ """Initialize model weights."""
+ for m in self.output_conv.modules():
+ if isinstance(m, nn.Conv3d):
+ xavier_init(m)
+
+ def set_train_epoch(self, epoch: int):
+ """set the epoch to control the activation of SSA loss."""
+ self._train_epoch = epoch
+
+ def forward(self, x, img_metas):
+ """Forward function."""
+ logits = []
+ for i, modal in enumerate(img_metas['modality']):
+ out = self.avg_pool(x[i])
+ out = self.dropout(out)
+ out = getattr(self.output_conv, modal)(out)
+ out = out.mean(3).mean(3)
+ logits.append(out)
+ return logits
+
+ @staticmethod
+ def _compute_corr(fmap):
+ """compute the self-correlation matrix of feature map."""
+ fmap = fmap.view(fmap.size(0), fmap.size(1), -1)
+ fmap = nn.functional.normalize(fmap, dim=2, eps=1e-8)
+ corr = torch.bmm(fmap.permute(0, 2, 1), fmap)
+ return corr.view(corr.size(0), -1)
+
+ def get_loss(self, logits, label, fmaps=None):
+ """Compute the Cross Entropy loss and SSA loss.
+
+ Note:
+ - batch_size: N
+ - number of classes: nC
+ - feature map channel: C
+ - feature map height: H
+ - feature map width: W
+ - feature map length: L
+ - logit length: Lg
+
+ Args:
+ logits (list[NxnCxLg]): predicted logits for each modality.
+ label (list(dict)): Category label.
+ fmaps (list[torch.Tensor[NxCxLxHxW]]): feature maps for each
+ modality.
+
+ Returns:
+ dict[str, torch.tensor]: computed losses.
+ """
+ losses = {}
+ ce_loss = [self.loss(logit.mean(dim=2), label) for logit in logits]
+
+ if self._train_epoch >= self.start_epoch:
+ ssa_loss = []
+ corrs = [self._compute_corr(fmap) for fmap in fmaps]
+ for idx1, idx2 in itertools.combinations(range(len(fmaps)), 2):
+ for i, j in ((idx1, idx2), (idx2, idx1)):
+ rho = (ce_loss[i] - ce_loss[j]).clamp(min=0)
+ rho = (torch.exp(self.beta * rho) - 1).detach()
+ ssa = corrs[i] - corrs[j].detach()
+ ssa = rho * ssa.pow(2).mean(dim=1).pow(0.5)
+ ssa_loss.append((ssa.mean() * self.lambda_).clamp(max=10))
+ losses['ssa_loss'] = sum(ssa_loss)
+ ce_loss = [loss.mean() for loss in ce_loss]
+ losses['ce_loss'] = sum(ce_loss)
+
+ return losses
+
+ def get_accuracy(self, logits, label, img_metas):
+ """Compute the accuracy of predicted gesture.
+
+ Note:
+ - batch_size: N
+ - number of classes: nC
+ - logit length: L
+
+ Args:
+ logits (list[NxnCxL]): predicted logits for each modality.
+ label (list(dict)): Category label.
+ img_metas (list(dict)): Information about data.
+ By default this includes:
+ - "fps: video frame rate
+ - "modality": modality of input videos
+
+ Returns:
+ dict[str, torch.tensor]: computed accuracy for each modality.
+ """
+ results = {}
+ for i, modal in enumerate(img_metas['modality']):
+ logit = logits[i].mean(dim=2)
+ acc = (logit.argmax(dim=1) == label).float().mean()
+ results[f'acc_{modal}'] = acc.item()
+ return results
diff --git a/model-index.yml b/model-index.yml
index b3bcd91e3c..500bac9e30 100644
--- a/model-index.yml
+++ b/model-index.yml
@@ -134,6 +134,7 @@ Import:
- configs/hand/2d_kpt_sview_rgb_img/topdown_heatmap/rhd2d/mobilenetv2_rhd2d.yml
- configs/hand/2d_kpt_sview_rgb_img/topdown_heatmap/rhd2d/resnet_rhd2d.yml
- configs/hand/3d_kpt_sview_rgb_img/internet/interhand3d/internet_interhand3d.yml
+- configs/hand/gesture_sview_rgbd_vid/mtut/nvgesture/i3d_nvgesture.yml
- configs/wholebody/2d_kpt_sview_rgb_img/associative_embedding/coco-wholebody/higherhrnet_coco-wholebody.yml
- configs/wholebody/2d_kpt_sview_rgb_img/associative_embedding/coco-wholebody/hrnet_coco-wholebody.yml
- configs/wholebody/2d_kpt_sview_rgb_img/topdown_heatmap/coco-wholebody/hrnet_coco-wholebody.yml
diff --git a/tests/data/nvgesture/bboxes.json b/tests/data/nvgesture/bboxes.json
new file mode 100644
index 0000000000..f6c1c06692
--- /dev/null
+++ b/tests/data/nvgesture/bboxes.json
@@ -0,0 +1,344 @@
+{
+ "": [
+ [
+ {
+ "bbox": {
+ "__ndarray__": [
+ 88.10102081298828,
+ 96.01033020019531,
+ 198.7417449951172,
+ 221.09027099609375,
+ 0.9996840953826904
+ ],
+ "dtype": "float32",
+ "shape": [
+ 5
+ ]
+ }
+ }
+ ],
+ [
+ {
+ "bbox": {
+ "__ndarray__": [
+ 96.0768814086914,
+ 98.94043731689453,
+ 205.73648071289062,
+ 231.98422241210938,
+ 0.9979396462440491
+ ],
+ "dtype": "float32",
+ "shape": [
+ 5
+ ]
+ }
+ }
+ ],
+ [
+ {
+ "bbox": {
+ "__ndarray__": [
+ 112.53093719482422,
+ 100.84430694580078,
+ 225.46414184570312,
+ 218.37539672851562,
+ 0.9948186278343201
+ ],
+ "dtype": "float32",
+ "shape": [
+ 5
+ ]
+ }
+ }
+ ],
+ [
+ {
+ "bbox": {
+ "__ndarray__": [
+ 140.26339721679688,
+ 100.93962097167969,
+ 239.01219177246094,
+ 213.0036163330078,
+ 0.9963332414627075
+ ],
+ "dtype": "float32",
+ "shape": [
+ 5
+ ]
+ }
+ }
+ ],
+ [
+ {
+ "bbox": {
+ "__ndarray__": [
+ 162.32077026367188,
+ 93.03462982177734,
+ 246.34483337402344,
+ 211.05172729492188,
+ 0.9872151613235474
+ ],
+ "dtype": "float32",
+ "shape": [
+ 5
+ ]
+ }
+ }
+ ],
+ [
+ {
+ "bbox": {
+ "__ndarray__": [
+ 169.93429565429688,
+ 92.26083374023438,
+ 275.7470703125,
+ 212.19854736328125,
+ 0.9966674447059631
+ ],
+ "dtype": "float32",
+ "shape": [
+ 5
+ ]
+ }
+ }
+ ],
+ [
+ {
+ "bbox": {
+ "__ndarray__": [
+ 177.5313720703125,
+ 105.4382095336914,
+ 316.52239990234375,
+ 208.23284912109375,
+ 0.9904665350914001
+ ],
+ "dtype": "float32",
+ "shape": [
+ 5
+ ]
+ }
+ }
+ ],
+ [
+ {
+ "bbox": {
+ "__ndarray__": [
+ 177.9888916015625,
+ 114.24292755126953,
+ 313.8994445800781,
+ 205.98202514648438,
+ 0.9988754391670227
+ ],
+ "dtype": "float32",
+ "shape": [
+ 5
+ ]
+ }
+ }
+ ],
+ [
+ {
+ "bbox": {
+ "__ndarray__": [
+ 177.15512084960938,
+ 121.3445816040039,
+ 306.1671142578125,
+ 205.3945770263672,
+ 0.9995057582855225
+ ],
+ "dtype": "float32",
+ "shape": [
+ 5
+ ]
+ }
+ }
+ ],
+ [
+ {
+ "bbox": {
+ "__ndarray__": [
+ 173.60049438476562,
+ 124.11859130859375,
+ 303.1548767089844,
+ 209.4412841796875,
+ 0.9997778534889221
+ ],
+ "dtype": "float32",
+ "shape": [
+ 5
+ ]
+ }
+ }
+ ],
+ [
+ {
+ "bbox": {
+ "__ndarray__": [
+ 167.17393493652344,
+ 126.2450180053711,
+ 297.64093017578125,
+ 211.53280639648438,
+ 0.9993647933006287
+ ],
+ "dtype": "float32",
+ "shape": [
+ 5
+ ]
+ }
+ }
+ ],
+ [
+ {
+ "bbox": {
+ "__ndarray__": [
+ 160.3257598876953,
+ 126.101318359375,
+ 289.13494873046875,
+ 214.8901824951172,
+ 0.9971745014190674
+ ],
+ "dtype": "float32",
+ "shape": [
+ 5
+ ]
+ }
+ }
+ ],
+ [
+ {
+ "bbox": {
+ "__ndarray__": [
+ 159.0267791748047,
+ 123.04993438720703,
+ 280.7857360839844,
+ 214.73959350585938,
+ 0.9962193369865417
+ ],
+ "dtype": "float32",
+ "shape": [
+ 5
+ ]
+ }
+ }
+ ],
+ [
+ {
+ "bbox": {
+ "__ndarray__": [
+ 149.41571044921875,
+ 118.96903991699219,
+ 254.2805938720703,
+ 214.06747436523438,
+ 0.9996248483657837
+ ],
+ "dtype": "float32",
+ "shape": [
+ 5
+ ]
+ }
+ }
+ ],
+ [
+ {
+ "bbox": {
+ "__ndarray__": [
+ 137.837890625,
+ 112.58697509765625,
+ 218.49127197265625,
+ 217.65696716308594,
+ 0.9997895359992981
+ ],
+ "dtype": "float32",
+ "shape": [
+ 5
+ ]
+ }
+ }
+ ],
+ [
+ {
+ "bbox": {
+ "__ndarray__": [
+ 128.7360382080078,
+ 106.2151107788086,
+ 207.93055725097656,
+ 220.00509643554688,
+ 0.9997987151145935
+ ],
+ "dtype": "float32",
+ "shape": [
+ 5
+ ]
+ }
+ }
+ ],
+ [
+ {
+ "bbox": {
+ "__ndarray__": [
+ 118.36389923095703,
+ 103.3556137084961,
+ 202.43719482421875,
+ 220.83615112304688,
+ 0.9996999502182007
+ ],
+ "dtype": "float32",
+ "shape": [
+ 5
+ ]
+ }
+ }
+ ],
+ [
+ {
+ "bbox": {
+ "__ndarray__": [
+ 99.54222106933594,
+ 99.98068237304688,
+ 196.3734130859375,
+ 227.61508178710938,
+ 0.999809205532074
+ ],
+ "dtype": "float32",
+ "shape": [
+ 5
+ ]
+ }
+ }
+ ],
+ [
+ {
+ "bbox": {
+ "__ndarray__": [
+ 87.78035736083984,
+ 97.02134704589844,
+ 193.37466430664062,
+ 232.29702758789062,
+ 0.9998823404312134
+ ],
+ "dtype": "float32",
+ "shape": [
+ 5
+ ]
+ }
+ }
+ ],
+ [
+ {
+ "bbox": {
+ "__ndarray__": [
+ 85.43366241455078,
+ 96.68350219726562,
+ 192.14898681640625,
+ 229.33612060546875,
+ 0.9999115467071533
+ ],
+ "dtype": "float32",
+ "shape": [
+ 5
+ ]
+ }
+ }
+ ]
+ ]
+}
\ No newline at end of file
diff --git a/tests/data/nvgesture/sk_color.avi b/tests/data/nvgesture/sk_color.avi
new file mode 100644
index 0000000000..781f348b54
Binary files /dev/null and b/tests/data/nvgesture/sk_color.avi differ
diff --git a/tests/data/nvgesture/sk_depth.avi b/tests/data/nvgesture/sk_depth.avi
new file mode 100644
index 0000000000..c8c32e54d6
Binary files /dev/null and b/tests/data/nvgesture/sk_depth.avi differ
diff --git a/tests/data/nvgesture/test_nvgesture.lst b/tests/data/nvgesture/test_nvgesture.lst
new file mode 100644
index 0000000000..b5ca38e9ad
--- /dev/null
+++ b/tests/data/nvgesture/test_nvgesture.lst
@@ -0,0 +1 @@
+path:./ depth:sk_depth:2:19 color:sk_color:1:18 duo_left:duo_left:169:262 label:5
\ No newline at end of file
diff --git a/tests/test_apis/test_inference.py b/tests/test_apis/test_inference.py
index a8b4005fed..b8088951d0 100644
--- a/tests/test_apis/test_inference.py
+++ b/tests/test_apis/test_inference.py
@@ -3,10 +3,12 @@
import os.path as osp
from glob import glob
+import json_tricks as json
import mmcv
import numpy as np
from mmpose.apis import (collect_multi_frames, inference_bottom_up_pose_model,
+ inference_gesture_model,
inference_top_down_pose_model, init_pose_model,
process_mmdet_results, vis_pose_result)
from mmpose.datasets import DatasetInfo
@@ -306,3 +308,24 @@ def test_collect_multi_frames():
_ = collect_multi_frames(video, frame_id, indices, online=True)
_ = collect_multi_frames(video, frame_id, indices, online=False)
+
+
+def test_hand_gesture_demo():
+
+ # build the pose model from a config file and a checkpoint file
+ pose_model = init_pose_model(
+ 'configs/hand/gesture_sview_rgbd_vid/mtut/nvgesture/'
+ 'i3d_nvgesture_bbox_112x112_fps15.py',
+ None,
+ device='cpu')
+
+ dataset_info = pose_model.cfg.data['test'].get('dataset_info', None)
+ video_files = [
+ 'tests/data/nvgesture/sk_color.avi',
+ 'tests/data/nvgesture/sk_depth.avi'
+ ]
+ with open('tests/data/nvgesture/bboxes.json', 'r') as f:
+ bbox = next(iter(json.load(f).values()))
+
+ pred_label, _ = inference_gesture_model(pose_model, video_files, bbox,
+ dataset_info)
diff --git a/tests/test_backbones/test_i3d.py b/tests/test_backbones/test_i3d.py
new file mode 100644
index 0000000000..8c6e68f745
--- /dev/null
+++ b/tests/test_backbones/test_i3d.py
@@ -0,0 +1,21 @@
+# Copyright (c) OpenMMLab. All rights reserved.
+import torch
+
+from mmpose.models.backbones import I3D
+
+
+def test_i3d_backbone():
+ """Test I3D backbone."""
+ model = I3D()
+ model.train()
+
+ vids = torch.randn(1, 3, 16, 112, 112)
+ feat = model(vids)
+ assert feat.shape == (1, 1024, 2, 3, 3)
+
+ model = I3D(expansion=0.5)
+ model.train()
+
+ vids = torch.randn(1, 3, 32, 224, 224)
+ feat = model(vids)
+ assert feat.shape == (1, 512, 4, 7, 7)
diff --git a/tests/test_datasets/test_gesture_dataset.py b/tests/test_datasets/test_gesture_dataset.py
new file mode 100644
index 0000000000..75d456d129
--- /dev/null
+++ b/tests/test_datasets/test_gesture_dataset.py
@@ -0,0 +1,63 @@
+# Copyright (c) OpenMMLab. All rights reserved.
+import copy
+
+import pytest
+import torch
+from mmcv import Config
+from numpy.testing import assert_almost_equal
+
+from mmpose.datasets import DATASETS
+
+
+def test_NVGesture_dataset():
+
+ dataset = 'NVGestureDataset'
+ dataset_info = Config.fromfile(
+ 'configs/_base_/datasets/nvgesture.py').dataset_info
+
+ dataset_class = DATASETS.get(dataset)
+
+ data_cfg = dict(
+ video_size=[320, 240],
+ modality=['rgb', 'depth'],
+ bbox_file='tests/data/nvgesture/bboxes.json',
+ )
+
+ # Test
+ data_cfg_copy = copy.deepcopy(data_cfg)
+ _ = dataset_class(
+ ann_file='tests/data/nvgesture/test_nvgesture.lst',
+ vid_prefix='tests/data/nvgesture/',
+ data_cfg=data_cfg_copy,
+ pipeline=[],
+ dataset_info=dataset_info,
+ test_mode=True)
+
+ custom_dataset = dataset_class(
+ ann_file='tests/data/nvgesture/test_nvgesture.lst',
+ vid_prefix='tests/data/nvgesture/',
+ data_cfg=data_cfg_copy,
+ pipeline=[],
+ dataset_info=dataset_info,
+ test_mode=False)
+
+ assert custom_dataset.dataset_name == 'nvgesture'
+ assert custom_dataset.test_mode is False
+ assert len(custom_dataset) == 1
+ sample = custom_dataset[0]
+
+ # make pseudo prediction for evaluation
+ sample['logits'] = {
+ modal: torch.zeros(1, 25, 1)
+ for modal in sample['modality']
+ }
+ sample['logits']['rgb'][:, sample['label']] = 1
+ sample['logits']['depth'][:, (sample['label'] + 1) % 25] = 1
+ sample['label'] = torch.tensor([sample['label']]).long()
+ infos = custom_dataset.evaluate([sample], metric=['AP'])
+ assert_almost_equal(infos['AP_rgb'], 1.0)
+ assert_almost_equal(infos['AP_depth'], 0.0)
+ assert_almost_equal(infos['AP_mean'], 0.5)
+
+ with pytest.raises(KeyError):
+ infos = custom_dataset.evaluate([sample], metric='mAP')
diff --git a/tests/test_models/test_gesture_forward.py b/tests/test_models/test_gesture_forward.py
new file mode 100644
index 0000000000..8c83278c0a
--- /dev/null
+++ b/tests/test_models/test_gesture_forward.py
@@ -0,0 +1,58 @@
+# Copyright (c) OpenMMLab. All rights reserved.
+
+import torch
+from addict import Dict
+
+from mmpose.models.detectors import GestureRecognizer
+
+
+def test_gesture_recognizer_forward():
+ model_cfg = dict(
+ type='GestureRecognizer',
+ pretrained=None,
+ modality=['rgb', 'depth'],
+ backbone=dict(
+ rgb=dict(
+ type='I3D',
+ in_channels=3,
+ expansion=0.25,
+ ),
+ depth=dict(
+ type='I3D',
+ in_channels=1,
+ expansion=0.25,
+ ),
+ ),
+ cls_head=dict(
+ type='MultiModalSSAHead',
+ num_classes=25,
+ avg_pool_kernel=(1, 2, 2),
+ in_channels=256),
+ train_cfg=dict(
+ beta=2,
+ lambda_=1e-3,
+ ssa_start_epoch=10,
+ ),
+ test_cfg=dict(),
+ )
+
+ detector = GestureRecognizer(model_cfg['backbone'], None,
+ model_cfg['cls_head'], model_cfg['train_cfg'],
+ model_cfg['test_cfg'], model_cfg['modality'],
+ model_cfg['pretrained'])
+ detector.set_train_epoch(11)
+
+ video = [torch.randn(1, 3, 16, 112, 112), torch.randn(1, 1, 16, 112, 112)]
+ labels = torch.tensor([1]).long()
+ img_metas = Dict()
+ img_metas.data = dict(modality=['rgb', 'depth'])
+
+ # Test forward train
+ losses = detector.forward(video, labels, img_metas, return_loss=True)
+ assert isinstance(losses, dict)
+ assert 'ssa_loss' in losses
+
+ # Test forward test
+ with torch.no_grad():
+ _ = detector.forward(
+ video, labels, img_metas=img_metas, return_loss=False)
diff --git a/tests/test_models/test_gesture_head.py b/tests/test_models/test_gesture_head.py
new file mode 100644
index 0000000000..b5a1339001
--- /dev/null
+++ b/tests/test_models/test_gesture_head.py
@@ -0,0 +1,38 @@
+# Copyright (c) OpenMMLab. All rights reserved.
+import numpy as np
+import torch
+
+from mmpose.models import MultiModalSSAHead
+
+
+def test_multi_modal_ssa_head():
+
+ # substantialize head
+ train_cfg = dict(ssa_start_epoch=10)
+ head = MultiModalSSAHead(
+ num_classes=25, modality=('rgb', 'depth'), train_cfg=train_cfg)
+
+ head.set_train_epoch(11)
+ assert head._train_epoch == 11
+ assert head._train_epoch > head.start_epoch
+
+ # forward
+ img_metas = dict(modality=['rgb', 'depth'])
+ feats = [torch.randn(2, 1024, 7, 7, 7) for _ in img_metas['modality']]
+ labels = torch.randint(25, (2, ))
+
+ logits = head(feats, img_metas)
+ assert logits[0].shape == (2, 25, 7)
+
+ losses = head.get_loss(logits, labels, feats)
+ assert 'ce_loss' in losses
+ assert 'ssa_loss' in losses
+ assert (losses['ssa_loss'] == losses['ssa_loss']).all() # check nan
+
+ logits[0][0, 1], logits[1][0, 1], labels[0] = 1e5, 1e5, 1
+ logits[0][1, 4], logits[1][1, 8], labels[1] = 1e5, 1e5, 8
+ accuracy = head.get_accuracy(logits, labels, img_metas)
+ assert 'acc_rgb' in accuracy
+ assert 'acc_depth' in accuracy
+ np.testing.assert_almost_equal(accuracy['acc_rgb'], 0.5)
+ np.testing.assert_almost_equal(accuracy['acc_depth'], 1.0)
diff --git a/tests/test_pipelines/test_gesture_pipelines.py b/tests/test_pipelines/test_gesture_pipelines.py
new file mode 100644
index 0000000000..34b7b06ece
--- /dev/null
+++ b/tests/test_pipelines/test_gesture_pipelines.py
@@ -0,0 +1,181 @@
+# Copyright (c) OpenMMLab. All rights reserved.
+import copy
+import os.path as osp
+from collections import defaultdict
+
+import json_tricks as json
+import numpy as np
+from numpy.testing import assert_array_almost_equal
+
+from mmpose.datasets.pipelines import (CenterSpatialCrop, CropValidClip,
+ GestureRandomFlip, LoadVideoFromFile,
+ ModalWiseChannelProcess,
+ MultiFrameBBoxMerge,
+ MultiModalVideoToTensor,
+ RandomAlignedSpatialCrop,
+ ResizedCropByBBox, ResizeGivenShortEdge,
+ TemporalPooling, VideoNormalizeTensor)
+
+
+def _check_flip(origin_vid, result_vid):
+ """Check if the origin_video are flipped correctly."""
+ l, h, w, c = origin_vid.shape
+
+ for t in range(l):
+ for i in range(h):
+ for j in range(w):
+ for k in range(c):
+ if result_vid[t, i, j, k] != origin_vid[t, i, w - 1 - j,
+ k]:
+ return False
+ return True
+
+
+def _check_num_frames(video_results, num_frame):
+ """Check if the video lengths match the given number of frames."""
+ if video_results['num_frames'] != num_frame:
+ return False
+ if 'bbox' in video_results and len(video_results['bbox']) != num_frame:
+ return False
+ for video in video_results['video']:
+ if video.shape[0] != num_frame:
+ return False
+ return True
+
+
+def _check_size(video_results, size):
+ """Check if the video sizes and size attributes match the given size."""
+ for h in video_results['height']:
+ if h != size[0]:
+ return False
+ for w in video_results['width']:
+ if w != size[1]:
+ return False
+ for video in video_results['video']:
+ if video.shape[1] != size[0]:
+ return False
+ if video.shape[2] != size[1]:
+ return False
+ return True
+
+
+def _check_normalize(origin_video, result_video, norm_cfg):
+ """Check if the origin_video are normalized correctly into result_video in
+ a given norm_cfg."""
+ target_video = result_video.clone()
+ for i in range(3):
+ target_video[i] *= norm_cfg['std'][i]
+ target_video[i] += norm_cfg['mean'][i]
+ assert_array_almost_equal(origin_video, target_video, decimal=4)
+
+
+def test_gesture_pipeline():
+ # test loading
+ data_prefix = 'tests/data/nvgesture'
+
+ results = defaultdict(list)
+ results['modality'] = ['rgb', 'depth']
+ results['label'] = 4
+ with open(osp.join(data_prefix, 'bboxes.json'), 'r') as f:
+ results['bbox'] = next(iter(json.load(f).values()))
+ results['ann_info'] = dict(flip_pairs=((0, 1), (4, 5), (19, 20)))
+
+ results['video_file'] = [
+ osp.join(data_prefix, 'sk_color.avi'),
+ osp.join(data_prefix, 'sk_depth.avi')
+ ]
+ transform = LoadVideoFromFile()
+ results = transform(copy.deepcopy(results))
+
+ assert results['video'][0].shape == (20, 240, 320, 3)
+ assert results['video'][1].shape == (20, 240, 320, 3)
+
+ # test CropValidClip
+ results['valid_frames'] = ((2, 19), (1, 18))
+ transform = CropValidClip()
+ results_valid = transform(copy.deepcopy(results))
+ assert _check_num_frames(results_valid, 17)
+ assert (results_valid['video'][0] == results['video'][0][2:19]).all()
+ assert (results_valid['video'][1] == results['video'][1][1:18]).all()
+
+ # test TemporalPooling
+ transform = TemporalPooling(ref_fps=15)
+ results_temp_pool = transform(copy.deepcopy(results_valid))
+ assert _check_num_frames(results_temp_pool, 9)
+
+ transform = TemporalPooling(length=10)
+ results_temp_pool = transform(copy.deepcopy(results_valid))
+ assert _check_num_frames(results_temp_pool, 10)
+ del results_temp_pool
+
+ # test ResizeGivenShortEdge
+ transform = ResizeGivenShortEdge(length=256)
+ results_resize = transform(copy.deepcopy(results_valid))
+ assert _check_size(results_resize, (256, 341))
+ del results_resize
+
+ # test MultiFrameBBoxMerge
+ transform = MultiFrameBBoxMerge()
+ results_bbox_merge = transform(copy.deepcopy(results_valid))
+ target_bbox = np.array([96.07688, 92.26083, 316.5224,
+ 231.98422]).astype(np.float32)
+ assert_array_almost_equal(results_bbox_merge['bbox'], target_bbox, 4)
+
+ # test ResizedCropByBBox
+ transform = ResizedCropByBBox(
+ size=112, scale=(0.8, 1.2), ratio=(0.8, 1.2), shift=0.3)
+ results_resize_crop = transform(copy.deepcopy(results_bbox_merge))
+ assert _check_size(results_resize_crop, (112, 112))
+ del results_bbox_merge
+
+ # test GestureRandomFlip
+ transform = GestureRandomFlip(prob=1.0)
+ results_flip = transform(copy.deepcopy(results_resize_crop))
+ assert results_flip['label'] == 5
+ assert _check_size(results_flip, (112, 112))
+ assert _check_flip(results_flip['video'][0],
+ results_resize_crop['video'][0])
+ assert _check_flip(results_flip['video'][1],
+ results_resize_crop['video'][1])
+ del results_resize_crop
+
+ # test RandomAlignedSpatialCrop & CenterSpatialCrop
+ transform = RandomAlignedSpatialCrop(length=112)
+ results_crop = transform(copy.deepcopy(results_valid))
+ assert _check_size(results_crop, (112, 112))
+
+ transform = CenterSpatialCrop(length=112)
+ results_crop = transform(copy.deepcopy(results_valid))
+ assert _check_size(results_crop, (112, 112))
+ del results_crop
+
+ # test ModalWiseChannelProcess
+ transform = ModalWiseChannelProcess()
+ results_modal_proc = transform(copy.deepcopy(results_valid))
+ for i, modal in enumerate(results_modal_proc['modality']):
+ if modal == 'rgb':
+ assert_array_almost_equal(
+ results_modal_proc['video'][i][..., ::-1],
+ results_valid['video'][i])
+ if modal == 'depth':
+ assert_array_almost_equal(results_modal_proc['video'][i],
+ results_valid['video'][i][..., :1])
+ del results_valid
+
+ # test MultiModalVideoToTensor
+ transform = MultiModalVideoToTensor()
+ results_tensor = transform(copy.deepcopy(results_modal_proc))
+ for i, video in enumerate(results_tensor['video']):
+ assert video.max() <= 1.0 and video.min() >= 0.0
+ assert video.shape[1:] == results_modal_proc['video'][i].shape[:-1]
+ assert video.shape[0] == results_modal_proc['video'][i].shape[-1]
+ del results_modal_proc
+
+ # test VideoNormalizeTensor
+ norm_cfg = {}
+ norm_cfg['mean'] = [0.485, 0.456, 0.406]
+ norm_cfg['std'] = [0.229, 0.224, 0.225]
+ transform = VideoNormalizeTensor(**norm_cfg)
+ results_norm = transform(copy.deepcopy(results_tensor))
+ _check_normalize(results_tensor['video'][0], results_norm['video'][0],
+ norm_cfg)
diff --git a/tools/webcam/configs/hand_gesture/README.md b/tools/webcam/configs/hand_gesture/README.md
new file mode 100644
index 0000000000..41ae6d33ab
--- /dev/null
+++ b/tools/webcam/configs/hand_gesture/README.md
@@ -0,0 +1,26 @@
+# Hand bounding box with gesture label effects
+
+This demo provides hand gesture recognition visualization. The label of gesture is shown on the left-up corner of hand bounding box.
+
+Example TBA.
+
+## Instruction
+
+### Get started
+
+Launch the demo from the mmpose root directory:
+
+```shell
+python tools/webcam/run_webcam.py --config tools/webcam/configs/hand_gesture/hand_bbox_with_gesture.py
+```
+
+### Hotkeys
+
+| Hotkey | Function |
+| ------ | -------------------------------- |
+| m | Show the monitoring information. |
+| q | Exit. |
+
+### Configuration
+
+See the [README](/tools/webcam/configs/examples/README.md#configuration) for model configurations.
diff --git a/tools/webcam/configs/hand_gesture/hand_bbox_with_gesture.py b/tools/webcam/configs/hand_gesture/hand_bbox_with_gesture.py
new file mode 100644
index 0000000000..b8d9485432
--- /dev/null
+++ b/tools/webcam/configs/hand_gesture/hand_bbox_with_gesture.py
@@ -0,0 +1,59 @@
+# Copyright (c) OpenMMLab. All rights reserved.
+runner = dict(
+ name='Gesture Recognition',
+ camera_id=0,
+ camera_fps=15,
+ synchronous=False,
+ buffer_sizes=dict(_input_=20, det_result=10),
+ nodes=[
+ dict(
+ type='MultiFrameDetectorNode',
+ name='Detector',
+ model_config='demo/mmdetection_cfg/'
+ 'ssdlite_mobilenetv2_scratch_600e_onehand.py',
+ model_checkpoint='https://download.openmmlab.com/mmpose/'
+ 'mmdet_pretrained/'
+ 'ssdlite_mobilenetv2_scratch_600e_onehand-4f9f8686_20220523.pth',
+ device='cpu',
+ inference_frame='last',
+ input_buffer='_input_',
+ output_buffer='det_result'),
+ dict(
+ type='HandGestureRecognizerNode',
+ name='GestureRecognizer',
+ model_config='configs/hand/gesture_sview_rgbd_vid/mtut/'
+ 'nvgesture/i3d_nvgesture_bbox_112x112_fps15_rgb.py',
+ model_checkpoint='https://download.openmmlab.com/mmpose/'
+ 'gesture/mtut/i3d_nvgesture/'
+ 'i3d_nvgesture_bbox_112x112_fps15-363b5956_20220530.pth',
+ device='cpu',
+ input_buffer='det_result',
+ output_buffer='gesture',
+ fps=15,
+ score_thr=0.7),
+ dict(
+ type='ModelResultBindingNode',
+ name='ResultBinder',
+ frame_buffer='_frame_',
+ result_buffer='gesture',
+ output_buffer='frame'),
+ dict(
+ type='GestureVisualizerNode',
+ name='Visualizer',
+ enable_key='v',
+ frame_buffer='frame',
+ output_buffer='vis'),
+ dict(
+ type='MonitorNode',
+ name='Monitor',
+ enable_key='m',
+ enable=False,
+ frame_buffer='vis',
+ output_buffer='display'),
+ dict(
+ type='RecorderNode',
+ name='Recorder',
+ out_video_file='record.mp4',
+ frame_buffer='display',
+ output_buffer='_display_')
+ ])
diff --git a/tools/webcam/webcam_apis/nodes/__init__.py b/tools/webcam/webcam_apis/nodes/__init__.py
index efdc63845e..3c8ee2db82 100644
--- a/tools/webcam/webcam_apis/nodes/__init__.py
+++ b/tools/webcam/webcam_apis/nodes/__init__.py
@@ -1,20 +1,22 @@
# Copyright (c) OpenMMLab. All rights reserved.
from .builder import NODES
from .faceswap_node import FaceSwapNode
-from .frame_effect_node import (BackgroundNode, BugEyeNode, MoustacheNode,
+from .frame_effect_node import (BackgroundNode, BugEyeNode,
+ GestureVisualizerNode, MoustacheNode,
NoticeBoardNode, PoseVisualizerNode,
SaiyanNode, SunglassesNode)
from .helper_node import ModelResultBindingNode, MonitorNode, RecorderNode
-from .mmdet_node import DetectorNode
-from .mmpose_node import TopDownPoseEstimatorNode
+from .mmdet_node import DetectorNode, MultiFrameDetectorNode
+from .mmpose_node import HandGestureRecognizerNode, TopDownPoseEstimatorNode
from .pose_tracker_node import PoseTrackerNode
from .valentinemagic_node import ValentineMagicNode
from .xdwendwen_node import XDwenDwenNode
__all__ = [
- 'NODES', 'PoseVisualizerNode', 'DetectorNode', 'TopDownPoseEstimatorNode',
- 'MonitorNode', 'BugEyeNode', 'SunglassesNode', 'ModelResultBindingNode',
- 'NoticeBoardNode', 'RecorderNode', 'FaceSwapNode', 'MoustacheNode',
- 'SaiyanNode', 'BackgroundNode', 'XDwenDwenNode', 'ValentineMagicNode',
- 'PoseTrackerNode'
+ 'NODES', 'PoseVisualizerNode', 'DetectorNode', 'MultiFrameDetectorNode',
+ 'TopDownPoseEstimatorNode', 'MonitorNode', 'BugEyeNode', 'SunglassesNode',
+ 'ModelResultBindingNode', 'NoticeBoardNode', 'RecorderNode',
+ 'FaceSwapNode', 'MoustacheNode', 'SaiyanNode', 'BackgroundNode',
+ 'XDwenDwenNode', 'ValentineMagicNode', 'GestureVisualizerNode',
+ 'HandGestureRecognizerNode', 'PoseTrackerNode'
]
diff --git a/tools/webcam/webcam_apis/nodes/frame_effect_node.py b/tools/webcam/webcam_apis/nodes/frame_effect_node.py
index c248c3820a..8cd5fe15a6 100644
--- a/tools/webcam/webcam_apis/nodes/frame_effect_node.py
+++ b/tools/webcam/webcam_apis/nodes/frame_effect_node.py
@@ -915,3 +915,89 @@ def draw(self, frame_msg):
self.num_frames * self.frame_period)
return canvas
+
+
+@NODES.register_module()
+class GestureVisualizerNode(FrameDrawingNode):
+ """Draw the bbox and gesture recognition results.
+
+ Args:
+ name (str, optional): The node name (also thread name).
+ frame_buffer (str): The name of the input buffer.
+ output_buffer (str|list): The name(s) of the output buffer(s).
+ enable_key (str|int, optional): Set a hot-key to toggle enable/disable
+ of the node. If an int value is given, it will be treated as an
+ ascii code of a key. Please note:
+ 1. If enable_key is set, the bypass method need to be
+ overridden to define the node behavior when disabled
+ 2. Some hot-key has been use for particular use. For example:
+ 'q', 'Q' and 27 are used for quit
+ Default: None
+ enable (bool): Default enable/disable status. Default: True.
+ bbox_color (str|tuple|dict): If a single color (a str like 'green' or
+ a tuple like (0, 255, 0)), it will used to draw the bbox.
+ Optionally, a dict can be given as a map from class labels to
+ colors.
+ """
+
+ default_bbox_color = {
+ 'hand': (148, 139, 255),
+ }
+
+ def __init__(self,
+ name: str,
+ frame_buffer: str,
+ output_buffer: Union[str, List[str]],
+ enable_key: Optional[Union[str, int]] = None,
+ enable: bool = True,
+ bbox_color: Optional[Union[str, Tuple, Dict]] = None):
+
+ super().__init__(name, frame_buffer, output_buffer, enable_key, enable)
+
+ if bbox_color is None:
+ self.bbox_color = self.default_bbox_color
+ elif isinstance(bbox_color, dict):
+ self.bbox_color = {k: color_val(v) for k, v in bbox_color.items()}
+ else:
+ self.bbox_color = color_val(bbox_color)
+
+ def draw(self, frame_msg):
+ canvas = frame_msg.get_image()
+ pose_results = frame_msg.get_pose_results()
+
+ if not pose_results:
+ return canvas
+
+ for pose_result in frame_msg.get_pose_results():
+
+ # Extract bboxes and poses
+ bbox_preds = []
+ bbox_labels = []
+ for pred in pose_result['preds']:
+ if 'bbox' in pred:
+ bbox_preds.append(pred['bbox'])
+ bbox_labels.append(pred.get('label', None))
+
+ # Get bbox colors
+ if isinstance(self.bbox_color, dict):
+ bbox_colors = [
+ self.bbox_color.get(label, (0, 255, 0))
+ for label in bbox_labels
+ ]
+ else:
+ bbox_labels = self.bbox_color
+
+ # Draw bboxes
+ if bbox_preds:
+ bboxes = np.vstack(bbox_preds)
+
+ imshow_bboxes(
+ canvas,
+ bboxes,
+ labels=bbox_labels,
+ colors=bbox_colors,
+ text_color='white',
+ font_scale=0.5,
+ show=False)
+
+ return canvas
diff --git a/tools/webcam/webcam_apis/nodes/mmdet_node.py b/tools/webcam/webcam_apis/nodes/mmdet_node.py
index aaeeb15872..4e1b7e1efb 100644
--- a/tools/webcam/webcam_apis/nodes/mmdet_node.py
+++ b/tools/webcam/webcam_apis/nodes/mmdet_node.py
@@ -1,8 +1,10 @@
# Copyright (c) OpenMMLab. All rights reserved.
from typing import List, Optional, Union
+import numpy as np
+
from .builder import NODES
-from .node import Node
+from .node import MultiInputNode, Node
try:
from mmdet.apis import inference_detector, init_detector
@@ -32,7 +34,6 @@ def __init__(self,
self.model_config = model_config
self.model_checkpoint = model_checkpoint
self.device = device.lower()
-
# Init model
self.model = init_detector(
self.model_config, self.model_checkpoint, device=self.device)
@@ -87,3 +88,58 @@ def _post_process(self, preds):
result['preds'].extend(preds_i)
return result
+
+
+@NODES.register_module()
+class MultiFrameDetectorNode(DetectorNode, MultiInputNode):
+ """Detect hand with one frame in a video clip. The length of clip is
+ decided on the frame rate and the inference speed of detector.
+
+ Parameters:
+ inference_frame (str): indicate the frame selected in a clip to run
+ detect hand. Can be set to ('begin', 'mid', 'last').
+ Default: 'mid'.
+ """
+
+ def __init__(self,
+ name: str,
+ model_config: str,
+ model_checkpoint: str,
+ input_buffer: str,
+ output_buffer: Union[str, List[str]],
+ inference_frame: str = 'mid',
+ enable_key: Optional[Union[str, int]] = None,
+ device: str = 'cuda:0'):
+ DetectorNode.__init__(
+ self,
+ name,
+ model_config,
+ model_checkpoint,
+ input_buffer,
+ output_buffer,
+ enable_key,
+ device=device)
+ self.inference_frame = inference_frame
+
+ def process(self, input_msgs):
+ """Select frame and detect hand."""
+ input_msg = input_msgs['input']
+ if self.inference_frame == 'last':
+ key_frame = input_msg[-1]
+ elif self.inference_frame == 'mid':
+ key_frame = input_msg[len(input_msg) // 2]
+ elif self.inference_frame == 'begin':
+ key_frame = input_msg[0]
+ else:
+ raise ValueError(f'Invalid inference_frame {self.inference_frame}')
+
+ img = key_frame.get_image()
+
+ preds = inference_detector(self.model, img)
+ det_result = self._post_process(preds)
+
+ imgs = [frame.get_image() for frame in input_msg]
+ key_frame.set_image(np.stack(imgs, axis=0))
+
+ key_frame.add_detection_result(det_result, tag=self.name)
+ return key_frame
diff --git a/tools/webcam/webcam_apis/nodes/mmpose_node.py b/tools/webcam/webcam_apis/nodes/mmpose_node.py
index 41000e0744..988bec41b2 100644
--- a/tools/webcam/webcam_apis/nodes/mmpose_node.py
+++ b/tools/webcam/webcam_apis/nodes/mmpose_node.py
@@ -1,12 +1,15 @@
# Copyright (c) OpenMMLab. All rights reserved.
from dataclasses import dataclass
-from typing import List, Optional, Union
+from typing import Dict, List, Optional, Union
-from mmpose.apis import (get_track_id, inference_top_down_pose_model,
- init_pose_model)
+import numpy as np
+
+from mmpose.apis import (get_track_id, inference_gesture_model,
+ inference_top_down_pose_model, init_pose_model)
from mmpose.core import Smoother
+from ..utils import Message
from .builder import NODES
-from .node import Node
+from .node import MultiInputNode, Node
@dataclass
@@ -120,3 +123,170 @@ def process(self, input_msgs):
input_msg.add_pose_result(pose_result, tag=self.name)
return input_msg
+
+
+@NODES.register_module()
+class HandGestureRecognizerNode(TopDownPoseEstimatorNode, MultiInputNode):
+
+ def __init__(self,
+ name: str,
+ model_config: str,
+ model_checkpoint: str,
+ input_buffer: str,
+ output_buffer: Union[str, List[str]],
+ enable_key: Optional[Union[str, int]] = None,
+ enable: bool = True,
+ device: str = 'cuda:0',
+ cls_ids: Optional[List] = None,
+ cls_names: Optional[List] = None,
+ bbox_thr: float = 0.5,
+ min_frame: int = 16,
+ fps: int = 30,
+ score_thr: float = 0.7):
+ TopDownPoseEstimatorNode.__init__(
+ self,
+ name=name,
+ model_config=model_config,
+ model_checkpoint=model_checkpoint,
+ input_buffer=input_buffer,
+ output_buffer=output_buffer,
+ enable_key=enable_key,
+ enable=enable,
+ device=device,
+ cls_ids=cls_ids,
+ cls_names=cls_names,
+ bbox_thr=bbox_thr)
+
+ # item of _clip_buffer: (clip message, num of frames)
+ self._clip_buffer = []
+ self.score_thr = score_thr
+ self.min_frame = min_frame
+ self.fps = fps
+
+ @property
+ def totol_clip_length(self):
+ return sum([clip[1] for clip in self._clip_buffer])
+
+ def _add_clips(self, clips: List[Message]):
+ """Push the newly loaded clips from buffer, and discard old clips."""
+ for clip in clips:
+ clip_length = clip.get_image().shape[0]
+ self._clip_buffer.append((clip, clip_length))
+ total_length = 0
+ for i in range(-2, -len(self._clip_buffer) - 1, -1):
+ total_length += self._clip_buffer[i][1]
+ if total_length >= self.min_frame:
+ self._clip_buffer = self._clip_buffer[i:]
+ break
+
+ def _merge_clips(self):
+ """Concat the clips into a longer video, and gather bboxes."""
+ videos = [clip[0].get_image() for clip in self._clip_buffer]
+ video = np.concatenate(videos)
+ det_results_lst = [
+ clip[0].get_detection_results() for clip in self._clip_buffer
+ ]
+ bboxes = [
+ self._process_clip_bbox(det_results)
+ for det_results in det_results_lst
+ ]
+ bboxes = list(filter(len, bboxes))
+ return video, bboxes
+
+ def _process_clip_bbox(self, det_results: List[Dict]):
+ """Filter and merge the bboxes of a video."""
+ full_det_preds = []
+ for det_result in det_results:
+ det_preds = det_result['preds']
+ if self.cls_ids:
+ # Filter detection results by class ID
+ det_preds = [
+ p for p in det_preds if p['cls_id'] in self.cls_ids
+ ]
+ elif self.cls_names:
+ # Filter detection results by class name
+ det_preds = [
+ p for p in det_preds if p['label'] in self.cls_names
+ ]
+ if self.bbox_thr > 0:
+ det_preds = [
+ p for p in det_preds if p['bbox'][-1] > self.bbox_thr
+ ]
+ full_det_preds.extend(det_preds)
+
+ merged_bbox = self._merge_bbox(full_det_preds)
+ return merged_bbox
+
+ def _merge_bbox(self, bboxes: List[Dict], ratio=0.5):
+ """Merge bboxes in a video to create a new bbox that covers the region
+ where hand moves in the video."""
+
+ if len(bboxes) <= 1:
+ return bboxes
+
+ def compute_area(bbox):
+ area = abs(bbox['bbox'][2] -
+ bbox['bbox'][0]) * abs(bbox['bbox'][3] -
+ bbox['bbox'][1])
+ return area
+
+ bboxes.sort(key=lambda b: compute_area(b), reverse=True)
+ merged = False
+ for i in range(1, len(bboxes)):
+ small_area = compute_area(bboxes[i])
+ x1 = max(bboxes[0]['bbox'][0], bboxes[i]['bbox'][0])
+ y1 = max(bboxes[0]['bbox'][1], bboxes[i]['bbox'][1])
+ x2 = min(bboxes[0]['bbox'][2], bboxes[i]['bbox'][2])
+ y2 = min(bboxes[0]['bbox'][3], bboxes[i]['bbox'][3])
+ area_ratio = (abs(x2 - x1) * abs(y2 - y1)) / small_area
+ if area_ratio > ratio:
+ bboxes[0]['bbox'][0] = min(bboxes[0]['bbox'][0],
+ bboxes[i]['bbox'][0])
+ bboxes[0]['bbox'][1] = min(bboxes[0]['bbox'][1],
+ bboxes[i]['bbox'][1])
+ bboxes[0]['bbox'][2] = max(bboxes[0]['bbox'][2],
+ bboxes[i]['bbox'][2])
+ bboxes[0]['bbox'][3] = max(bboxes[0]['bbox'][3],
+ bboxes[i]['bbox'][3])
+ merged = True
+ break
+
+ if merged:
+ bboxes.pop(i)
+ return self._merge_bbox(bboxes, ratio)
+ else:
+ return [bboxes[0]]
+
+ def process(self, input_msgs: Dict[str, Message]) -> Message:
+ """Load and process the clips with hand detection result, and recognize
+ the gesture."""
+
+ input_msg = input_msgs['input']
+ self._add_clips(input_msg)
+ video, bboxes = self._merge_clips()
+ msg = input_msg[-1]
+
+ gesture_result = {
+ 'preds': [],
+ 'model_cfg': self.model.cfg.copy(),
+ }
+ if self.totol_clip_length >= self.min_frame and len(
+ bboxes) > 0 and max(map(len, bboxes)) > 0:
+ # Inference gesture
+ pred_label, pred_score = inference_gesture_model(
+ self.model,
+ video,
+ bboxes=bboxes,
+ dataset_info=dict(
+ name='camera', fps=self.fps, modality=['rgb']))
+ for i in range(len(pred_label)):
+ result = bboxes[-1][0].copy()
+ if pred_score[i] > self.score_thr:
+ label = pred_label[i].item()
+ label = self.model.cfg.dataset_info.category_info[label]
+ result['label'] = label
+ gesture_result['preds'].append(result)
+
+ msg.add_pose_result(gesture_result, tag=self.name)
+
+ return msg
diff --git a/tools/webcam/webcam_apis/nodes/node.py b/tools/webcam/webcam_apis/nodes/node.py
index 178d743316..fefab741be 100644
--- a/tools/webcam/webcam_apis/nodes/node.py
+++ b/tools/webcam/webcam_apis/nodes/node.py
@@ -370,3 +370,51 @@ def event_listener():
self._send_output_to_buffers(output_msg)
logging.info(f'{self.name}: process ending.')
+
+
+class MultiInputNode(Node):
+ """Base interface of functional module which accept multiple inputs."""
+
+ def _get_input_from_buffer(self) -> Tuple[bool, Optional[Dict]]:
+ """Get and pack input data if it's ready. The function returns a tuple
+ of a status flag and a packed data dictionary. If input_buffer is
+ ready, the status flag will be True, and the packed data is a dict
+ whose items are buffer names and corresponding messages (unready
+ additional buffers will give a `None`). Otherwise, the status flag is
+ False and the packed data is None. Notice that.
+
+ Returns:
+ bool: status flag
+ dict[str, list[Message]]: the packed inputs where the key
+ is the buffer name and the value is the Message got from the
+ corresponding buffer.
+ """
+ buffer_manager = self._buffer_manager
+
+ if buffer_manager is None:
+ raise ValueError(f'{self.name}: Runner not set!')
+
+ # Check that essential buffers are ready
+ for buffer_info in self._input_buffers:
+ if buffer_info.trigger and buffer_manager.is_empty(
+ buffer_info.buffer_name):
+ return False, None
+
+ # Default input
+ result = {
+ buffer_info.input_name: None
+ for buffer_info in self._input_buffers
+ }
+
+ for buffer_info in self._input_buffers:
+
+ while not buffer_manager.is_empty(buffer_info.buffer_name):
+ if result[buffer_info.input_name] is None:
+ result[buffer_info.input_name] = []
+ result[buffer_info.input_name].append(
+ buffer_manager.get(buffer_info.buffer_name, block=False))
+
+ if buffer_info.trigger and result[buffer_info.input_name] is None:
+ return False, None
+
+ return True, result