-
Notifications
You must be signed in to change notification settings - Fork 85
/
其他.md
212 lines (155 loc) · 6.27 KB
/
其他.md
1
2
3
4
5
6
7
8
9
10
11
12
13
14
15
16
17
18
19
20
21
22
23
24
25
26
27
28
29
30
31
32
33
34
35
36
37
38
39
40
41
42
43
44
45
46
47
48
49
50
51
52
53
54
55
56
57
58
59
60
61
62
63
64
65
66
67
68
69
70
71
72
73
74
75
76
77
78
79
80
81
82
83
84
85
86
87
88
89
90
91
92
93
94
95
96
97
98
99
100
101
102
103
104
105
106
107
108
109
110
111
112
113
114
115
116
117
118
119
120
121
122
123
124
125
126
127
128
129
130
131
132
133
134
135
136
137
138
139
140
141
142
143
144
145
146
147
148
149
150
151
152
153
154
155
156
157
158
159
160
161
162
163
164
165
166
167
168
169
170
171
172
173
174
175
176
177
178
179
180
181
182
183
184
185
186
187
188
189
190
191
192
193
194
195
196
197
198
199
200
201
202
203
204
205
206
207
208
209
210
211
212
# 其他
----
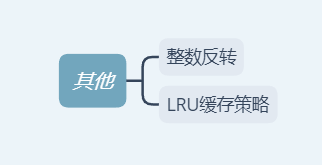
<br>
<br>
## 两个大文件中找出共同记录
#### 解题思路
- step1:遍历文件a,对每个url求取hash(url)%1000,然后根据所取得的值将url分别存储到1000个小文件(记为a0,a1,...,a999,每个小文件约300M),为什么是1000?主要根据内存大小和要分治的文件大小来计算,我们就大致可以把320G大小分为1000份,每份大约300M(当然,到底能不能分布尽量均匀,得看hash函数的设计)
- step2:遍历文件b,采取和a相同的方式将url分别存储到1000个小文件(记为b0,b1,...,b999)(为什么要这样做? 文件a的hash映射和文件b的hash映射函数要保持一致,这样的话相同的url就会保存在对应的小文件中,比如,如果a中有一个url记录data1被hash到了a99文件中,那么如果b中也有相同url,则一定被hash到了b99中)所以现在问题转换成了:找出1000对小文件中每一对相同的url(不对应的小文件不可能有相同的url)
- step3:因为每个hash大约300M,所以我们再可以采用(1)中的想法
## ip地址与int类型的转换
#### 解题思路
- ip地址转int类型,例如ip为“192.168.1.116”,相当于“.“将ip地址分为了4部分,各部分对应的权值为256^3, 256^2, 256, 1,相成即可
- int类型转ip地址,思路类似,除以权值即可,但是有部分字符串的操作
## 整数反转
----
将一个整数中的数字进行`颠倒`,当颠倒后的整数`溢出时`,返回 0 (标记为 32 位整数)。
示例 :
```css
输入: -123
输出: -321
```
#### 解题思路
利用`除 10 取余`的方法,将最低位和最高`倒序输出`即可
```java
public int reverseInteger(int n) {
int reversed_n = 0;
while (n != 0) {
int temp = reversed_n * 10 + n % 10;
n = n / 10;
if (temp / 10 != reversed_n) {
reversed_n = 0;
break;
}
reversed_n = temp;
}
return reversed_n;
}
```
<br>
<br>
## LRU缓存策略
----
运用你所掌握的数据结构,设计和实现一个 LRU (最近最少使用) 缓存机制。它应该支持以下操作: 获取数据 get 和 写入数据 put 。
获取数据 `get(key)` - 如果密钥 `(key) 存在`于缓存中,则获取密钥的值(总是正数),否则返回 -1。
写入数据 `put(key, value)` - 如果密钥`不存在`,则`写入`其数据值。当缓存容量达到上限时,它应该在写入新数据之前`删除`最近最少使用的数据值,从而为新的数据值留出空间。
示例:
```css
LRUCache cache = new LRUCache( 2 /* 缓存容量 */ );
cache.put(1, 1);
cache.put(2, 2);
cache.get(1); // 返回 1
cache.put(3, 3); // 该操作会使得密钥 2 作废
cache.get(2); // 返回 -1 (未找到)
cache.put(4, 4); // 该操作会使得密钥 1 作废
cache.get(1); // 返回 -1 (未找到)
cache.get(3); // 返回 3
cache.get(4); // 返回 4
```
#### 解题思路
**解法一:**
自定义数据结构:
- 实现一个链表用于记录缓存,并处理调用使用频率
- 定义一个 `HashMap` 用于记录缓存内容
```java
public class LRUCache {
private class Node{
Node prev;
Node next;
int key;
int value;
public Node(int key, int value) {
this.key = key;
this.value = value;
this.prev = null;
this.next = null;
}
}
private int capacity;
private HashMap<Integer, Node> hs = new HashMap<Integer, Node>();
private Node head = new Node(-1, -1);// 头
private Node tail = new Node(-1, -1);// 尾
public LRUCache(int capacity) {
this.capacity = capacity;
tail.prev = head;
head.next = tail;
}
public int get(int key) {
if( !hs.containsKey(key)) { //key找不到
return -1;
}
// remove current
Node current = hs.get(key);
current.prev.next = current.next;
current.next.prev = current.prev;
// move current to tail
move_to_tail(current); //每次get,使用次数+1,最近使用,放于尾部
return hs.get(key).value;
}
public void set(int key, int value) { //数据放入缓存
// get 这个方法会把key挪到最末端,因此,不需要再调用 move_to_tail
if (get(key) != -1) {
hs.get(key).value = value;
return;
}
if (hs.size() == capacity) { //超出缓存上限
hs.remove(head.next.key); //删除头部数据
head.next = head.next.next;
head.next.prev = head;
}
Node insert = new Node(key, value); //新建节点
hs.put(key, insert);
move_to_tail(insert); //放于尾部
}
private void move_to_tail(Node current) { //移动数据至尾部
current.prev = tail.prev;
tail.prev = current;
current.prev.next = current;
current.next = tail;
}
}
```
**解法二:**
题目要求实现 `LRU` 缓存机制,需要在 `O(1)`时间内完成如下操作:
- 获取键 / 检查键是否存在
- 设置键
- 删除最先插入的键
- 前两个操作可以用标准的哈希表在 `O(1)` 时间内完成。
有一种叫做`有序字典`的数据结构,综合了`哈希表`和`链表`,在 Java 中为 `LinkedHashMap`。
下面用这个数据结构来实现。
```java
class LRUCache extends LinkedHashMap<Integer, Integer>{
private int capacity;
public LRUCache(int capacity) {
super(capacity, 0.75F, true);
this.capacity = capacity;
}
public int get(int key) {
return super.getOrDefault(key, -1);
}
public void put(int key, int value) {
super.put(key, value);
}
@Override
protected boolean removeEldestEntry(Map.Entry<Integer, Integer> eldest) {
return size() > capacity;
}
}
```
#### 复杂度分析
- 时间复杂度:对于 put 和 get 操作复杂度是 $O(1)$,因为有序字典中的所有操作:
- `get/in/set/move_to_end/popitem(get/containsKey/put/remove)`都可以在常数时间内完成。
空间复杂度:$O(capacity)$,因为空间只用于有序字典存储最多 capacity + 1 个元素。
<br>
<br>