-
Notifications
You must be signed in to change notification settings - Fork 904
New issue
Have a question about this project? Sign up for a free GitHub account to open an issue and contact its maintainers and the community.
By clicking “Sign up for GitHub”, you agree to our terms of service and privacy statement. We’ll occasionally send you account related emails.
Already on GitHub? Sign in to your account
Different mels/wavs for same speech and model. Is it normal? #95
Comments
@jgarciadominguez , You can try to set dropout=0.0 when you synthesize speech. |
I believe I already answered this in the TODO issue but what the heck let's answer again :) Your batch size is too small, never work with tacotron_batch_size smaller than 32. To avoid OOM when doing so, you can use "outputs_per_step=3" and limit your mel samples using the "max_mel_frames" parameter. Finally, generated samples are always slightly different because we just dropout during synthesis inside the decoder prenet. This can be found here: Tacotron-2/tacotron/models/modules.py Line 252 in c205b45
|
We have trained 200.000 steps with a small corpus. Not good result, but the weird thing is that everytime we syntetize the result is slightly different.
python3 synthesize.py --model='Tacotron' --mode='eval' --hparams='symmetric_mels=False,max_abs_value=4.0,power=1.1,outputs_per_step=1' --text_to_speak='this is a test'
and hparams
Hyperparameters: allow_clipping_in_normalization: True attention_dim: 128 attention_filters: 32 attention_kernel: (31,) cleaners: english_cleaners cumulative_weights: True decoder_layers: 2 decoder_lstm_units: 1024 embedding_dim: 512 enc_conv_channels: 512 enc_conv_kernel_size: (5,) enc_conv_num_layers: 3 encoder_lstm_units: 256 fft_size: 1024 fmax: 7600 fmin: 125 frame_shift_ms: None griffin_lim_iters: 60 hop_size: 256 impute_finished: False input_type: raw log_scale_min: -32.23619130191664 mask_encoder: False mask_finished: False max_abs_value: 4.0 max_iters: 2500 min_level_db: -100 num_freq: 513 num_mels: 80 outputs_per_step: 1 postnet_channels: 512 postnet_kernel_size: (5,) postnet_num_layers: 5 power: 1.1 predict_linear: False prenet_layers: [256, 256] quantize_channels: 65536 ref_level_db: 20 rescale: True rescaling_max: 0.999 sample_rate: 22050 signal_normalization: True silence_threshold: 2 smoothing: False stop_at_any: True symmetric_mels: False tacotron_adam_beta1: 0.9 tacotron_adam_beta2: 0.999 tacotron_adam_epsilon: 1e-06 tacotron_batch_size: 2 tacotron_decay_learning_rate: True tacotron_decay_rate: 0.4 tacotron_decay_steps: 50000 tacotron_dropout_rate: 0.5 tacotron_final_learning_rate: 1e-05 tacotron_initial_learning_rate: 0.001 tacotron_reg_weight: 1e-06 tacotron_scale_regularization: True tacotron_start_decay: 50000 tacotron_teacher_forcing_ratio: 1.0 tacotron_zoneout_rate: 0.1 trim_silence: True use_lws: True Constructing model: Tacotron
Any ideas why this could be happening?
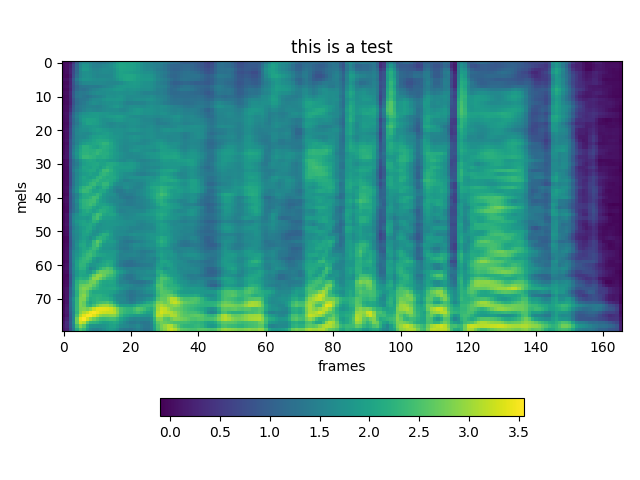
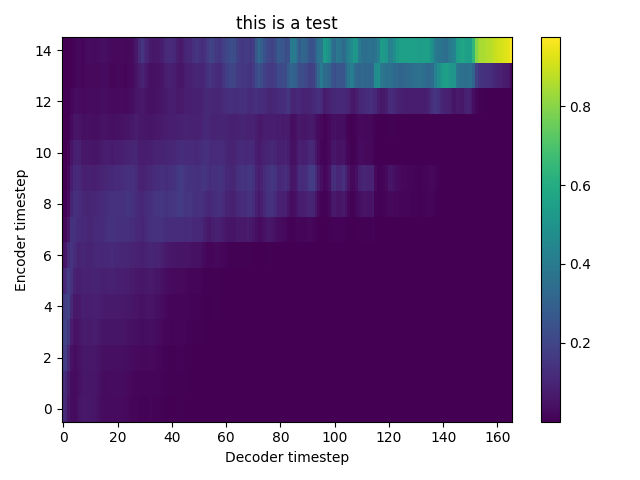
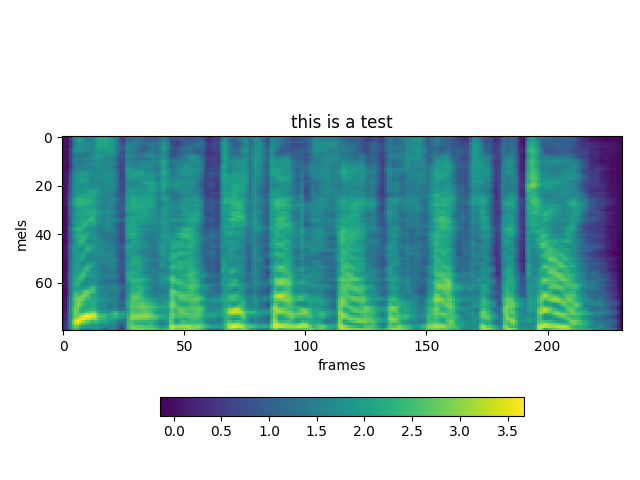
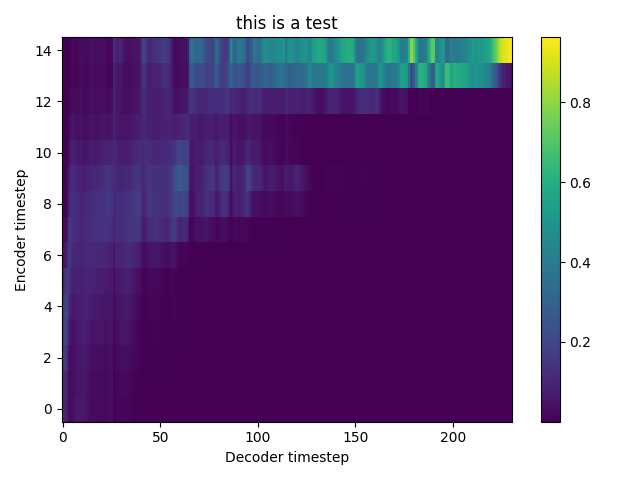
TEST 1:
TEST 2:
The text was updated successfully, but these errors were encountered: