You signed in with another tab or window. Reload to refresh your session.You signed out in another tab or window. Reload to refresh your session.You switched accounts on another tab or window. Reload to refresh your session.Dismiss alert
Transformer에 적합한 Semantic Segmentation 모델을 제안하자는게 본 논문의 내용입니다.
Segmentation 모델은 그동안 Encoder-Decoder 형태가 대다수였는데, Encoder에서는 Feature의 Representation을 학습하고, Decoder에서는 픽셀 수준의 Regressor였습니다.
Decoder에서는 더 넓은 Receptive를 보고자 filter의 크기를 키우거나, Atrous Convolution, Featrue Pyramid를 적용하는 등 다양한 기법들이 제안되어 왔는데, 이는 Global Context를 추출하는 데에 한계가 있었기 때문입니다.
최근에는 attention만을 사용해서, convolution을 제외한 채로 Segmentation 네트워크들을 구성하려는 많은 시도들이 있었습니다.
하지만 그럼에도 불구하고, 이들의 구조는 Encoder-Decoder의 형태를 완전히 벗어나지는 못했습니다.
그러므로, 본 논문에서는 Stacked Convolution Layer의 Segmentation 구조를 탈피하고, Pure Transformer 만을 사용해서 Segmentation에 좋은 성능을 낼 수 있는 모델을 제안합니다.
또한, 본 논문에서는 Global Context를 사용하는 Attention 계열의 모델에서는 Encoder-Decoder 형태로 해상도를 줄였다가 늘릴 필요가 없다는 것을 검증했습니다.
모델의 구조는 다음과 같습니다.
이미지를 고정된 크기의 patch들로 자르고, positional encoding을 한 후에, embedding해서 transformer에서 학습합니다.
transformer를 통해서 출력된 값은, Segmentation 모델을 통해서 원본 크기로 복원이 됩니다.
제안된 모델은 3가지 입니다.
1.Naive upsampling (Naive)
Pointwise Convolution으로 pixelwise classification을 하고, Upsampling으로 해상도를 맞춥니다.
2.Progressive UPsampling (PUP)
Convolution을 사용하면서, 점짐적으로 Upsampling을 합니다.
간단하게 보면, Upsampling + Convolution입니다.
3.Multi-Level feature Aggregation (MLA)
Auxiliary Loss와 유사한데, Transformer의 각 단계별로 출력값을 Concat해서 Upsampling과 Convolution으로 원본 해상도로 키운 것입니다.
성능은 다음과 같습니다.
2번과 3번 모델이 서로 좋은 성능을 보여주고 있습니다.
2번은 사실 왜 좋게 나오는지는 잘 모르겠습니다.
구조가 단순한데 생각보다 잘 나옵니다.
3번은 auxiliary loss와 유사하기 떄문에 구현이 꽤 복잡한데 비해서 성능은 2번과 크게 차이가 나지 않는 것 같습니다.
구현의 편의성을 생각하면 2번을 채택하면 될 거 같습니다.
다만, 아직 해당 방법론이 큰 이미지 데이터에 대해서는 어떻게 구성해야 하는지 잘 연구가 되어 있지 않습니다.
또한, 여기 있는 모든 모델들은 auxiliary loss를 사용해주는게 성능 향상에 도움이 된다고 하는데, 레퍼런스 논문을 참조한 결과 1.2~1.4의 AP를 올려주는 것으로 보입니다.
The text was updated successfully, but these errors were encountered:
Transformer에 적합한 Semantic Segmentation 모델을 제안하자는게 본 논문의 내용입니다.
Segmentation 모델은 그동안 Encoder-Decoder 형태가 대다수였는데, Encoder에서는 Feature의 Representation을 학습하고, Decoder에서는 픽셀 수준의 Regressor였습니다.
Decoder에서는 더 넓은 Receptive를 보고자 filter의 크기를 키우거나, Atrous Convolution, Featrue Pyramid를 적용하는 등 다양한 기법들이 제안되어 왔는데, 이는 Global Context를 추출하는 데에 한계가 있었기 때문입니다.
최근에는 attention만을 사용해서, convolution을 제외한 채로 Segmentation 네트워크들을 구성하려는 많은 시도들이 있었습니다.
하지만 그럼에도 불구하고, 이들의 구조는 Encoder-Decoder의 형태를 완전히 벗어나지는 못했습니다.
그러므로, 본 논문에서는 Stacked Convolution Layer의 Segmentation 구조를 탈피하고, Pure Transformer 만을 사용해서 Segmentation에 좋은 성능을 낼 수 있는 모델을 제안합니다.
또한, 본 논문에서는 Global Context를 사용하는 Attention 계열의 모델에서는 Encoder-Decoder 형태로 해상도를 줄였다가 늘릴 필요가 없다는 것을 검증했습니다.
모델의 구조는 다음과 같습니다.
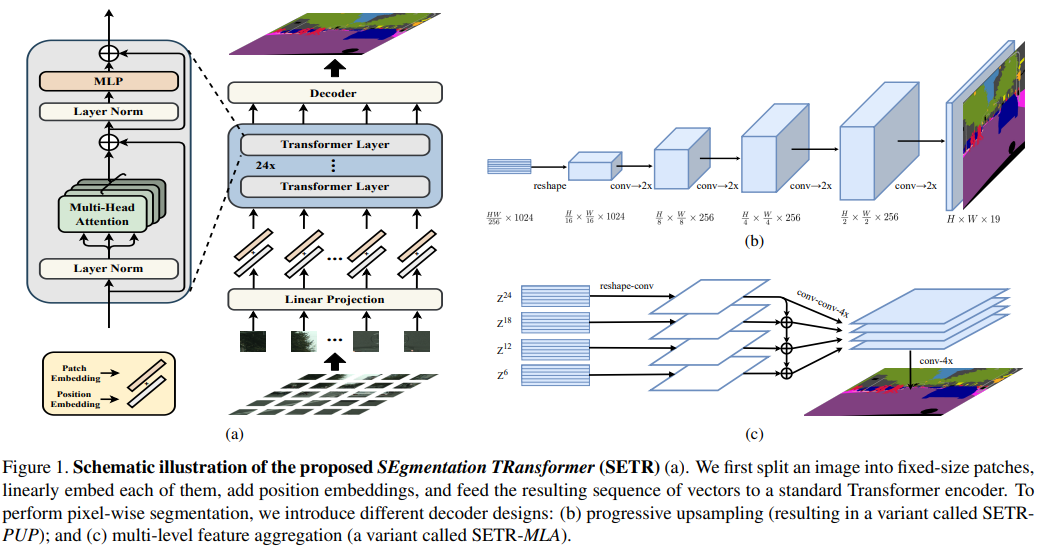
이미지를 고정된 크기의 patch들로 자르고, positional encoding을 한 후에, embedding해서 transformer에서 학습합니다.
transformer를 통해서 출력된 값은, Segmentation 모델을 통해서 원본 크기로 복원이 됩니다.
제안된 모델은 3가지 입니다.
1.Naive upsampling (Naive)
Pointwise Convolution으로 pixelwise classification을 하고, Upsampling으로 해상도를 맞춥니다.
2.Progressive UPsampling (PUP)
Convolution을 사용하면서, 점짐적으로 Upsampling을 합니다.
간단하게 보면, Upsampling + Convolution입니다.
3.Multi-Level feature Aggregation (MLA)
Auxiliary Loss와 유사한데, Transformer의 각 단계별로 출력값을 Concat해서 Upsampling과 Convolution으로 원본 해상도로 키운 것입니다.
성능은 다음과 같습니다.
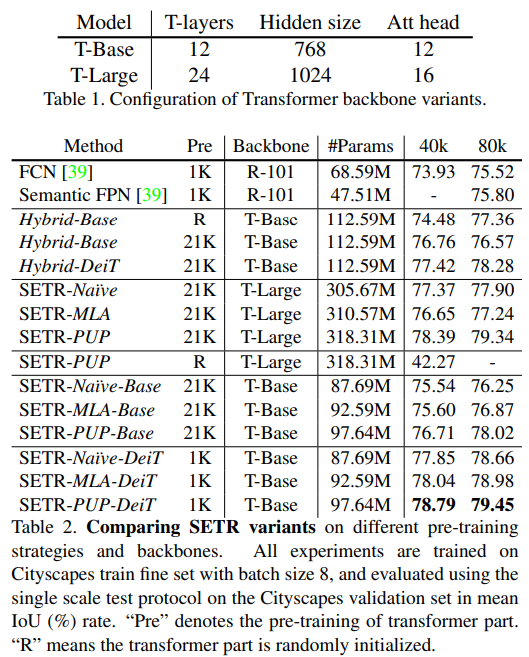
2번과 3번 모델이 서로 좋은 성능을 보여주고 있습니다.
2번은 사실 왜 좋게 나오는지는 잘 모르겠습니다.
구조가 단순한데 생각보다 잘 나옵니다.
3번은 auxiliary loss와 유사하기 떄문에 구현이 꽤 복잡한데 비해서 성능은 2번과 크게 차이가 나지 않는 것 같습니다.
구현의 편의성을 생각하면 2번을 채택하면 될 거 같습니다.
다만, 아직 해당 방법론이 큰 이미지 데이터에 대해서는 어떻게 구성해야 하는지 잘 연구가 되어 있지 않습니다.
또한, 여기 있는 모든 모델들은 auxiliary loss를 사용해주는게 성능 향상에 도움이 된다고 하는데, 레퍼런스 논문을 참조한 결과 1.2~1.4의 AP를 올려주는 것으로 보입니다.
The text was updated successfully, but these errors were encountered: