-
Notifications
You must be signed in to change notification settings - Fork 1.3k
New issue
Have a question about this project? Sign up for a free GitHub account to open an issue and contact its maintainers and the community.
By clicking “Sign up for GitHub”, you agree to our terms of service and privacy statement. We’ll occasionally send you account related emails.
Already on GitHub? Sign in to your account
Tacotron2 + WaveRNN experiments #26
Comments
Here is the first WaveRNN based result. |
Here is a pocket article read by the mode WaveRNN + Tacotron2 |
@erogol Awesome results. Did you train tacotron2 with batch size of 32 and r=1? Was it done on multi-gpu or just one? |
@erogol Fatcord's wavernn works very well with 10 bit audio as well, which helps to eliminate quite a bit of the background static. |
@ZohaibAhmed I trained it with 4 GPUs with 16 batch size per GPU which is the max I can fit into a 1080ti. Much better results are coming through with couple of architectural changes. @G-Wang thx for pointing. I am going to try. I just trained WaveRNN once and now I plan to discover some model updates and quantization schemes. I ll let you know here |
I'm currently pretty happy with https://github.com/h-meru/Tacotron-WaveRNN/blob/master/README.md I struggled some time when I integrated a similar model (something between that and the amazon universal vocoder architecture) but in the end I found the reason was just the Noam LR scheme performed much worse than just the fixed LR with Adam which in the worst case I could just reduce a little bit manually when the loss starts to behave funny. |
Training WaveRNN with 10bit quantization. Also the final solution in #50 improved the model performance significantly. Results soon to be shared... |
@erogol I've tried a few variants such as mu-law, gaussian/beta output, mixture logistics, but I found 9 and 10-bit at the end of the day gave the best result and fastest training, you can hear some samples here: https://github.com/G-Wang/WaveRNN-Pytorch |
@erogol There is also a highly optimized pytorch and C++ implementation which I am training on the output of TTS extract_features script: https://github.com/geneing/WaveRNN-Pytorch |
@erogol thanks erogol. When could we use the tacotron2 + WaveRNN model to train on our datasets? |
Is any pretrained wavernn model available for test? |
This is the latest result. Problems:
Below is the comparison of BN vs Dropout prenets as explain in #50 Audio Sample: Audio Sample with the best GL based model: |
Interesting results! |
@m-toman I don't see what you mean by the second option I trained it with the specs from tacotron with teacher forcing. |
Thanks, with the second option I mean just calculating Mel specs from the recordings and then training on those, without using Tacotron at all. In the Amazon universal vocoder paper they did that (I assume) because they train from lots of data from many different speakers and recording conditions, so it's a trickier to use GTA mel specs (although you could do it with a very large multispeaker model I suppose) |
@m-toman I was not aware that they show better results by using ground-truth mels specs. However, it does not really make sense to me (without any experimentation) since I believe vocoder is able to learn to obviate the mistakes done by the first network as it is trained with synthesized specs. But I guess to be sure, I need to try first. |
No, they were worse (lower MOS 1-5 with 5 as the best score) as expected.
So that's fine. |
Can you give me any hints on porting the Tacotron model to OpenCV? |
sorry, no idea. |
Hi again, Is there a reason to pad externally with ConstantPad1d and not use the Conv1d padding in Tacotron/CBHG/BatchNorm1d? If there is no difference, I think using the Conv1d padding would be faster. |
Conv1d does not support asymmetric padding. And please don't place random questions to random places. |
I have tested tacotron2 + wavernn models on CPU, I have checked
to https://github.com/erogol/WaveRNN/blob/master/utils/distribution.py |
But still if I call same model on same input twice in a row I don't get the same result, does it have some internal state that should be release before second run? As I can see here: Also seems here hidden state is setted to zeros, so do I need it to set it to zeros before call generate function? Update:
|
hey Eren, |
Hey! ... no change. |
I'm trying to reproduce WaveRnn results on single GPU and it's quite slow(i.e. days of training). Here is my samples at checkpoint_230000 vs checkpoint_433000 (provided by @erogol) for same predicted mel spectrogram from tacotron2: examples.zip I wonder this meaningless speech is normal and is just due to smaller number of steps or something is really broken? |
something is really broken. I guess the char symbol order in the code does not match with the model. |
Yes, it still broken at 600k steps, but I'm training WaveRnn model only (on mel-spectrograms prepared by tacotron2 model), so as I understand Also I have checked prepared mel-spectrogram *.npy file by griffin-lim and by |
In In Tacotron2 config: In WaveRNN config: Also I have tried just to clone master of WaveRnn and use pretrained model:
Next saved checkpoint |
Yes, seems problem was in But why WaveRnn have problems with |
I am finalizing this thread since we solved Tacotron2 + WaveRNN |
Seems about 300k steps is sufficient: @erogol Can you elaborate on |
trims silences at the beginning and the end by thresholding. |
When training WaveRNN with mels from Tacotron2, which wavs do you use, the ground truth wavs file or the wavs generated by Tacotron2? When I use the ground truth wavs, there are some bugs in But I don't know how to fix it. |
May i ask how much time that cost you to train 300k steps using wavernn? |
@chynphh I faced that problem too. Have you solved it yet ? |
I am facing the same issue. Is it solved yet? |
it might be about triming the noise. Try diabling it if it is enabled or the otherway around. |
Hi @ethanstan what branches are you using? I found out that you need to use Tacotron 2 from the branch https://github.com/mozilla/TTS/tree/Tacotron2-iter-260K-824c091 and I think I used the WaveRNN from the Master branch. |
@Ivona221 I'm using the current TTS tacotron 2 master branch. Does this mean I need to retrain from that branch? I'm pretty happy with the output from Tacotron2 right now. Do you know if it was something in the config or what changed? Thank you! |
Yes when i was training I needed to change to that branch and train another model. The results are a bit worst, at least for my dataset (Macedonian language) but it is the only way I made it work. |
Tacotron2: https://arxiv.org/pdf/1712.05884.pdf
WaveRNN: https://github.com/erogol/WaveRNN forked from https://github.com/fatchord/WaveRNN
The idea is to add Tacotron2 as another alternative if it is really useful then the current model.
Best result so far: https://soundcloud.com/user-565970875/ljspeech-logistic-wavernn
Some findings:
Here is the alignment with entropy loss. However, if you keep the loss weight high, then it degrades the model's generalization for new words.
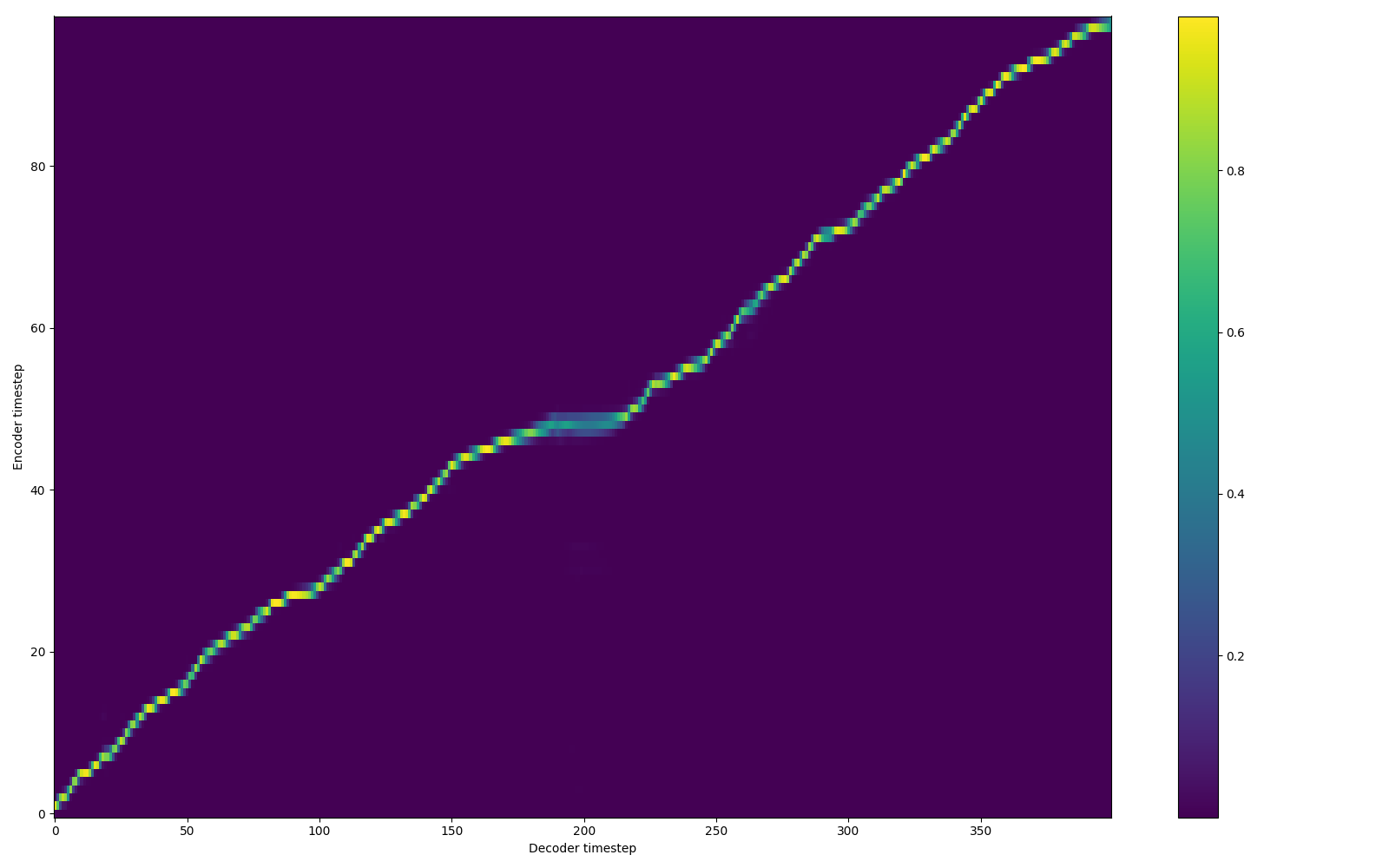
The text was updated successfully, but these errors were encountered: