You signed in with another tab or window. Reload to refresh your session.You signed out in another tab or window. Reload to refresh your session.You switched accounts on another tab or window. Reload to refresh your session.Dismiss alert
Therefore, a 3rd order polynomial with 4 variables expands to 34 polynomials (plus a zeroth order polynomial)
as a rule of thumb, with an L2 constraint the number of data points D should be approx
D ~= 10 * M
However, Lasso-Type Regularization and Bayesian Compressed Sensing can both fit against a large fraction of polynomials vs data points less than 10 * M
mesh perturbation
mesh size is a “hyperparameter”
numerical and not physical
Khachik is unsure if we can use this methodology to get sensitivity
notes
sparse quadrature
can different sampling methods per-variable improve the accuracy / efficiency of the surrogate?
here's an example of a sparse sampling method that the authors call "Smolyak sparse grid from the extrema of Chebyshev polynomials (Clenshaw-Curtis quadrature)"
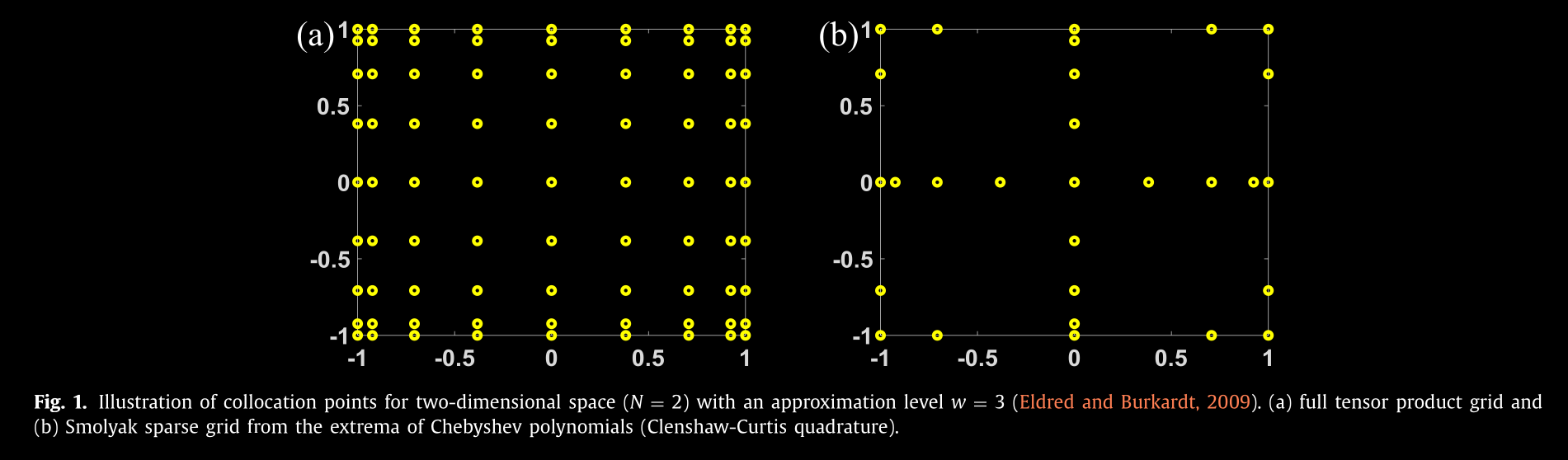
from https://www.sciencedirect.com/science/article/pii/S0098135419310026
methods and constraints
polynomial order / count determination
the number of expanded polynomials
M
can be found bywhere
N
is the number of variables andP
is the highest order.This is equation 5 from https://www.sciencedirect.com/science/article/pii/S0098135419310026:
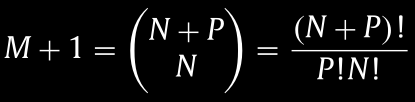
Therefore, a 3rd order polynomial with 4 variables expands to 34 polynomials (plus a zeroth order polynomial)
as a rule of thumb, with an L2 constraint the number of data points
D
should be approxHowever, Lasso-Type Regularization and Bayesian Compressed Sensing can both fit against a large fraction of polynomials vs data points less than
10 * M
mesh perturbation
next steps
The text was updated successfully, but these errors were encountered: