-
Notifications
You must be signed in to change notification settings - Fork 313
/
cs105_lab1b_word_count.py
411 lines (305 loc) · 20.4 KB
/
cs105_lab1b_word_count.py
1
2
3
4
5
6
7
8
9
10
11
12
13
14
15
16
17
18
19
20
21
22
23
24
25
26
27
28
29
30
31
32
33
34
35
36
37
38
39
40
41
42
43
44
45
46
47
48
49
50
51
52
53
54
55
56
57
58
59
60
61
62
63
64
65
66
67
68
69
70
71
72
73
74
75
76
77
78
79
80
81
82
83
84
85
86
87
88
89
90
91
92
93
94
95
96
97
98
99
100
101
102
103
104
105
106
107
108
109
110
111
112
113
114
115
116
117
118
119
120
121
122
123
124
125
126
127
128
129
130
131
132
133
134
135
136
137
138
139
140
141
142
143
144
145
146
147
148
149
150
151
152
153
154
155
156
157
158
159
160
161
162
163
164
165
166
167
168
169
170
171
172
173
174
175
176
177
178
179
180
181
182
183
184
185
186
187
188
189
190
191
192
193
194
195
196
197
198
199
200
201
202
203
204
205
206
207
208
209
210
211
212
213
214
215
216
217
218
219
220
221
222
223
224
225
226
227
228
229
230
231
232
233
234
235
236
237
238
239
240
241
242
243
244
245
246
247
248
249
250
251
252
253
254
255
256
257
258
259
260
261
262
263
264
265
266
267
268
269
270
271
272
273
274
275
276
277
278
279
280
281
282
283
284
285
286
287
288
289
290
291
292
293
294
295
296
297
298
299
300
301
302
303
304
305
306
307
308
309
310
311
312
313
314
315
316
317
318
319
320
321
322
323
324
325
326
327
328
329
330
331
332
333
334
335
336
337
338
339
340
341
342
343
344
345
346
347
348
349
350
351
352
353
354
355
356
357
358
359
360
361
362
363
364
365
366
367
368
369
370
371
372
373
374
375
376
377
378
379
380
381
382
383
384
385
386
387
388
389
390
391
392
393
394
395
396
397
398
399
400
401
402
403
404
405
406
407
408
409
410
411
# Databricks notebook source exported at Sat, 23 Jul 2016 13:31:09 UTC
# MAGIC %md
# MAGIC <a rel="license" href="http://creativecommons.org/licenses/by-nc-nd/4.0/"> <img alt="Creative Commons License" style="border-width:0" src="https://i.creativecommons.org/l/by-nc-nd/4.0/88x31.png"/> </a> <br/> This work is licensed under a <a rel="license" href="http://creativecommons.org/licenses/by-nc-nd/4.0/"> Creative Commons Attribution-NonCommercial-NoDerivatives 4.0 International License. </a>
# COMMAND ----------
# MAGIC %md
# MAGIC #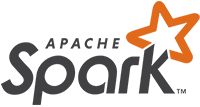 + 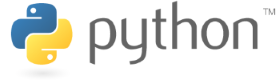
# MAGIC # **Word Count Lab: Building a word count application**
# MAGIC
# MAGIC This lab will build on the techniques covered in the Spark tutorial to develop a simple word count application. The volume of unstructured text in existence is growing dramatically, and Spark is an excellent tool for analyzing this type of data. In this lab, we will write code that calculates the most common words in the [Complete Works of William Shakespeare](http://www.gutenberg.org/ebooks/100) retrieved from [Project Gutenberg](http://www.gutenberg.org/wiki/Main_Page). This could also be scaled to larger applications, such as finding the most common words in Wikipedia.
# MAGIC
# MAGIC ** During this lab we will cover: **
# MAGIC * *Part 1:* Creating a base DataFrame and performing operations
# MAGIC * *Part 2:* Counting with Spark SQL and DataFrames
# MAGIC * *Part 3:* Finding unique words and a mean value
# MAGIC * *Part 4:* Apply word count to a file
# MAGIC
# MAGIC Note that for reference, you can look up the details of the relevant methods in [Spark's Python API](https://spark.apache.org/docs/latest/api/python/pyspark.html#pyspark.sql).
# COMMAND ----------
labVersion = 'cs105x-word-count-df-0.1.0'
# COMMAND ----------
# MAGIC %md
# MAGIC #### ** Part 1: Creating a base DataFrame and performing operations **
# COMMAND ----------
# MAGIC %md
# MAGIC In this part of the lab, we will explore creating a base DataFrame with `sqlContext.createDataFrame` and using DataFrame operations to count words.
# COMMAND ----------
# MAGIC %md
# MAGIC ** (1a) Create a DataFrame **
# MAGIC
# MAGIC We'll start by generating a base DataFrame by using a Python list of tuples and the `sqlContext.createDataFrame` method. Then we'll print out the type and schema of the DataFrame. The Python API has several examples for using the [`createDataFrame` method](http://spark.apache.org/docs/latest/api/python/pyspark.sql.html#pyspark.sql.SQLContext.createDataFrame).
# COMMAND ----------
wordsDF = sqlContext.createDataFrame([('cat',), ('elephant',), ('rat',), ('rat',), ('cat', )], ['word'])
wordsDF.show()
print type(wordsDF)
wordsDF.printSchema()
# COMMAND ----------
# MAGIC %md
# MAGIC ** (1b) Using DataFrame functions to add an 's' **
# MAGIC
# MAGIC Let's create a new DataFrame from `wordsDF` by performing an operation that adds an 's' to each word. To do this, we'll call the [`select` DataFrame function](http://spark.apache.org/docs/latest/api/python/pyspark.sql.html#pyspark.sql.DataFrame.select) and pass in a column that has the recipe for adding an 's' to our existing column. To generate this `Column` object you should use the [`concat` function](http://spark.apache.org/docs/latest/api/python/pyspark.sql.html#pyspark.sql.functions.concat) found in the [`pyspark.sql.functions` module](http://spark.apache.org/docs/latest/api/python/pyspark.sql.html#module-pyspark.sql.functions). Note that `concat` takes in two or more string columns and returns a single string column. In order to pass in a constant or literal value like 's', you'll need to wrap that value with the [`lit` column function](http://spark.apache.org/docs/latest/api/python/pyspark.sql.html#pyspark.sql.functions.lit).
# MAGIC
# MAGIC Please replace `<FILL IN>` with your solution. After you have created `pluralDF` you can run the next cell which contains two tests. If you implementation is correct it will print `1 test passed` for each test.
# MAGIC
# MAGIC This is the general form that exercises will take. Exercises will include an explanation of what is expected, followed by code cells where one cell will have one or more `<FILL IN>` sections. The cell that needs to be modified will have `# TODO: Replace <FILL IN> with appropriate code` on its first line. Once the `<FILL IN>` sections are updated and the code is run, the test cell can then be run to verify the correctness of your solution. The last code cell before the next markdown section will contain the tests.
# MAGIC
# MAGIC > Note:
# MAGIC > Make sure that the resulting DataFrame has one column which is named 'word'.
# COMMAND ----------
# TODO: Replace <FILL IN> with appropriate code
from pyspark.sql.functions import lit, concat
pluralDF = wordsDF.<FILL IN>
pluralDF.show()
# COMMAND ----------
# Load in the testing code and check to see if your answer is correct
# If incorrect it will report back '1 test failed' for each failed test
# Make sure to rerun any cell you change before trying the test again
from databricks_test_helper import Test
# TEST Using DataFrame functions to add an 's' (1b)
Test.assertEquals(pluralDF.first()[0], 'cats', 'incorrect result: you need to add an s')
Test.assertEquals(pluralDF.columns, ['word'], "there should be one column named 'word'")
# COMMAND ----------
# MAGIC %md
# MAGIC ** (1c) Length of each word **
# MAGIC
# MAGIC Now use the SQL `length` function to find the number of characters in each word. The [`length` function](http://spark.apache.org/docs/latest/api/python/pyspark.sql.html#pyspark.sql.functions.length) is found in the `pyspark.sql.functions` module.
# COMMAND ----------
# TODO: Replace <FILL IN> with appropriate code
from pyspark.sql.functions import length
pluralLengthsDF = pluralDF.<FILL IN>
pluralLengthsDF.show()
# COMMAND ----------
# TEST Length of each word (1e)
from collections import Iterable
asSelf = lambda v: map(lambda r: r[0] if isinstance(r, Iterable) and len(r) == 1 else r, v)
Test.assertEquals(set(asSelf(pluralLengthsDF.collect())), {4, 9, 4, 4, 4},
'incorrect values for pluralLengths')
# COMMAND ----------
# MAGIC %md
# MAGIC #### ** Part 2: Counting with Spark SQL and DataFrames **
# COMMAND ----------
# MAGIC %md
# MAGIC Now, let's count the number of times a particular word appears in the 'word' column. There are multiple ways to perform the counting, but some are much less efficient than others.
# MAGIC
# MAGIC A naive approach would be to call `collect` on all of the elements and count them in the driver program. While this approach could work for small datasets, we want an approach that will work for any size dataset including terabyte- or petabyte-sized datasets. In addition, performing all of the work in the driver program is slower than performing it in parallel in the workers. For these reasons, we will use data parallel operations.
# COMMAND ----------
# MAGIC %md
# MAGIC ** (2a) Using `groupBy` and `count` **
# MAGIC
# MAGIC Using DataFrames, we can preform aggregations by grouping the data using the [`groupBy` function](http://spark.apache.org/docs/latest/api/python/pyspark.sql.html#pyspark.sql.DataFrame.groupBy) on the DataFrame. Using `groupBy` returns a [`GroupedData` object](http://spark.apache.org/docs/latest/api/python/pyspark.sql.html#pyspark.sql.GroupedData) and we can use the functions available for `GroupedData` to aggregate the groups. For example, we can call `avg` or `count` on a `GroupedData` object to obtain the average of the values in the groups or the number of occurrences in the groups, respectively.
# MAGIC
# MAGIC To find the counts of words, group by the words and then use the [`count` function](http://spark.apache.org/docs/latest/api/python/pyspark.sql.html#pyspark.sql.GroupedData.count) to find the number of times that words occur.
# COMMAND ----------
# TODO: Replace <FILL IN> with appropriate code
wordCountsDF = (wordsDF
<FILL IN>)
wordCountsDF.show()
# COMMAND ----------
# TEST groupBy and count (2a)
Test.assertEquals(set(wordCountsDF.collect()), {('rat', 2), ('elephant', 1), ('cat', 2)},
'incorrect counts for wordCountsDF')
# COMMAND ----------
# MAGIC %md
# MAGIC #### ** Part 3: Finding unique words and a mean value **
# COMMAND ----------
# MAGIC %md
# MAGIC ** (3a) Unique words **
# MAGIC
# MAGIC Calculate the number of unique words in `wordsDF`. You can use other DataFrames that you have already created to make this easier.
# COMMAND ----------
from spark_notebook_helpers import printDataFrames
#This function returns all the DataFrames in the notebook and their corresponding column names.
printDataFrames(True)
# COMMAND ----------
# TODO: Replace <FILL IN> with appropriate code
uniqueWordsCount = <FILL IN>
print uniqueWordsCount
# COMMAND ----------
# TEST Unique words (3a)
Test.assertEquals(uniqueWordsCount, 3, 'incorrect count of unique words')
# COMMAND ----------
# MAGIC %md
# MAGIC ** (3b) Means of groups using DataFrames **
# MAGIC
# MAGIC Find the mean number of occurrences of words in `wordCountsDF`.
# MAGIC
# MAGIC You should use the [`mean` GroupedData method](http://spark.apache.org/docs/latest/api/python/pyspark.sql.html#pyspark.sql.GroupedData.mean) to accomplish this. Note that when you use `groupBy` you don't need to pass in any columns. A call without columns just prepares the DataFrame so that aggregation functions like `mean` can be applied.
# COMMAND ----------
# TODO: Replace <FILL IN> with appropriate code
averageCount = (wordCountsDF
<FILL IN>)
print averageCount
# COMMAND ----------
# TEST Means of groups using DataFrames (3b)
Test.assertEquals(round(averageCount, 2), 1.67, 'incorrect value of averageCount')
# COMMAND ----------
# MAGIC %md
# MAGIC #### ** Part 4: Apply word count to a file **
# COMMAND ----------
# MAGIC %md
# MAGIC In this section we will finish developing our word count application. We'll have to build the `wordCount` function, deal with real world problems like capitalization and punctuation, load in our data source, and compute the word count on the new data.
# COMMAND ----------
# MAGIC %md
# MAGIC ** (4a) The `wordCount` function **
# MAGIC
# MAGIC First, define a function for word counting. You should reuse the techniques that have been covered in earlier parts of this lab. This function should take in a DataFrame that is a list of words like `wordsDF` and return a DataFrame that has all of the words and their associated counts.
# COMMAND ----------
# TODO: Replace <FILL IN> with appropriate code
def wordCount(wordListDF):
"""Creates a DataFrame with word counts.
Args:
wordListDF (DataFrame of str): A DataFrame consisting of one string column called 'word'.
Returns:
DataFrame of (str, int): A DataFrame containing 'word' and 'count' columns.
"""
return <FILL IN>
wordCount(wordsDF).show()
# COMMAND ----------
# TEST wordCount function (4a)
res = [(row[0], row[1]) for row in wordCount(wordsDF).collect()]
Test.assertEquals(sorted(res),
[('cat', 2), ('elephant', 1), ('rat', 2)],
'incorrect definition for wordCountDF function')
# COMMAND ----------
# MAGIC %md
# MAGIC ** (4b) Capitalization and punctuation **
# MAGIC
# MAGIC Real world files are more complicated than the data we have been using in this lab. Some of the issues we have to address are:
# MAGIC + Words should be counted independent of their capitialization (e.g., Spark and spark should be counted as the same word).
# MAGIC + All punctuation should be removed.
# MAGIC + Any leading or trailing spaces on a line should be removed.
# MAGIC
# MAGIC Define the function `removePunctuation` that converts all text to lower case, removes any punctuation, and removes leading and trailing spaces. Use the Python [regexp_replace](http://spark.apache.org/docs/latest/api/python/pyspark.sql.html#pyspark.sql.functions.regexp_replace) module to remove any text that is not a letter, number, or space. If you are unfamiliar with regular expressions, you may want to review [this tutorial](https://developers.google.com/edu/python/regular-expressions) from Google. Also, [this website](https://regex101.com/#python) is a great resource for debugging your regular expression.
# MAGIC
# MAGIC You should also use the `trim` and `lower` functions found in [pyspark.sql.functions](http://spark.apache.org/docs/latest/api/python/pyspark.sql.html#pyspark.sql.functions).
# MAGIC
# MAGIC > Note that you shouldn't use any RDD operations or need to create custom user defined functions (udfs) to accomplish this task
# COMMAND ----------
# TODO: Replace <FILL IN> with appropriate code
from pyspark.sql.functions import regexp_replace, trim, col, lower
def removePunctuation(column):
"""Removes punctuation, changes to lower case, and strips leading and trailing spaces.
Note:
Only spaces, letters, and numbers should be retained. Other characters should should be
eliminated (e.g. it's becomes its). Leading and trailing spaces should be removed after
punctuation is removed.
Args:
column (Column): A Column containing a sentence.
Returns:
Column: A Column named 'sentence' with clean-up operations applied.
"""
return <FILL IN>
sentenceDF = sqlContext.createDataFrame([('Hi, you!',),
(' No under_score!',),
(' * Remove punctuation then spaces * ',)], ['sentence'])
sentenceDF.show(truncate=False)
(sentenceDF
.select(removePunctuation(col('sentence')))
.show(truncate=False))
# COMMAND ----------
# TEST Capitalization and punctuation (4b)
testPunctDF = sqlContext.createDataFrame([(" The Elephant's 4 cats. ",)])
Test.assertEquals(testPunctDF.select(removePunctuation(col('_1'))).first()[0],
'the elephants 4 cats',
'incorrect definition for removePunctuation function')
# COMMAND ----------
# MAGIC %md
# MAGIC ** (4c) Load a text file **
# MAGIC
# MAGIC For the next part of this lab, we will use the [Complete Works of William Shakespeare](http://www.gutenberg.org/ebooks/100) from [Project Gutenberg](http://www.gutenberg.org/wiki/Main_Page). To convert a text file into a DataFrame, we use the `sqlContext.read.text()` method. We also apply the recently defined `removePunctuation()` function using a `select()` transformation to strip out the punctuation and change all text to lower case. Since the file is large we use `show(15)`, so that we only print 15 lines.
# COMMAND ----------
fileName = "dbfs:/databricks-datasets/cs100/lab1/data-001/shakespeare.txt"
shakespeareDF = sqlContext.read.text(fileName).select(removePunctuation(col('value')))
shakespeareDF.show(15, truncate=False)
# COMMAND ----------
# MAGIC %md
# MAGIC ** (4d) Words from lines **
# MAGIC
# MAGIC Before we can use the `wordcount()` function, we have to address two issues with the format of the DataFrame:
# MAGIC + The first issue is that that we need to split each line by its spaces.
# MAGIC + The second issue is we need to filter out empty lines or words.
# MAGIC
# MAGIC Apply a transformation that will split each 'sentence' in the DataFrame by its spaces, and then transform from a DataFrame that contains lists of words into a DataFrame with each word in its own row. To accomplish these two tasks you can use the `split` and `explode` functions found in [pyspark.sql.functions](http://spark.apache.org/docs/latest/api/python/pyspark.sql.html#pyspark.sql.functions).
# MAGIC
# MAGIC Once you have a DataFrame with one word per row you can apply the [DataFrame operation `where`](http://spark.apache.org/docs/latest/api/python/pyspark.sql.html#pyspark.sql.DataFrame.where) to remove the rows that contain ''.
# MAGIC
# MAGIC > Note that `shakeWordsDF` should be a DataFrame with one column named `word`.
# COMMAND ----------
# TODO: Replace <FILL IN> with appropriate code
from pyspark.sql.functions import split, explode
shakeWordsDF = (shakespeareDF
<FILL IN>)
shakeWordsDF.show()
shakeWordsDFCount = shakeWordsDF.count()
print shakeWordsDFCount
# COMMAND ----------
# TEST Remove empty elements (4d)
Test.assertEquals(shakeWordsDF.count(), 882996, 'incorrect value for shakeWordCount')
Test.assertEquals(shakeWordsDF.columns, ['word'], "shakeWordsDF should only contain the Column 'word'")
# COMMAND ----------
# MAGIC %md
# MAGIC ** (4e) Count the words **
# MAGIC
# MAGIC We now have a DataFrame that is only words. Next, let's apply the `wordCount()` function to produce a list of word counts. We can view the first 20 words by using the `show()` action; however, we'd like to see the words in descending order of count, so we'll need to apply the [`orderBy` DataFrame method](http://spark.apache.org/docs/latest/api/python/pyspark.sql.html#pyspark.sql.DataFrame.orderBy) to first sort the DataFrame that is returned from `wordCount()`.
# MAGIC
# MAGIC You'll notice that many of the words are common English words. These are called stopwords. In a later lab, we will see how to eliminate them from the results.
# COMMAND ----------
# TODO: Replace <FILL IN> with appropriate code
from pyspark.sql.functions import desc
topWordsAndCountsDF = <FILL IN>
topWordsAndCountsDF.show()
# COMMAND ----------
# TEST Count the words (4e)
Test.assertEquals(topWordsAndCountsDF.take(15),
[(u'the', 27361), (u'and', 26028), (u'i', 20681), (u'to', 19150), (u'of', 17463),
(u'a', 14593), (u'you', 13615), (u'my', 12481), (u'in', 10956), (u'that', 10890),
(u'is', 9134), (u'not', 8497), (u'with', 7771), (u'me', 7769), (u'it', 7678)],
'incorrect value for top15WordsAndCountsDF')
# COMMAND ----------
# MAGIC %md
# MAGIC #### ** Prepare to the course autograder **
# MAGIC Once you confirm that your lab notebook is passing all tests, you can submit it first to the course autograder and then second to the edX website to receive a grade.
# MAGIC <img src="https://d37djvu3ytnwxt.cloudfront.net/asset-v1:BerkeleyX+CS105x+1T2016+type@asset+block/url-process.png" alt="Drawing" />
# MAGIC
# MAGIC ** Note that you can only submit to the course autograder once every 1 minute. **
# COMMAND ----------
# MAGIC %md
# MAGIC ** (a) Restart your cluster by clicking on the dropdown next to your cluster name and selecting "Restart Cluster".**
# MAGIC
# MAGIC You can do this step in either notebook, since there is one cluster for your notebooks.
# MAGIC
# MAGIC <img src="http://spark-mooc.github.io/web-assets/images/submit_restart.png" alt="Drawing" />
# COMMAND ----------
# MAGIC %md
# MAGIC ** (b) _IN THIS NOTEBOOK_, click on "Run All" to run all of the cells. **
# MAGIC
# MAGIC <img src="http://spark-mooc.github.io/web-assets/images/submit_runall.png" alt="Drawing" />
# MAGIC
# MAGIC This step will take some time. While the cluster is running all the cells in your lab notebook, you will see the "Stop Execution" button.
# MAGIC
# MAGIC <img src="http://spark-mooc.github.io/web-assets/images/stop_execution.png" alt="Drawing" />
# MAGIC
# MAGIC Wait for your cluster to finish running the cells in your lab notebook before proceeding.
# COMMAND ----------
# MAGIC %md
# MAGIC ** (c) Verify that your LAB notebook passes as many tests as you can. **
# MAGIC
# MAGIC Most computations should complete within a few seconds unless stated otherwise. As soon as the expression of a cell have been successfully evaluated, you will see one or more "test passed" messages if the cell includes test expressions:
# MAGIC
# MAGIC <img src="http://spark-mooc.github.io/web-assets/images/test_passed.png" alt="Drawing" />
# MAGIC
# MAGIC or just execution time otherwise:
# MAGIC <img src="http://spark-mooc.github.io/web-assets/images/execution_time.png" alt="Drawing" />
# COMMAND ----------
# MAGIC %md
# MAGIC ** (d) Publish your LAB notebook(this notebook) by clicking on the "Publish" button at the top of your LAB notebook. **
# MAGIC
# MAGIC <img src="http://spark-mooc.github.io/web-assets/images/Lab0_Publish0.png" alt="Drawing" />
# MAGIC
# MAGIC When you click on the button, you will see the following popup.
# MAGIC
# MAGIC <img src="http://spark-mooc.github.io/web-assets/images/Lab0_Publish1.png" alt="Drawing" />
# MAGIC
# MAGIC When you click on "Publish", you will see a popup with your notebook's public link. __Copy the link and set the notebook_URL variable in the AUTOGRADER notebook(not this notebook).__
# MAGIC
# MAGIC <img src="http://spark-mooc.github.io/web-assets/images/Lab0_Publish2.png" alt="Drawing" />