-
-
Notifications
You must be signed in to change notification settings - Fork 3.5k
New issue
Have a question about this project? Sign up for a free GitHub account to open an issue and contact its maintainers and the community.
By clicking “Sign up for GitHub”, you agree to our terms of service and privacy statement. We’ll occasionally send you account related emails.
Already on GitHub? Sign in to your account
ONNX model output boxes are all zeros. #1172
Comments
To make things more clear, I also tested with |
@vandesa003 the warning is normal, but opset 9 export is unsupported, so you are on your own if you choose to pass that argument. Recommend you retry with the latest versions of pytorch and onnx, and opset 10 or 11. |
@vandesa003 also make sure you are using the latest code when you convert: run |
Sure @glenn-jocher I also tested with |
Hi @glenn-jocher , finally I fixed the problem after pulling the new code. Thanks a lot! But I compared the box output from pytorch and onnx model and found that:
Just wonder is the onnx box outputs are normalized? I need to multiply by the image size? |
@vandesa003 yes they are normalized. These are the requirements of the the coreml model in |
@glenn-jocher Oh I see. I tried to restore the result by multiply the image size, but it seems not exact same. How can I restore the exact result? |
@vandesa003 actually looking at the code there are no normalization steps, so they should be in pixel space. You can compare how the two outputs are handled here: Lines 196 to 217 in 3f27ef1
|
@vandesa003 ah yes, I was correct originally. 1/ng is normalizing it in grid space. |
First of all thanks for your work. I successfully converted the model to a onnx model (opset_version = 10) and to a tensorrt. I just don't know how to use this prediction to draw the bounding boxes etc...
The output(prediction) of the torch model: with shape:
The output(prediction) of tensorrt/onnx model: with shape:
I just want to know how to use these values to build the predictions of the model. |
@gasparramoa use netron to view. |
I used, I just don't know how to use the result to build the prediction. |
@gasparramoa the outputs are the boxes and the confidences (0-1) of each class (looks like you have a single-class model), you can see them right there in your screenshot. What else do you need? |
So, what I need to do is to find the max value of confidence and use the bounding boxes for that confidence. Am I right? |
@gasparramoa I can't advise you on this, if you want please open a new issue as this original issue is resolved. |
This issue has been resolved in a commit in early May 2020. If you are having this issue update your code with git pull or clone a new repo. |
@gasparramoa as I said in previous reply, the output of onnx model is normalised in anchor wise. You only need to remove the normalised process, then everything is ok. |
@glenn-jocher Sorry I should have closed this issue. Now I restored the normalised value and I can use it! thanks again for you guys great work! learned a lot from your repo. |
So I can not restore the result by multiply the image size? What is normalization in achor wise ? Can you give me a simple example of how to remove this normalization process please? |
Just multiply by the
|
Thank you @vandesa003 !!! |
@gasparramoa Where you able to get the onnx model into a tensorRT model? If so, did you use the onnx-tensorRT github for that? If not, what tool did you use and what is your performance? Thanks in advance! |
@gasparramoa @mbufi there's a request for tensorrt on our new repo as well. I personally don't have experience with it, but if you guys have time or suggestions that would be awesome. There is a tensorrt export here as well that is already working for this repo: |
@glenn-jocher Yes I saw! Thanks for the suggestion. I may look into it. |
@vandesa003 @gasparramoa @glenn-jocher I'm running into some issues where the torch output and onnx model outputs do not match in the current version of the repo. Steps to reproduce:
However, when I was debugging, I saw that the onnxruntime output and the pytorch model outputs do not match: pytorch output (after running inference but before nms):
onnx runtime output (after running inference but before nms):
I added the fix from @vandesa003 ( |
@prathik-naidu we offer model export to onnx and coreml as a service. If you are interested please send us a request via email. |
@glenn-jocher I'm just running on the current open source yolov3 code given that it has ONNX export functionality. Does this not work? I'm using the default yolov3-spp.cfg and yolov3-spp.pt files that are from the repo but still not able to match the outputs between pytorch and onnxruntime. |
@glenn-jocher yes, there is limited export functionality available here! If you can get by with this then great :) |
@glenn-jocher I see so just to clarify, what is currently possible with the export functionality in this repo? It seems like there is capability to export to an onnx file but that onnx file doesn't actually replicate the results of the pytorch model. Is that expected? Is there something that needs to be changed with this open source code to get that working (not sure if I'm missing something) or does this functionality not exist? |
@prathik-naidu export works as intended here. If you need additional help we can provide it as a service. |
So can't we use the exported onnx model normally? I wanted to use OPENCV of C + + to call the exported onnx model, and then use C + + reasoning to deploy the project. But if the prediction result of onnx model is not correct, does that mean that the result of subsequent deployment will also be incorrect |
@sky-fly97 export operates correctly. |
Oh, Thank you! I see that the above person said that the output of the exported onnx model is quite inconsistent with the original pytorch model, so I have such a question.By the way, thank you very much for your work, which is really important |
@sky-fly97 Let me know if you are able to get consistent results with your work. I'm still not able to figure out why the exported onnx model generates different results from the pytorch model (even on simple inputs like torch.ones). If export works correctly, I assume that means the model that is loaded from the onnx file should also work as well? |
OK。I will try. I have another question, why does the onnx model take (320,192) as the input size.Does it matter? |
Hello, I have also successfully converted the downloaded yolov3.weights into onnx, but the error in converting to tensor RT is as follows: [TensorRT] ERROR: Network must have at least one output Traceback (most recent call last): context = engine.create_ execution_ context() AttributeError: 'NoneType' object has no attribute 'create_ execution_ context' |
@prathik-naidu , I have results similar to yours (onnx pred probabilities around 10e-7), have you figured it out ? |
hi , my onnx model have two output how to decode this output and get final result? could you give me some advice? thanks!!!! |
Hi @dengfenglai321! Great to hear that you've made progress and resolved the issue after updating the code! Regarding the differences in box output between PyTorch and ONNX, the ONNX output appears to be normalized. Depending on your requirements, you may need to multiply the ONNX box outputs by the image size to obtain the final result. As for decoding the output and obtaining the final result, we have inferred that you could benefit from the documentation at https://docs.ultralytics.com for guidance on decoding the ONNX output. Please feel free to reach out if you have any more questions or need further assistance. Good luck with your project! |
🐛 Bug
Hi @glenn-jocher , first of all, thanks again for your great work. I met this problem after I trained on my own dataset and convert the model to ONNX. While I am running the ONNX model on a normal input image, I got the output like this:
the classes output seems normal just between 0 and 1.
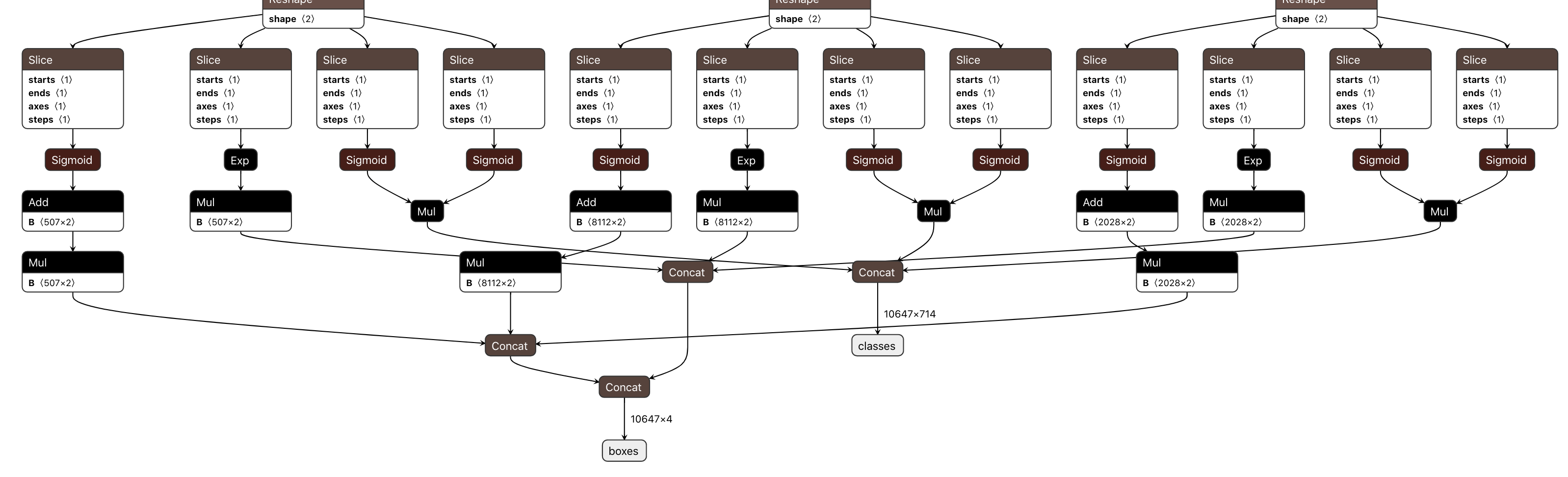
this is the ONNX model:
which I think should be correct.
To Reproduce
REQUIRED: Code to reproduce your issue below
First, I set
ONNX_EXPORT = True
in [model.py])(yolov3/models.py
Line 5 in b2fcfc5
Then, due to the machine env problem, I have to use
opset_version=9
in detect.pyAfter this I convert the model to onnx:
I will receive a warning during conversion:
which I think is related to
yolov3/utils/layers.py
Line 60 in b2fcfc5
But I am not sure whether this warning will cause this issue.
After then I run the inference through onnxruntime. and got a normal
classes
output and a all zero outputboxes
.Expected behavior
Expected behavior the boxes definitely not all zeros.
Environment
If applicable, add screenshots to help explain your problem.
The text was updated successfully, but these errors were encountered: