forked from alibaba/EasyTransfer
-
Notifications
You must be signed in to change notification settings - Fork 0
Commit
This commit does not belong to any branch on this repository, and may belong to a fork outside of the repository.
- Loading branch information
1 parent
b49fc43
commit a98f0e4
Showing
6 changed files
with
183 additions
and
0 deletions.
There are no files selected for viewing
Binary file not shown.
Binary file not shown.
Binary file not shown.
This file contains bidirectional Unicode text that may be interpreted or compiled differently than what appears below. To review, open the file in an editor that reveals hidden Unicode characters.
Learn more about bidirectional Unicode characters
Original file line number | Diff line number | Diff line change |
---|---|---|
@@ -0,0 +1,183 @@ | ||
# 知识蒸馏实践 | ||
|
||
# 目录 | ||
1. [基础知识蒸馏](#IWsvI) | ||
1. [Probes蒸馏](#sDvg8) | ||
1. [AdaBERT蒸馏](#kvb8c) | ||
|
||
|
||
|
||
# 简介 | ||
随着BERT等预训练语言模型在各项任务上都取得了STOA效果,BERT这类模型已经成为 NLP 深度迁移学习管道中的重要组成部分。但 BERT 并不是完美无瑕的,这类模型仍然存在以下两个问题: | ||
|
||
1. **模型参数量太大**:BERT-base 模型能够包含一亿个参数,较大的 BERT-large 甚至包含 3.4 亿个参数。显然,很难将这种规模的模型部署到资源有限的环境(例如移动设备或嵌入式系统)当中。 | ||
1. **训练/推理速度慢**:在基于 Pod 配置的 4 个 Cloud TPUs(总共 16 个 TPU 芯片)上对 BERT-base 进行训练,或者在 16 个 Cloud TPU(总共 64 个 TPU 芯片)上对 BERT-large 进行训练,每次预训练都需要至少 4 天的时间才能完成。而BERT的推理速度更是严重影响到了需要较高QPS的线上场景,部署成本非常高。 | ||
|
||
|
||
|
||
而这个问题,不仅仅是在NLP领域存在,计算机视觉也同样存在,通常来讲有以下三种解决方案: | ||
|
||
1. **架构改进**:将原有的架构改进为更小/更快的架构,例如,将 RNN 替换为 Transformer 或 CNN,ALBERT替代BERT等;使用需要较少计算的层等。当然也可以采用其他优化,例如从学习率和策略、预热步数,较大的批处理大小等; | ||
1. **模型压缩**:通常使用量化和修剪来完成,从而能够在架构不变(或者大部分架构不变)的情况下减少计算总量; | ||
1. **知识蒸馏**:训练一个小的模型,使得其在相应任务行为上能够逼近大的模型的效果,如DistillBERT,BERT-PKD,TinyBERT等 | ||
|
||
|
||
|
||
PAI迁移学习团队,在知识蒸馏上有相关的积累,如我们提出的AdaBERT,利用可微神经网络架构搜索来自动将BERT蒸馏成适应不同特定任务的小型模型。在多个NLP任务上的结果表明这些任务适应型压缩模型在保证表现相当的同时,推理速度比BERT快10~30倍,同时参数缩小至BERT的十分之一。本教程提供简单易用的示例,来进行BERT的知识蒸馏。 | ||
|
||
|
||
本教程的自定义构图代码、脚本文件可见 [[链接]](https://github.com/alibaba/EasyTransfer/tree/master/scripts/knowledge_distillation) | ||
|
||
|
||
# 1. 基础知识蒸馏 | ||
基础知识蒸馏主要是Student学习Teacher的logits,即DarkKnowledge。 | ||
|
||
|
||
相关论文: | ||
[Distilling the knowledge in a neural network](https://arxiv.org/abs/1503.02531). Hinton et al. _NIPS Deep Learning and Representation Learning Workshop_ (2015) | ||
**数据准备** | ||
本教程以SST-2数据作为样例数据,用户可以下载 [训练集](http://atp-modelzoo-sh.oss-cn-shanghai.aliyuncs.com/tutorial/knowledge_distillation/SST-2/train.tsv) 和 [评估集](http://atp-modelzoo-sh.oss-cn-shanghai.aliyuncs.com/tutorial/knowledge_distillation/SST-2/dev.tsv),这些 `.csv` 文件以 `\t` 分隔,分为content和label两列,如下所示: | ||
``` | ||
it 's a charming and often affecting journey . 1 | ||
unflinchingly bleak and desperate 0 | ||
``` | ||
### 1.1 训练Teacher | ||
我们可以输入以下命令训练基础蒸馏下的Teacher模型 | ||
```bash | ||
$ sh run_distill.sh vanilla teacher_train | ||
``` | ||
待模型训练完毕后,用以下命令输出最优teacher模型的logits | ||
```bash | ||
$ sh run_distill.sh vanilla teacher_predict | ||
``` | ||
> 注:如果要选用最优的checkpoint,我们可以通过训练时的evaluation结果选择最优的checkpoint,然后修改 `./config/vanilla_teacher_config.json` 中的 `predict_checkpoint_path` 。 | ||
|
||
|
||
最后会生成 `sst2_train_logits.tsv` 文件和 `sst2_dev_logits.tsv` 文件,示例如下: | ||
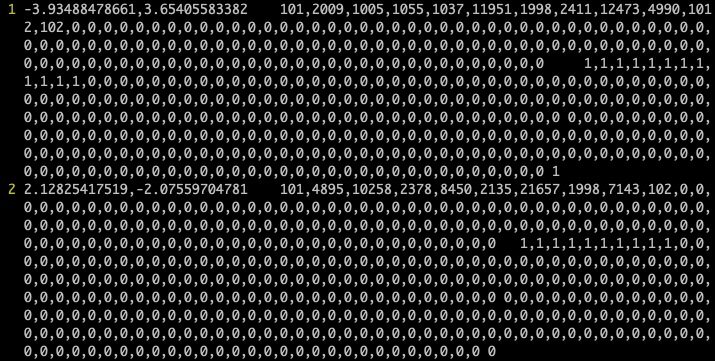 | ||
### 1.2 训练Student | ||
在生成logits文件后,Student通过直接读取logits来进行蒸馏,训练命令如下: | ||
```bash | ||
$ sh run_distill.sh vanilla student_train | ||
``` | ||
训练好的模型进行预测,命令如下: | ||
```bash | ||
$ sh run_distill.sh vanilla student_predict | ||
``` | ||
# 2. Probes知识蒸馏 | ||
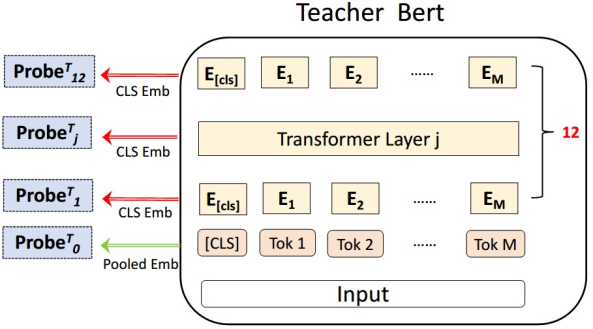 | ||
AdaBERT中提出将每一层的输出作为输入,学习相应的Probes来对Student进行蒸馏。本节主要用到了这个方法,Student为同构的Transformer架构。 | ||
|
||
|
||
相关论文: | ||
[AdaBERT,Task-Adaptive BERT Compression with Differentiable Neural Architecture Search](https://arxiv.org/abs/2001.04246), Daoyuan Chen, Yaliang Li, Minghui Qiu, Zhen Wang, Bofang Li, Bolin Ding, Hongbo Deng, Jun Huang, Wei Lin, Jingren Zhou, IJCAI, 2020 | ||
### 2.1 训练Teacher | ||
我们用第1章同样的数据训练Probes的Teacher,可以输入以下命令训练Probes Teacher模型 | ||
```bash | ||
$ sh run_distill.sh probes teacher_train | ||
``` | ||
待模型训练完毕后,用以下命令输出最优teacher模型的logits | ||
```bash | ||
$ sh run_distill.sh probes teacher_predict | ||
``` | ||
最后会生成 `sst2_train_probes.tsv` 文件和 `sst2_dev_probes.tsv` 文件,示例如下: | ||
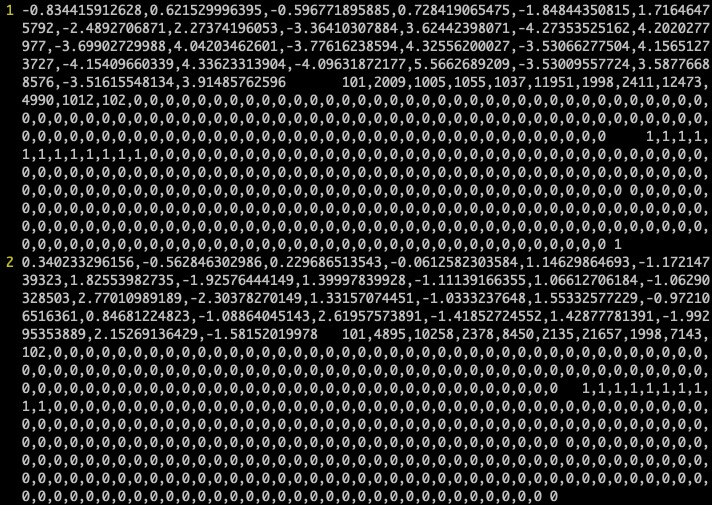 | ||
|
||
|
||
### 2.2 训练Student | ||
在生成logits文件后,Student通过直接读取logits来进行蒸馏,训练命令如下: | ||
```bash | ||
$ sh run_distill.sh probes student_train | ||
``` | ||
训练好的模型进行预测,命令如下: | ||
```bash | ||
$ sh run_distill.sh probes student_predict | ||
``` | ||
# 3. AdaBERT知识蒸馏 | ||
AdaBERT算法在目标任务上通过神经架构搜索(NAS)的方法搜索到性能相当且模型大小与推理速度能满足约束的神经网络架构。在多个NLP任务上的结果表明这些任务适应型压缩模型在保证表现相当的同时,推理速度比BERT快10~30倍,同时参数缩小至BERT的十分之一。本框架重点研究,以及提供简单易用的工具,来进行BERT的知识蒸馏。 | ||
|
||
|
||
我们在搜索过程中首先定义如下图所示的神经网络架构: | ||
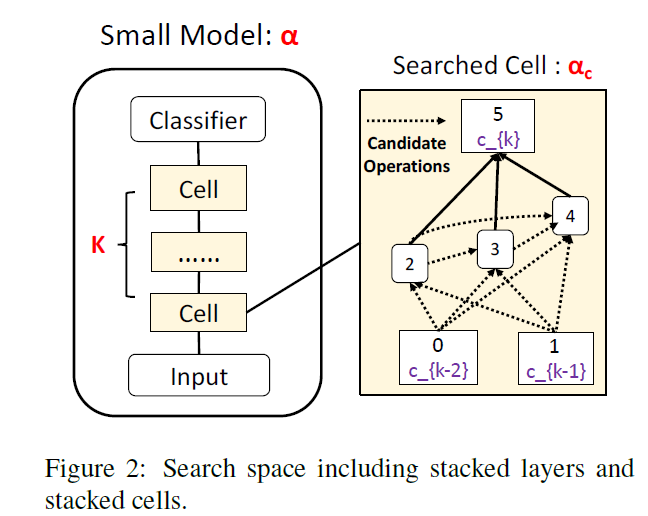 | ||
图中每个cell中,除了两个输入节点和一个输出节点,我们考虑3个中间节点。每条进入中间节点的边有10种候选算子:cnn3, cnn5, cnn7, dilated_cnn3, dilated_cnn5, dilated_cnn7, avg_pool, max_pool, identity, zero。我们的目的就是学习架构参数,表明每条边我们应该选取哪种算子,同时表明哪些边应该保留(最终要求每个中间节点只有两条入边)。 | ||
我们通过优化由三部分组成的loss来学习模型和架构参数,我们通过两个超参数进行平衡: | ||
 | ||
其中是分类任务本身的cross entropy loss,是每个cell对应的output logits与teacher提供的logits之间的cross entropy,可以理解为知识蒸馏(knowledge distill),是惩罚参数更大推理速度更慢的op以及cell个数的efficiency loss,目的是鼓励更轻量化的神经架构。 | ||
搜索过程中我们轮巡地相对于模型参数和相对于架构参数来最小化上述损失函数,最终记录下架构参数。 | ||
finetune步骤我们根据架构参数导出对应的最优神经架构,根据该结构建立计算图描述轻量化的模型,进行finetune。 | ||
详细的算法介绍见我们的论文:[AdaBERT,Task-Adaptive BERT Compression with Differentiable Neural Architecture Search](https://arxiv.org/abs/2001.04246), Daoyuan Chen, Yaliang Li, Minghui Qiu, Zhen Wang, Bofang Li, Bolin Ding, Hongbo Deng, Jun Huang, Wei Lin, Jingren Zhou, IJCAI, 2020 | ||
> 注意:本教程所用代码是AdaBERT的tensorflow复现版,pytorch正式版将在不久发布 | ||
|
||
|
||
### 3.1 获取输入 | ||
我们用第二章Probes知识蒸馏可以得到以下的文件: | ||
|
||
- train,dev的probes logits文件 | ||
- 最优的checkpoint | ||
|
||
我们可以用下面这段代码获取 这个checkpoint 模型的word embedding 和position embedding: | ||
```python | ||
import numpy as np | ||
import tensorflow as tf | ||
|
||
with tf.Session() as sess: | ||
saver = tf.train.import_meta_graph(checkpoint_path + ".meta") | ||
saver.restore(sess, checkpoint_path) | ||
graph = tf.get_default_graph() | ||
|
||
w = graph.get_tensor_by_name("bert_pre_trained_model/bert/embeddings/word_embeddings:0") | ||
w_saved = sess.run(w) | ||
np.save(os.path.join(output_dir, "wemb.npy"), w_saved) | ||
w = graph.get_tensor_by_name("bert_pre_trained_model/bert/embeddings/position_embeddings:0" | ||
) | ||
w_saved = sess.run(w) | ||
np.save(os.path.join(output_dir, "pemb.npy"), w_saved) | ||
``` | ||
在这个教程中,我们给出MRPC数据的相关输入文件,可从这个[链接](http://atp-modelzoo-sh.oss-cn-shanghai.aliyuncs.com/tutorial/knowledge_distillation/adabert/mrpc.zip)下载 | ||
### 3.2 搜索最优框架 | ||
```bash | ||
$ sh run_adabert.sh search | ||
``` | ||
### 3.3 Finetune并预测 | ||
我们获得了一系列search的模型后,从log中挑选最优Acc的checkpoint,将相应文件拷贝到 ./adabert_models/search/best目录中,如下所示: | ||
```bash | ||
$ ls adabert_models/search/best | ||
arch.json | ||
checkpoint | ||
model.ckpt-308.data-00000-of-00002 | ||
model.ckpt-308.data-00001-of-00002 | ||
model.ckpt-308.index | ||
model.ckpt-308.meta | ||
pemb.npy | ||
wemb.npy | ||
``` | ||
然后我们继续运行脚本,选用finetune模式 | ||
```bash | ||
$ sh run_adabert.sh finetune | ||
``` | ||
最后看到AdaBERT结果输出: | ||
 | ||
|
||
|
||
# 4. 效果评测 | ||
| SST-2 | #Parameters | Accuracy | | ||
| :---: | :---: | :---: | | ||
| **Teacher** | ||
(uncased_L-12_H-768_A-12) | 103M | 92.1% | | ||
| **Vanilla-KD** | ||
(uncased_L-4_H-512_A-8) | 29M | 89.2% | | ||
| **Probes-KD** | ||
(uncased_L-4_H-512_A-8) | 29M | 90.9% | | ||
|
||
|
||
|
||
|
||
| MRPC | F1 | | ||
| :---: | :---: | | ||
| AdaBERT (w/o DA, paper) | 78.70% | | ||
| AdaBERT (w/o DA, reproduce) | 80.86%±1.28% | | ||
|
||
|
||
|
Binary file not shown.
Binary file not shown.