This repository has been archived by the owner on Nov 17, 2023. It is now read-only.
-
Notifications
You must be signed in to change notification settings - Fork 6.8k
Commit
This commit does not belong to any branch on this repository, and may belong to a fork outside of the repository.
[MXNET-102] Added tutorial on how to use data augmenters.
- Loading branch information
Thom Lane
committed
Mar 14, 2018
1 parent
2a9c7d9
commit a0c4fb9
Showing
1 changed file
with
209 additions
and
0 deletions.
There are no files selected for viewing
This file contains bidirectional Unicode text that may be interpreted or compiled differently than what appears below. To review, open the file in an editor that reveals hidden Unicode characters.
Learn more about bidirectional Unicode characters
Original file line number | Diff line number | Diff line change |
---|---|---|
@@ -0,0 +1,209 @@ | ||
# Methods of applying data augmentation | ||
|
||
Data Augmentation is a regularization technique that's used to avoid overfitting when training Machine Learning models. Although the technique can be applied in a variety of domains, it's very common in Computer Vision. Adjustments are made to the original images in the training dataset before being used in training. Some example adjustments include translating, croping, scaling, rotating, changing brightness and contrast. We do this to reduce the dependence of the model on spurious characteristics; e.g. training data may only contain faces that fill 1/4 of the image, so the model trained without data augmentation might unhelpfully learn that faces can only be of this size. | ||
|
||
In this tutorial we discuss the different interfaces avaliable in MXNet to perform data augmentation. We start by showing a complete example using Gluon's [`mxnet.gluon.data.Dataset`](https://mxnet.incubator.apache.org/api/python/gluon/data.html#mxnet.gluon.data.Dataset), and then unpack the example to gain a greater understanding of the internals. In the process you'll learn about augmentation functions, [`mxnet.image.Augmenter`](https://mxnet.incubator.apache.org/api/python/image/image.html?highlight=augmen#mxnet.image.Augmenter) classes and Augmenter lists. We also provide examples using [`mxnet.image.ImageIter`](https://mxnet.incubator.apache.org/api/python/image/image.html?highlight=imageiter#mxnet.image.ImageIter) if you haven't yet moved to Gluon. | ||
|
||
|
||
```python | ||
%matplotlib inline | ||
import mxnet as mx # used version '1.0.0' at time of writing | ||
import numpy as np | ||
from matplotlib.pyplot import imshow | ||
import multiprocessing | ||
|
||
mx.random.seed(42) # set seed for repeatability | ||
``` | ||
|
||
We define a utility function below, that will be used for visualising the augmentations in the tutorial. | ||
|
||
|
||
```python | ||
def plot_mx_array(array): | ||
""" | ||
Array expected to be height x width x 3 (channels), and values are floats between 0 and 255. | ||
""" | ||
assert array.shape[2] == 3, "RGB Channel should be last" | ||
imshow((array.clip(0, 255)/255).asnumpy()) | ||
``` | ||
|
||
```python | ||
!mkdir -p data/images | ||
!wget https://raw.githubusercontent.com/dmlc/web-data/master/mxnet/doc/tutorials/data_aug/inputs/0.jpg -P ./data/images/ | ||
``` | ||
|
||
```python | ||
example_image = mx.image.imread("./data/images/0.jpg").astype("float32") | ||
plot_mx_array(example_image) | ||
``` | ||
|
||
|
||
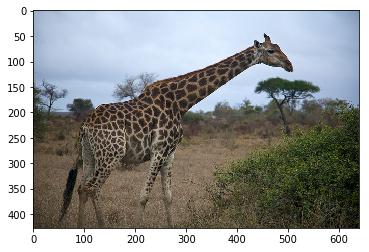 | ||
|
||
|
||
## Quick start with Gluon | ||
|
||
Using Gluon, it's simple to add data augmentation to your training pipeline. When creating either [`ImageFolderDataset`](https://mxnet.incubator.apache.org/api/python/gluon/data.html#mxnet.gluon.data.vision.datasets.ImageFolderDataset) or [`ImageRecordDataset`](https://mxnet.incubator.apache.org/api/python/gluon/data.html#mxnet.gluon.data.vision.datasets.ImageRecordDataset), you can pass a `transform` function that will be applied to each image in the dataset, every time it's loaded from disk. Augmentations are intended to be random, so you'll pass a slightly different version of the image to the network on each epoch. | ||
|
||
When training models that require multiple passes through your data (multiple training epochs) | ||
|
||
We define `aug_transform` below to perform a selection of augmentation steps, and pass it to our dataset. It's worth noting that augmentations should only be applied to the training data (and not the test data), so you don't want to pass this augementation transform function to the testing dataset. | ||
|
||
[`mxnet.image.CreateAugmenter`](https://mxnet.incubator.apache.org/api/python/image/image.html?highlight=createaugmenter#mxnet.image.CreateAugmenter) is a useful function for creating a diverse set of augmentations at once. Despite the singular `CreateAugmenter`, this function actually returns a list of Augmenters. We can then loop through this list and apply each type of augmentation one after another. Although the parameters of `CreateAugmenter` are fixed, the random augmentations (such as `rand_mirror` and `brightness`) will be different each time `aug_transform` is called. | ||
|
||
|
||
```python | ||
def aug_transform(data, label): | ||
data = data.astype('float32')/255 | ||
augs = mx.image.CreateAugmenter(data_shape=(3, 300, 300), | ||
rand_crop=0.5, rand_mirror=True, inter_method=10, | ||
brightness=0.125, contrast=0.125, saturation=0.125, | ||
pca_noise=0.02) | ||
for aug in augs: | ||
data = aug(data) | ||
return data, label | ||
|
||
|
||
training_dataset = mx.gluon.data.vision.ImageFolderDataset('./data', transform=aug_transform) | ||
``` | ||
|
||
|
||
We can quickly inspect the augmentations using the `__getitem__` method of the dataset. When this method is called (with an index) the correct image is read from disk, and the `transform` is applied. We can see the result of the augmentations when comparing the image below with the original image above. | ||
|
||
|
||
```python | ||
sample = training_dataset.__getitem__(0)[0] | ||
plot_mx_array(sample*255) | ||
``` | ||
|
||
|
||
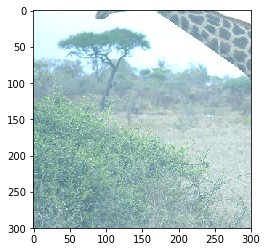 | ||
|
||
|
||
In practice you should load images from a dataset with a [`mxnet.gluon.data.DataLoader`](https://mxnet.incubator.apache.org/api/python/gluon/data.html?highlight=dataloader#mxnet.gluon.data.DataLoader) to take advantage of automatic batching and shuffling. Under the hood the `DataLoader` calls `__getitem__`, but you shouldn't need to call directly for anything other than debugging. Some practitioners pre-augment their datasets by applying a fixed number of augmentations to each image and saving the outputs to disk with the aim of increased throughput. With the `num_workers` parameter of `DataLoader` you can use all CPU cores to apply the augmentations, which often mitigates the need to perform pre-augmentation; reducing complexity and saving disk space. | ||
|
||
|
||
```python | ||
batch_size = 1 | ||
training_data_loader = mx.gluon.data.DataLoader(training_dataset, batch_size=1, shuffle=True) | ||
|
||
for data_batch, label_batch in training_data_loader: | ||
plot_mx_array(data_batch[0]*255) | ||
assert data_batch.shape == (1, 300, 300, 3) | ||
assert label_batch.shape == (1,) | ||
break | ||
``` | ||
|
||
|
||
 | ||
|
||
|
||
We will now dive into some of the lower level methods for image augmentation, which you will be able to use when writing your own `transform` functions. | ||
|
||
## Augmentation Functions | ||
|
||
MXNet provides a small number of augmentation functions that are quick and easy to use, but they are limited to positional augmentations (such as [`mxnet.image.random_crop`](https://mxnet.incubator.apache.org/api/python/image/image.html?highlight=random_crop#mxnet.image.random_crop) and [`mxnet.image.resize_short`](https://mxnet.incubator.apache.org/api/python/image/image.html?highlight=random_crop#mxnet.image.resize_short) functions) as opposed to color augmentations (such as brightness jitter). Although these functions are easy to apply, the [`mxnet.image.Augmenter`](https://mxnet.incubator.apache.org/api/python/image/image.html?highlight=augmen#mxnet.image.Augmenter) classes are much more comprehensive and just as easy to use, as we'll see in the next section. | ||
|
||
|
||
```python | ||
aug_image, crop_box = mx.image.random_crop(example_image, size=(100, 100)) | ||
plot_mx_array(aug_image) | ||
assert aug_image.shape == (100, 100, 3) | ||
``` | ||
|
||
|
||
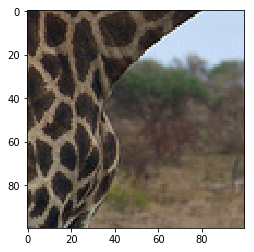 | ||
|
||
|
||
## Augmenter Classes | ||
|
||
You can apply a wide variety of positional and color augmentations with [`mxnet.image.Augmenter`](https://mxnet.incubator.apache.org/api/python/image/image.html?highlight=augmen#mxnet.image.Augmenter) classes, and using them is the recommended approach. After creating an instance of an Augmenter with the required parameters, you can call the Augmenter just as you would a function. Under the hood a `__call__` method is defined which applies the augmentation. Augmenters with randomness are randomized each time the Augmenter is called, so calling the same Augmenter twice will give different results on the same input. | ||
|
||
|
||
```python | ||
aug = mx.image.RandomCropAug(size=(100, 100)) | ||
aug_image = aug(example_image) | ||
plot_mx_array(aug_image) | ||
assert aug_image.shape == (100, 100, 3) | ||
``` | ||
|
||
|
||
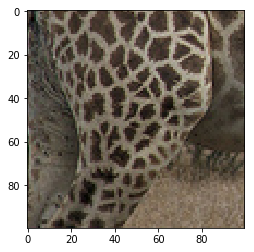 | ||
|
||
|
||
## Augmenter list | ||
|
||
Very often you'll want to apply many different types of augmentation to an image. Instead of nesting the calls of Augmenters, a natural structure for handling a large number of Augmenters is a list. You can construct this list manually, or you can use helper functions like [`mxnet.image.CreateAugmenter`](https://mxnet.incubator.apache.org/api/python/image/image.html?highlight=createaugmenter#mxnet.image.CreateAugmenter) to create these lists automatically. | ||
|
||
|
||
```python | ||
# created manually | ||
aug_list = [mx.image.RandomCropAug(size=(100, 100)), mx.image.BrightnessJitterAug(brightness=1)] | ||
aug_image = example_image.copy() | ||
for aug in aug_list: | ||
aug_image = aug(aug_image) | ||
plot_mx_array(aug_image) | ||
assert all([isinstance(a, mx.image.Augmenter) for a in aug_list]) | ||
``` | ||
|
||
|
||
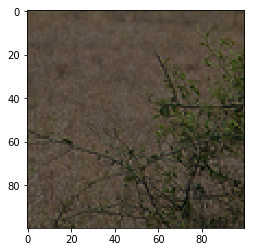 | ||
|
||
|
||
|
||
```python | ||
# created automatically | ||
aug_list = mx.image.CreateAugmenter(data_shape=(3, 300, 300), rand_crop=0.5, | ||
rand_mirror=True, mean=True, brightness=0.125, contrast=0.125, | ||
saturation=0.125, pca_noise=0.05, inter_method=10) | ||
aug_image = example_image.copy() | ||
for aug in aug_list: | ||
aug_image = aug(aug_image) | ||
plot_mx_array(aug_image) | ||
assert all([isinstance(a, mx.image.Augmenter) for a in aug_list]) | ||
``` | ||
|
||
|
||
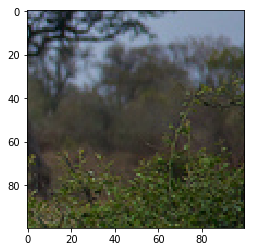 | ||
|
||
|
||
__*Watch Out!*__ Check some examples that are output after applying all the augmentations. You may find that the augmentation steps are too severe, and may actually prevent the model from learning. Some of the augmentation parameters used in this tutorial are set high for demostration purposes (e.g. `brightness=1`); you might want to reduce them if your training error stays too high during training. Some examples of excessive augmentation are shown below: | ||
|
||
<img src="https://raw.githubusercontent.com/dmlc/web-data/master/mxnet/doc/tutorials/data_aug/outputs/use//severe_aug.png" alt="Drawing" style="width: 700px;"/> | ||
|
||
## Optional: Augmentation with DataIters | ||
|
||
If you're still using the Module API you can apply augmentations directly using [`mxnet.image.ImageIter`](https://mxnet.incubator.apache.org/api/python/image/image.html?highlight=imageiter#mxnet.image.ImageIter) and [`mxnet.io.ImageRecordIter`](https://mxnet.incubator.apache.org/api/python/io/io.html?highlight=imagerecorditer#mxnet.io.ImageRecordIter). | ||
|
||
Using [`ImageIter`](https://mxnet.incubator.apache.org/api/python/image/image.html?highlight=imageiter#mxnet.image.ImageIter) (and [`ImageDetIter`](https://mxnet.incubator.apache.org/api/python/image/image.html?highlight=imagedetiter#mxnet.image.ImageDetIter) similarly) you can specify `aug_list` which expects a list of Augmenters. As in the section above, this list will be iterated though and the augmentations applied in turn. Conveniently you can also pass the arguments expected by [`CreateAugmenter`](https://mxnet.incubator.apache.org/api/python/image/image.html?highlight=createaugmenter#mxnet.image.CreateAugmenter) directly to the [`ImageIter`](https://mxnet.incubator.apache.org/api/python/image/image.html?highlight=imageiter#mxnet.image.ImageIter) constructor; they are picked up as keyword argumenets (`**kwargs`). If you are using [`ImageDetIter`](https://mxnet.incubator.apache.org/api/python/image/image.html?highlight=imagedetiter#mxnet.image.ImageDetIter) for object detection tasks, the keyword arguments are passed to [`CreateDetAugmenter`](https://mxnet.incubator.apache.org/api/python/image/image.html?highlight=createdetaugmenter#mxnet.image.CreateDetAugmenter) instead. Use [`tools/im2rec.py`](https://github.com/apache/incubator-mxnet/blob/master/tools/im2rec.py) to create the `images.lst` if you don't already have this for your data. | ||
|
||
```python | ||
!echo -e "0\t0.000000\timages/0.jpg" > ./data/images.lst | ||
``` | ||
|
||
```python | ||
training_iter = mx.image.ImageIter(batch_size = 1, | ||
data_shape = (3, 300, 300), | ||
path_root= './data', path_imglist='./data/images.lst', | ||
rand_crop=0.5, rand_mirror=True, inter_method=10, | ||
brightness=0.125, contrast=0.125, saturation=0.125, | ||
pca_noise=0.02 | ||
) | ||
``` | ||
|
||
|
||
```python | ||
for batch in training_iter: | ||
assert batch.data[0].shape == (1, 3, 300, 300) | ||
assert batch.label[0].shape == (1,) | ||
sample = batch.data[0][0].transpose(axes=[1,2,0]) | ||
plot_mx_array(sample) | ||
break | ||
``` | ||
|
||
|
||
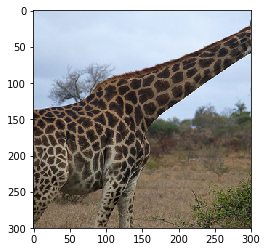 | ||
|
||
|
||
[`mxnet.io.ImageRecordIter`](https://mxnet.incubator.apache.org/api/python/io/io.html?highlight=imagerecorditer#mxnet.io.ImageRecordIter) has a slightly different interface, so reference the documentation [here](https://mxnet.incubator.apache.org/api/python/io/io.html?highlight=imagerecorditer#mxnet.io.ImageRecordIter) if you're using Record IO data format. |