-
-
Notifications
You must be signed in to change notification settings - Fork 719
New issue
Have a question about this project? Sign up for a free GitHub account to open an issue and contact its maintainers and the community.
By clicking “Sign up for GitHub”, you agree to our terms of service and privacy statement. We’ll occasionally send you account related emails.
Already on GitHub? Sign in to your account
Root-ish tasks all schedule onto one worker #6573
Comments
This is a work stealing problem. If we disable work stealing, it works as expected import time
import dask
import distributed
with dask.config.set({"distributed.scheduler.work-stealing": False}):
client = distributed.Client(n_workers=4, threads_per_worker=1)
root = dask.delayed(lambda n: "x" * n)(dask.utils.parse_bytes("1MiB"), dask_key_name="root")
results = [dask.delayed(lambda *args: None)(root, i) for i in range(10000)]
dask.compute(results) |
I looked into this briefly today and could narrow this down to a couple of issues
Whether or not unknown tasks are allowed to be stolen is actually a disputed topic. |
The initial imbalance is cause by work stealing being selecting potential victims greedily in case there are no saturated workers around. Specifically the following lines distributed/distributed/stealing.py Lines 424 to 429 in 99a2db1
Therefore, as soon as at least one of the workers is classified as idle due to being slightly faster than another worker, this idle worker is allowed to steal work from basically everywhere causing all work to gravitate towards this specific worker. Finally, this for-loop is exhausting the entire stealable set for the targeted victim/"saturated" worker without accounting for any in-flight occupancy. in-flight occupancy is used for sorting and therefore picking a victim (see |
I investigated what's causing the initial spike of stealing events. Very early in the computation we see that slightly less than 7.5k stealing decisions are enacted which causes this initial imbalance. Assuming perfect initial task placement, this is explained by the way we update occupancies. Right after the root task finished and all tasks are assigned we can see that the occupancies are already biased due to #7004 this bias is amplified in this example due to a double counting problem of occupancies #7003 after an update of the task duration, will reevaluate this, causing in this example the occupancy of the selected worker to drop dramatically since the unknown-duration is, by default 0.5s but the runtime of these tasks is much smaller. This drastic drop causes this worker to be classified as idle and it will steal all the tasks. Even if this round robin recalculation is replaced with an exact, "always compute all workers" function, the bias introduced by the double counting causes the worker with the dependency to always be classified as idle which causes heavy stealing as well xref #5243 Due to how we determine if keys are allowed to be stolen, this imbalance may never be corrected again, see distributed/distributed/stealing.py Lines 225 to 233 in 3655f13
In this specific case, this will never be rebalance again because of the fast compute time of the tasks |
The reproducer no longer works after #7036. import time
import dask
import distributed
client = distributed.Client(n_workers=4, threads_per_worker=1)
root = dask.delayed(lambda n: "x" * n)(dask.utils.parse_bytes("1MiB"), dask_key_name="root")
results = [dask.delayed(lambda *args: None)(root, i) for i in range(10000)]
r2 = dask.persist(results)
distributed.wait(r2)
for ws in client.cluster.scheduler.workers.values():
print(ws.address, len(ws.has_what)) Before #7036: After #7036: |
Initially a few
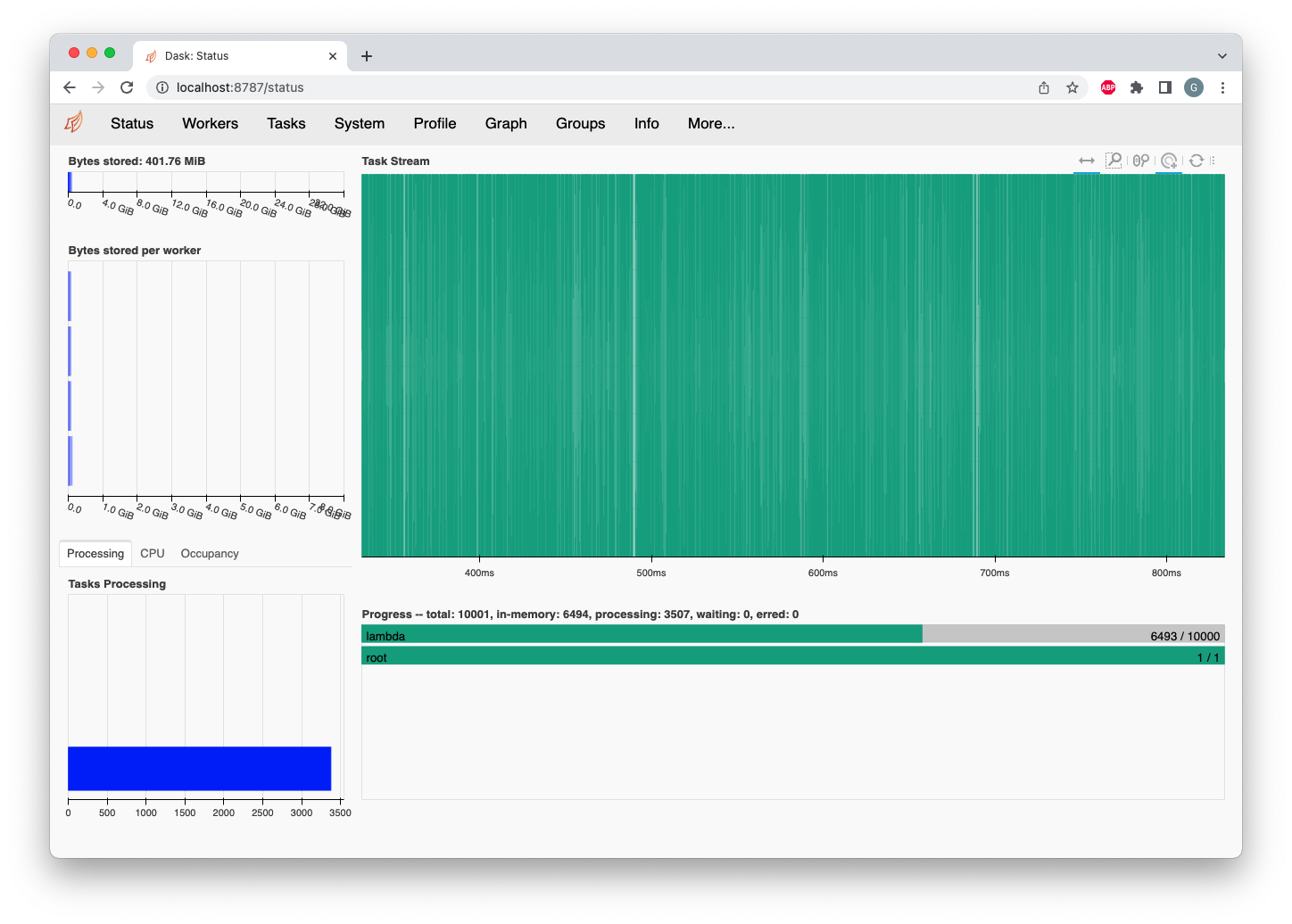
results
tasks run on other workers, but after about .5 sec, all tasks are just running on a single worker and the other three are idle.I would have expected these tasks to be evenly assigned to all workers up front
Some variables to play with:
dask_key_name="root"
, then all tasks (including the root) will all run on the same worker. I assume this is because they have similar same key names (lambda
) and therefore the same task group, and some scheduling heuristics are based not on graph structure but on naming heuristicsDistributed version: 2022.6.0
The text was updated successfully, but these errors were encountered: