-
Notifications
You must be signed in to change notification settings - Fork 2k
New issue
Have a question about this project? Sign up for a free GitHub account to open an issue and contact its maintainers and the community.
By clicking “Sign up for GitHub”, you agree to our terms of service and privacy statement. We’ll occasionally send you account related emails.
Already on GitHub? Sign in to your account
Adding multi gpu support for DPR inference #1414
Conversation
Hi @MichelBartels thank you very much for your contribution and especially for providing the speed comparison with 1 GPU vs. 2 GPUs. We will need some time to think about and discuss your proposed changes to |
Hey @MichelBartels , Thanks a lot for working on this! Let me address your questions:
|
I have now added the suggested changes. I have also added a warning in case the batch size is less than the device size. |
The check seems to fail because https://github.com/deepset-ai/haystack/blob/master/test/test_retriever.py#L199 seems to keep the default parameter of use_gpu (true). The test server, however, does not seem to have gpu support. Usually, initialize_device_settings switches to CPU silently in this case (https://github.com/deepset-ai/haystack/blob/master/test/test_retriever.py#L199). Should I also implement this? Or is a warning/crash expected when use_gpu is set to true without a gpu available? |
Yep, the behaviour on a CPU machine should be to silently "fallback" to CPU even when |
I have now also implemented the fallback. I think you can do your thorough review now. |
There was a problem hiding this comment.
Choose a reason for hiding this comment
The reason will be displayed to describe this comment to others. Learn more.
Hey @MichelBartels ,
This is all looking good to me. I only added minor updates to docstring, replaced the deprecated logger.warn()
and made sure that the supplied devices
values are also stores within the object config (important for saving/loading).
I think this is a pragmatic, first implementation of multi-gpu support.
Later on, we should probably rethink some implementation details (see comment) and potentially support other options like DDP, TPUs or similar. HF's accelearte lib might be interesting to keep in mind here.
=> Ready-to-merge. Congrats to your first contribution 🎉
self.devices = [torch.device(device) for device in range(torch.cuda.device_count())] | ||
else: | ||
self.devices = [torch.device("cpu")] | ||
|
There was a problem hiding this comment.
Choose a reason for hiding this comment
The reason will be displayed to describe this comment to others. Learn more.
As we already have a helper method for device resolution (initialize_device_settings()), it might make sense to rather adjust this method and use it then everywhere within haystack than implementing it now here in a custom way. However, as this method is currently part of FARM and we are just in the middle of migrating FARM to Haystack, let's not complicate things and move forward with the custom code here. (FYI @Timoeller )
There was a problem hiding this comment.
Choose a reason for hiding this comment
The reason will be displayed to describe this comment to others. Learn more.
here is the link to the function - lets use this once the FARM migration PR is merged
Proposed changes:
Status (please check what you already did):
This is an initial draft, which has a raised a few questions for me:
I have added one optional parameter to DPR's constructor called devices. This takes a list of torch.devices or integer/string identifiers of gpus specifying the gpus used for inference. This is, however, a bit counterintuitive as, despite its name, only cpu devices are invalid and whether or not to use a gpu is specified with another parameter. Also, usually the specific devices don't matter as normally only one gpu type is present. To address this, I have multiple ideas:
I am not sure which of these options is the best. (Or if there is better option.)
The existing parameter batch_size has an ambiguous meaning when using multiple gpus. It could either mean per device batch size or total batch size. It should either be defined or per_device_batch_size and/or total_batch_size could be added as parameters.
Benchmark:
I have extended the benchmark_index method, because it seems like adding another function would create a lot of overlap. However, this seems to further complicate the function by introducing two additional nested loops (number of gpus and batch size). Should I perhaps split the benchmark in two and refactor the benchmark_index method so its functionality can be reused? Also, similarly to the question above, should the benchmark parameters specify the specific gpu devices or just the number of gpus?
Performance:
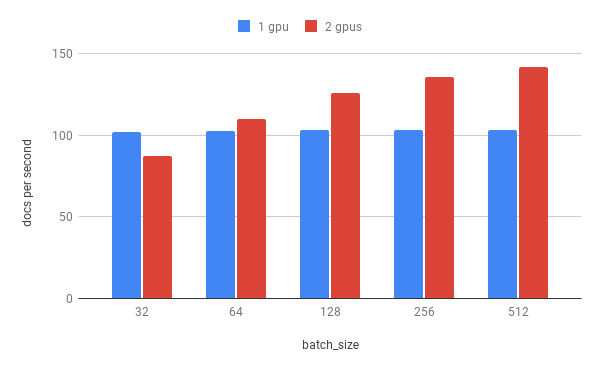
torch.nn.DataParallel seems to add a lot of overhead. This means that for small batch sizes using two gpus is slower than using one. However, with a batch size of 512 I could measure a performance improvement of 37.6% using two RTX 3060. This is probably higher with more VRAM.