-
Notifications
You must be signed in to change notification settings - Fork 19
Novel miRNA prediction
Novel miRNAs in a species are generally those for which the mature sequence is not present in miRBase. This may be because the species has not been well studied or insufficient evidence is available to consider a sequence a real miRNA. There are problems with false positives in general for miRNA discovery from deep sequencing and this should be kept in mind when running an analysis of your reads. It is advised to use at least two algorithms for such an analysis. Most of the higher abundance miRNAs have been identified in the commonly studied species. Detection/prediction of new mirs will therefore involve looking at the low abundance (or tissue specific) forms which will need further evidence such as conservation, experimental verification and perhaps identification of function.
There are by now multiple algorithms available for predicting novel miRNAs from small RNA sequencing data. The most popular is probably miRDeep2. This algorithm is accessible via the mirdeep2 module assuming you have installed mirdeep2 as well. However we include a native algorithm in smallrnaseq for novel prediction, this is described below.
The built-in method for novel prediction should be considered a somewhat 'quick and dirty' method at present but is relatively fast and convenient to use. The basic idea is to take clusters of reads that could be mature sequence and find suitable precursors. Structural features of each precursor are then scored using a classifier. The best candidate is selected is there is at least one. We have followed a similar approach to the miRanalyzer method.
The following features are currently used in our algorithm, most are the same as those used in sRNAbench (miRanalyzer). The diagram below may help to clarify some of the terminology used.
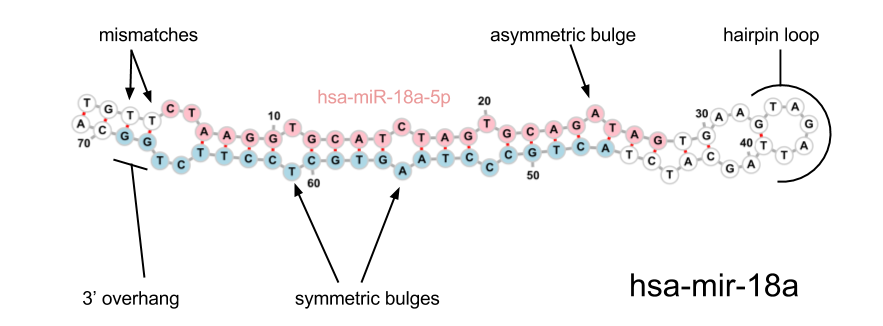
Feature | Description |
---|---|
Length | The length of the longest hairpin structure |
Stem length | The length of the longest hairpin structure stem |
Mfe | The mean free energy of the hairpin |
Loop length | The number of bases in the loop of the hairpin |
Loop GC | The GC-content of the loop |
GC | The GC-content of the small hairpin |
Asymmetric bulges | The number of asymmetric bulges and mismatches in the stem |
Symmetric bulges | The number of symmetric bulges and mismatches in the stem |
Bulges | The number of bulges in the stem |
Longest bulge | The number of non-pairing nucleotides of the longest bulge |
Hairpin mismatches | The number of single mismatches in the hairpin |
Mature mismatches | The number of single mismatches in the mature microRNA region of the hairpin |
Triplet-SVM features | All features that were proposed by Xue et al. |
The basic steps for novel precursor detection are as follows.
- if multiple samples, read counts for all samples are put together using each sam file and total counts for each unique read (after collapsing the original fastq files)
- the reads are sorted by read count
- reads are clustered using cluster trees
- read clusters are themselves clustered to detect pairs within 100 nt (for animals). these are considered to be possible mature/star arms and checked for hairpin structure
- single clusters are checked for precursors by creating multiple possible precursors at the 5' and 3' ends and evaluating the features
- a precursor is discarded if:
- it has no hairpin
- its read cluster overlaps with the hairpin loop
- it has less than 19 bindings in the stem
- it has less than 11 bindings to the region occupied by the read cluster
- a random forest classifier/regressor trained on known positives and negatives is used to score the precursor features
- the precursor with the lowest energy and highest score is used as the most likely candidate
- for single clusters this region is considered the mature arm and the star sequence is estimated
- the list of novel miRNAs with precursor, mature, star, reads is output
Using the command line tool it is simply a matter of setting novel = 1
in the config file. Reads will first be mapped to mirbase to remove known miRNAs and also any libraries you included in the indexes option.
The novel prediction step from the command line tool will produce a file called novel_mirna.csv
in the output folder.
from smallrnaseq import novel
import pandas as pd
#single file prediction
readcounts = pd.read_csv('countsfile.csv')
samfile = 'mysamfile.sam'
reads = utils.get_aligned_reads(samfile, readcounts)
new = novel.find_mirnas(reads, ref_fasta)
- Kang, W. & Friedländer, M.R., 2015. Computational Prediction of miRNA Genes from Small RNA Sequencing Data. Frontiers in Bioengineering and Biotechnology, 3, p.7.
- Hackenberg, M. et al., 2009. miRanalyzer: a microRNA detection and analysis tool for next-generation sequencing experiments. Nucleic Acids Research, 37(Web Server), pp.W68–W76.
- Friedländer, M.R. et al., 2012. miRDeep2 accurately identifies known and hundreds of novel microRNA genes in seven animal clades. Nucleic acids research, 40(1), pp.37–52.
- Shi, J. et al., 2015. mirPRo–a novel standalone program for differential expression and variation analysis of miRNAs. Scientific Reports, 5, p.14617.
- Xue, C. et al., 2005. Classification of real and pseudo microRNA precursors using local structure-sequence features and support vector machine. BMC bioinformatics, 6, p.310.
- Lopes, I.D.O.N. et al., 2014. The discriminant power of RNA features for pre-miRNA recognition. BMC bioinformatics, 15(1), p.124.