-
Notifications
You must be signed in to change notification settings - Fork 783
Commit
This commit does not belong to any branch on this repository, and may belong to a fork outside of the repository.
Browse files
Browse the repository at this point in the history
- Loading branch information
Showing
2 changed files
with
219 additions
and
0 deletions.
There are no files selected for viewing
This file contains bidirectional Unicode text that may be interpreted or compiled differently than what appears below. To review, open the file in an editor that reveals hidden Unicode characters.
Learn more about bidirectional Unicode characters
This file contains bidirectional Unicode text that may be interpreted or compiled differently than what appears below. To review, open the file in an editor that reveals hidden Unicode characters.
Learn more about bidirectional Unicode characters
Original file line number | Diff line number | Diff line change |
---|---|---|
@@ -0,0 +1,206 @@ | ||
--- | ||
title: "使用 BentoML 部署 🤗 Hugging Face 上的模型:DeepFloyd IF 实战" | ||
thumbnail: /blog/assets/deploy-deepfloydif-using-bentoml/thumbnail.png | ||
authors: | ||
- user: Sherlockk | ||
guest: true | ||
- user: larme | ||
guest: true | ||
translators: | ||
- user: Sherlockk | ||
--- | ||
|
||
# 使用 BentoML 部署 🤗 Hugging Face 上的模型:DeepFloyd IF 实战 | ||
|
||
<!-- {blog_metadata} --> | ||
<!-- {authors} --> | ||
|
||
Hugging Face 的 Model Hub 可以让我们轻松地上传、分享和部署模型,为开发者们节省了从头开始训练模型所需的时间和计算资源。然而,在真实世界的生产环境中或以云原生的方式部署模型则仍然可能带来挑战。 | ||
|
||
在这一方面,不妨试试 BentoML。BentoML 是一个用于机器学习(ML)模型服务和部署的开源平台,提供了统一的框架,以构建、传输和扩展各类生产就绪的 AI 应用程序,支持传统、预训练、生成式以及大语言模型等各类模型。BentoML 框架的运作原理大致如下: | ||
|
||
1. **定义模型**:使用 BentoML 之前,需要准备一个或多个机器学习模型。模型可以使用 TensorFlow 和 PyTorch 等机器学习库进行训练。 | ||
1. **保存模型**:模型训练后,将其保存到 BentoML 的本地模型仓库(Model Store)中,这样便于管理所有本地的模型,之后可随时访问并提供服务。 | ||
1. **创建 BentoML 服务**: 创建 service.py 文件来包装模型并定义服务逻辑。[服务](https://docs.bentoml.org/en/latest/concepts/service.html) 中会为模型定义 [Runner](https://docs.bentoml.org/en/latest/concepts/runner.html) 以便于大规模的模型推断,并暴露对外 API,定义输入和输出的处理逻辑。 | ||
4. **构建 Bento**: 通过创建一个 YAML 配置文件(`bentofile.yaml`),将所有模型和服务打包成一个可部署的制品,即 [Bento](https://docs.bentoml.org/en/latest/concepts/bento.html),其中包含所有代码和依赖文件等。 | ||
5. **部署 Bento**:Bento 准备好后,可以将 Bento 容器化以创建 Docker 镜像并在 K8s 上运行。或者,直接将 Bento 部署到 BentoCloud 或 Yatai,以便于在 K8s 上自动化部署流程,进行大规模运行。 | ||
|
||
在这篇博客文章中,我们将展示如何按照上述工作流程来通过 BentoML 运行 [DeepFloyd IF](https://huggingface.co/docs/diffusers/api/pipelines/if)。 | ||
|
||
## DeepFloyd IF 简介 | ||
|
||
DeepFloyd IF 是一个开源的文本到图像生成式模型。其独特的运行机制和架构让它与 Stable Diffusion 等潜在扩散模型(Latent Diffusion Model)区别开来。 | ||
|
||
DeepFloyd IF 提供了高度的照片真实性,能理解复杂的语言。与 Stable Diffusion 不同,DeepFloyd IF 工作时直接作用于像素维度,其模块化的结构包括一个文本编码器和三个级联像素扩散模块。每个模块在过程中都起到独特的作用:第一阶段负责创建一个基本的 64x64 像素图像,然后在第二和第三阶段逐步放大到 1024x1024 像素。DeepFloyd IF 独特性的另一个关键因素是其集成了大语言模型(T5-XXL-1.1)来编码提示词,这让它对复杂的提示词也能有良好的理解能力。更多信息请参阅 [Stability AI 这篇关于 DeepFloyd IF 的博客文章](https://stability.ai/blog/deepfloyd-if-text-to-image-model)。 | ||
|
||
为了确保基于 DeepFloyd IF 的应用程序在生产中能高性能地运行,你可能需要巧妙地去分配和管理资源。在这方面,BentoML 支持独立地为每个阶段去扩展 Runner。例如,你可以为第一阶段的 Runner 使用更多的 Pod 或为它们分配性能更强的 GPU 服务器。 | ||
|
||
## 准备环境 | ||
|
||
[此 GitHub 仓库](https://github.com/bentoml/IF-multi-GPUs-demo) 中存储了此项目的所有文件。要在本地运行此项目,请确保先满足以下条件: | ||
|
||
- 已安装 Python 3.8 及以上版本 | ||
- 已安装 `pip` | ||
- 安装机器至少具备 2x16 GB VRAM GPU 或 1x40 VRAM GPU。对于此项目,我们使用了 Google Cloud 的 `n1-standard-16` 机型,加上 64 GB 的 RAM 和 2 个 NVIDIA T4 GPU。请注意,虽然可以在单个 T4 上运行 DeepFloyd IF,但不建议用于生产级服务。 | ||
|
||
满足条件后,将项目仓库克隆到本地机器并进入项目目录。 | ||
|
||
```bash | ||
git clone https://github.com/bentoml/IF-multi-GPUs-demo.git | ||
cd IF-multi-GPUs-demo | ||
``` | ||
|
||
在构建应用程序前,我们先简要浏览一下此目录中的主要文件: | ||
|
||
- `import_models.py`: 指定 [`IFPipeline`](https://huggingface.co/docs/diffusers/api/pipelines/if) 中每个阶段的模型。此文件将所有模型下载到本地,以便将它们打包成一个 Bento; | ||
- `requirements.txt`: 定义此项目所需的所有包和依赖; | ||
- `service.py`: 定义 BentoML 服务,该服务使用 `to_runner` 创建三个 Runner,并暴露一个用于生成图像的 API。该 API 输入为 JSON 对象(即提示词和相反提示词),在使用一系列模型后输出图像; | ||
- `start-server.py`: 通过在 `service.py` 中定义的服务启动一个 BentoML HTTP 服务器,并创建一个 Gradio Web UI,用于输入提示词生成图像; | ||
- `bentofile.yaml`: 定义要构建的 Bento 的元数据,包括服务、Python 包和模型等。 | ||
|
||
建议创建一个虚拟环境用于依赖项隔离。例如,运行以下命令激活 `myenv`: | ||
|
||
```bash | ||
python -m venv venv | ||
source venv/bin/activate | ||
``` | ||
|
||
安装所需的依赖项: | ||
|
||
```bash | ||
pip install -r requirements.txt | ||
``` | ||
|
||
如果之前没有使用过命令行从 Hugging Face 下载模型,你必须先登录: | ||
|
||
```bash | ||
pip install -U huggingface_hub | ||
huggingface-cli login | ||
``` | ||
|
||
## 下载模型到 BentoML 模型仓库 | ||
|
||
如上所述,你需要下载每个 DeepFloyd IF 阶段所使用的模型。设置好环境后,运行以下命令将模型下载到你的本地模型仓库,该过程可能需要一些时间。 | ||
|
||
```bash | ||
python import_models.py | ||
``` | ||
|
||
下载完成后,查看模型仓库中的模型。 | ||
|
||
```bash | ||
$ bentoml models list | ||
|
||
Tag Module Size Creation Time | ||
sd-upscaler:bb2ckpa3uoypynry bentoml.diffusers 16.29 GiB 2023-07-06 10:15:53 | ||
if-stage2:v1.0 bentoml.diffusers 13.63 GiB 2023-07-06 09:55:49 | ||
if-stage1:v1.0 bentoml.diffusers 19.33 GiB 2023-07-06 09:37:59 | ||
``` | ||
|
||
## 启动 BentoML 服务 | ||
|
||
可以直接使用 `start-server.py` 文件启动 BentoML HTTP 服务器,运行 Gradio Web UI,这也是该应用程序的入口。可以通过各种选项来自定义运行环境,管理不同阶段的 GPU 资源分配。根据你的 GPU 设置,你可能会使用不同的命令: | ||
|
||
- 对于具有超过 40 GB VRAM 的 GPU,可在同一 GPU 上运行所有模型: | ||
|
||
```bash | ||
python start-server.py | ||
``` | ||
|
||
- 如果有两个 Tesla T4 且每个具有 15 GB VRAM,可将第一个 GPU 分配给第一阶段模型,将第二个 GPU 分配给第二和第三阶段模型: | ||
|
||
```bash | ||
python start-server.py --stage1-gpu=0 --stage2-gpu=1 --stage3-gpu=1 | ||
``` | ||
|
||
- 如果一个 Tesla T4 具有 15 GB VRAM 而另两个 GPU 的 VRAM 较小,可将 T4 分配给第一阶段模型,第二和第三个 GPU 分别分配给第二和第三阶段模型: | ||
|
||
```bash | ||
python start-server.py --stage1-gpu=0 --stage2-gpu=1 --stage3-gpu=2 | ||
``` | ||
|
||
要查看所有可自定义的选项(如服务器的端口),可运行以下命令: | ||
|
||
```bash | ||
python start-server.py --help | ||
``` | ||
|
||
## 测试服务器 | ||
|
||
服务器启动后,可以通过 http://localhost:7860 访问该 Web UI。 | ||
BentoML API Endpoint 也可在 http://localhost:3000 访问。以下是提示词和相反提示词示例。 | ||
|
||
提示词(Prompt): | ||
|
||
> orange and black, head shot of a woman standing under street lights, dark theme, Frank Miller, cinema, ultra realistic, ambiance, insanely detailed and intricate, hyper realistic, 8k resolution, photorealistic, highly textured, intricate details | ||
相反提示词(Negative Prompt): | ||
|
||
> tiling, poorly drawn hands, poorly drawn feet, poorly drawn face, out of frame, mutation, mutated, extra limbs, extra legs, extra arms, disfigured, deformed, cross-eye, body out of frame, blurry, bad art, bad anatomy, blurred, text, watermark, grainy | ||
输出结果: | ||
|
||
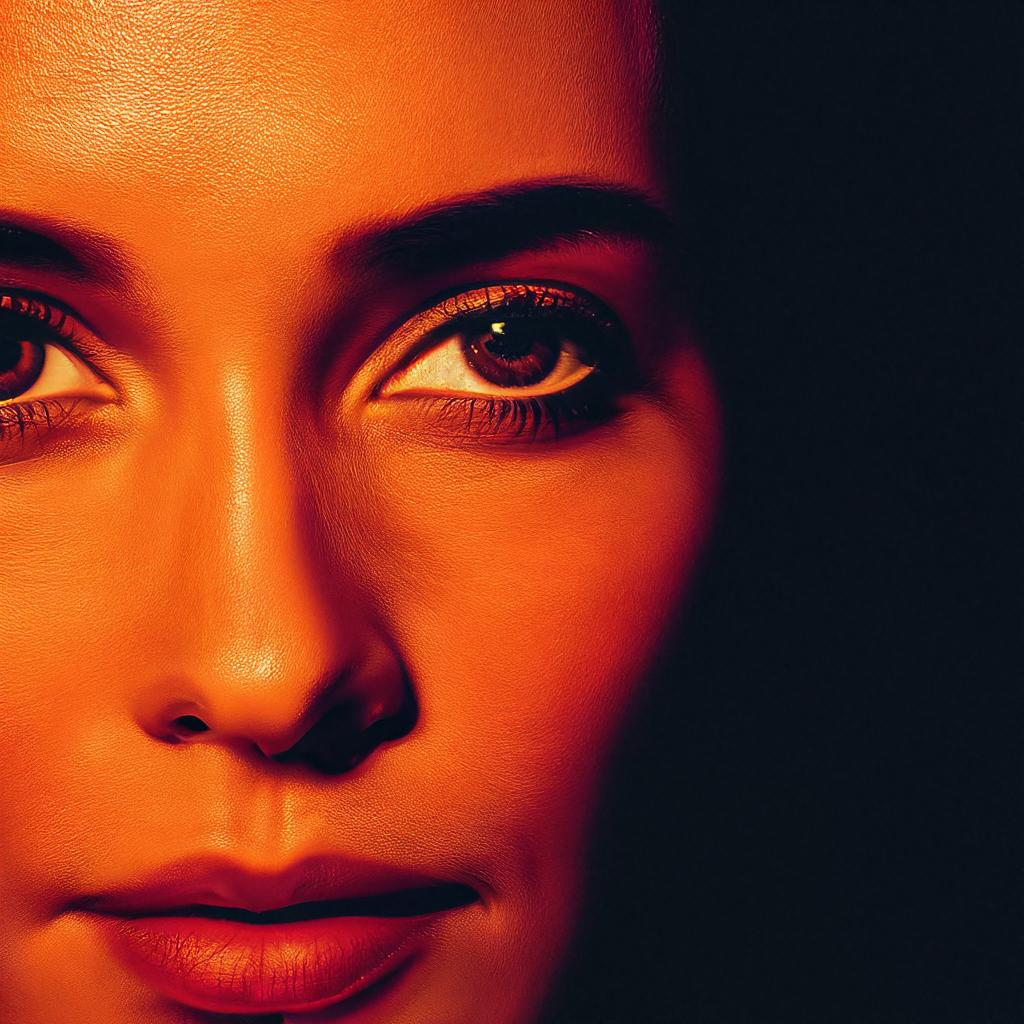 | ||
|
||
## 构建 Bento 并提供服务 | ||
|
||
成功在本地运行 DeepFloyd IF 后,可在项目目录下运行以下命令将其打包成 Bento。 | ||
|
||
```bash | ||
$ bentoml build | ||
|
||
Converting 'IF-stage1' to lowercase: 'if-stage1'. | ||
Converting 'IF-stage2' to lowercase: 'if-stage2'. | ||
Converting DeepFloyd-IF to lowercase: deepfloyd-if. | ||
Building BentoML service "deepfloyd-if:6ufnybq3vwszgnry" from build context "/Users/xxx/Documents/github/IF-multi-GPUs-demo". | ||
Packing model "sd-upscaler:bb2ckpa3uoypynry" | ||
Packing model "if-stage1:v1.0" | ||
Packing model "if-stage2:v1.0" | ||
Locking PyPI package versions. | ||
|
||
██████╗░███████╗███╗░░██╗████████╗░█████╗░███╗░░░███╗██╗░░░░░ | ||
██╔══██╗██╔════╝████╗░██║╚══██╔══╝██╔══██╗████╗░████║██║░░░░░ | ||
██████╦╝█████╗░░██╔██╗██║░░░██║░░░██║░░██║██╔████╔██║██║░░░░░ | ||
██╔══██╗██╔══╝░░██║╚████║░░░██║░░░██║░░██║██║╚██╔╝██║██║░░░░░ | ||
██████╦╝███████╗██║░╚███║░░░██║░░░╚█████╔╝██║░╚═╝░██║███████╗ | ||
╚═════╝░╚══════╝╚═╝░░╚══╝░░░╚═╝░░░░╚════╝░╚═╝░░░░░╚═╝╚══════╝ | ||
|
||
Successfully built Bento(tag="deepfloyd-if:6ufnybq3vwszgnry"). | ||
``` | ||
|
||
查看本地 Bento 仓库中的 Bento: | ||
|
||
```bash | ||
$ bentoml list | ||
|
||
Tag Size Creation Time | ||
deepfloyd-if:6ufnybq3vwszgnry 49.25 GiB 2023-07-06 11:34:52 | ||
``` | ||
|
||
可以在生产环境中使用该 Bento 提供服务: | ||
|
||
```bash | ||
bentoml serve deepfloyd-if:6ufnybq3vwszgnry | ||
``` | ||
|
||
要以更云原生的方式部署 Bento,运行以下命令生成 Docker 镜像: | ||
|
||
```bash | ||
bentoml containerize deepfloyd-if:6ufnybq3vwszgnry | ||
``` | ||
|
||
之后可以在 K8s 上部署该模型。 | ||
|
||
## 下一步计划 | ||
|
||
[BentoML](https://github.com/bentoml/BentoML) 为生产部署 Hugging Face 模型提供了高效且简单的方法。它支持一系列 ML 框架,提供易于使用的 API,可以在短时间内将模型部署到生产环境。无论是 DeepFloyd IF 还是 Hugging Face Model Hub 上的任何其他模型,BentoML 都可以帮助快速部署模型到生产环境。 | ||
|
||
感兴趣的朋友可以查看以下资源,了解 BentoML 及其生态系统,关注有关 BentoML 的更多信息。 | ||
|
||
- [OpenLLM](https://github.com/bentoml/OpenLLM) - 在生产环境中运行和管理大语言模型的开源平台; | ||
- [StableDiffusion](https://github.com/bentoml/stable-diffusion-bentoml) - 在生产环境中运行和管理扩散模型的开源平台; | ||
- [Transformer NLP Service](https://github.com/bentoml/transformers-nlp-service) - Transformer NLP 模型的在线推理 API; | ||
- 加入 [BentoML 在 Slack 上的线上社区](https://l.bentoml.com/join-slack); | ||
- 在 [Twitter](https://twitter.com/bentomlai) 和 [LinkedIn](https://www.linkedin.com/company/bentoml/) 关注 BentoML | ||
|
||
本文(中文版)[原载](https://mp.weixin.qq.com/s/I1vZhAqZocQELAiLmj2aJQ) 于公众号「语言挖掘师」,为本文英文原文作者、本文译者的内容发布平台。 |