-
Notifications
You must be signed in to change notification settings - Fork 533
New issue
Have a question about this project? Sign up for a free GitHub account to open an issue and contact its maintainers and the community.
By clicking “Sign up for GitHub”, you agree to our terms of service and privacy statement. We’ll occasionally send you account related emails.
Already on GitHub? Sign in to your account
ARIMA: pre-allocation of temporary memory to reduce latencies #3895
Conversation
… and add alpha to doxygen
There was a problem hiding this comment.
Choose a reason for hiding this comment
The reason will be displayed to describe this comment to others. Learn more.
Hi @Nyrio, thanks for this PR! I am halfway through the review, I will share what I have now.
Overall it looks good, I have mostly smaller comments. I see a potential issue with the lifetime management of the ARIMAMemory
, but that can be easily fixed.
I hope to finish reviewing rest later today.
There was a problem hiding this comment.
Choose a reason for hiding this comment
The reason will be displayed to describe this comment to others. Learn more.
Hi Louis, I have finished my review. I just have a few additional questions, overall it looks great.
There was a problem hiding this comment.
Choose a reason for hiding this comment
The reason will be displayed to describe this comment to others. Learn more.
Thanks Louis for the updates, the PR looks good to me!
@rapidsai/cuml-python-codeowners can you review this PR? |
rerun tests |
Codecov Report
@@ Coverage Diff @@
## branch-21.06 #3895 +/- ##
===============================================
Coverage ? 85.44%
===============================================
Files ? 226
Lines ? 17306
Branches ? 0
===============================================
Hits ? 14787
Misses ? 2519
Partials ? 0
Flags with carried forward coverage won't be shown. Click here to find out more. Continue to review full report at Codecov.
|
@Nyrio it is a bit hard to find, but from the log the error is a small doxygen issue: Generating /workspace/cpp/include/cuml/tsa/arima_common.h:342: error: argument 'in_buff' of command @param is not found in the argument list of ML::ARIMAMemory< T, ALIGN >::ARIMAMemory(const ARIMAOrder &order, int batch_size, int n_obs, char *in_buf) (warning treated as error, aborting now) |
@gpucibot merge |
…ai#3895) This PR can speed up the evaluation of the log-likelihood in ARIMA by 5x for non-seasonal datasets (the impact is smaller for seasonal datasets). It achieves this by pre-allocating all the temporary memory only once instead of every iteration and providing all the pointers with a very low overhead thanks to a dedicated structure. Additionally, I removed some unnecessary copies. 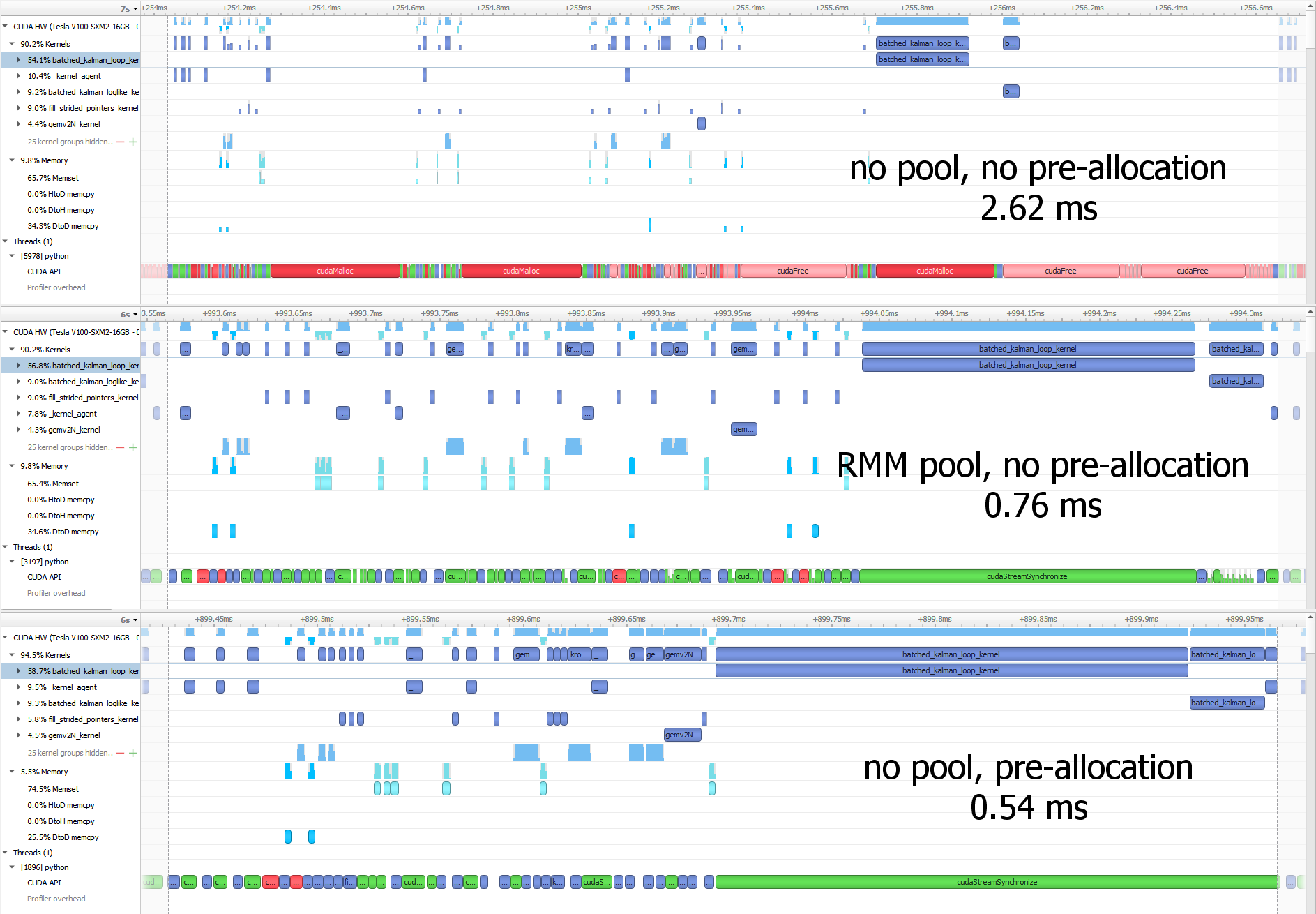 Regarding the unnecessary synchronizations, I'll fix that later in a separate PR. Note that non-seasonal ARIMA performance is now even more limited by the python-side solver bottleneck: 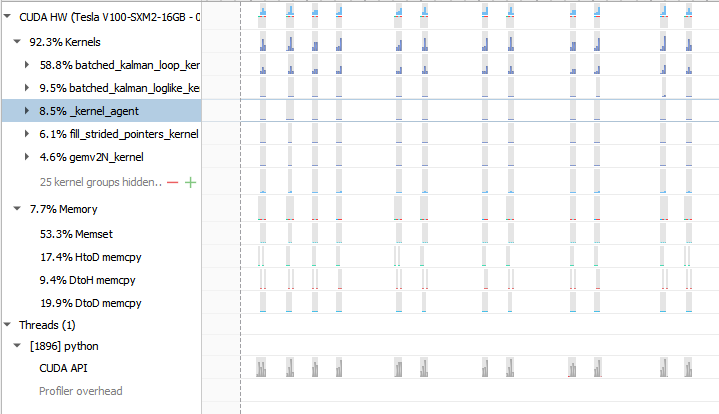 One problem is that batched matrix operations are quite memory-hungry so I've duplicated or refactored some bits to avoid allocating extra memory there, but that leads to some duplication that I'm not entirely happy with. Both the ARIMA code and batched matrix prims are due some refactoring. Authors: - Louis Sugy (https://github.com/Nyrio) Approvers: - Tamas Bela Feher (https://github.com/tfeher) - Dante Gama Dessavre (https://github.com/dantegd) URL: rapidsai#3895
This PR can speed up the evaluation of the log-likelihood in ARIMA by 5x for non-seasonal datasets (the impact is smaller for seasonal datasets). It achieves this by pre-allocating all the temporary memory only once instead of every iteration and providing all the pointers with a very low overhead thanks to a dedicated structure. Additionally, I removed some unnecessary copies.
Regarding the unnecessary synchronizations, I'll fix that later in a separate PR. Note that non-seasonal ARIMA performance is now even more limited by the python-side solver bottleneck:
One problem is that batched matrix operations are quite memory-hungry so I've duplicated or refactored some bits to avoid allocating extra memory there, but that leads to some duplication that I'm not entirely happy with. Both the ARIMA code and batched matrix prims are due some refactoring.