-
Notifications
You must be signed in to change notification settings - Fork 1
Commit
This commit does not belong to any branch on this repository, and may belong to a fork outside of the repository.
Merge pull request #7 from valentingol/features
✨ Add features: gradient backward, whitening and randomized svd + benchmark with sklearn
- Loading branch information
Showing
12 changed files
with
664 additions
and
62 deletions.
There are no files selected for viewing
This file contains bidirectional Unicode text that may be interpreted or compiled differently than what appears below. To review, open the file in an editor that reveals hidden Unicode characters.
Learn more about bidirectional Unicode characters
This file contains bidirectional Unicode text that may be interpreted or compiled differently than what appears below. To review, open the file in an editor that reveals hidden Unicode characters.
Learn more about bidirectional Unicode characters
Original file line number | Diff line number | Diff line change |
---|---|---|
@@ -0,0 +1,63 @@ | ||
"""Comparison between sklearn and torch PCA models.""" | ||
|
||
# Copyright (c) 2024 Valentin Goldité. All Rights Reserved. | ||
|
||
from time import time | ||
|
||
# NOTE: requires matplotlib (not in requirements(-dev).txt) | ||
import matplotlib.pyplot as plt | ||
import numpy as np | ||
import torch | ||
from sklearn.decomposition import PCA as PCA_sklearn | ||
|
||
from torch_pca import PCA | ||
|
||
|
||
def main() -> None: | ||
"""Measure and compare the time of execution of the PCA.""" | ||
configs = [(75, 75), (100, 2000), (10_000, 500)] | ||
torch_times, sklearn_times = [], [] | ||
for config in configs: | ||
inputs = torch.randn(*config) | ||
t0 = time() | ||
PCA(n_components=50).fit_transform(inputs) | ||
torch_times.append(round(time() - t0, 4)) | ||
t0 = time() | ||
PCA_sklearn(n_components=50).fit_transform(inputs) | ||
sklearn_times.append(round(time() - t0, 4)) | ||
ticks = np.arange(len(configs)) | ||
labels = [f"n_samples={config[0]}, n_features={config[1]}" for config in configs] | ||
width = 0.35 | ||
fig, ax = plt.subplots() | ||
rects1 = ax.bar(ticks - width / 2, torch_times, width, label="Pytorch PCA") | ||
rects2 = ax.bar(ticks + width / 2, sklearn_times, width, label="Sklearn PCA") | ||
ax.set_ylabel("Time of execution (s)") | ||
ax.set_title("Comparison of execution time between Pytorch and Sklearn PCA.") | ||
ax.set_xticks(ticks) | ||
ax.set_xticklabels(labels) | ||
ax.legend() | ||
autolabel(rects1, ax) | ||
autolabel(rects2, ax) | ||
fig.tight_layout() | ||
plt.show() | ||
|
||
|
||
def autolabel(rects: list, ax: plt.Axes) -> None: | ||
"""Attach a text label above each bar in *rects*, displaying its height. | ||
From https://matplotlib.org/3.1.1/gallery/lines_bars_and_markers/barchart.html | ||
""" | ||
for rect in rects: | ||
height = rect.get_height() | ||
ax.annotate( | ||
str(height), | ||
xy=(rect.get_x() + rect.get_width() / 2, height), | ||
xytext=(0, 3), # 3 points vertical offset | ||
textcoords="offset points", | ||
ha="center", | ||
va="bottom", | ||
) | ||
|
||
|
||
if __name__ == "__main__": | ||
main() |
Loading
Sorry, something went wrong. Reload?
Sorry, we cannot display this file.
Sorry, this file is invalid so it cannot be displayed.
This file contains bidirectional Unicode text that may be interpreted or compiled differently than what appears below. To review, open the file in an editor that reveals hidden Unicode characters.
Learn more about bidirectional Unicode characters
Original file line number | Diff line number | Diff line change |
---|---|---|
@@ -0,0 +1,6 @@ | ||
# Comparison of execution time with sklearn's PCA | ||
|
||
As we can see below the PyTorch PCA is faster than sklearn's PCA, in all the | ||
configs tested with the parameter by default (for each PCA model): | ||
|
||
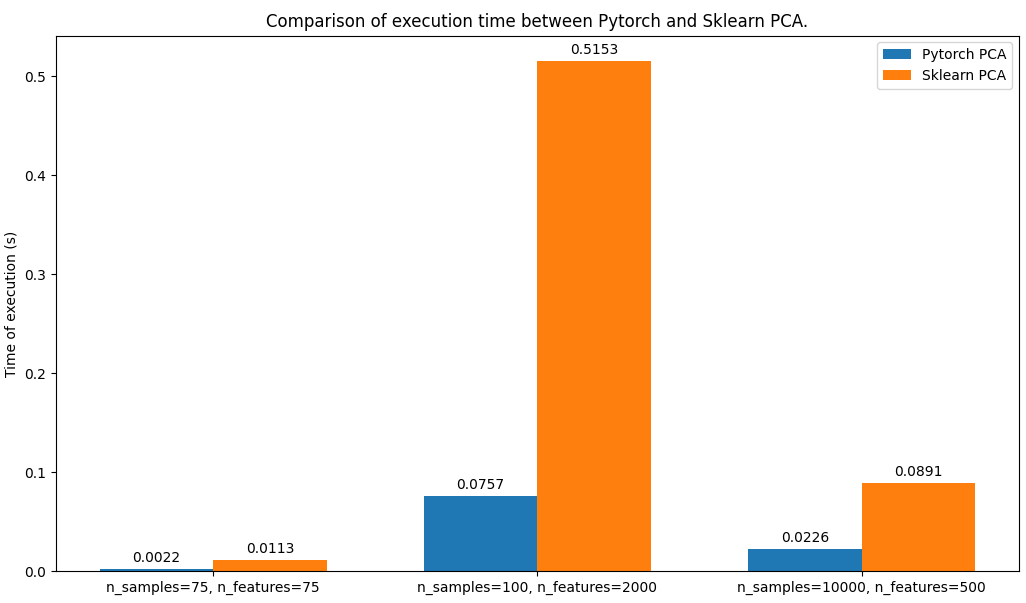 |
This file contains bidirectional Unicode text that may be interpreted or compiled differently than what appears below. To review, open the file in an editor that reveals hidden Unicode characters.
Learn more about bidirectional Unicode characters
Original file line number | Diff line number | Diff line change |
---|---|---|
@@ -0,0 +1,36 @@ | ||
# Gradient backward pass | ||
|
||
Use the pytorch framework allows the automatic differentiation of the PCA! | ||
|
||
The PCA transform method is always differentiable so it is always possible to | ||
compute gradient like that: | ||
|
||
```python | ||
pca = PCA() | ||
for ep in range(n_epochs): | ||
optimizer.zero_grad() | ||
out = neural_net(inputs) | ||
with torch.no_grad(): | ||
pca.fit(out) | ||
out = pca.transform(out) | ||
loss = loss_fn(out, targets) | ||
loss.backward() | ||
``` | ||
|
||
If you want to compute the gradient over the full PCA model (including the | ||
fitted `pca.n_components`), you can do it by using the "full" SVD solver | ||
and removing the part of the `fit` method that enforce the deterministic | ||
output by passing `determinist=False` in `fit` or `fit_transform` method. | ||
This part sort the components using the singular values and change their sign | ||
accordingly so it is not differentiable by nature but may be not necessary if | ||
you don't care about the determinism of the output: | ||
|
||
```python | ||
pca = PCA(svd_solver="full") | ||
for ep in range(n_epochs): | ||
optimizer.zero_grad() | ||
out = neural_net(inputs) | ||
out = pca.fit_transform(out, determinist=False) | ||
loss = loss_fn(out, targets) | ||
loss.backward() | ||
``` |
This file contains bidirectional Unicode text that may be interpreted or compiled differently than what appears below. To review, open the file in an editor that reveals hidden Unicode characters.
Learn more about bidirectional Unicode characters
Oops, something went wrong.